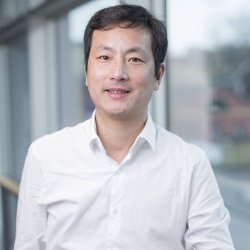
Professor Yi Ma
Academic and research departments
Institute for Communication Systems, School of Computer Science and Electronic Engineering.About
Biography
Dr. Yi Ma is a Chair Professor at the Institute for Communication Systems (ICS), the Home of 6G Innovation Centre (6GIC). He has extensive expertise in the area of Signal Processing, Deep Learning and Information Theory, with their applications in Wireless Communication, Positioning and Sensing.
ResearchResearch interests
- Semantic/effective communication and sensing
- Machine learning for future RF and physical layer design
- Transceiver optimization for future communication systems such as URLLC.
- Scalable Distributed MIMO technology
- Opportunistic networking and cooperative communications
- Hybrid data fusion and machine learning for mobile localization
- Estimation, detection and synchronization
- Information theory and coding
Research projects
- 2025-2029 ICON (UKRI-EPSRC), Co-I
- 2024-2028 ISAC (Industry fund), Co-I
- 2023-2025 Deep Learning for Digital Pre-distortion (industry fund), PI
- 2024-2025 Opportunistic perturbation-coded modulation (Industry fund), PI
- 2022-2025 Space MIMO (Industry fund), PI
- 2023-2025 TUDOR (DSIT fund), Co-I
- 2022-2023 Nested polar code (industry fund), Co-I
- 2020-2023 Large massive-MIMO (Industry fund), PI
- 2017-2021 Artificial intelligence for future communications (Industry fund), PI
- 2013-2016 RESCUE (FP7 ICT Consortium), PI
- 2013-2014 LTE Machine-Type Communications: Phase II (Industry fund), Co-I
- 2012-2013 LTE Machine-Type Communications: Phase I (Industry fund), Co-I
- 2010-2013 WHERE2 (FP7 ICT Consortium), PI
- 2011-2012 Wi-Fi Indoor Positioning (EPSRC), Co-PI
- 2010-2013 EXALTED (FP7 ICT Consortium), Co-I
- 2007-2010 WHERE (FP7 ICT Consortium), PI
- 2005-2007 WINNER2 (FP6 ICT Consortium)
- 2004-2005 4MORE (FP6 ICT Consortium)
- 2006-2009 Mobile VCE-Core 4 (EPSRC)
PhD Position
I am constantly looking for well self-motivated PhD candidates with excellent background in Physics, Mathematics, Wireless Communications, or Computer Science. Prior to submit your application, please make sure you have met the following University requirements :
- A 1st class BSc degree or MSc with a Distinction (or equivalent to top 10% internationally).
- A good research proposal (if ICS funding support is requested, please clearly indicate why the proposed research should be financially supported by the ICS).
- For international students, it is essential to meet the University's English requirements (IELTS 6.5 or above (overall) with each section of 6.0 or above).
Research interests
- Semantic/effective communication and sensing
- Machine learning for future RF and physical layer design
- Transceiver optimization for future communication systems such as URLLC.
- Scalable Distributed MIMO technology
- Opportunistic networking and cooperative communications
- Hybrid data fusion and machine learning for mobile localization
- Estimation, detection and synchronization
- Information theory and coding
Research projects
- 2025-2029 ICON (UKRI-EPSRC), Co-I
- 2024-2028 ISAC (Industry fund), Co-I
- 2023-2025 Deep Learning for Digital Pre-distortion (industry fund), PI
- 2024-2025 Opportunistic perturbation-coded modulation (Industry fund), PI
- 2022-2025 Space MIMO (Industry fund), PI
- 2023-2025 TUDOR (DSIT fund), Co-I
- 2022-2023 Nested polar code (industry fund), Co-I
- 2020-2023 Large massive-MIMO (Industry fund), PI
- 2017-2021 Artificial intelligence for future communications (Industry fund), PI
- 2013-2016 RESCUE (FP7 ICT Consortium), PI
- 2013-2014 LTE Machine-Type Communications: Phase II (Industry fund), Co-I
- 2012-2013 LTE Machine-Type Communications: Phase I (Industry fund), Co-I
- 2010-2013 WHERE2 (FP7 ICT Consortium), PI
- 2011-2012 Wi-Fi Indoor Positioning (EPSRC), Co-PI
- 2010-2013 EXALTED (FP7 ICT Consortium), Co-I
- 2007-2010 WHERE (FP7 ICT Consortium), PI
- 2005-2007 WINNER2 (FP6 ICT Consortium)
- 2004-2005 4MORE (FP6 ICT Consortium)
- 2006-2009 Mobile VCE-Core 4 (EPSRC)
PhD Position
I am constantly looking for well self-motivated PhD candidates with excellent background in Physics, Mathematics, Wireless Communications, or Computer Science. Prior to submit your application, please make sure you have met the following University requirements :
- A 1st class BSc degree or MSc with a Distinction (or equivalent to top 10% internationally).
- A good research proposal (if ICS funding support is requested, please clearly indicate why the proposed research should be financially supported by the ICS).
- For international students, it is essential to meet the University's English requirements (IELTS 6.5 or above (overall) with each section of 6.0 or above).
Supervision
Postgraduate research supervision
Current PhD Students:
- Yupeng Zheng: Future MIMO technology (ELAA)
- Ashkan Jafari: ISAC MAC protocol
- Zohre M. Bakhsh (transfered): Space MIMO link layer design
Ex-PhD Students:
- Hongju Liu (04 - 08): Channel estimation for multicarrier transmissions
- Yuanyuan Zhang (06 - 10): Adaptive cooperative relays
- Mohammad Movahhedian (07 - 10): Frequency synchronization for multiuser multicarrier transmissions.
- Parisa Cheraghi (09 -12): Advanced spectrum sensing techniques
- Ziming He (08 - 12): Advanced mobile positioning and tracking techniques
- Hui Luo (07 - 11): Cooperative communications for satellite systems
- Zhengwei Lu (09 -13): Pilot-assisted fast spectrum sensing techniques
- Jiancao Hou (10 -14): Advanced multiuser-MIMO transmitter design
- Chuyi Qian (10 -14): Opportunistic relaying protocols
- Erik Yngvesson (13 -17): Coexistence of Massive MIMO in unlicensed bands
- Juan Carlos De Luna Ducoing (14-17): Advanced modulations for scalable multiuser MIMO
- Abdullah Alonazi (11-16): Less-calibrated indoor mobile localization
- Guangyi Wang (12-17): Estimation of pilot contaminated channels
- Raouf Yamani (15 - 17): Low-complexity vector perturbation for MIMO nonlinear precoding
- Songyan Xue (17 - 21): Deep learning for future modem design
- Ang Li (18 - 22): Deep learning for NOMA/multi-carrier transmissions
- Lifu Liu (18 - 22): Low-cost mmWave solutions
- Jinfei Wang (19 - 23): MIMO URLLC
- Jiuyu Liu (20-24): Future MIMO technology (ELAA) and channel modelling
- Siqi Zhang (20-24): Goal-oriented semantic communication
- Ryan I Fernandez (24-25): LEO-satellite MIMO constellation design (transferred)
Teaching
- EEEM017: Fundamentals of Mobile Communications
- EEE3006: Digital Communications
- Personal and tutorial tutor for undergraduate students.
- EEE examination officer
Publications
In this paper, a novel end-to-end learning approach, namely JTRD-Net, is proposed for uplink multiuser single-input multiple-output (MU-SIMO) joint transmitter and non-coherent receiver design (JTRD) in fading channels. The basic idea lies in the use of artificial neural networks (ANNs) to replace traditional communication modules at both transmitter and receiver sides. More specifically, the transmitter side is modeled as a group of parallel linear layers, which are responsible for multiuser waveform design; and the non-coherent receiver is formed by a deep feed-forward neural network (DFNN) so as to provide multiuser detection (MUD) capabilities. The entire JTRD-Net can be trained from end to end to adapt to channel statistics through deep learning. After training, JTRD-Net can work efficiently in a non-coherent manner without requiring any levels of channel state information (CSI). In addition to the network architecture, a novel weight-initialization method, namely symmetrical-interval initialization, is proposed for JTRD-Net. It is shown that the symmetrical-interval initialization outperforms the conventional method (e.g. Xavier initialization) in terms of well-balanced convergence-rate among users. Simulation results show that the proposed JTRD-Net approach takes significant advantages in terms of reliability and scalability over baseline schemes on both i.i.d. complex Gaussian channels and spatially-correlated channels.
Generative foundation models can revolutionize the design of semantic communication (SemCom) systems allowing high fidelity exchange of semantic information at ultra low rates. In this work, a generative SemCom framework with pretrained foundation models is proposed, where both uncoded forward-with-error and coded discard-with-error schemes are developed for the semantic decoder. To characterize the impact of transmission reliability on the perceptual quality of the regenerated signal, their mathematical relationship is analyzed from a rate-distortion-perception perspective, which is proved to be non-decreasing. The semantic values are defined to measure the semantic information of multimodal semantic features accordingly. We also investigate semantic-aware power allocation problems aiming at power consumption minimization for ultra low rate and high fidelity SemComs. To solve these problems, two semantic-aware power allocation methods are proposed by leveraging the non-decreasing property of the perception-error relationship. Numerically, perception-error functions and semantic values of semantic data streams under both schemes for image tasks are obtained based on the Kodak dataset. Simulation results show that our proposed semanticaware method significantly outperforms conventional approaches, particularly in the channel-coded case (up to 90% power saving).
— In this paper, it is identified that lowering the reference level at the vector signal analyzer can significantly improve the performance of iterative learning control (ILC). We present a mathematical explanation for this phenomenon, where the signals experience logarithmic transform prior to analogue-to-digital conversion, resulting in non-uniform quantization. This process reduces the quantization noise of low-amplitude signals that constitute a substantial portion of orthogonal frequency division multiplexing (OFDM) signals, thereby improving ILC performance. Measurement results show that compared to setting the reference level to the peak amplitude, lowering the reference level achieves 3 dB improvement on error vector magnitude (EVM) and 15 dB improvement on normalized mean square error (NMSE) for 320 MHz WiFi OFDM signals.
This paper explores the concept of information importance in multi-modal task-oriented semantic communication systems, emphasizing the need for high accuracy and efficiency to fulfill task-specific objectives. At the transmitter, generative AI (GenAI) is employed to partition visual data objects into semantic segments, each representing distinct, task-relevant information. These segments are subsequently encoded into tokens, enabling precise and adaptive transmission control. Building on this framework, we present importance-aware source and channel coding strategies that dynamically adjust to varying levels of significance at the segment, token, and bit levels. The proposed strategies prioritize high fidelity for essential information while permitting controlled distortion for less critical elements, optimizing overall resource utilization. Furthermore, we address the source-channel coding challenge in semantic multiuser systems, particularly in multicast scenarios, where segment importance varies among receivers. To tackle these challenges, we propose solutions such as rate-splitting coded progressive transmission, ensuring flexibility and robustness in task-specific semantic communication.
Generative foundation models can revolutionize the design of semantic communication (SemCom) systems allowing high fidelity exchange of semantic information at ultra low rates. In this work, a generative SemCom framework with pretrained foundation models is proposed, where both uncoded forward-with-error and coded discard-with-error schemes are developed for the semantic decoder. To characterize the impact of transmission reliability on the perceptual quality of the regenerated signal, their mathematical relationship is analyzed from a rate-distortion-perception perspective, which is proved to be non-decreasing. The semantic values are defined to measure the semantic information of multimodal semantic features accordingly. We also investigate semantic-aware power allocation problems aiming at power consumption minimization for ultra low rate and high fidelity SemComs. To solve these problems, two semantic-aware power allocation methods are proposed by leveraging the non-decreasing property of the perception-error relationship. Numerically, perception-error functions and semantic values of semantic data streams under both schemes for image tasks are obtained based on the Kodak dataset. Simulation results show that our proposed semanticaware method significantly outperforms conventional approaches, particularly in the channel-coded case (up to 90% power saving).
—This paper proposes a multi-stage position and velocity estimation algorithm in the context of near-field (NF) integrated sensing and communications (ISAC). We consider colocated multiple-input multiple-output (MIMO) orthogonal frequency-division multiplexing (OFDM) system. Both wavefront curvature in NF and wideband feature of OFDM are utilized to jointly estimate the range and the angle of the targets. The proposed algorithm relies on spectral estimation methods and maximum likelihood (ML) refinement steps for target sensing. Also, the estimation performance is compared with the Cramér-Rao lower bound (CRLB). Given the initial estimations of the targets' position and mobility parameters through our proposed algorithm, we further develop a precoding (i.e., beamfocusing) design. The proposed design exploits the finite beam depth and width in NF with minimal beam cross-correlation of different targets across both range and angular domains, allowing better overall resolution. The precoding design also accounts for a trade-off behavior between communication and sensing performance.
