11am - 12 noon BST
Wednesday 28 July 2021
Texture analysis in radiotherapy
PhD open viva presentation by Helen Yu Chi Wang. All are welcome!
Free
This event has passed
Speakers
Abstract
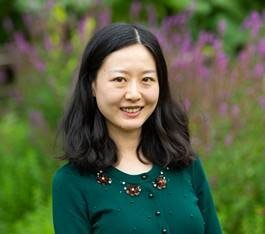
Since the adaptation of medical imaging as a standard clinical diagnostic tool, an ever-growing number of images has been taken. Radiomics took the big-data approach to extract quantitative features from medical images invisible to the naked eye to support clinical decision making, known as biomarkers. Over 440 radiomics features are available including size and shape based features, features derived from intensity histogram and texture features that describes the relationship between voxels. To date, there are no widely accepted biomarkers like TNM staging in oncology due to concerns on the robustness of features.
This thesis presents a detailed robustness study of 43 commonly used radiomics mainly 3-dimensional texture features extracted from the CT part of PET/CT images of patients diagnosed with non-small cell lung cancer (NSCLC) in two aspects: repeatability and reproducibility. Repeatability of features were studied using an open source dataset with 31 sets of repeat scans taken 15 minutes apart on quantisation parameters including quantisation method; levels of quantisation and the use of an intensity threshold. Reproducibility of features were studied using a locally acquired dataset of 50 scans on acquisition parameters including scanner model/make and the embedded reconstruction method; variation in tumour delineation and the afore mentioned quantisation parameters. The robust features were validated using the two independent dataset before highly correlated features were identified to reduce type one error caused by over-fitting. The robust texture features were applied to clinical applications from patient stratification to tumour delineation.
Features were extremely sensitive to changes in the quantisation parameters. Stability of features were improved by quantising with an intensity threshold and at high levels of quantisation such as 128. The group uniform quantiser (GUQ) produced the most stable results among the four quantisers tested. Using the same quantisation parameters, radiomics features were highly repeatable, 31/43 features had a Spearman's rank correlation ($r_{s}$) greater than 0.9 across all quantisers. Features were highly reproducible to scanner make/model and variation in tumour delineation under the same quantisation parameters. Features were not reproducible to different quantisation methods, using features quantised with GUQ at 128 intensity levels as a reference, 32 features correlated (rs>0.8) when quantised using other quantisers with a threshold, 26 features correlated when quantised using other quantisers without a threshold. 7 features correlated with tumour volume (rs>0.8). All features expect GLSZM GLN and ZSN features were successfully validated. The robust features were able to stratify patients and improve delineation accuracy in clinical applications.
Robustness and validation of 43 commonly used radiomics features were investigated from CT scans of patients with NSCLC. Features were extremely sensitive to quantisation parameters. Features were repeatable, reproducible and validated successfully using the same quantisation parameters. Based on the results of this study, it is suggested to quantise using GUQ at 128 intensity levels with a threshold.
Attend the event
This is a free online event open to everyone. You can attend via Zoom.