10am - 11am
Thursday 3 September 2020
Adaptation and generalization across domains in visual recognition with deep neural networks
PhD Viva Open Presentation by Kaiyang Zhou. All welcome.
Free
This event has passed
Abstract
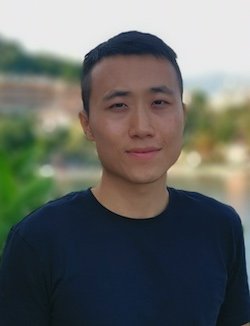
Visual recognition has been greatly advanced in recent years with the emergence of convolutional neural networks (CNNs). With large-scale, manually labeled datasets, CNN-based recognition models have demonstrated huge success on many computer vision tasks, such as image classification, semantic segmentation and facial recognition.
However, most learning algorithms assume training and test data are drawn from the same data distribution, which is often violated in practice where test data comes from a different distribution. The problem is known as domain shift, which could lead to significant performance drops.
The domain shift problem is usually studied under two settings: domain generalization (DG) and unsupervised domain adaptation (UDA). DG aims to leverage source data composed of multiple domains to learn a domain-invariant model, while UDA exploits unlabeled target data along with abundant labeled source data for model adaptation. This thesis mainly focuses on multi-source scenarios.
In this thesis, three contributions are made to address domain shift. First, we investigate domain shift in instance recognition, which is largely overlooked by existing work. Specifically, we focus on person re-identification (re-ID), a cross-domain instance matching problem, and propose a novel CNN architecture termed omni-scale network (OSNet) to learn generalizable, omni-scale features. OSNet not only achieves state-of-the-art for same-dataset re-ID, but also generalizes well when deployed across different re-ID datasets.
Second, a data augmentation-based DG approach is proposed where a neural network is learned to generate images from unseen domains. The learning objective is formulated in such a way that the generated images can be recognized by a label classifier but fool a domain classifier. Extensive experiments on a wide range of DG datasets demonstrate the effectiveness of this approach.
Finally, we introduce a collaborative ensemble learning approach, which exploits complementarity between source domains to train a multi-expert ensemble that is robust to domain shift in unseen domains. Unlabeled target data is also handled by pseudo-labeling. This approach can be used for both DG and UDA settings, thus providing a versatile framework to tackle domain shift in practice.