—In this paper, a novel environmental mapping method is proposed to outline the indoor layout utilizing the line-of-sight (LoS) state information of extremely large aperture array (ELAA) channels. It leverages the spatial resolution provided by ELAA and the mobile terminal (MT)'s mobility to infer the presence and location of obstacles in the environment. The LoS state estimation is formulated as a binary hypothesis testing problem, and the optimal decision rule is derived based on the likelihood ratio test. Subsequently, the theoretical error probability of LoS estimation is derived, showing close alignment with simulation results. Then, an environmental mapping method is proposed, which progressively outlines the layout by combining LoS state information from multiple MT locations. It is demonstrated that the proposed method can accurately outline the environment layout, with the mapping accuracy improving as the number of service-antennas and MT locations increases. This paper also investigates the impact of channel estimation error and non-LoS (NLoS) components on the quality of environmental mapping. The proposed method exhibits particularly promising performance in LoS dominated wireless environments characterized by high Rician K-factor. Specifically, it achieves an average intersection over union (IoU) exceeding 80% when utilizing 256 service antennas and 18 MT locations. Index Terms—Extremely large aperture array (ELAA), integrated sensing and communication (ISAC), environmental mapping , line-of-sight (LoS) state, binary hypothesis testing.
—This paper presents a novel framework for importance-aware adaptive data transmission, designed specifically for real-time computer vision (CV) applications where task-specific fidelity is critical. An importance-weighted mean square error (IMSE) metric is introduced, assigning data importance based on bit positions within pixels and semantic relevance within visual segments, thus providing a task-oriented measure of reconstruction quality. To minimize IMSE under total power constraints, a data-importance-aware waterfilling approach is proposed to optimally allocate transmission power according to data importance and channel conditions. Simulation results demonstrate that the proposed approach significantly outper-forms margin-adaptive waterfilling and equal power allocation strategies, achieving more than 7 dB and 10 dB gains in normalized IMSE at high SNRs (> 10 dB), respectively. These results highlight the potential of the proposed framework to enhance data efficiency and robustness in real-time CV applications , especially in bandwidth-limited and resource-constrained environments.
—Deep learning (DL)-based channel state information (CSI) feedback has shown great potential in improving spectrum efficiency in massive MIMO systems. However, DL models optimized for specific environments often experience performance degradation in others due to model mismatch. To overcome this barrier in the practical deployment, we propose UniversalNet, an ID-photo-inspired universal CSI feedback framework that enhances model generalizability by standardizing the input format across diverse data distributions. Specifically, UniversalNet employs a standardized input format to mitigate the influence of environmental variability, coupled with a lightweight sparsity-aligning operation in the transformed sparse domain and marginal control bits for original format recovery. This enables seamless integration with existing CSI feedback models, requiring minimal modifications in preprocessing and postpro-cessing without updating neural network weights. Furthermore, we propose an efficient eigenvector joint optimization method to enhance the sparsity of the precoding matrix by projecting the channel correlation into the eigenspace, thus improving the implicit CSI compression efficiency. Test results demonstrate that UniversalNet effectively improves generalization performance and ensures precise CSI feedback, even in scenarios with limited training diversity and previously unseen CSI environments.
In this paper, we address a physical layer security (PLS) framework for the integrated sensing and semantic communication (ISASC) system, where a multi-antenna dualfunction semantic base station serves multiple single-antenna semantic communication users (SCUs) and monitors a malicious sensing target (MST), in the presence of a single-antenna eavesdropper (EVE), with both the MST and EVE aiming to wiretap information from the SCUs’ signals. To enhance PLS, we employ joint artificial noise (AN) and dedicated sensing signal (DSS) in addition to wiretap coding. To evaluate the sensing accuracy, we derive the Cramer-Rao bound (CRB) as a ´ function of the communication, sensing, and AN beamforming (BF) vectors. Subsequently, to assess the PLS level of the ISASC system, we determine a closed-form expression for the semantic secrecy rate (SSR). To achieve an optimal trade-off region between these two competing objectives, we formulate a multi-objective optimization problem for the joint design of the BF vectors. We apply semi-definite programming, Gaussian randomization method, and golden-section search techniques to address this problem. Simulation results demonstrate that the proposed scheme outperforms baseline sc
In vehicle-to-infrastructure (V2I) networks, a cluster of multi-antenna access points (APs) can collaboratively conduct transmitter beamforming to provide data services (e.g., eMBB or URLLC). The collaboration between APs effectively forms a networked linear antenna-array with extra-large aperture (i.e., network-ELAA), where the wireless channel exhibits spatial non-stationarity. Major contribution of this work lies in the analysis of beamforming gain and radio coverage for network-ELAA non-stationary Rician channels considering the AP clustering. Assuming that: 1) the total transmit-power is fixed and evenly distributed over APs, 2) the beam is formed only based on the line-of-sight (LoS) path, it is found that the beamforming gain is concave to the cluster size. The optimum size of the AP cluster varies with respect to the user's location, channel uncertainty as well as data services. A user located farther from the ELAA requires a larger cluster size. URLLC is more sensitive to the channel uncertainty when comparing to eMBB, thus requiring a larger cluster size to mitigate the channel fading effect and extend the coverage. Finally, it is shown that the network-ELAA can offer significant coverage extension (50% or more in most of cases) when comparing with the single-AP scenario.
In this paper, a novel nonlinear precoding (NLP) technique, namely constellation-oriented perturbation (COP), is proposed to tackle the scalability problem inherent in conventional NLP techniques. The basic concept of COP is to apply vector perturbation (VP) in the constellation domain instead of symbol domain; as often used in conventional techniques. By this means, the computational complexity of COP is made independent to the size of multi-antenna (i.e., MIMO) networks. Instead, it is related to the size of symbol constellation. Through widely linear transform, it is shown that COP has its complexity flexibly scalable in the constellation domain to achieve a good complexityperformance tradeoff. Our computer simulations show that COP can offer very comparable performance with the optimum VP in small MIMO systems. Moreover, it significantly outperforms current sub-optimum VP approaches (such as degree-2 VP) in large MIMO whilst maintaining much lower computational complexity.
This paper aims to handle the joint transmitter and noncoherent receiver design for multiuser multiple-input multiple-output (MU-MIMO) systems through deep learning. Given the deep neural network (DNN) based noncoherent receiver, the novelty of this work mainly lies in the multiuser waveform design at the transmitter side. According to the signal format, the proposed deep learning solutions can be divided into two groups. One group is called pilot-aided waveform, where the information-bearing symbols are time-multiplexed with the pilot symbols. The other is called learning-based waveform, where the multiuser waveform is partially or even completely designed by deep learning algorithms. Specifically, if the information-bearing symbols are directly embedded in the waveform, it is called systematic waveform. Otherwise, it is called non-systematic waveform, where no artificial design is involved. Simulation results show that the pilot-aided waveform design outperforms the conventional zero forcing receiver with least squares (LS) channel estimation on small-size MU-MIMO systems. By exploiting the time-domain degrees of freedom (DoF), the learning-based waveform design further improves the detection performance by at least 5 dB at high signal-to-noise ratio (SNR) range. Moreover, it is found that the traditional weight initialization method might cause a training imbalance among different users in the learning-based waveform design. To tackle this issue, a novel weight initialization method is proposed which provides a balanced convergence performance with no complexity penalty.
This paper presents a parallel computing approach that is employed to reconstruct original information bits from a non-recursive convolutional codeword in noise, with the goal of reducing the decoding latency without compromising the performance. This goal is achieved by means of cutting a received codeword into a number of sub-codewords (SCWs) and feeding them into a two-stage decoder. At the first stage, SCWs are decoded in parallel using the Viterbi algorithm or equivalently the brute force algorithm. Major challenge arises when determining the initial state of the trellis diagram for each SCW, which is uncertain except for the first one; and such results in multiple decoding outcomes for every SCW. To eliminate or more precisely exploit the uncertainty, an Euclidean-distance minimization algorithm is employed to merge neighboring SCWs; and this is called the merging stage, which can also run in parallel. Our work reveals that the proposed two-stage decoder is optimal and has its latency growing logarithmically, instead of linearly as for the Viterbi algorithm, with respect to the codeword length. Moreover, it is shown that the decoding latency can be further reduced by employing artificial neural networks for the SCW decoding. Computer simulations are conducted for two typical convolutional codes, and the results confirm our theoretical analysis.
Quantization is the characterization of analogue-to-digital converters (ADC) in massive MIMO systems. The design of quantization function or quantization thresholds is found to relate to quantization step, which is the factor that adapts with the changing of transmit power and noise variance. With the objective of utilizing low-resolution ADC is reducing the cost of massive MIMO, we propose an idea as if it is necessary to have adaptive-threshold quantization function. It is found that when maximum-likelihood (ML) is employed as the detection method, having quantization thresholds fixed for low-resolution ADCs will not cause significant performance loss. Moreover, such fixed-threshold quantization function does not require any information of signal power which can reduce the hardware cost of ADCs. Simulations have been carried out in this paper to make comparisons between fixed-threshold and adaptive-threshold quantization regarding various factors.
Detecting high-resolution signals at binary-array receivers can yield a stochastic-resonance phenomenon. It is found, through mathematical means, that the error probability of maximum-likelihood detection (MLD) forms a convex function of the SNR; and the optimum operating-SNR increases monotonically with the signal resolution. This phenomenon encourages the use of MIMO at higher SNRs and SIMO at lower SNRs in terms of the error probability; as the former often has their signal resolutions higher than the latter. This observation also motivates a fundamental rethinking to determine whether to use MIMO or SIMO for wireless communications given binary-array receivers. In fact, there are a number of arguable advantages for SIMO, including wider coverage, higher point-to-point throughput, as well as lower complexity of the MLD. All of these are extensively investigated in this paper through both analytical work and computer simulations.
Advancements in satellite technology have made direct satellite-to-device connectivity a viable solution for ensuring global access. This method is designed to provide internet connectivity to remote, rural, or underserved areas where traditional cellular or broadband networks are lacking or insufficient. This paper is a survey providing an in-depth review of multi-satellite Multiple Input Multiple Output (MIMO) systems as a potential solution for addressing the link budget challenge in direct satellite-to-device communication. Special attention is given to works considering multi-satellite MIMO systems, both with and without satellite collaboration. In this context, collaboration refers to sharing data between satellites to improve the performance of the system. This survey starts by highlighting the industry's views on the importance of enabling the direct satellite-to-device communications. It follows by explaining several fundamental aspects of satellite communications (SatComs), which are vital prerequisites before investigating the multi-satellite MIMO systems. These aspects encompass satellite orbits, the structure of satellite systems, SatCom links, including the inter-satellite links (ISL) which facilitate satellite cooperation, satellite frequency bands, satellite antenna design, and satellite channel models, which should be known or estimated for effective data transmission to and from multiple satellites. Furthermore, this survey distinguishes itself by providing more comprehensive insights in comparison to other surveys. It specifically delves into the Orthogonal Time Frequency Space (OTFS) within the channel model section. It goes into detail about ISL noise and ISL channel model, and it extends the ISL section by thoroughly investigating hybrid FSO/RF ISLs. Furthermore, analytical comparisons of simulation results from these works are presented to highlight the advantages of employing multi-satellite MIMO systems.
Extremely large aperture array (ELAA) is a promising multiple-input multiple-output (MIMO) technique for next generation mobile networks. In this paper, we propose two novel approaches to accelerate the convergence of current iterative MIMO detectors in ELAA channels. Our approaches exploit the static components of the ELAA channel, which include line of sight (LoS) paths and deterministic non-LoS (NLoS) components due to channel hardening effects. This paper proposes novel convergence acceleration techniques for fast iterative ELAA-MIMO detection by leveraging the static channel component, including the LoS paths and deterministic NLoS components that arise due to channel hardening. Specifically, these static channel components are utilized in two ways: as preconditioning matrices for general iterative algorithms, and as initialization for quasi-Newton (QN) methods. Simulation results show that the proposed approaches converge significantly faster compared to current iterative MIMO detectors, especially under strong LoS conditions with high Rician K-factor. Furthermore, QN methods with the proposed initialization matrix consistently achieve the best convergence performance while maintaining low complexity.
