11am - 12 noon
Friday 5 February 2021
Delving deep into the sketch and photo relation
PhD Open Viva Presentation by PhD student: Conghui Hu.
Free
This event has passed
Abstract
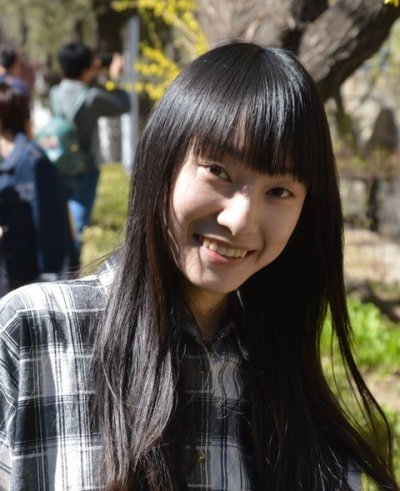
Sketches drawn by humans can play a similar role to photos in terms of conveying shape, posture as well as fine-grained information, and this fact has stimulated one line of cross-domain research that is related to sketch and photo, including sketch-based photo synthesis and retrieval. In this thesis, we aim to further investigate the relationship between sketch and photo. More specifically, we study certain under-explored traits in this relationship, and propose novel applications to reinforce the understanding of sketch and photo relation.
Our exploration starts with the problem of sketch-based photo synthesis, where the unique trait of non-rigid alignment between sketch and photo is overlooked in existing research. We then carry on with our investigation from a new angle to study whether sketch can facilitate photo classifier generation. Building upon this, we continue to explore how sketch and photo are linked together on a more fine-grained level by tackling with the sketch-based photo segmenter prediction. Furthermore, we address the data scarcity issue identified in nearly all sketch-photo-related applications by examining their inherent correlation in the semantic aspect using sketch-based image retrieval (SBIR) as a test-bed. In general, we make four main contributions to the research on relationship between sketch and photo.
Firstly, to mitigate the effect of deformation in sketch-based photo synthesis, we introduce the spatial transformer network to our image-image regression framework, which subtly deals with non-rigid alignment between the sketches and photos. The qualitative and quantitative experiments consistently reveal the superior quality of our synthesised photos over those generated by existing approaches.
Secondly, sketch-based photo classifier generation is achieved with a novel model regression network, which maps the sketch to the parameters of photo classification model. It is shown that our model regression network is able to generalise across categories and photo classifiers for novel classes not involved in training are just a sketch away. Comprehensive experiments illustrate the promising performance of the generated binary and multi-class photo classifiers, and demonstrate that sketches can also be employed to enhance the granularity of existing photo classifiers.
Thirdly, to achieve the goal of sketch-based photo segmentation, we propose a photo segmentation model generation algorithm that predicts the weights of a deep photo segmentation network according to the input sketch. The results confirm that one single sketch is the only prerequisite for unseen category photo segmentation, and the segmentation performance can be further improved by utilising sketch that is aligned with the object to be segmented in shape and position.
Finally, we present an unsupervised representation learning framework for SBIR, the purpose of which is to eliminate the barrier imposed by data annotation scarcity. Prototype and memory bank reinforced joint distribution optimal transport is integrated into the unsupervised representation learning framework, so that the mapping between the sketches and photos could be automatically detected to learn a semantically meaningful yet domain-agnostic feature space. Extensive experiments and feature visualisation validate the efficacy of our proposed algorithm.
Attend the seminar
You can join the seminar via Zoom.