Predictive modelling of the social networks influencing obesity
Start date
03 January 2012End date
21 December 2012Overview
The incidence of obesity in the UK has risen significantly with treatment costs rising to over £5 billion by 2025. The many social and biological causes of obesity have been explored in the Foresight obesity system map. Although useful for illustration, the qualitative nature and complexity of this map have prevented consensus on targets for public health intervention.
In this project we will apply, for the first time, dynamic neural simulations to quantitatively model the social parameters influencing the increase in obesity rates to help identify variables best targeted by public health interventions.
Aims and objectives
The aim of this project is to develop a spiking neural network model to quantify the social parameters influencing the increase in overweight and obesity in the UK over the last 30 years.
Specific objectives are to:
- Identify key social parameters from the Foresight qualitative map (e.g. watching television, walking to school, media) and obtain the relevant epidemiological data from the Economic and Social Data Service and map to network.
- Develop network models using these parameters to enable measurement of the spatial dynamics of the interactions between individuals observed.
- Quantify outcomes, specifically: the emergence of polychronous groups, the average firing rates and the connectivity pattern.
- Future expansion could include other parameters within Foresight and would focus on likely parameters for public health intervention.
This project clearly meets the MILES assessment criteria in initiating a multidisciplinary collaboration between biologists, computer scientists and mathematicians. The research planned is an entirely novel exploitation of the dynamics of spiking neural networks in the context of how social parameters influence the development of obesity.
The modelling of connectivity, influence and information flow within this system will be predictive and therefore offer the potential of identifying critical nodes for public health intervention. As such this project offers tremendous potential to lead to a successful bid securing external funding to continue this work.
Additional information
In 2007 24 per cent of adults and 17 per cent of children in the UK were obese; the estimated costs of treating the consequences of obesity were £1 billion in 2002 and projected to be £5.3 billion by 2025 (NHS Information Centre, Lifestyle Statistics, 2009). Numerous social and biological factors have been implicated in the aetiology of obesity, and in 2007 the Foresight Programme of the UK Government Office for Science published a conceptual obesity system map that illustrates the multifactorial nature of this disease. With 108 variables this qualitative atlas highlights the tremendous complexity underlying obesity and the need to move away from single intervention approaches. However, this very complexity and the qualitative nature of the map have also prevented the development of a consensus on where public health interventions are most likely to make a difference.
At first glance, an unlikely correlate of this multifactorial, highly interconnected and complex network of influences is the human brain. The complexities of the brain have been studied at many levels, from chemical reactions in individual neurons through to high-level cognitive architectures. These studies have demonstrated that small-scale behaviour, such as when and how neurons fire, can have a large-scale influence on a population. However, it is only recently that advances in modelling the dynamics of neural interactions have led to an increase in the scale of models that can be developed (Izhikevich & Edelman, 2008). In these models, neurons are represented by biologically plausible dynamic equations, with each neuron highly connected with others. By stimulating a handful of neurons over time, behaviour emerges from the model in the form of polychronous groups: Repeating patterns of neurons that learn to activate together. These patterns result from the stimulation but demonstrate the wide influence that single inputs can establish.
These emergent patterns of behaviour may, by analogy, represent the dissemination of social influences and consequent changes in behaviour. For example, we can treat neurons as people within a population who are highly connected to others. An increase by one person in, say, watching television, modelled as a pattern of neural activity, will influence those to whom the person is connected. Over time, this influence causes the emergence of polychronous groups tuned to this pattern of activity, and hence a wider number of individuals have been influenced. The challenges are identifying social parameters that we can model and developing a plausible architecture for the influences of the parameters to be observed. With the computational efficiency of these spiking neural networks, many such parameters and architectures can be evaluated and compared with the known increase in obesity of the last 30 years in the UK to establish a benchmark. We can then test different parameter values to find target interventions.
Bringing together a team of biologists, computer scientists and mathematicians, this project aims to develop a spiking neural network model to quantify key social parameters from the Foresight qualitative map influencing the increase in overweight and obesity in the UK over the last 30 years.
E.M. Izhikevich & G.M. Edelman (2008) Large-scale model of mammalian thalamocortical systems, PNAS, 105(9):3593-3598.
Funding amount
£650,305
Funder
Team
Collaborators
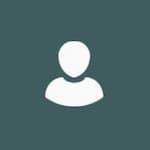
Dr Theresa Hague
Postgraduate Medical School, Faculty of Health and Medical Sciences