—Recent advancements in diffusion models have led to a significant breakthrough in generative modeling. The combination of the generative model and semantic communication (SemCom) enables high-fidelity semantic information exchange at ultra-low rates. In this paper, a novel generative SemCom framework for image tasks is proposed, utilizing pre-trained foundation models as semantic encoders and decoders for semantic feature extraction and image regeneration, respectively. The mathematical relationship between transmission reliability and the perceptual quality of regenerated images is modeled and the semantic values of extracted features are defined accordingly. This relationship is derived through numerical simulations on the Kodak dataset. Furthermore, we investigate the semantic-aware power allocation problem, aiming to minimize total power consumption while guaranteeing semantic performance. To solve this problem, two semantic-aware power allocation methods are proposed by constraint decoupling and bisection search, respectively. Numerical results demonstrate that the proposed semantic-aware methods outperform conventional approach in terms of total power consumption.
The ubiquitous availability of wireless networks and devices provides a unique opportunity to leverage the corresponding communication signals to enable wireless sensing applications. In this article, we develop a new framework for environment sensing by opportunistic use of the mmWave communication signals. The proposed framework is based on a mixture of the conventional and Neural Network (NN) signal processing techniques for simultaneous counting and localization of multiple targets in the environment in a bi-static setting. In this framework, multi-modal delay, Doppler, angular features are first derived from the Channel State Information (CSI) estimated at the receiver, and then a transformer-based NN architecture exploiting attention mechanisms, called CSIformer, is designed to extract the most effective features for sensing. We also develop a novel post-processing technique based on Kullback-Leibler (KL) minimization to transfer knowledge between the counting and localization tasks, thereby simplifying the NN architecture. Our numerical results show accurate counting and localization capabilities that significantly outperform the existing works based on pure conventional signal processing techniques, as well as NN-based approaches. The simulation codes are available at: https://github.com/University-of-Surrey-Mahdi/Attention-on-the-Preambles-Sensing-with-mmWave-CSI.
Signal detection in large multiple-input multiple-output (large-MIMO) systems presents greater challenges compared to conventional massive-MIMO for two primary reasons. First, large-MIMO systems lack favorable propagation conditions as they do not require a substantially greater number of service antennas relative to user antennas. Second, the wireless channel may exhibit spatial non-stationarity when an extremely large aperture array (ELAA) is deployed in a large-MIMO system. In this paper, we propose a scalable iterative large-MIMO detector named ANPID, which simultaneously delivers 1) close to maximum-likelihood detection performance, 2) low computational-complexity (i.e., square-order of transmit antennas), 3) fast convergence, and 4) robustness to the spatial non-stationarity in ELAA channels. ANPID incorporates a damping demodulation step into stationary iterative (SI) methods and alternates between two distinct demodulated SI methods. Simulation results demonstrate that ANPID fulfills all the four features concurrently and outperforms existing low-complexity MIMO detectors, especially in highly-loaded large-MIMO systems.
In this paper, a novel spatially non-stationary fading channel model is proposed for multiple-input multiple-output (MIMO) system with extremely-large aperture service-array (ELAA). The proposed model incorporates three key factors which cause the channel spatial non-stationarity: 1) link-wise path-loss; 2) shadowing effect; 3) line-of-sight (LoS)/non-LoS state. With appropriate parameter configurations, the proposed model can be used to generate computer-simulated channel data that matches the published measurement data from practical ELAA-MIMO channels. Given such appealing results, the proposed fading channel model is employed to study the cumulative distribution function (CDF) of ELAA-MIMO channel capacity. For all of our studied scenarios, it is unveiled that the ELAA-MIMO channel capacity obeys the skew normal distribution. Moreover, the channel capacity is also found close to the Gaussian or Weibull distribution, depending on users' geo-location and distribution. More specifically, for single-user equivalent scenarios or multiuser scenarios with short user-to-ELAA distances (e.g., 1 m), the channel capacity is close to the Gaussian distribution; and for others, it is close to the Weibull distribution. Finally, the proposed channel model is also employed to study the impact of channel spatial non-stationarity on linear MIMO receivers through computer simulations. The proposed fading channel model is available at https://github.com/ELAA-MIMO/non-stationary-fading-channel-model .
This work aims to handle the joint transmitter and noncoherent receiver optimization for multiuser single-input multiple-output (MU-SIMO) communications through unsupervised deep learning. It is shown that MU-SIMO can be modeled as a deep neural network with three essential layers, which include a partially-connected linear layer for joint multiuser waveform design at the transmitter side, and two nonlinear layers for the noncoherent signal detection. The proposed approach demonstrates remarkable MU-SIMO noncoherent communication performance in Rayleigh fading channels.
In this paper, federated learning (FL) over wireless networks is investigated. In each communication round, a subset of devices is selected to participate in the aggregation with limited time and energy. In order to minimize the convergence time, global loss and latency are jointly considered in a Stackelberg game based framework. Specifically, age of information (AoI) based device selection is considered at leader-level as a global loss minimization problem, while sub-channel assignment, computational resource allocation, and power allocation are considered at follower-level as a latency minimization problem. By dividing the follower-level problem into two sub-problems, the best response of the follower is obtained by a monotonic optimization based resource allocation algorithm and a matching based sub-channel assignment algorithm. By deriving the upper bound of convergence rate, the leader-level problem is reformulated, and then a list based device selection algorithm is proposed to achieve Stackelberg equilibrium. Simulation results indicate that the proposed device selection scheme outperforms other schemes in terms of the global loss, and the developed algorithms can significantly decrease the time consumption of computation and communication.
Considering a densely populated area where a mobile device, with a single RF chain, shares its message with a set of mobile devices through narrowband mmWave channel, an analogue-beam splitting approach is proposed to achieve a good capacity and coverage trade-off. The proposed approach aims at maximizing the capacity of the mmWave multicast channel through antenna-element grouping and adaptive phase shifting, which takes into account of the inter-beam interference. When receivers are randomly distributed on a circle centered at the transmitter, according to the uniform distribution, it is found that the impact of inter-beam interference on the channel capacity can be negligibly small, and thus the analoguebeam splitting approach can be largely simplified in practice. Computer simulations are carried out to elaborate our theoretical study and demonstrate considerable advantages of the proposed analogue-beam splitting approach.
—With the huge number of broadband users, automated network management becomes of huge interest to service providers. A major challenge is automated monitoring of user Quality of Experience (QoE), where Artificial Intelligence (AI) and Machine Learning (ML) models provide powerful tools to predict user QoE from basic protocol indicators such as Round Trip Time (RTT), retransmission rate, etc. In this paper, we introduce an effective feature selection method along with the corresponding classification algorithms to address this challenge. The simulation results show a prediction accuracy of 78% on the benchmark ITU ML5G-PS-012 dataset, improving 11% over the state-of-the-art result whilst reducing the model complexity at the same time. Moreover, we show that the local area network round trip time (LAN RTT) value during daytime and midweek plays the most prominent factor affecting the user QoE.
In this paper, several novel test methods are proposed for 5G mobile network field trials. As it is known, field trial plays an important role in wireless network commercialization process. However, several new technologies have been considered in 5G communication networks, such as massive MIMO. Therefore, conventional filed trial methods used in 4G mobile networks cannot fulfill the new test requirements for 5G mobile networks. Four new field trial methods have been discussed in our work. Moreover, real measurement data has been collected to demonstrate the proposed methods from 5G large scale filed trial supported by China Mobile Communications Group Co., Ltd.
In this paper, a correlation matching pursuit (CMP) procedure is proposed to handle the vector perturbation (VP) problem for nonlinear precoding (NLP) in downlink multiuser multi-antenna (i.e., MU-MIMO) systems. Basically, CMP consists of two sub-procedures, i.e., correlation matching and correlation pursuit. The former takes the charge of direct calculation of the perturbation integers through a set of established convex optimization problems; the latter is responsible for selecting the perturbation vector for updating the precoded vector. Iterative execution of both procedures renders CMP being modelled as a searching tree which consists of multiple nodes and paths. The sequence correlation between the precoded vector (node vector) and perturbation vectors (path vectors) is revealed crucial to the performance optimality and thus used as metric of the path search. Given that the single-path search is suboptimal, we propose multi-path schemes to enable exploitation on the path diversity and thus further improve the performance. Complexity analysis and computer simulations demonstrate that CMP-based NLP algorithms serve as low-cost VP solutions with significantly lowered processing latency and meanwhile, comparable performance compared to prior arts.
In this paper, the real-time deployment of unmanned aerial vehicles (UAVs) as flying base stations (BSs) for optimizing the throughput of mobile users is investigated for UAV networks. This problem is formulated as a time-varying mixed-integer non-convex programming (MINP) problem, which is challenging to find an optimal solution in a short time with conventional optimization techniques. Hence, we propose an actor-critic-based (AC-based) deep reinforcement learning (DRL) method to find near-optimal UAV positions at every moment. In the proposed method, the process searching for the solution iteratively at a particular moment is modeled as a Markov decision process (MDP). To handle infinite state and action spaces and improve the robustness of decision process, two powerful neural networks (NNs) are configured to evaluate the UAV position adjustments and make decisions, respectively. Compared with heuristic, sequential least-squares programming and fixed methods, Simulation results have shown that the proposed method outperforms in terms of the throughput at every moment in UAV networks.
This letter presents a new posterior Cramér-Rao bound (PCRB) for inertial sensors enhanced mobile positioning, which performs hybrid data fusion of parameters including position estimates, pedestrian step size, pedestrian heading, and the knowledge of random walk motion model. Moreover, a non-matrix closed form of the PCRB is derived without position estimates. Finally, our numerical results show that when the accuracy of step size and heading measurements is high enough, the knowledge of random walk model becomes redundant.
In this paper, we investigate the uplink transmission of a single-antenna handheld user to a cluster of satellites. Taking advantage of the inter-satellite links, the satellites can cooperate which each other to jointly detect the received signal. We examine a scenario in which the satellite cluster lacks access to the instantaneous channel state information (CSI). Thus, using only statistical CSI, we design the joint detection by minimizing the mean square error (MSE). We calculate the ergodic capacity using the properties of the Wishart matrix, and then for low-SNR scenarios we provide a closed-form approximation for it. Our numerical results demonstrate the effectiveness of the detection scheme, along with the proximity of the approximation to the actual ergodic capacity. Considering a mega constellation with 3168 satellites in low earth orbit (LEO), we show that a capacity of more than 10 MB/sec can be achieved even if only the statistical CSI is known to the receiver, and a capacity of up to 38 MB/sec can be achieved with perfect instantaneous CSI.
In this paper, unsupervised deep learning solutions for multiuser single-input multiple-output (MU-SIMO) coherent detection are extensively investigated. According to the ways of utilizing the channel state information at the receiver side (CSIR), deep learning solutions are divided into two groups. One group is called equalization and learning, which utilizes the CSIR for channel equalization and then employ deep learning for multiuser detection (MUD). The other is called direct learning, which directly feeds the CSIR, together with the received signal, into deep neural networks (DNN) to conduct the MUD. It is found that the direct learning solutions outperform the equalizationand- learning solutions due to their better exploitation of the sequence detection gain. On the other hand, the direct learning solutions are not scalable to the size of SIMO networks, as current DNN architectures cannot efficiently handle many cochannel interferences. Motivated by this observation, we propose a novel direct learning approach, which can combine the merits of feedforward DNN and parallel interference cancellation. It is shown that the proposed approach trades off the complexity for the learning scalability, and the complexity can be managed due to the parallel network architecture.
One of the major challenges of Cellular network based localization techniques is lack of hearability between mobile terminals (MTs) and base stations (BSs), thus the number of available anchors is limited. In order to solve the hearability problem, previous work assume some of the MTs have their location information via Global Positioning System (GPS). These located MT can be utilized to find the location of an un-located MT without GPS receiver. However, its performance is still limited by the number of available located MTs for cooperation. This paper consider a practical scenario that hearability is only possible between a MT and its home BS. Only one located MT together with the home BS are utilized to find the location of the un-located MT. A hybrid cooperative localization approach is proposed to combine time-of-arrival and received signal strength based fingerprinting techniques. It is shown in simulations that the proposed hybrid approach outperform the stand-alone time-of-arrival techniques or received signal strength based fingerprinting techniques in the considered scenario. It is also found that the proposed approach offer better accuracy with larger distance between the located MT and the home BS. © 2011 IEEE.
