11am - 12 noon
Monday 8 November 2021
The role of Sketch in the 3D world
PhD open presentation for Anran Qi. All welcome!
Free
This event has passed
Abstract
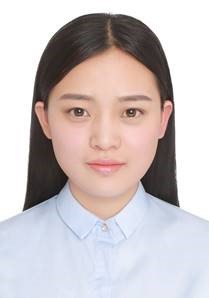
Sketching is a natural form of expression for people across cultures throughout human history. Especially in recent times, with the proliferation of touch-screen devices, free-hand sketch is much easier to obtain than ever before.
In this thesis, we explore the problem of sketch-based 3D retrieval in a coarse-to-fine manner, i.e., from category-level to instance-level to part-level retrieval. And show that sketch is especially powerful when communicating with the high-dimensional 3D world, where currently humans still have difficulty in interpreting its visuals and understanding how interaction occurs. The main challenge for category-level sketch-based 3D shape retrieval lies with the large domain gap between the 2D sketch and the 3D shape, since sketch and 3D shape have different shape representations with different dimensions. Most existing works attempt to overcome the domain gap by learning a joint feature embedding space to align the two domains. However, we observe that since both sketches and 3D shapes belong to the same set of object classes, their class label space are shared and when such a space is used as joint embedding space, perfect alignment is intrinsically achievable. Based on this observation, we propose to align them in their common class label space instead of the feature space.
Following this work, we start to think that is the category-level retrieval desirable? Since if given a specific chair sketch (eg, swivel) as input, it would be non-optimal to retrieve a 3D shape that is indeed a chair, but with different shape (e.g., H-shape stretcher). This motivates us to conduct the retrieval on an instance-level, so instead of returning any 3D chair, the system will yield just the one particular chair. This instance-level retrieval shares the challenges faced by category-level retrieval, on the other hand, the subtle intra-class differences need to be captured across domains as well. Based on this observation, we introduce the first two fine-grained sketch-based 3D shape retrieval datasets and propose to learn a deep joint embedding space that captures the fine-grained details.
Standing on superb fine-grained retrieval performance, we investigate on improving the user experience. As humans' perception of beauty are diverse, the 3D designs they wish for vary as well. Therefore, there might not exist the exact 3D shape in the dataset. On the other hand, it's time-consuming to design the 3D shapes, even for professional 3D shape designers. But it is highly possible that we could get a similar alternative by assembling suitable 3D parts in the repository models. This motivates us to dive into the task of sketch part-based 3D retrieval. It is worth noting that the sketch needs to be segmented into parts in order to conduct this retrieval. This emphasises the need for practical semantic sketch segmentation. We operate under one-shot setting, not requiring annotated dataset for training. This powers us the ability to label any amount of gallery sketches in a consistent manner by conforming to the same user-defined (personalised) semantic interpretation, and robustly to differences in drawing style and abstraction between the input and gallery sketches, given one input sketch that is annotated in part-level. To this end, we design a sketch-specific hierarchical deformation network to morph the one-shot sketch into gallery for effective personalised sketch segmentation.