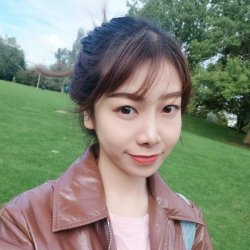
Xiaoqi Nong
About
My research project
To be confirmedTo be confirmed.
To be confirmed.
Publications
The development of industrial automation is closely related to the evolution of mobile robot positioning and navigation mode. In this paper, we introduce ASL-SLAM, the first line-based SLAM system operating directly on robots using the event sensor only. This approach maximizes the advantages of the event information generated by a bio-inspired sensor. We estimate the local Surface of Active Events (SAE) to get the planes for each incoming event in the event stream. Then the edges and their motion are recovered by our line extraction algorithm. We show how the inclusion of event-based line tracking significantly improves performance compared to state-of-the-art frame-based SLAM systems. The approach is evaluated on publicly available datasets. The results show that our approach is particularly effective with poorly textured frames when the robot faces simple or low texture environments. We also experimented with challenging illumination situations to order to be suitable for various industrial environments, including low-light and high motion blur scenarios. We show that our approach with the event-based camera has natural advantages and provides up to 85% reduction in error when performing SLAM under these conditions compared to the traditional approach.
The development of industrial automation is closely related to the evolution of mobile robot positioning and navigation mode. In this paper, we introduce ASL-SLAM, the first line-based SLAM system operating directly on robots using the event sensor only. This approach maximizes the advantages of the event information generated by a bio-inspired sensor. We estimate the local Surface of Active Events (SAE) to get the planes for each incoming event in the event stream. Then the edges and their motion are recovered by our line extraction algorithm. We show how the inclusion of event-based line tracking significantly improves performance compared to state-of-the-art frame-based SLAM systems. The approach is evaluated on publicly available datasets. The results show that our approach is particularly effective with poorly textured frames when the robot faces simple or low texture environments. We also experimented with challenging illumination situations to order to be suitable for various industrial environments, including low-light and high motion blur scenarios. We show that our approach with the event-based camera has natural advantages and provides up to 85% reduction in error when performing SLAM under these conditions compared to the traditional approach.