—Numerous low-complexity iterative algorithms have been proposed to offer the performance of linear multiple-input multiple-output (MIMO) detectors bypassing the channel matrix inverse. These algorithms exhibit fast convergence in well-conditioned MIMO channels. However, in the emerging MIMO paradigm utilizing extremely large aperture arrays (ELAA), the wireless channel may become ill-conditioned because of spatial non-stationarity, which results in a considerably slower convergence rate for these algorithms. In this paper, we propose a novel ELAA-MIMO detection scheme that leverages user-wise singular value decomposition (UW-SVD) to accelerate the convergence of these iterative algorithms. By applying UW-SVD, the MIMO signal model can be converted into an equivalent form featuring a better-conditioned transfer function. Then, existing iterative algorithms can be utilized to recover the transmitted signal from the converted signal model with accelerated convergence towards zero-forcing performance. Our simulation results indicate that proposed UW-SVD scheme can significantly accelerate the convergence of the iterative algorithms in spatially non-stationary ELAA channels. Moreover, the computational complexity of the UW-SVD is comparatively minor in relation to the inherent complexity of the iterative algorithms. Index Terms—User-wise singular value decomposition (UW-SVD), extremely large aperture array (ELAA), multiple-input multiple-output (MIMO), iterative algorithms.
This letter presents a novel opportunistic cooperative positioning approach for orthogonal frequency-division multiple access (OFDMA) systems. The basic idea is to allow idle mobile terminals (MTs) opportunistically estimating the arrival timing of the training sequences for uplink synchronization from active MTs. The major advantage of the proposed approach over state-of-the-arts is that the positioning-related measurements among MTs are performed without the paid of training overhead. Moreover, Cramer-Rao lower bound (CRLB) is utilized to derive the positioning accuracy limit of the proposed approach, and the numerical results show that the proposed approach can improve the accuracy of non-cooperative approaches with the a-priori stochastic knowledge of clock bias among idle MTs.
This paper conceives a novel sparse code multiple access (SCMA) codebook design which is motivated by the strong need for providing ultra-low decoding complexity and good error performance in downlink Internet-of-things (IoT) networks, in which a massive number of low-end and low-cost IoT communication devices are served. By focusing on the typical Rician fading channels, we analyze the pair-wise error probability of superimposed SCMA codewords and then deduce the design metrics for multi-dimensional constellation construction and sparse codebook optimization. For significant reduction of the decoding complexity, we advocate the key idea of projecting the multi-dimensional constellation elements to a few overlapped complex numbers in each dimension, called low projection (LP). An emerging modulation scheme, called golden angle modulation (GAM), is considered for multi-stage LP optimization, where the resultant multi-dimensional constellation is called LP-GAM. Our analysis and simulation results show the superiority of the proposed LP codebooks (LPCBs) including one-shot decoding convergence and excellent error rate performance. In particular, the proposed LPCBs lead to decoding complexity reduction by at least 97% compared to that of the conventional codebooks, whilst owning large minimum Euclidean distance. Some examples of the proposed LPCBs are available at https://github.com/ethanlq/SCMA-codebook.
This paper presents an overview of preliminary results of investigations within the WHERE2 Project [1] on identifying promising avenues for location aided enhancements to wireless communication systems. The wide ranging contributions are organized according to the following targeted systems: cellular networks, mobile ad hoc networks (MANETs) and cognitive radio. Location based approaches are found to alleviate significant signaling overhead in various forms of modern communication paradigms that are very information hungry in terms of channel state information at the transmitter(s). And this at a reasonable cost given the ubiquitous availability of location information in recent wireless standards or smart phones. Location tracking furthermore opens the new perspective of slow fading prediction. © VDE VERLAG GMBH.
The reflective elements on a reconfigurable intelligent surface (RIS) can be tuned to improve the propagation environment and, in turn, the system performance. However, RIS also brings challenges in terms of joint active and passive beamforming optimization. First, transmit beamforming has to be jointly designed with RIS to fully reap beamforming gain and signal-to-interference plus noise ratio (SINR) performance. Also, given that the number of beamforming options grows with the number of antennas/elements on the base station(BS)/RIS, it makes exhaustive search unpractical for multi-user (MU) multiple-input-multiple-output (MIMO) systems. In order to reduce the search overhead for joint BS and RIS beamforming optimization, we propose a novel joint active BS and passive RIS beamforming scheme based on a bespoke convolutional neural network (CNN) architecture. More specifically, we develop a fast converge and lightweight CNN with loss function designed based on the probability density function (PDF) of beams. By using this CNN, exhaustive search is only needed in data collection part to derive labels and no longer needed in prediction part. We also adopt a realistic blockage scenario to simulate non-stationary channels. Our proposed solution exhibits fast convergence speed, low neural network (NN) complexity and high prediction accuracy. Simulation results, based on benchmark dataset, show that our approach can significantly outperform comparable existing machine learning algorithms. It can achieve 70% shorter convergence time with around 91% beam prediction accuracy.
Spectrum sensing is one of key enabling techniques to advanced radio technologies such as cognitive radios and ALOHA. This paper presents a novel non-cooperative spectrum sensing approach that can achieve a good trade-off between latency, reliability and computational complexity. Our major idea is to exploit the first-order cyclostationarity of the primary user's signal to reduce the noise-uncertainty problem inherent in the conventional energy detection approach. It is shown that the proposed approach is suitable for detecting the primary user's activity in the interweave paradigm of cognitive spectrum sharing, while the active primary user is periodically sending training sequence. Computer simulations are carried out for the typical IEEE 802.11g system. It is observed that the proposed approach outperforms both the energy detection and the second-order cyclostationarity approach when the observation period is more than 10 frames corresponding to 0.56 ms. ©2010 IEEE.
In this paper, we propose a rate-adaptive bit and power loading approach for the OFDM-based relaying communications. The cooperative relay operates in the half-duplex amplify-and-forward mode. The source and the relay has the separate power constraints. The maximum-ratio combining is employed at -the destination for maximizing the received SNR. Assuming the perfect channel knowledge available at all nodes, the proposed approach is to maximize the throughput (the number of bits/symbol) at the given power constraint and the target link performance. Unlike the water-filling method, the proposed approach does not need the iterative loading process, and can otTer the sub-optimum performance. Computer simulations are used to test the proposed approach for various scenarios with respect to the relay location or the distributed power allocation. © 2008 IEEE.
—The synergistic amalgamation of sparse code multiple access (SCMA) and multiple-input multiple-output (MIMO) technologies can be exploited for improving spectral efficiency and providing enhanced wireless services to massive users. In this case, however, channel estimation is a burning issue with the increasing number of users and/or antennas. To tackle this problem, we propose a novel non-coherent transmission scheme for SCMA, referred to as NC-SCMA. In the proposed NC-SCMA, each user first maps its binary data to sparse codewords, and then perform differential modulation on the non-zero dimensions. Upon receiving all users' signals, we leverage the channel hardening effect to carry out differential demodulation and multiuser detection without any instantaneous channel state information. In addition, the design of the sparse codebooks in the NC-SCMA system is investigated with the aid of the pair-wise probability. Numerical results demonstrate the superiority of the proposed technique over the benchmark scheme in terms of bit error rate performance.
In the above article [1] , we have found that Figs. 5 and 6 were the same, while the detailed explanations for the two figures were correct. After carefully checking the final-accepted file uploaded to the submission system and the journal publication file, the abovementioned mix-up may be triggered by the incautious manuscript proof procedure. More specifically, the mix-up contents were only related with Fig. 6 and did not affect other contents (e.g., Conclusion section) in the manuscript. The correct Fig. 6 is provided as follows, which is consistent with other EIT metasensors [2] , [3] .
—The cumulative distribution function (CDF) of a non-central χ 2-distributed random variable (RV) is often used when measuring the outage probability of communication systems. For ultra-reliable low-latency communication (URLLC), it is important but mathematically challenging to determine the outage threshold for an extremely small outage target. This motivates us to investigate lower bounds of the outage threshold, and it is found that the one derived from the Chernoff inequality (named Cher-LB) is the most effective lower bound. This finding is associated with three rigorously established properties of the Cher-LB with respect to the mean, variance, reliability requirement , and degrees of freedom of the non-central χ 2-distributed RV. The Cher-LB is then employed to predict the beamforming gain in URLLC for both conventional multi-antenna systems (i.e., MIMO) under first-order Markov time-varying channel and reconfigurable intellgent surface (RIS) systems. It is exhibited that, with the proposed Cher-LB, the pessimistic prediction of the beamforming gain is made sufficiently accurate for guaranteed reliability as well as the transmit-energy efficiency.
The cumulative distribution function (CDF) of a non-central χ2 -distributed random variable (RV) is often used when measuring the outage probability of communication systems. For adaptive transmitters, it is important but mathematically challenging to determine the outage threshold for an extreme target outage probability (e.g., 10−5 or less). This motivates us to investigate lower bounds of the outage threshold, and it is found that the one derived from the Chernoff inequality (named Cher-LB) is the most {effective} lower bound. The Cher-LB is then employed to predict the multi-antenna transmitter beamforming-gain in ultra-reliable and low-latency communication, concerning the first-order Markov time-varying channel. It is exhibited that, with the proposed Cher-LB, pessimistic prediction of the beamforming gain is made sufficiently accurate for guaranteed reliability as well as the transmit-energy efficiency.
Signal detection in large multiple-input multiple-output (large-MIMO) systems presents greater challenges compared to conventional massive-MIMO for two primary reasons. First, large-MIMO systems lack favorable propagation conditions as they do not require a substantially greater number of service antennas relative to user antennas. Second, the wireless channel may exhibit spatial non-stationarity when an extremely large aperture array (ELAA) is deployed in a large-MIMO system. In this paper, we propose a scalable iterative large-MIMO detector named ANPID, which simultaneously delivers 1) close to maximum-likelihood detection performance, 2) low computational-complexity (i.e., square-order of transmit antennas), 3) fast convergence, and 4) robustness to the spatial non-stationarity in ELAA channels. ANPID incorporates a damping demodulation step into stationary iterative (SI) methods and alternates between two distinct demodulated SI methods. Simulation results demonstrate that ANPID fulfills all the four features concurrently and outperforms existing low-complexity MIMO detectors, especially in highly-loaded large MIMO systems.
Deep learning is driving a radical paradigm shift in wireless communications, all the way from the application layer down to the physical layer. Despite this, there is an ongoing debate as to what additional values artificial intelligence (or machine learning) could bring to us, particularly on the physical layer design; and what penalties there may have? These ques-tions motivate a fundamental rethinking of the wireless modem design in the artificial intelli-gence era. Through several physical-layer case studies, we argue for a significant role that ma-chine learning could play, for instance in parallel error-control coding and decoding, channel equalization, interference cancellation, as well as multiuser and multiantenna detection. In addition, we discuss the fundamental bottlenecks of machine learning as well as their poten-tial solutions in this paper.
Orthogonal relay based cooperative communication enjoys distributed spatial diversity gain at the price of spectral efficiency. This work aims at improving the spectral efficiency for orthogonal opportunistic decode-and-forward (DF) relaying through employment of novel adaptive modulation scheme. The proposed scheme allows source and relay to transmit information in different modulation formats, while the MAP receiver is employed at destination for the diversity combining. Given the individual power constraint and target bit-error-rate (BER), the proposed scheme can significantly improve the spectral efficiency in comparison with the non-adaptive DF relaying and adaptive direct transmission.
In this paper, a novel spatially non-stationary channel model is proposed for link-level computer simulations of massive multiple-input multiple-output (mMIMO) with extremely large aperture array (ELAA). The proposed channel model allows a mix of non-line-of-sight (NLoS) and LoS links between a user and service antennas. The NLoS/LoS state of each link is characterized by a binary random variable, which obeys a correlated Bernoulli distribution. The correlation is described in the form of an exponentially decaying window. In addition, the proposed model incorporates shadowing effects which are non-identical for NLoS and LoS states. It is demonstrated, through computer emulation, that the proposed model can capture almost all spatially non-stationary fading behaviors of the ELAA-mMIMO channel. Moreover, it has a low implementational complexity. With the proposed channel model, Monte-Carlo simulations are carried out to evaluate the channel capacity of ELAA-mMIMO. It is shown that the ELAA-mMIMO channel capacity has considerably different stochastic characteristics from the conventional mMIMO due to the presence of channel spatial non-stationarity.
