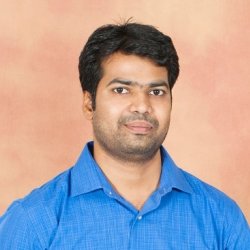
Dr Suryakanta Biswal
About
Biography
Dr Suryakanta Biswal joined the Department of Civil and Environmental Engineering as a Research Fellow, and is working in Structural Health Monitoring, a project funded by the Engineering & Physical Sciences Research Council.
Suryakanta has a PhD along with a Masters degree from Indian Institute of Science Bangalore. His thesis was on “Uncertainty based damage identification and prediction of long-time deformation in concrete structures”. In this work algorithms were developed for identifying different types of damages in concrete structures, when various types of uncertainties (both aleatory and epistemic) were present in the measurement, in the finite element model, and in the prior distribution of the parameters in the finite element model. The damages considered in this work were loss of stiffness, loss of mass, and loss of bond between concrete and steel. The other major section of this work dealt with predicting the long-time prestress losses in post-tensioned concrete beams and slabs, given the uncertainties in the measurements taken during the short-time period.
Suryakanta has worked as a Research Fellow in the BRNS/DAE, India Project entitled “Development of a Model for Evaluating Prestress Losses Considering Creep & Shrinkage Losses in Concrete & Relaxation Losses in Steel over 100 Years”, from SEPTEMBER 2012 TO March 2015. The last one year he has been working as Lecturer at SSN College of Engineering, Chennai.
Suryakanta has years of experience in scientific programming (mainly Matlab), and commercial software for finite element analysis (mainly ANSYS and ABAQUS), and is happy to help other colleagues with this.
Areas of specialism
My qualifications
Previous roles
Affiliations and memberships
Publications
Deep learning algorithms are transforming a variety of research areas with accuracy levels that the traditional methods cannot compete with. Recently, increasingly more research efforts have been put into the structural health monitoring domain. In this work, we propose a new deep convolutional neural network, namely SHMnet, for a challenging structural condition identification case, that is, steel frame with bolted connection damage. We perform systematic studies on the optimisation of network architecture and the preparation of the training data. In the laboratory, repeated impact hammer tests are conducted on a steel frame with different bolted connection damage scenarios, as small as one bolt loosened. The time-domain monitoring data from a single accelerometer are used for training. We conduct parametric studies on different layer numbers, different sensor locations, the quantity of the training datasets and noise levels. The results show that the proposed SHMnet is effective and reliable with at least four independent training datasets and by avoiding vibration node points as sensor locations. Under up to 60% additive Gaussian noise, the average identification accuracy is over 98%. In comparison, the traditional methods based on the identified modal parameters inevitably fail due to the unnoticeable changes of identified natural frequencies and mode shapes. The results provide confidence in using the developed method as an effective structural condition identification framework. It has the potential to transform the structural health monitoring practice. The code and relevant information can be found at https://github.com/capepoint/SHMnet.
Gravity-based foundations are an attractive solution for certain offshore sites due to various environment-friendly reasons: no-piling, low energy installation through float-sink, less noise installation, use of low-carbon concrete, and increased use of local content. These types of foundations have been used in the past for supporting oil and gas installations. This chapter provides a simplified design approach for foundation sizing to assess financial viability at the preliminary stage. An example is provided to show the importance of steps in design.
