Surrogate based runtime difference mitigation in asynchronous multi-disciplinary search tasks
Start date
January 2019End date
December 2021Summary
Bayesian approaches to the optimisation of complex systems have attracted much research in recent years and have achieved encouraging success.
The project has mainly two aims:
- Develop new training algorithms and new optimisation methods that can deal with very low amount of training data for surrogate models and optimisation evaluations.
- Develop new infill criteria for Bayesian approaches to optimisation which integrate multiple models for estimating different criteria of a multi-objective problem or constraints.
Funding amount
£148,429
Funder
Team
Principal Investigator
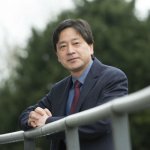
Professor Yaochu Jin
Distinguished Chair, Head of the NICE Group, Director of Research
See profile