This paper studies issues that arise with respect to the joint optimization for convergence time in federated learning over wireless networks (FLOWN). We consider the criterion and protocol for selection of participating devices in FLOWN under the energy constraint and derive its impact on device selection. In order to improve the training efficiency, age-of-information (AoI) enables FLOWN to assess the freshness of gradient updates among participants. Aiming to speed up convergence, we jointly investigate global loss minimization and latency minimization in a Stackelberg game based framework. Specifically, we formulate global loss minimization as a leader-level problem for reducing the number of required rounds, and latency minimization as a follower-level problem to reduce time consumption of each round. By decoupling the follower-level problem into two sub-problems, including resource allocation and sub-channel assignment, we achieve an optimal strategy of the follower through monotonic optimization and matching theory. At the leader-level, we derive an upper bound of convergence rate and subsequently refor-mulate the global loss minimization problem and propose a new age-of-update (AoU) based device selection algorithm. Simulation results indicate the superior performance of the proposed AoU based device selection scheme in terms of the convergence rate, as well as efficient utilization of available sub-channels.
Current iterative multiple-input multiple-output (MIMO) detectors suffer from slow convergence when the wireless channel is ill-conditioned. The ill-conditioning is mainly caused by spatial correlation between channel columns corresponding to the same user equipment, known as intra-user interference. In addition, in the emerging MIMO systems using an extremely large aperture array (ELAA), spatial non-stationarity can make the channel even more ill-conditioned. In this paper, user-wise singular value decomposition (UW-SVD) is proposed to accelerate the convergence of iterative MIMO detectors. Its basic principle is to perform SVD on each user's sub-channel matrix to eliminate intra-user interference. Then, the MIMO signal model is effectively transformed into an equivalent signal (e-signal) model, comprising an e-channel matrix and an e-signal vector. Existing iterative algorithms can be used to recover the e-signal vector, which undergoes post-processing to obtain the signal vector. It is proven that the e-channel matrix is better conditioned than the original MIMO channel for spatially correlated (ELAA-)MIMO channels. This implies that UW-SVD can accelerate current iterative algorithms, which is confirmed by our simulation results. Specifically, it can speed up convergence by up to 10 times in both uncoded and coded systems. Index Terms—Linear MIMO detectors, extremely large aperture array (ELAA), user-wise singular value decomposition (UW-SVD), channel ill-conditioning, fast convergence.
Underlay cognitive beamforming allows secondary transmitters to suppress interferences to the primary users, whilst maintain their own quality of services. This paper aims at investigating joint power and interference trade-off inherent in the underlay cognitive beamforming scheme. It is shown that the work of interests leads to a non-convex optimization problem, which can be resolved by employing the second-order cone programming. It is theoretically proved that introducing zero-interference to the primary user does not always lead to the system optimality; moreover, we exhibit two conditions, for which the interference should be treated as noise in order to maximize the sum-rate of the considered beamforming system. © VDE VERLAG GMBH.
Electrochemical carbon dioxide (CO2) reduction reaction (CO2RR) is an attractive approach to deal with the excessive emission of CO2 and to produce valuable fuels and chemicals in a carbon-neutral way. Many efforts have been devoted to boost the activity and selectivity of high-value multicarbon products (C2+) on Cu-based electrocatalysts. However, Cu-based CO2RR electrocatalysts suffer from poor catalytic stability mainly due to the structural degradation and loss of active species under CO2RR condition. To date, most reported Cu-based electrocatalysts present stabilities over dozens of hours, which limits the advance of Cu-based electrocatalysts for CO2RR. Here, a porous chlorine-doped Cu electrocatalyst is reported and exhibits high C2+ Faradaic efficiency (FE) of 53.8 % at-1.00 V versus reversible hydrogen electrode (VRHE). Importantly, the catalyst exhibited an outstanding catalytic stability in long-term electrocatalysis over 240 hours. Experimental results show that the chlorine-induced stable cationic Cu 0-Cu + species and the well-preserved structure with abundant active sites are found to be critical to maintain the high FE of C2+ in the long-term run of electrochemical CO2 reduction.
The design of iterative linear precoding is recently challenged by extremely large aperture array (ELAA) systems, where conventional preconditioning techniques could hardly improve the channel condition. In this paper, it is proposed to regularize the extreme singular values to improve the channel condition by deducting a rank-one matrix from the Wishart matrix of the channel. Our analysis proves the feasibility to reduce the largest singular value or to increase multiple small singular values with a rank-one matrix when the singular value decomposition of the channel is available. Knowing the feasibility, we propose a low-complexity approach where an approximation of the regularization matrix can be obtained based on the statistical property of the channel. It is demonstrated, through simulation results, that the proposed low-complexity approach significantly outperforms current preconditioning techniques in terms of reduced iteration number in both ELAA systems as well as symmetric multi-antenna (i.e., MIMO) systems when the channel is i.i.d. Rayleigh fading.
This paper consider cooperative localization in cellular networks. In this scenario, several located mobile terminals (MTs) are employed as reference nodes to find the location of an un-located MT. The located MTs sent training sequences in the uplink, then the un-located MT perform distance estimation using received signal strength techniques. The localization accuracy of the un-located MT is characterized in terms of squared position error bound (SPEB) [1]. By taking into account the imperfect a priori location knowledge of the located MTs, the SPEB is derived in a closed-form. The closed-form indicate that the effect of the imperfect location knowledge on SPEB is equivalent to the increase of the variance of distance estimation. Moreover, based on the obtained closed-form, a MT selection scheme is proposed to decrease the number of located MTs sending training sequences, thus reduce the training overhead for localization. The simulation results show that the proposed scheme can reduce the training overhead with the paid of accuracy. and with the same training overhead, the accuracy of the proposed scheme is better than that of random selection. © 2011 IEEE.
This paper examines the uplink transmission of a single-antenna handsheld user to a cluster of satellites, with a focus on utilizing the inter-satellite links to enable cooperative signal detection. Two cases are studied: one with full CSI and the other with partial CSI between satellites. The two cases are compared in terms of capacity, overhead, and bit error rate. Additionally, the impact of channel estimation error is analyzed in both designs, and robust detection techniques are proposed to handle channel uncertainty up to a certain level. The performance of each case is demonstrated, and a comparison is made with conventional satellite communication schemes where only one satellite can connect to a user. The results of our study reveal that the proposed constellation with a total of 3168 satellites in orbit can enable a capacity of 800 Mbits/sec through cooperation of $12$ satellites with and occupied bandwidth of 500 MHz. In contrast, conventional satellite communication approaches with the same system parameters yield a significantly lower capacity of less than 150 Mbits/sec for the nearest satellite.
Numerous low-complexity iterative algorithms have been proposed to offer the performance of linear multiple-input multiple-output (MIMO) detectors bypassing the channel matrix inverse. These algorithms exhibit fast convergence in well-conditioned MIMO channels. However, in the emerging MIMO paradigm utilizing extremely large aperture arrays (ELAA), the wireless channel may become ill-conditioned because of spatial non-stationarity, which results in a considerably slower convergence rate for these algorithms. In this paper, we propose a novel ELAA-MIMO detection scheme that leverages user-wise singular value decomposition (UW-SVD) to accelerate the convergence of these iterative algorithms. By applying UW-SVD, the MIMO signal model can be converted into an equivalent form featuring a better-conditioned transfer function. Then, existing iterative algorithms can be utilized to recover the transmitted signal from the converted signal model with accelerated convergence towards zero-forcing performance. Our simulation results indicate that proposed UW-SVD scheme can significantly accelerate the convergence of the iterative algorithms in spatially non-stationary ELAA channels. Moreover, the computational complexity of the UW-SVD is comparatively minor in relation to the inherent complexity of the iterative algorithms.
Consider robot swarm wireless networks where mobile robots offload their computing tasks to a computing server located at the mobile edge. Our aim is to maximize the swarm lifetime through efficient exploitation of the correlation between distributed data sources. The optimization problem is handled by selecting appropriate robot subsets to send their sensed data to the server. In this work, the data correlation between distributed robot subsets is modelled as an undirected graph. A least-degree iterative partitioning (LDIP) algorithm is proposed to partition the graph into a set of subgraphs. Each subgraph has at least one vertex (i.e., subset), termed representative vertex (R-Vertex), which shares edges with and only with all other vertices within the subgraph; only R-Vertices are selected for data transmissions. When the number of subgraphs is maximized, the proposed subset selection approach is shown to be optimum in the AWGN channel. For independent fading channels, the max-min principle can be incorporated into the proposed approach to achieve the best performance.
In this paper, a symbol-level selective transmission for full-duplex (FD) relaying networks is proposed to mitigate error propagation effects and improve system spectral efficiency. The idea is to allow the FD relay node to predict the correctly decoded symbols of each frame, based on the generalized square deviation method, and discard the erroneously decoded symbols, resulting in fewer errors being forwarded to the destination node. Using the capability for simultaneous transmission and reception at the FD relay node, our proposed strategy can improve the transmission efficiency without extra cost of signalling overhead. In addition, targeting on the derived expression for outage probability, we compare it with half-duplex (HD) relaying case, and provide the transmission power and relay location optimization strategy to further enhance system performances. The results show that our proposed scheme outperforms the classic relaying protocols, such as cyclic redundancy check based selective decode-and-forward (S-DF) relaying and threshold based SDF relaying in terms of outage probability and bit-error-rate. Moreover, the performances with optimal power allocation are better than those with equal power allocation, especially when the FD relay node encounters strong self-interference and/or it is close to the destination node.
In this paper, we propose the Turbo principle (of using parallel concatenated channel encoders by an interleaver and iterative soft-input and soft-output decoding between the constituent decoders)onto Polar and LDPC codes resulting in Turbo-Polar, Turbo-LDPC-Polar, and Turbo-LDPC schemes with the aim of enhancing the BLER performance while alsoreducing the decoding complexity. All the proposed turbocoded schemes are decoded using the traditional Belief Propagation (BP) algorithm based on low-density paritycheck iterative decoding through a factor graph. Monte Carlo simulation results confirm the superiority of TurboLDPC and Turbo-LDPC-Polar schemes in BLER performance over state-of-the-art cyclic redundancy check-aided successive cancellation List decoding (CASCL) of Polar Codes with a large list size of 32 for large block lengths (larger than 3072 bits) while having reduced computational decoding complexity in comparison to CASCL decoding in an additive white Gaussian noise (AWGN) channel. Furthermore, Turbo-LDPC (based on 5G New Radio specifications for LDPC code) outperforms the standalone 5G-NR LDPC code and achieves about 1dB gain at a BLER of − over correlated slow fadingRayleigh channel; however, being turbo-iterative in nature, it has higher complexity (about six-fold) than the standalone 5GNR LDPC code.
In this paper, a cooperative iterative water-filling approach is investigated for two-user Gaussian interference channel. State-of-the-art approaches only maximize the individual user's own rate and always model interference as noise. Our proposed approach establishes user cooperation through sharing network side information. It iteratively maximizes the sum-rate of both users subject to distributed power constraint. Interference is optimally regarded as message or noise. Three efficient rate-sharing schemes are also investigated between two users based on priority. Numerical results are performed in frequency-selective environment. It is observed that the proposed approach offers significantly performance improvement in comparison with conventional iterative water-filling approaches.
Previous work about cooperative localization in cellular networks usually consider a centralized processor (CP) is available for location estimation. This paper consider cooperative localization in a distributed base station (BS) scenario, where there is no CP, and the distributed BSs are responsible for location estimation. In this scenario, Global Positioning System (GPS) enable mobile terminals (MTs), i.e., located MTs, are employed as reference nodes. Then, several located MTs can help to find the locations of an un-located MT, by estimating the distance between the un-located MT using received signal strength techniques. Two localization approaches are proposed, the first approach requires only one BS to collect all the assistance information for localization and estimate the location. The second approach distribute the location estimation task to several BSs. The communication overhead between distributed BSs are investigated for these two approaches. Moreover, by taking into account the effect of imperfect location knowledge of the located MTs, the accuracy limits of both approaches are derived. The simulation results shows that compared with the first approach, the second approach can reduce the communication overhead between distributed BSs with the paid of accuracy. © 2011 IEEE.
Wi-Fi sensing has become an attractive option for non-invasive monitoring of human activities and vital signs. This paper explores the feasibility of using state-of-the-art commercial off-the-shelf (COTS) devices for Wi-Fi sensing applications, particularly respiration monitoring and motion detection. We utilize the Intel AX210 network interface card (NIC) to transmit Wi-Fi signals in both 2.4 GHz and 6 GHz frequency bands. Our experiments rely on channel frequency response (CFR) and received signal strength indicator (RSSI) data, which are processed using a moving average algorithm to extract human behavior patterns. The experimental results demonstrate the effectiveness of our approach in capturing and representing human respiration and motion patterns. Furthermore, we compare the performance of Wi-Fi sensing across different frequency bands, highlighting the advantages of using higher frequencies for improved sensitivity and clarity. Our findings showcase the practicality of using COTS devices for Wi-Fi sensing and lay the groundwork for the development of non-invasive, contactless sensing systems. These systems have potential applications in various fields, including healthcare, smart homes, and Metaverse.
