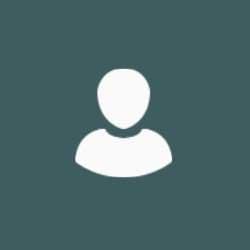
Dr Sylvain Delahaies
About
Biography
PhD, University of Surrey. Complex and contact geometry in geophysical fluid dynamics.
Msc in Hydroinformatics, department of Mathematics, University of Surrey.
DEA (Msc) Mathematiques et applications, LAREMA, Université d'Angers.
ResearchResearch interests
Member of the NCEO Theme 7: Data Assimilation, I am working on data assimilation techniques for the Carbon Cycle.
Over the last decade, several studies have demonstrated the ability of various data assimilation techniques to improve the quality of carbon fluxes predictions for terrestrial ecosystems. Williams et al. (2005), Quaife et al. (2009), Fox et al. (2009) and Hill et al. (2011) among others, used the data linked ecosystem (DALEC) model as a C-cycle model to conduct these model data fusion experiments. The DALEC model is a simple box model describing the evolution a evergreen forest ecosystem represented by five carbon pools. The gross primary production (GPP) is calculated at a daily time step as a function of the Carbon foliage pool and meteorological drivers, it is then allocated to each pools and respiration (autotrophic and heterotrophic) via fluxes.
The net ecosystem exchange (NEE) defined as the difference between the GPP and respirations provides information on the amount of carbon in the atmosphere due to the forest ecosystem. The GPP process, the allocation to pools and the respirations are controlled by a set of 11 parameters, different for each ecosystem. The studies conducted thus far used NEE observations and leaf area index (LAI) observations, to confront the DALEC model to observations, and data assimilation methods such as ensemble Kalman filter (EnKF) (Quaife (2009) ...) or Monte Carlo Markov chains methods (MCMC). Our work aims at assessing the validity of 4D variational (4DVar) techniques and adjoint methods for DALEC in comparison with the previous studies. 4DVar data assimilation finds the trajectory that best combines a background estimation of the control variable, the model and observations.
The optimal control variable is obtained by minimizing a cost function defined by the square of the model fit to observations and background weighted by the inverse of their respective error covariance matrix. When Gaussian errors are applied and when the model and observation operators are weakly nonlinear the Hessian of the cost function provides a good estimate of the error covariance matrix of the optimal control variable. Theoretically, adjoint methods provide a useful tool to perform the minimization of the cost function and to compute the Hessian as well as to derive sensitivity informations.
Our work is organized around three main axes:
1- Initial pools estimation, parameter calibration, and quantifying the uncertainty of these analysis: Meteorological drivers are provided by the REFLEX experiment at an evergreen forest site for a 3 years period. These fields are resampled to provide meteorological drivers for longer time windows (15 years, 30 years). NEE and LAI observations are then generated using a forward run of DALEC model and introducing gaps and noise in the observations. Using synthetic observations offers many advantages for our purpose: they are generated at (almost) no cost and (quasi) instantly; we keep the complete knowledge of the initial conditions, of the parameters and of the errors used to generate them. The first experiments assume perfect observations, the minimization of the cost function is performed using a conjugate gradient method. The gradient is computed using the adjoint of DALEC. Code was derived for the adjoint model and tested against automatic differentiation tools (OpenAD, Tapenade). The results of these experiments, in agreement with the previous studies, are subject of a paper in preparation.
2- Sensitivity of the analysis (or any quantity derived from the analysis) to observations (or other parameters). Within NCEO, Hill & Ryan (submitted) perform statistical studies to compare the quality of the prediction of NEE using EnKF and MCMC methods with different observations sets, varying the time window for the observations and their sparsity. The adjoint of DALEC provides us with a useful framework to address this question, the information content of the observations can be obtained via the sensitivity equations described by Daescu (2007). We apply this formalism with the settings used by Hill et al. to build comparison between assimilation techniques. Results of this work are subject of a paper in preparation.
3- Setting the plan for a coupling of DALEC with a chemical transport model to perform a global inversion experiment: a large effort was put on developing an appropriate transport model for a toy inversion experiment for multiple forest sites spread over a sub continental region (eg Northern Europe). At first, the experiment was intended to use a simple and easy to code, though non trivial transport model. However the need for a model capable of simulating seasonal variability of temperatures and solar radiation (responsible for the most part of the dynamics) as well as wind fields at each sites led us to reconsider this choice. The setting of this experiment is still in preparation.
This work, supervised by Prof. I. Roulstone is carried out in collaboration with A. Chutter, A. Skeldon and P. Aston at Surrey, T. Hill and M. Williams members of NCEO theme 2 at Edinburgh university, and members of NCEO theme 3: Atmospheric composition.
References
Fox, A, M Williams, AD Richardson, D Cameron, JH Gove, T Quaife, D Ricciuto, M Reichstein, E Tomelleri, C Trudinger, MT van Wijk. The REFLEX project: comparing different algorithms and implementations for the inversion of a terrestrial ecosystem model against eddy covariance data, Agricultural and Forest Meteorology 149, 1597-1615, 2009.
Hill, T.C., E. Ryan and M. Williams. The use of CO2 flux time series for parameter and carbon stock estimation in carbon cycle research. Global Change Biology, 2011.
Quaife T., Lewis P., De Kauwe M., Williams M., Law B. E., Disney M., Bowyer P. Assimilating canopy reflectance data into an ecosystem model with an Ensemble Kalman FilterRemote Sensing of Environment 112, 1347-1364, 2008.
Williams, M., P.A. Schwarz, B.E. Law, J. Irvine & M. Kurpius. An improved analysis of forest carbon dynamics using data assimilation. Global Change Biology 11, 89-105, 2005.
