11am - 12 noon
Thursday 4 August 2022
Automatic Sign Language Production
PhD Viva Open Presentation by Stephanie Stoll
All Welcome!
Free
This event has passed
Speakers
Automatic Sign Language Production
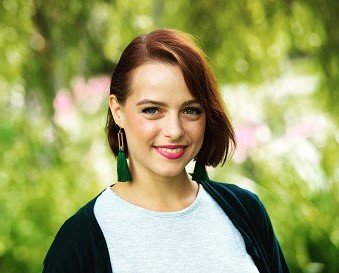
Abstract:
Sign Languages are the dominant form of communication used by the Deaf and Hard of Hearing (HoH). Whilst there are technologies to translate between the hearing and the Deaf and HoH, none of them provide the means for clear and easy communication. This is due to the asynchronous multi-channel nature of sign languages, which makes it a more complex translation problem, than translating between spoken languages. A common misconception is that a sign language is just a sign for word replacement and that written language is sufficient. However, a Deaf or HoH who was raised and educated in sign language might not be able to read the spoken language of their country well enough. Similarly, an avatar, driven using parametrised signs often produces incoherent and hard to understand signings. If it is driven using motion capture data, the avatar produces acceptable signings, but this approach is costly and not scalable. The research in this thesis addresses this problem by proposing data-driven, deep-learning-based Sign Language Production (SLP). In our first contribution chapter we introduce a novel approach to automatic SLP using recent developments in Neural Machine Translation (NMT), Generative Adversarial Networks (GANs), and motion generation. This preliminary system is capable of producing sign videos from spoken language sentences. Contrary to former approaches that are dependent on heavily annotated data, this approach requires minimal gloss and skeletal level annotations for training. We achieve this by breaking down the task into dedicated sub-processes. We first translate spoken language sentences into sign pose sequences by combining an NMT network with a Motion Graph (MG). The resulting pose information is then used to condition a generative model that produces photo realistic sign language video sequences. This is the first approach to continuous sign video generation that does not use an avatar. We evaluate the translation abilities of our approach on the PHOENIX14T Sign Language Translation dataset and set a baseline for text-to-gloss translation. We further demonstrate the video generation capabilities of our approach for both multi-signer and high-definition settings qualitatively and quantitatively using broadcast quality assessment metrics. In our second contribution chapter we focus on incorporating non-manuals (e.g. facial expres_sions, body and head pose) and increasing the resolution of produced utterances. We introduce a gloss2pose network architecture that is capable of generating human pose sequences conditioned on glosses. Combined with a generative adversarial pose2video network, we are able to produce natural-looking, high definition sign language video. For sign pose sequence generation, we outperform our previous contribution by a factor of 18, with a Mean Square Error of 1.0673 in pixels. For video generation we report superior results on three broadcast quality assessment metrics. To evaluate our full gloss-to-video pipeline we introduce two novel error metrics, to assess the perceptual quality and sign representativeness of generated videos. We present promising results, significantly outperforming the then state-of-the-art in both metrics. Our previous two contributions relied on gloss information. To make automatic SLP truly scalable this reliance needs to be eliminated. Hence, the final contribution chapter introduces the first method to automatically generate dense 3D sign sequences from text only. The approach requires simple 2D annotations for training, which can be automatically extracted from video. Rather than incorporating high-definition or motion capture data, we propose back-translation as a powerful paradigm for supervision: By first addressing the arguably simpler problem of translating 2D pose sequences to text, we can leverage this to drive a transformer-based architecture to translate text to 2D poses. These are then used to drive a 3D mesh generator. Our mesh generator P ose2Mesh uses temporal information, to enforce temporal coherence and significantly reduce processing time. The approach is evaluated by generating 2D pose, and 3D mesh sequences. An extensive quantitative and qualitative analysis of the approach and its sub-networks is conducted, reporting BLEU and ROUGE scores, as well as Mean 2D Joint Distance. Our proposed T ext2P ose model outperforms the current state-of-the-art in SLP, and we establish the first benchmark for the complex task of text-to-3D-mesh-sequence generation with our T ext2Mesh model.