The H2020 project 5G-DRIVE (5G HarmoniseD Research and Trials for serVice Evolution between EU and China) Ill cooperates with the Chinese twin project to trial and validate key functions of 5G networks operating at 3.5 GHz bands for enhanced Mobile Broadband (eMBB) and 3.5 GHz & 5.9 GHz bands for V2X scenarios. This paper describes the ongoing standardisation efforts for eMBB and V2X scenarios. Furthermore, it introduces the use cases considered by the 5G-DRIVE project.
The upcoming 6G technology is expected to operate in near-field (NF) radiating conditions thanks to high-frequency and electrically large antenna arrays. While several studies have already addressed this possibility, it is worth noting that NF models introduce heightened complexity, the justification for which is not always evident in terms of performance improvements. Therefore, this paper delves into the implications of the disparity between NF and far-field (FF) models concerning communication, localization, and sensing systems. Such disparity might lead to a degradation of performance metrics like localization accuracy, sensing reliability, and communication efficiency. Through an exploration of the effects arising from the mismatches between NF and FF models, this study seeks to illuminate the challenges confronting system designers and offer valuable insights into the balance between model accuracy, which typically requires a high complexity and achievable performance. To substantiate our perspective, we also incorporate a numerical performance assessment confirming the repercussions of the mismatch between NF and FF models.
In this paper, a novel nonlinear precoding (NLP) technique, namely constellation-oriented perturbation (COP), is proposed to tackle the scalability problem inherent in conventional NLP techniques. The basic concept of COP is to apply vector perturbation (VP) in the constellation domain instead of symbol domain; as often used in conventional techniques. By this means, the computational complexity of COP is made independent to the size of multi-antenna (i.e., MIMO) networks. Instead, it is related to the size of symbol constellation. Through widely linear transform, it is shown that COP has its complexity flexibly scalable in the constellation domain to achieve a good complexityperformance tradeoff. Our computer simulations show that COP can offer very comparable performance with the optimum VP in small MIMO systems. Moreover, it significantly outperforms current sub-optimum VP approaches (such as degree-2 VP) in large MIMO whilst maintaining much lower computational complexity.
Computation task offloading plays a crucial role in facilitating computation-intensive applications and edge intelligence, particularly in response to the explosive growth of massive data generation. Various enabling techniques, wireless technologies and mechanisms have already been proposed for task offloading, primarily aimed at improving the quality of services (QoS) for users. While there exists an extensive body of literature on this topic, exploring computation offloading from the standpoint of task types has been relatively underrepresented. This motivates our survey, which seeks to classify the state-ofthe-art (SoTA) from the task type point-of-view. To achieve this, a thorough literature review is conducted to reveal the SoTA from various aspects, including architecture, objective, offloading strategy, and task types, with the consideration of task generation. It has been observed that task types are associated with data and have an impact on the offloading process, including elements like resource allocation and task assignment. Building upon this insight, computation offloading is categorized into two groups based on task types: static task-based offloading and dynamic task-based offloading. Finally, a prospective view of the challenges and opportunities in the field of future computation offloading is presented.
—In this paper, computational task allocation schemes with correlated data are investigated to minimize the energy consumption for symbiotic robot swarm. In such swarm, task needs to be computed cooperatively with the data from multiple robots. Therefore, data need to be transmitted to one selected robot. However, the correlated data among robots can increase the energy consumption of transmission and computation due to the reduandunt. To solve this problem, a model is proposed to investigate the data correlation versus distance among the robots. Based on this model, three task allocation strategies are further proposed. Energy consumption of the robot swarm is reduced through selected robot to transmit data either based on channel gain or data correlation. MATLAB based simulation results show that the proposed task allocation strategies can significantly reduce the energy consumption of symbiotic robotic swarm compared to state-of-the-art.
Multi-access edge computing for mobile computingtask offloading is driving the extreme utilization of available degrees of freedom (DoF) for ultra-reliable low-latency downlink communications. The fundamental aim of this work is to find latency-constrained transmission protocols that can achieve a very-low outage probability (e.g. 0:001%). Our investigation is mainly based upon the Polyanskiy-Poor-Verd´u formula on the finite-length coded channel capacity, which is extended from the quasi-static fading channel to the frequency selective channel. Moreover, the use of a suitable duplexing mode is also critical to the downlink reliability. Specifically, time-division duplexing (TDD) outperforms frequency-division duplexing (FDD) in terms of the frequency diversity-gain. On the other hand, FDD takes the advantage of having more temporal DoF in the downlink, which can be exchanged into the spatial diversity-gain through the use of space-time coding. Numerical study is carried out to compare the reliability between FDD and TDD under various latency constraints.
This paper proposes a novel carrier frequency offset (CFO) estimation method for generalized MC-CDMA systems in unknown frequency-selective channels utilizing hidden pi- lots. It is established that CFO is identifiable in the frequency domain by employing cyclic statistics (CS) and linear re-gression (LR) algorithms. We show that the CS-based estimator is capable of mitigating the normalized CFO (NCFO) to a small error value. Then, the LR-based estimator can be employed to offer more accurate estimation by removing the residual quantization error after the CS-based estimator.
The technology of using massive transmit-antennas to enable ultra-reliable single-shot transmission (URSST) is challenged by the transmitter-side channel knowledge (i.e., CSIT) imperfection. When the imperfectness mainly comes from the channel time-variation, the outage probability of the matched filter (MF) transmitter beamforming is investigated based on the first-order Markov model of the aged CSIT. With a fixed transmit-power, the transmitter-side uncertainty of the instantaneous signal-to-noise ratio (iSNR) is mathematically characterized. In order to guarantee the outage probability for every single shot, a transmit-power adaptation approach is proposed to satisfy a pessimistic iSNR requirement, which is predicted using the Chernoff lower bound of the beamforming gain. Our numerical results demonstrate a remarkable transmit-power efficiency when comparing with power control approaches using other lower bounds. In addition, a combinatorial approach of the MF beamforming and grouped space-time block code (G-STBC) is proposed to further mitigate the detrimental impact of the CSIT uncertainty. It is shown, through both theoretical analysis and computer simulations, that the combinatorial approach can further improve the transmit-power efficiency with a good tradeoff between the outage probability and the latency.
A pilot-based spectrum sensing approach in the presence of unknown timing and frequency offset is proposed in this paper. Our major idea is to utilize the second order statistics of the received samples, such as autocorrelation, to avoid the frequency offset problem. Base on the property of the pilot symbols, where the different symbol blocks usually have the same pilot symbols, some nonzero terms will appear in the frequency domain. To test the proposed approach, computer simulations are carried out for the typical Orthogonal frequency-division multiplexing (OFDM) system. It is observed that the proposed approach always outperforms the classic time domain Neyman-Pearson approach at least 4dB. Moreover, the proposed approach get the same performance as the weighted linear combination based approach when the transmitted data block size is equal to 2048, while a small computational cost is keep at the same time. Therefore, it can be said that the proposed approach can achieve a good trade-off between reliability, latency and the computational cost, when the transmitted data block size of the primary system is larger than 1000. © VDE VERLAG GMBH.
The aim of this article is to share a novel concept termed pseudo pilot, which offers a simple and efficient approach of non-pilot-assisted channel estimation. Our key idea is to transfer the uncertainty of several payload symbols into the uncertainty of symbol interleavers by employing a bank of interleavers at the transmitter. Those uncertainty-transferred symbols serve as pseudo pilots for the receiver to perform channel estimation. The uncertainty of symbol interleavers is then removed in the procedure of decoding. Performance and scalability of the pseudo pilot technique are evaluated through both theoretical analysis and computer simulations.
The aim of this paper is to handle the multifrequency synchronization problem inherent in orthogonal frequency-division multiple access (OFDMA) uplink communications, where the carrier frequency offset (CFO) for each user may be different, and they can be hardly compensated at the receiver side. Our major contribution lies in the development of a novel OFDM receiver that is resilient to unknown random CFO thanks to the use of a CFO-compensator bank. Specifically, the whole CFO range is evenly divided into a set of sub-ranges, with each being supported by a dedicated CFO compensator. Given the optimization for CFO compensator a NP-hard problem, a machine deep-learning approach is proposed to yield a good sub-optimal solution. It is shown that the proposed receiver is able to offer inter-carrier interference free performance for OFDMA systems operating at a wide range of SNRs.
The aim of this letter is to exhibit some advantages of using real constellations in large multi-user (MU) MIMO systems. It is shown that a widely linear zero-forcing (WLZF) receiver with M-ASK modulation enjoys a spatial-domain diversity gain, which linearly increases with the MIMO size even in fully- and over-loaded systems. Using the decision of WLZF as the initial state, the likelihood ascent search (LAS) achieves nearoptimal BER performance in fully-loaded large MIMO systems. Interestingly, for coded systems, WLZF shows a much closer BER to that of WLZF-LAS with a gap of only 0:9-2 dB in SNR.
Quantization is the characterization of analogueto- digital converters (ADC) in massive MIMO systems. The design of quantization function or quantization thresholds is found to relate to quantization step, which is the factor that adapts with the changing of transmit power and noise variance. With the objective of utilizing low-resolution ADC is reducing the cost of massive MIMO, we propose an idea as if it is necessary to have adaptive-threshold quantization function. It is found that when maximum-likelihood (ML) is employed as the detection method, having quantization thresholds fixed for low-resolution ADCs will not cause significant performance loss. Moreover, such fixed-threshold quantization function does not require any information of signal power which can reduce the hardware cost of ADCs. Simulations have been carried out in this paper to make comparisons between fixed-threshold and adaptive-threshold quantization regarding various factors.
Self-supervised monocular depth and visual odometry (VO) are often cast as coupled tasks. Accurate depth contributes to precise pose estimation and vice versa. Existing architectures typically exploit stacking convolution layers and long short-term memory (LSTM) units to capture long-range dependencies. However, their intrinsic locality hinders the model from getting the expected performance gain. In this article, we propose a Transformer-based architecture, named Transformer-based self-supervised monocular depth and VO (TSSM-VO), to tackle these problems. It comprises two main components: 1) a depth generator that leverages the powerful capability of multihead self-attention (MHSA) on modeling long-range spatial dependencies and 2) a pose estimator built upon a Transformer to learn long-range temporal correlations of image sequences. Moreover, a new data augmentation loss based on structural similarity (SSIM) is introduced to constrain further the structural similarity between the augmented depth and the augmented predicted depth. Rigorous ablation studies and exhaustive performance comparison on the KITTI and Make3D datasets demonstrate the superiority of TSSM-VO over other self-supervised methods. We expect that TSSM-VO would enhance the ability of intelligent agents to understand the surrounding environments.
—We focus on the signal detection for large quasi-symmetric (LQS) multiple-input multiple-output (MIMO) systems , where the numbers of both service (M) and user (N) antennas are large and N/M → 1. It is challenging to achieve maximum-likelihood detection (MLD) performance with square-order complexity due to the ill-conditioned channel matrix. In the emerging MIMO paradigm termed with an extremely large aperture array, the channel matrix can be more ill-conditioned due to spatial non-stationarity. In this paper, projected-Jacobi (PJ) is proposed for signal detection in (non-) stationary LQS-MIMO systems. It is theoretically and empirically demonstrated that PJ can achieve MLD performance, even when N/M = 1. Moreover, PJ has square-order complexity of N and supports parallel computation. The main idea of PJ is to add a projection step and to set a (quasi-) orthogonal initialization for the classical Jacobi iteration. Moreover, the symbol error rate (SER) of PJ is mathematically derived and it is tight to the simulation results.
This letter proposes a novel carrier frequency offset (CFO) estimation method for generalized multicarrier code-division multiple access systems in unknown frequency-selective channels utilizing hidden pilots. It is established that CFO is identifiable in the frequency domain by employing cyclic statistics (CS) and linear regression (LR) algorithms. We show that the CS-based estimator is capable of mitigating the normalized CFO (NCFO) to a small error value. Then, the LR-based estimator can be employed to offer more accurate estimation by removing the residual quantization error after the CS-based estimator. Simulation results are presented together with the theoretical analysis, and a good match between them is observed.