Research interests
Member of the NCEO Theme 7: Data Assimilation, I am working on data assimilation techniques for the Carbon Cycle.
Over the last decade, several studies have demonstrated the ability of various data assimilation techniques to improve the quality of carbon fluxes predictions for terrestrial ecosystems. Williams et al. (2005), Quaife et al. (2009), Fox et al. (2009) and Hill et al. (2011) among others, used the data linked ecosystem (DALEC) model as a C-cycle model to conduct these model data fusion experiments. The DALEC model is a simple box model describing the evolution a evergreen forest ecosystem represented by five carbon pools. The gross primary production (GPP) is calculated at a daily time step as a function of the Carbon foliage pool and meteorological drivers, it is then allocated to each pools and respiration (autotrophic and heterotrophic) via fluxes.
The net ecosystem exchange (NEE) defined as the difference between the GPP and respirations provides information on the amount of carbon in the atmosphere due to the forest ecosystem. The GPP process, the allocation to pools and the respirations are controlled by a set of 11 parameters, different for each ecosystem. The studies conducted thus far used NEE observations and leaf area index (LAI) observations, to confront the DALEC model to observations, and data assimilation methods such as ensemble Kalman filter (EnKF) (Quaife (2009) ...) or Monte Carlo Markov chains methods (MCMC). Our work aims at assessing the validity of 4D variational (4DVar) techniques and adjoint methods for DALEC in comparison with the previous studies. 4DVar data assimilation finds the trajectory that best combines a background estimation of the control variable, the model and observations.
The optimal control variable is obtained by minimizing a cost function defined by the square of the model fit to observations and background weighted by the inverse of their respective error covariance matrix. When Gaussian errors are applied and when the model and observation operators are weakly nonlinear the Hessian of the cost function provides a good estimate of the error covariance matrix of the optimal control variable. Theoretically, adjoint methods provide a useful tool to perform the minimization of the cost function and to compute the Hessian as well as to derive sensitivity informations.
Our work is organized around three main axes:
1- Initial pools estimation, parameter calibration, and quantifying the uncertainty of these analysis: Meteorological drivers are provided by the REFLEX experiment at an evergreen forest site for a 3 years period. These fields are resampled to provide meteorological drivers for longer time windows (15 years, 30 years). NEE and LAI observations are then generated using a forward run of DALEC model and introducing gaps and noise in the observations. Using synthetic observations offers many advantages for our purpose: they are generated at (almost) no cost and (quasi) instantly; we keep the complete knowledge of the initial conditions, of the parameters and of the errors used to generate them. The first experiments assume perfect observations, the minimization of the cost function is performed using a conjugate gradient method. The gradient is computed using the adjoint of DALEC. Code was derived for the adjoint model and tested against automatic differentiation tools (OpenAD, Tapenade). The results of these experiments, in agreement with the previous studies, are subject of a paper in preparation.
2- Sensitivity of the analysis (or any quantity derived from the analysis) to observations (or other parameters). Within NCEO, Hill & Ryan (submitted) perform statistical studies to compare the quality of the prediction of NEE using EnKF and MCMC methods with different observations sets, varying the time window for the observations and their sparsity. The adjoint of DALEC provides us with a useful framework to address this question, the information content of the observations can be obtained via the sensitivity equations described by Daescu (2007). We apply this formalism with the settings used by Hill et al. to build comparison between assimilation techniques. Results of this work are subject of a paper in preparation.
3- Setting the plan for a coupling of DALEC with a chemical transport model to perform a global inversion experiment: a large effort was put on developing an appropriate transport model for a toy inversion experiment for multiple forest sites spread over a sub continental region (eg Northern Europe). At first, the experiment was intended to use a simple and easy to code, though non trivial transport model. However the need for a model capable of simulating seasonal variability of temperatures and solar radiation (responsible for the most part of the dynamics) as well as wind fields at each sites led us to reconsider this choice. The setting of this experiment is still in preparation.
This work, supervised by Prof. I. Roulstone is carried out in collaboration with A. Chutter, A. Skeldon and P. Aston at Surrey, T. Hill and M. Williams members of NCEO theme 2 at Edinburgh university, and members of NCEO theme 3: Atmospheric composition.
References
Fox, A, M Williams, AD Richardson, D Cameron, JH Gove, T Quaife, D Ricciuto, M Reichstein, E Tomelleri, C Trudinger, MT van Wijk. The REFLEX project: comparing different algorithms and implementations for the inversion of a terrestrial ecosystem model against eddy covariance data, Agricultural and Forest Meteorology 149, 1597-1615, 2009.
Hill, T.C., E. Ryan and M. Williams. The use of CO2 flux time series for parameter and carbon stock estimation in carbon cycle research. Global Change Biology, 2011.
Quaife T., Lewis P., De Kauwe M., Williams M., Law B. E., Disney M., Bowyer P. Assimilating canopy reflectance data into an ecosystem model with an Ensemble Kalman FilterRemote Sensing of Environment 112, 1347-1364, 2008.
Williams, M., P.A. Schwarz, B.E. Law, J. Irvine & M. Kurpius. An improved analysis of forest carbon dynamics using data assimilation. Global Change Biology 11, 89-105, 2005.
Teaching
- L3 MAT3015 : Numerical solution for partial differential equations
Publications
Additional publications
S.B. Delahaies, P. Hydon, Multi-symplectic formulation of near-local Hamiltonian balanced models, Proc. R. Soc. A (2011).
S.B. Delahaies, I. Roulstone, Hyper-Kaehler geometry and semi-geostrophic theory, Proc. R. Soc. Lond. A (2009).