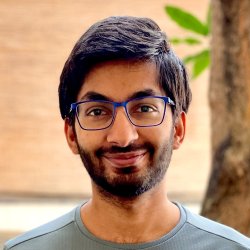
Umar Marikkar
Academic and research departments
Surrey Institute for People-Centred Artificial Intelligence (PAI).About
My research project
Foundation models for understanding medical data"Foundation models like "BERT: Pre-training of deep Bidirectional Transformers for Language Understanding" & "Generative Pretraining Transformer: Improving language understanding by generative pre-training (GPT-N)" have transformed natural language processing (NLP). However, the foundation models for vision started to emerge 2.5 years later at the beginning of 2021 with the introduction of group masked model learning (GMML) in "SiT: Self-supervised vision Transformer". The use of these foundation models in healthcare is underexplored.
The research project proposes to study the role of these foundation models in multimodal healthcare analysis and develop Machine Learning models for classifying cardiopulmonary conditions (e.g. Pneumonia) using multimodal data: vital signs, chest X-rays and meta-data including Electronic Health Records. The project will use recently published large database (MIMIC-CXR) of 377,110 images for 65,379 patients presenting to the Emergency department in Boston between 2011-2016. Each imaging study contains one or more images, typically frontal and lateral views (over 65 %). A recent work of the current team (CLMIU: Commonsense Learning in Multimodal Image Understanding) has established that using foundation models for vision & NLP for vision-language pre-training is more beneficial and
has already alleviated the need of object detector which is considered as a critical pre-processing step for visual input. The PhD research will build advanced multimodal healthcare analysis algorithms suitable for several downstream applications by building upon foundation models. Some of the downstream healthcare application can include, classification, detection, segmentation, grounding of disease in imaging data and unsupervised discovery of patterns. Due to healthcare, a particular emphasis will be given to the explainability of the decisions made by the algorithms.
Supervisors
"Foundation models like "BERT: Pre-training of deep Bidirectional Transformers for Language Understanding" & "Generative Pretraining Transformer: Improving language understanding by generative pre-training (GPT-N)" have transformed natural language processing (NLP). However, the foundation models for vision started to emerge 2.5 years later at the beginning of 2021 with the introduction of group masked model learning (GMML) in "SiT: Self-supervised vision Transformer". The use of these foundation models in healthcare is underexplored.
The research project proposes to study the role of these foundation models in multimodal healthcare analysis and develop Machine Learning models for classifying cardiopulmonary conditions (e.g. Pneumonia) using multimodal data: vital signs, chest X-rays and meta-data including Electronic Health Records. The project will use recently published large database (MIMIC-CXR) of 377,110 images for 65,379 patients presenting to the Emergency department in Boston between 2011-2016. Each imaging study contains one or more images, typically frontal and lateral views (over 65 %). A recent work of the current team (CLMIU: Commonsense Learning in Multimodal Image Understanding) has established that using foundation models for vision & NLP for vision-language pre-training is more beneficial and
has already alleviated the need of object detector which is considered as a critical pre-processing step for visual input. The PhD research will build advanced multimodal healthcare analysis algorithms suitable for several downstream applications by building upon foundation models. Some of the downstream healthcare application can include, classification, detection, segmentation, grounding of disease in imaging data and unsupervised discovery of patterns. Due to healthcare, a particular emphasis will be given to the explainability of the decisions made by the algorithms.