10am - 11am GMT
Thursday 2 December 2021
Noisy web supervision for audio classification
PhD Viva Open Presentation by Turab Iqbal. All are welcome!
Free
This event has passed
Speakers
Abstract
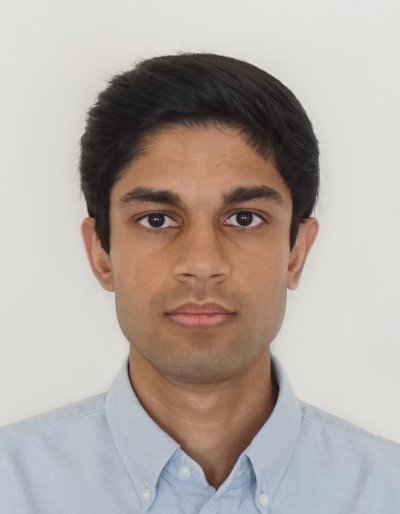
Audio classification and other fields of pattern recognition have developed at an astounding pace due to advances in machine learning. The availability of training data, especially labelled training data, remains an important factor for pushing the boundaries further. For this reason, there has been interest in utilising the massive amounts of annotated audio data on the web, which can be retrieved and labelled using automated procedures. Due to labelling errors (label noise) invariably being present, dataset curators have traditionally verified the data and labels manually. This has limited the amount of labelled data available, as manual verification is expensive. Motivated by a desire to remove this cost barrier, this thesis investigates training audio classifiers despite the presence of label noise. This work focuses on learning with web data in particular and in the context of training deep neural networks.
To study the effects of real-world label noise, experiments based on synthetic label noise are generally not appropriate. On the other hand, existing audio datasets do not facilitate running controlled experiments, which limits the analysis significantly. To address this, the first contribution of this thesis is a novel audio dataset called ARCA23K, which contains over \SI{23}{\kilo{}} labelled audio clips sourced from the web. Listening tests are carried out to characterise the label noise present in ARCA23K, revealing that most incorrectly-labelled audio clips are out-of-vocabulary (OOV). A wide array of experiments are conducted to study the impact of label noise on conventional neural network architectures and their learned representations.
After studying the effects of label noise on conventional neural networks, a compelling question is whether these effects can be mitigated. To this end, the second contribution is the development of a pseudo-labelling algorithm that automatically relabels training examples believed to be labelled incorrectly. While previous work in this area has only considered pseudo-labelling for in-vocabulary training examples, the work presented in this thesis specifically argues that learning with OOV examples can be beneficial if the examples are labelled appropriately. The proposed method uses confidence estimation for a data-driven approach to generating labels. Experiments are carried out to confirm the hypothesis and demonstrate the proposed method's superiority to other pseudo-labelling methods.
Finally, the third contribution is the proposal of a multi-task learning method for learning with noisy labels. More specifically, two tasks are formulated: one task is associated with clean examples and another task is associated with noisy examples. By separating clean and noisy examples in this manner, the effects of label noise can be isolated while also exploiting the overlap between the two tasks via joint training. The proposed multi-task learning approach introduces multiple components to enhance the performance further, including a regularisation technique based on consistency learning and the incorporation of pseudo-labelling. A key advantage of the proposed method is its resilience to overfitting on the noisy labels. Using this approach, it is shown that state-of-the-art performance can be attained.
Attend the event
This is a free online event open to everyone. You can attend via Zoom.