—In this paper, a novel spatially non-stationary fading channel model is proposed for multiple-input multiple-output (MIMO) system with extremely-large aperture service-array (ELAA). The proposed model incorporates three key factors which cause the channel spatial non-stationarity: 1) link-wise path-loss; 2) shadowing effect; 3) line-of-sight (LoS)/non-LoS state. With appropriate parameter configurations, the proposed model can be used to generate computer-simulated channel data that matches the published measurement data from practical ELAA-MIMO channels. Given such appealing results, the proposed fading channel model is employed to study the cumulative distribution function (CDF) of ELAA-MIMO channel capacity. For all of our studied scenarios, it is unveiled that the ELAA-MIMO channel capacity obeys the skew normal distribution. Moreover, the channel capacity is also found close to the Gaussian or Weibull distribution, depending on users' geo-location and distribution. More specifically, for single-user equivalent scenarios or multiuser scenarios with short user-to-ELAA distances (e.g., 1 m), the channel capacity is close to the Gaussian distribution; and for others, it is close to the Weibull distribution. Finally, the proposed channel model is also employed to study the impact of channel spatial non-stationarity on linear MIMO receivers through computer simulations. The proposed fading channel model is available at https://github.com/ELAA-MIMO/ non-stationary-fading-channel-model. Index Terms—Channel model, extremely-large aperture array (ELAA), multiple-input multiple-output (MIMO), spatially non-stationary fading.
Doubly differential modem turns out to be a promising technology for coping with unknown frequency offsets with the pay of signal-to-noise ratio (SNR). In this paper, we propose to compensate the SNR loss by employing the detection-forward cooperative relay. The receiver can employ two kind of combiners to attain the achievable spatial diversity-gain. Performance analysis is carefully investigated for the Rayleigh-fading channel. It is shown that the SNR-compensation is satisfied for the large-SNR range.
This paper presents a parallel computing approach that is employed to reconstruct original information bits from a non-recursive convolutional codeword in noise, with the goal of reducing the decoding latency without compromising the performance. This goal is achieved by means of cutting a received codeword into a number of sub-codewords (SCWs) and feeding them into a two-stage decoder. At the first stage, SCWs are decoded in parallel using the Viterbi algorithm or equivalently the brute force algorithm. Major challenge arises when determining the initial state of the trellis diagram for each SCW, which is uncertain except for the first one; and such results in multiple decoding outcomes for every SCW. To eliminate or more precisely exploit the uncertainty, an Euclidean-distance minimization algorithm is employed to merge neighboring SCWs; and this is called the merging stage, which can also run in parallel. Our work reveals that the proposed two-stage decoder is optimal and has its latency growing logarithmically, instead of linearly as for the Viterbi algorithm, with respect to the codeword length. Moreover, it is shown that the decoding latency can be further reduced by employing artificial neural networks for the SCW decoding. Computer simulations are conducted for two typical convolutional codes, and the results confirm our theoretical analysis.
Multiuser multiple-input multiple-output (MUMIMO) nonlinear precoding techniques face the problem of poor computational scalability to the size of the network. In this paper, the fundamental problem of MU-MIMO scalability is tackled through a novel signal-processing approach, which is called degree-2 vector perturbation (D2VP). Unlike the conventional VP approaches that aim at minimizing the transmit-to-receive energy ratio through searching over an N-dimensional Euclidean space, D2VP shares the same target through an iterative-optimization procedure. Each iteration performs vector perturbation over two optimally selected subspaces. By this means, the computational complexity is managed to be in the cubic order of the size of MUMIMO, and this mainly comes from the inverse of the channel matrix. In terms of the performance, it is shown that D2VP offers comparable bit-error-rate to the sphere encoding approach for the case of small MU-MIMO. For the case of medium and large MU-MIMO when the sphere encoding does not apply due to unimplementable complexity, D2VP outperforms the lattice reduction VP by around 5-10 dB in Eb/No and 10-50 dB in normalized computational complexity.
This paper presents a novel approach for mobile positioning in IEEE 802.11a wireless LANs with acceptable computational complexity. The approach improves the positioning accuracy by utilizing the time and frequency domain channel information obtained from the orthogonal frequency-division multiplexing (OFDM) signals. The simulation results show that the proposed approach outperforms the multiple signal classification (MUSIC) algorithm, Ni's algorithm and achieve a positioning accuracy of 1 m with a 97% probability in an indoor scenario.
Task offloading to mobile edge computing (MEC) has emerged as a key technology to alleviate the computation workloads of mobile devices and decrease service latency for the computation-intensive applications. Device battery consumption is one of the limiting factors needs to be considered during task offloading. In this paper, multi-task offloading strategies have been investigated to improve device energy efficiency. Correlations among tasks in time domain as well as task domain are proposed to be employed to reduce the number of tasks to be transmitted to MEC. Furthermore, a binary decision tree based algorithm is investigated to jointly optimize the mobile device clock frequency, transmission power, structure and number of tasks to be transmitted. MATLAB based simulation is employed to demonstrate the performance of our proposed algorithm. It is observed that the proposed dynamic multi-task offloading strategies can reduce the total energy consumption at device along various transmit power versus noise power point compared with the conventional one.
The cumulative distribution function (CDF) of a non-central χ2-distributed random variable (RV) is often used when measuring the outage probability of communication systems. For adaptive transmitters, it is important but mathematically challenging to determine the outage threshold for an extreme target outage probability (e.g., 10 −5 or less). This motivates us to investigate lower bounds of the outage threshold, and it is found that the one derived from the Chernoff inequality (named Cher-LB) is the most effective lower bound. The Cher-LB is then employed to predict the multi-antenna transmitter beamforming-gain in ultra-reliable and low-latency communication, concerning the first-order Markov time-varying channel. It is exhibited that, with the proposed Cher-LB, pessimistic prediction of the beamforming gain is made sufficiently accurate for guaranteed reliability as well as the transmit-energy efficiency. Index Terms—Chernoff bound, beamforming gain, non-central χ2-distribution, reliability, multi-input multi-output (MIMO).
Signal detection in large multiple-input multiple-output (large-MIMO) systems presents greater challenges compared to conventional massive-MIMO for two primary reasons. First, large-MIMO systems lack favorable propagation conditions as they do not require a substantially greater number of service antennas relative to user antennas. Second, the wireless channel may exhibit spatial non-stationarity when an extremely large aperture array (ELAA) is deployed in a large-MIMO system. In this paper, we propose a scalable iterative large-MIMO detector named ANPID, which simultaneously delivers 1) close to maximum-likelihood detection performance, 2) low computational-complexity (i.e., square-order of transmit antennas), 3) fast convergence, and 4) robustness to the spatial non-stationarity in ELAA channels. ANPID incorporates a damping demodulation step into stationary iterative (SI) methods and alternates between two distinct demodulated SI methods. Simulation results demonstrate that ANPID fulfills all the four features concurrently and outperforms existing low-complexity MIMO detectors, especially in highly-loaded large-MIMO systems.
In this paper, we optimize both a very-low Earth orbit (VLEO) satellite mega-constellation and a low Earth orbit (LEO) satellite mega-constellation to achieve a high-rank channel matrix in line-of-sight (LOS) conditions for satellite-to-mobile communications. The optimization of these constellations is achieved through the spacing of the satellites in adjacent planes. The significance of the optimization is that it creates a high-rank channel matrix that delivers the spatial gain in the distributed multiple-input-multiple-output (MIMO). The distributed MIMO is modeled as the 4 closest satellites to an unmodified mobile phone, where each satellite is assumed to be a single transmit antenna. For each second the satellites fly over the receiver's location, a Monte Carlo simulation of the Rician flat fading channel was completed to obtain the mean channel to calculate the capacity. The simulations have shown achievable peak data rates of 177.2 Mbps for the n255 frequency band and 140.9 Mbps for the n256 frequency band at VLEO of 360km and the LEO we obtained peak data rates of 135.1 Mbps and 102.0 Mbps for the n255 and n256 frequency bands respectively.
This paper presents a novel frequency-domain energy detection scheme based on extreme statistics for robust sensing of OFDM sources in the low SNR region. The basic idea is to exploit the frequency diversity gain inherited by frequency selective channels with the aid of extreme statistics of the differential energy spectral density (ESD). Thanks to the differential stage the proposed spectrum sensing is robust to noise uncertainty problem. The low computational complexity requirement of the proposed technique makes it suitable for even machine-to-machine sensing. Analytical performance analysis is performed in terms of two classical metrics, i.e. probability of detection and probability of false alarm. The computer simulations carried out further show that the proposed scheme outperforms energy detection and second order cyclostationarity based approach for up to 10 dB gain in the low SNR range. © 2011 IEEE.
In this paper, a novel spatially non-stationary channel model is proposed for link-level computer simulations of massive multiple-input multiple-output (mMIMO) with extremely large aperture array (ELAA). The proposed channel model allows a mix of none line-of-sight (NLoS) and LoS links between a user and service antennas. The NLoS/LoS state of each link is characterized by a binary random variable, which obeys a correlated Bernoulli distribution. The correlation is described in the form of an exponentially decaying window. In addition, the proposed model incorporates shadowing effects which are non-identical for NLoS and LoS states. It is demonstrated, through computer emulation, that the proposed model can capture almost all spatially non-stationary fading behaviors of the ELAA-mMIMO channel. Moreover, it has a low implementational complexity. With the proposed channel model, Monte-Carlo simulations are carried out to evaluate the channel capacity of ELAAmMIMO. It is shown that the ELAA-mMIMO channel capacity has considerably different stochastic characteristics from the conventional mMIMO due to the presence of channel spatial non-stationarity.
Cooperative communications can exploit distributed spatial diversity gain to improve link performance. When the message is coded at a low rate, source and relay can send different parts of a codeword to destination. This is referred to as the coded cooperation. In this paper, we propose two novel coded cooperation schemes for three-node relay networks, i.e., adaptive coded cooperation and ARQ-based coded cooperation. The former one needs the channel quality information available at source. The codeword splits adaptively to minimize the overall BER. The latter one is devised for relay network with erasure. In the first time slot, source sends a high-rate sub-codeword. Once destination reports the decoding errors, either source or relay can send one or two new bits selected from the mother codeword. Unlike random rateless erasure codes, such as Fountain code, the proposed scheme is based on the deterministic code generator and puncture pattern. It is experimentally shown that the proposed scheme can offer improved throughput in comparison with the conventional approach.
This paper presents two contributions towards incremental decode-forward relaying over asymmetric fading channels. One is about the outage probability of incremental relay network accommodating i.n.d. cooperative paths. Our contribution is mainly on formulating a closed-form of the outage probability through employment of the Inverse Laplace Transform and Eular Summation. The other is about the proposal of transmit-power efficient relay-selection strategy through exploitation of the relationship between position of relays and the outage probability.
The objective of this work is to address one of the recent ITU AI/machine learning challenges, specifically on the RF power amplifier (PA) behavioral modelling. This paper presents a novel model namely real-valued time-delay temporal convo-lutional network (RVTDTCN) to learn the long-term memory effect from PA in 5G base-station transmitters. The proposed model employs dilated convolutions to incorporate information from various time stamps and capture intricate temporal dependencies. This is achieved without the requirement of additional coefficients. In our experiment, the ITU ML5G-PS-007 datasets collected from commercial 5G base-stations are employed for both training and testing purposes. The performance of the learned PA behavior is evaluated using both normalized mean square error (NMSE) and adjacent channel error power ratio (ACEPR). The results show that the RVTDTCN model achieves competitive performance with the state-of-the-art feedforward neural network (FNN) models but reducing the computational complexity by 60%. Moreover, the proposed model outperforms the state-of-the-art convolutional neural network (CNN) model by 2-3dB in NMSE and ACEPR, with a similar complexity. Index Terms—Power amplifier (PA), digital pre-distortion (DPD), neural network (NN), temporal convolutional network (TCN), real-valued time-delay temporal convolutional network (RVTDTCN), long-term memory effects, 5G.
This letter presents a reduced-complexity algorithm for coordinated beamforming aimed at solving the multicell downlink max-min signal-to- interference-plus-noise problem under per-base-station power constraints. It is shown that the proposed algorithm can achieve close performance to the optimum algorithm with faster convergence and lower complexity. © 2014 IEEE.
