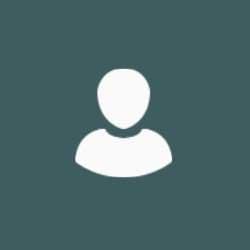
Dr Xihan Bian
Publications
The ability to generalize to previously unseen tasks with little to no supervision is a key challenge in modern machine learning research. It is also a cornerstone of a future "General AI". Any artificially intelligent agent deployed in a real world application, must adapt on the fly to unknown environments. Researchers often rely on reinforcement and imitation learning to provide online adaptation to new tasks, through trial and error learning. However, this can be challenging for complex tasks which require many timesteps or large numbers of subtasks to complete. These "long horizon" tasks suffer from sample inefficiency and can require extremely long training times before the agent can learn to perform the necessary longterm planning. In this work, we introduce CASE which attempts to address these issues by training an Imitation Learning agent using adaptive "near future" subgoals. These subgoals are recalculated at each step using compositional arithmetic in a learned latent representation space. In addition to improving learning efficiency for standard long-term tasks, this approach also makes it possible to perform one-shot generalization to previously unseen tasks, given only a single reference trajectory for the task in a different environment. Our experiments show that the proposed approach consistently outperforms the previous state-of-the-art compositional Imitation Learning approach by 30%.
Conference Title: 2022 IEEE/RSJ International Conference on Intelligent Robots and Systems (IROS) Conference Start Date: 2022, Oct. 23 Conference End Date: 2022, Oct. 27 Conference Location: Kyoto, JapanIn this work, we introduce a new perspective for learning transferable content in multi-task imitation learning. Humans are capable of transferring skills and knowledge. If we can cycle to work and drive to the store, we can also cycle to the store and drive to work. We take inspiration from this and hypothesize the latent memory of a policy network can be disentangled into two partitions. These contain either the knowledge of the environmental context for the task or the generalisable skill needed to solve the task. This allows an improved training efficiency and better generalization over previously unseen combinations of skills in the same environment, and the same task in unseen environments. We used the proposed approach to train a disentangled agent for two different multi-task IL environments. In both cases, we out-performed the SOTA by 30% in task success rate. We also demonstrated this for navigation on a real robot.
Robots need to be able to work in multiple different environments. Even when performing similar tasks, different behaviour should be deployed to best fit the current environment. In this paper, We propose a new approach to navigation, where it is treated as a multi-task learning problem. This enables the robot to learn to behave differently in visual navigation tasks for different environments while also learning shared expertise across environments. We evaluated our approach in both simulated environments as well as real-world data. Our method allows our system to converge with a 26% reduction in training time, while also increasing accuracy.
In this work, we introduce a new perspective for learning transferable content in multi-task imitation learning. Humans are able to transfer skills and knowledge. If we can cycle to work and drive to the store, we can also cycle to the store and drive to work. We take inspiration from this and hypothesize the latent memory of a policy network can be disentangled into two partitions. These contain either the knowledge of the environmental context for the task or the generalizable skill needed to solve the task. This allows improved training efficiency and better generalization over previously unseen combinations of skills in the same environment, and the same task in unseen environments. We used the proposed approach to train a disentangled agent for two different multi-task IL environments. In both cases we out-performed the SOTA by 30% in task success rate. We also demonstrated this for navigation on a real robot.