2pm - 3pm
Thursday 15 October 2020
Sketch abstraction
PhD Open Viva Presentation by Umar Riaz Muhammad. All are welcome!
Free
This event has passed
Abstract
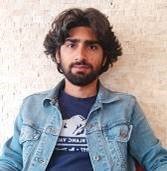
Sketching is an intuitive process which has been used throughout human history as a communication tool. Due to the recent proliferation of touch-screen devices, free-hand sketching has now become more pervasive than ever before: sketches can be drawn at anytime and anywhere on a smartphone using one’s finger. With this mass usage and an imminent commercial potential, amateur human free-hand sketching has attracted increasing attention from the research community.
Various sketch related problems have been studied, including sketch recognition, sketch based image retrieval, and sketch synthesis. A fundamental challenge in analyzing free-hand sketches is that sketches drawn by different people for the same object category/instance often differ significantly, especially in their levels of abstraction. It does not seem to matter if a particular sketch is drawn out of memory, given the object name or it is drawn using a reference photo.
However, we notice that detailed sketches with low levels of abstraction are easily recognizable, but as the abstraction level increases and the details in the depicted sketches become scarce, it becomes harder to recognize the object in the sketches. Furthermore, the variations of abstraction level can also be influenced by what an individual person perceives as salient in the context of a particular goal or objective. The peculiarity of sketches in fact consists of their sparse and abstract representation which highlights specific visual features while downplaying others.
Depending on what the goal or the objective is, different parts of the sketch may be drawn with prominence while others are reduced or completely removed. Another peculiar characteristic of free-hand sketches is that they are distinctively different to photos. Photos are static and pixel-perfect two-dimensional visual representations of the world (e.g. scenes, objects). Sketches on the other hand are the result of a dynamic drawing process consisting of a sequence of strokes. While it is possible to represent a sketch as a 2D pixel image, the direct adaptation of models initially proposed for 2D photos often fail or provide poor results due to the sparse nature of sketches with no colour or texture information to exploit. This underlines the need for sketch-specific solutions that can exploit sketch-specific characteristics to solve a sketch-specific problem such as abstraction.
In this work we tackle the problem of sketch abstraction by first defining it as a process of trade-off between recognizability and brevity/compactness (number of strokes). Based on this insight, we propose two distinct approaches to solve the abstraction problem: elimination-based abstraction (EA) and selection-based abstraction (SA). In the first approach the EA model learns to find the most compact subset of input strokes for which the sketch is still recognizable. It does so by observing parts of the sketch (strokes) one by one following the original human drawing order, and deciding for each part whether it should be kept or removed from the abstracted output sketch.
The impact of any given stroke removal on recognizability is interdependent on which other strokes are kept/removed. We thus model this dependency as a sequential decision making problem described by the Markov Decision Process (MDP). In the second approach the objective is again to find a compact subset of strokes for which the sketch is still recognizable, but this is achieved by applying an inverse strategy to the EA approach. Here we aim to learn which strokes should be selected, rather than removed, from the input sketch to form the most compact abstracted sketch representation that doesn’t lose its recognizability.
The input is processed holistically, rather than being constrained by the original order, and the output is built sequentially by picking one stroke among all the available strokes at each time step. In both approaches the sequential decision process is formalized as the Markov Decision Process (MDP), which is trained with the reinforcement learning (RL) framework due to the lack of relevant stroke-level annotation.
We introduce a novel goal-driven abstraction task, that aims to extract/preserve particular parts of the input sketch according to a specific goal. This implies that the same input can output a different abstracted sketch depending on the abstraction goal. Due to the particular RL based architecture of the aforementioned approaches for sketch abstraction (EA and SA), the goal-driven sketch abstraction task can easily be amalgamated with these proposed methods by solely adjusting the reward function.
We also explore the potential of applying the abstraction problem and the proposed solutions to other sequential domains, such as videos and text. We do this by following the same problem formulation and model architecture of the EA/SA approaches proposed for sketches, with the substitution of stroke (coordinates) data with video-shots (frames) and sentences (words).
Finally, we explore the potential of combining sketch abstraction and synthesis tasks, so that a model can not only autonomously generate a sketch of given visual concept but can also achieve recognizable rendition with very few strokes. In order to effectively evaluate the performance of such a drawing agent, we set up a Pictionary-like game that we name Pixelary, where the competitors are pixel-synchronized instead of time. We imagine a common game scenario where two parties (our drawing agent and a human competitor) simultaneously sketch a common visual concept – stroke-by-stroke – with a judge in the background constantly guessing, and the winner is the one whose sketch the judge recognizes first. In achieving this goal, we importantly advance the line of sketch research by contributing an agent that not only knows how to sketch – but more importantly masters the dynamics of sketching, so as to surpass humans at conveying meaning quickly.
For all the aforementioned tasks, we perform thorough experiments on various publicly available datasets. The quantitative and qualitative results are reported to demonstrate the effectiveness of all the proposed solutions.