—In vehicle-to-infrastructure (V2I) networks, a cluster of multi-antenna access points (APs) can collaboratively conduct transmitter beamforming to provide data services (e.g., eMBB or URLLC). The collaboration between APs effectively forms a networked linear antenna-array with extra-large aperture (i.e., network-ELAA), where the wireless channel exhibits spatial non-stationarity. Major contribution of this work lies in the analysis of beamforming gain and radio coverage for network-ELAA non-stationary Rician channels considering the AP clustering. Assuming that: 1) the total transmit-power is fixed and evenly distributed over APs, 2) the beam is formed only based on the line-of-sight (LoS) path, it is found that the beamforming gain is concave to the cluster size. The optimum size of the AP cluster varies with respect to the user's location, channel uncertainty as well as data services. A user located farther from the ELAA requires a larger cluster size. URLLC is more sensitive to the channel uncertainty when comparing to eMBB, thus requiring a larger cluster size to mitigate the channel fading effect and extend the coverage. Finally, it is shown that the network-ELAA can offer significant coverage extension (50% or more in most of cases) when comparing with the single-AP scenario.
In this paper, the Hermite polynomials are employed to study linear approximation models of narrowband multiantenna signal reception (i.e., MIMO) with low-resolution quantizations. This study results in a novel linear approximation using the second-order Hermite expansion (SOHE). The SOHE model is not based on those assumptions often used in existing linear approximations. Instead, the quantization distortion is characterized by the second-order Hermite kernel, and the signal term is characterized by the first-order Hermite kernel. It is shown that the SOHE model can explain almost all phenomena and characteristics observed so far in the low-resolution MIMO signal reception. When the SOHE model is employed to analyze the linear minimum-mean-square-error (LMMSE) channel equalizer, it is revealed that the current LMMSE algorithm can be enhanced by incorporating a symbol-level normalization mechanism. The performance of the enhanced LMMSE algorithm is demonstrated through computer simulations for narrowband MIMO systems in Rayleigh fading channels.
This paper aims to investigate an intra-cell overlay opportunistic spectrum sharing scheme by employing 1-bit feedback beamforming. The work of interests is that base station broadcasts independent signal messages to two relay stations (RS-1 and RS-2). RS-2 decodes the signal messages in subcell 2 and attempts to share the spectrum of sub-cell 1 for its own transmission. For this reason, RS-2 makes a deal with RS-1 in sub-cell 1 to help RS-1 send its signal messages. As presented in the paper, by employing 1-bit feedback transmit beamforming, RS-2 can further improve RS-1's achievable rate and automatically eliminate the interference from RS-2 to subcell 1. Meanwhile, the achievable sum-rate upper bound of RS-2 is also analyzed. © VDE VERLAG GMBH.
In this paper, single-input multiple-output (SIMO) system when employing massive binary array-receiver has been investigated while constructive noise has been observed in the single user system to detect the higher-order QAM modulated signals. To fully understand the interesting phenomenon, mathematical model has been established and analyzed in this paper. Theorems of the signal detectability are studied to understand the best operating signal-to-noise ratio (SNR) range based on the error behaviours of the single user SIMO system. Within the observation and analysis, a novel new multiuser SIMO with binary array-receiver structure has been proposed and can be considered as a solution to deal with the high complexity problem that the traditional model has when using maximum likelihood (ML) detection. The key idea of this approach is to set up the multiuser multiple-input multiple-output (MIMO) model into a frequency division multiple access (FDMA) scenario and regard each user as single user SIMO to achieve the goal of decreasing the exponentially increased complexity of ML detection method to the number of users. It is shown by numerical results that each user in this system can achieve a promising error behaviour in the specific best operating SNR range.
The paper presents a time-difference-of-arrival (TDOA) position estimation algorithm for indoor positioning in the present of clock drift in a mobile terminal. Then, a new Cramér-Rao bound is derived as a benchmark of the algorithm. The simulation results show that an acceptable positioning accuracy can be achieved when at least five access points in wireless local area networks are involved in positioning. Moreover, when the clock drift or the TDOA is considerably large, the proposed algorithm outperforms the algorithm without considering the clock drift. © VDE VERLAG GMBH.
Guessing random additive noise decoding (GRAND) is a universal decoding that estimates the transmitted codewords by guessing the noise bit sequences and reversing their impact until legitimate codewords are found. This paper investigates one of its variants, namely Soft-GRAND (or SGRAND) of Polar codes over an interference channel where power domain non-orthogonal multiple access (NOMA) channel access protocol is in operation. Monte Carlo simulation results confirm the robustness of the SGRAND decoding over these channels. More specifically, the joint CRC-aided SGRAND decoding outperforms state-of-the-art CRC-aided list decoding (of Polar codes) with a list size of 32 by approximately 0.5 dB and 1.5 dB at block error rate (BLER) of 10-4 over additive white Gaussian noise (AWGN) and Rayleigh fading channels, respectively, and also offers lower complexity at mid-to-high signal to noise ratios (beyond 5 dB).
In this paper, a novel spatially non-stationary fading channel model is proposed for multiple-input multiple-output (MIMO) system with extremely-large aperture service-array (ELAA). The proposed model incorporates three key factors which cause the channel spatial non-stationarity: 1) link-wise path-loss; 2) shadowing effect; 3) line-of-sight (LoS)/non-LoS state. With appropriate parameter configurations, the proposed model can be used to generate computer-simulated channel data that matches the published measurement data from practical ELAA-MIMO channels. Given such appealing results, the proposed fading channel model is employed to study the cumulative distribution function (CDF) of ELAA-MIMO channel capacity. For all of our studied scenarios, it is unveiled that the ELAA-MIMO channel capacity obeys the skew-normal distribution. Moreover, the channel capacity is also found close to the Gaussian or Weibull distribution, depending on users' geo-location and distribution. More specifically, for single-user equivalent scenarios or multiuser scenarios with short user-to-ELAA distances (e.g., 1 m), the channel capacity is close to the Gaussian distribution; and for others, it is close to the Weibull distribution. Finally, the proposed channel model is also employed to study the impact of channel spatial non-stationarity on linear MIMO receivers through computer simulations. The proposed fading channel model is available at https://github.com/ELAA-MIMO/non-stationary-fading-channel-model.
The Wireless Hybrid Enhanced Mobile Radio Estimators (WHERE) consortium researches radio positioning techniques to improve various aspects of communications systems. In order to provide the benefits of position information available to communications systems, hybrid data fusion (HDF) techniques estimate reliable position information. Within this paper, we first present the scenarios and radio technologies evaluated by the WHERE consortium for wireless positioning. We compare conventional HDF approaches with two novel approaches developed within the framework of WHERE. Yet, HDF may still provide insufficient localization accuracy and reliability. Hence, we will research and develop new cooperative positioning algorithms, which exploit the available communications links among mobile terminals of heterogeneous wireless networks, to further enhance the positioning accuracy and reliability.
The reflective elements on an intelligent reconfigurable surface (IRS) can be tuned to improve the propagation environment and, in turn, the system performance, provided reliable channel information. However, IRS also brings challenges in terms of channel estimation by creating a very large dimensional channel between the IRS and the transmitter/receiver. A channel size being proportional to the number of users, user equipments (UE) antennas, IRS phase shifters, base station (BS) antennas, in an IRS-aided multi-user (MU) multiple-input-multiple-output (MIMO) system. Estimating such a large channel implies a huge amount of training overheads when all IRS phase shifters are passive, or requires extra power consumption and prohibitive hardware complexity, when IRS phase shifters are active. To tackle these challenges, this paper proposes a practical channel estimation process, based on a realistic codebook design constraint, for optimizing the IRS reflective elements in a MU-MIMO scenario. More specifically, we propose two novel machine-learning based algorithms (i.e. a deep supervised and a deep reinforcement) for optimizing the reflective elements of a typical passive IRS as well as a reliable channel estimation technique for IRS. Deep Supervised network uses exhaustive search to try every reflection pattern to train the network while the reinforcement network uses Q-learning to get the best reward. Our two algorithms can use the imperfect estimated channel knowledge to optimize the IRS in terms of sum-rate or minimum rate among all users. Simulation results show that our practical algorithms can achieve sum-rate and minimum rate performances close to the theoretical ones.
In this paper, a novel approach, namely realcomplex hybrid modulation (RCHM), is proposed to scale up multiuser multiple-input multiple-output (MU-MIMO) detection with particular concern on the use of equal or approximately equal service antennas and user terminals. By RCHM, we mean that user terminals transmit their data sequences with a mix of real and complex modulation symbols interleaved in the spatial and temporal domain. It is shown, through the system outage probability, RCHM can combine the merits of real and complex modulations to achieve the best spatial diversity-multiplexing trade-off that minimizes the required transmit-power given a sum-rate. The signal pattern of RCHM is optimized with respect to the real-to-complex symbol ratio as well as power allocation. It is also shown that RCHM equips the successive interference canceling MU-MIMO receiver with near-optimal performances and fast convergence in Rayleigh fading channels. This result is validated through our mathematical analysis of the average biterror- rate as well as extensive computer simulations considering the case with single or multiple base-stations.
Deep learning is driving a radical paradigm shift in wireless communications, all the way from the application layer down to the physical layer. Despite this, there is an ongoing debate as to what additional values artificial intelligence (or machine learning) could bring to us, particularly on the physical layer design; and what penalties there may have? These questions motivate a fundamental rethinking of the wireless modem design in the artificial intelligence era. Through several physical-layer case studies, we argue for a significant role that machine learning could play, for instance in parallel error-control coding and decoding, channel equalization, interference cancellation, as well as multiuser and multiantenna detection. In addition, we will also discuss the fundamental bottlenecks of machine learning as well as their potential solutions in this paper.
—Concerning ultra-reliable low-latency communication (URLLC) for the downlink operating in the frequency-division multiple-access with random channel assignment, a lightweight power allocation approach is proposed to maximize the number of URLLC users subject to transmit-power and individual user-reliability constraints. Provided perfect channel-state-information at the transmitter (CSIT), the proposed approach is proven to ensure maximized URLLC users. Assuming imperfect CSIT, the proposed approach still aims to maximize the URLLC users without compromising the individual user reliability by using a pessimistic evaluation of the channel gain. It is demonstrated , through numerical results, that the proposed approach can significantly improve the user capacity and the transmit-power efficiency in Rayleigh fading channels. With imperfect CSIT, the proposed approach can still provide remarkable user capacity at limited cost of transmit-power efficiency.
Sparse code multiple access (SCMA) is a promising code-domain non-orthogonal multiple access (NOMA) scheme for the enabling of massive machine-type communication. In SCMA, the design of good sparse codebooks and efficient multiuser decoding have attracted tremendous research attention in the past few years. This paper aims to leverage deep learning to jointly design the downlink SCMA encoder and decoder with the aid of autoencoder. We introduce a novel end-to-end learning based SCMA (E2E-SCMA) design framework, under which improved sparse codebooks and low-complexity decoder are obtained. Compared to conventional SCMA schemes, our numerical results show that the proposed E2E-SCMA leads to significant improvements in terms of error rate and computational complexity. Index Terms SCMA, codebook design, deep neural network, autoencoder, multi-task learning.
In this paper, an orthogonal stochastic gradient descent (O-SGD) based learning approach is proposed to tackle the wireless channel over-training problem inherent in artificial neural network (ANN)-assisted MIMO signal detection. Our basic idea lies in the discovery and exploitation of the training-sample orthogonality between the current training epoch and past training epochs. Unlike the conventional SGD that updates the neural network simply based upon current training samples, O-SGD discovers the correlation between current training samples and historical training data, and then updates the neural network with those uncorrelated components. The network updating occurs only in those identified null subspaces. By such means, the neural network can understand and memorize uncorrelated components between different wireless channels, and thus is more robust to wireless channel variations. This hypothesis is confirmed through our extensive computer simulations as well as performance comparison with the conventional SGD approach.
In this paper, a novel unsupervised deep learning approach is proposed to tackle the multiuser frequency synchronization problem inherent in orthogonal frequency-division multiple-access (OFDMA) uplink communications. The key idea lies in the use of the feed-forward deep neural network (FF-DNN) for multiuser interference (MUI) cancellation taking advantage of their strong classification capability. Basically, the proposed FF-DNN consists of two essential functional layers. One is called carrier-frequency-offsets (CFOs) classification layer that is responsible for identifying the users’ CFO range, and another is called MUI-cancellation layer responsible for joint multiuser detection (MUD) and frequency synchronization. By such means, the proposed FF-DNN approach showcases remarkable MUIcancellation performances without the need of multiuser CFO estimation. In addition, we also exhibit an interesting phenomenon occurred at the CFO-classification stage, where the CFO-classification performance get improved exponentially with the increase of the number of users. This is called multiuser diversity gain in the CFO-classification stage, which is carefully studied in this paper.