•HTCM model in a Bayesian framework for creep and shrinkage in concrete.•Uncertainty reduction by Bayesian inference and global response sensitivity.•Validation using experiments in Northwestern University database.•Estimation of long-time prestress losses in concrete beams and slabs. Prestress losses occur in concrete structures due to long-time creep and shrinkage in concrete, and stress relaxation in prestressing strands. The existing formulations for creep and shrinkage modeling in concrete are empirical in nature, which have been developed based on a particular set of measured data. In this study a coupled hygro-thermo-mechanical formulation is used to model the physics associated with creep and shrinkage in concrete structures. Uncertainty tools based on a probabilistic framework are used to model the possible variations in the input variables in the hygro-thermo-mechanical formulation. A distance measure based global response sensitivity analysis is adopted to identify the parameters having maximum impact on the output responses. Uncertainties in the selected set of important variables are reduced using Bayesian inference and short-time measured responses. For validating efficiency of the uncertainty reduction technique adopting Bayesian inference and global response sensitivity, the predictions of creep and shrinkage in concrete are compared with a few experiments from the North-Western University (NU) data base. The uncertainty reduction technique is then used to predict the long-time prestress losses in post-tensioned concrete beams and slabs cast in the laboratory.
Prestressed concrete structures are widely used in long span bridge girders and nuclear power plants. Long-time creep and shrinkage in concrete cause loss of prestress forces in the concrete structures. The creep and shrinkage models existing in the literature are empirical in nature. Each model has been developed on the basis of an arbitrary selection of data sets among all the data sets available. In this study it is claimed that each model contains some information about the creep and shrinkage patterns in the concrete structures. A single best model is developed in this study using all existing models, based on the concept of model averaging. In model averaging all the models are updated independently based on the short-time measurements. Weights are assigned to each model based on the likeliness of predictions from that model to the actual measurements. The time period for short-time measurements is evaluated using stochastic simulations. The proposed single best model for creep and shrinkage, and the time period for short-time measurements are (i) first validated against experiments reported in the Northwestern University (NU) database, and (ii) then used to predict the long-time losses of prestress forces in the concrete beams and slabs cast in the laboratory. (C) 2017 Elsevier Ltd. All rights reserved.
Failure of bolted connections in steel structures may result in catastrophic effects. Many algorithms in existing literature use modal information of a structure to identify damage in that structure, based on the data acquired from accelerometers which record the vibration time histories at different points on the structure. The location of these points may have significant effects on the quality of the acquired data, and thus the identified modal information. In this paper, a distance measure based Markov chain Monte Carlo algorithm is proposed to determine the optimal locations for the accelerometers, and the optimal location of the impact hammer if need. Different damage cases with various combinations of bolt failures are considered in this study. Failures at various levels are simulated by loosening the bolts in a predefined order. To compare the efficiency of the proposed method, the total effect of various damage cases on the accelerations at the optimal locations are calculated for the proposed method and a state-of-the-art method from the existing literature. The results demonstrate the efficiency of the proposed strategy in locating the accelerometers, which can produce data that are more sensitive to the bolted connection failures.
Structural condition identification based on monitoring data is important for automatic civil infrastructure asset management. Nevertheless, the monitoring data are almost always insufficient because the real-time monitoring data of a structure only reflect a limited number of structural conditions, while the number of possible structural conditions is infinite. With insufficient monitoring data, the identification performance may significantly degrade. This study aims to tackle this challenge by proposing a deep transfer learning (TL) approach for structural condition identification. It effectively integrates physics-based and data-driven methods by generating various training data based on the calibrated finite element (FE) model, pretraining a deep learning (DL) network, and transferring its embedded knowledge to the real monitoring/testing domain. Its performance is demonstrated in a challenging case, vibration-based condition identification of steel frame structures with bolted connection damage. First, disparate subsets of test data are used as training data, and the identification accuracy of the whole dataset is evaluated. The results demonstrate that the proposed approach can achieve high identification accuracy with limited types of training data, with the identification accuracy increasing up to 8.57%. Second, numerical simulation data are used as training data, and then different TL strategies and different DL architectures are compared on the performance of structural condition identification. The results show that even though the training data are from a different domain and with different types of labels, intrinsic physics can be learned through the pretraining process, and the TL results can be clearly improved, with the identification accuracy increasing from 81.8% to 89.1%. The comparative studies show that SHMnet with three convolutional layers stands out as the pretraining DL architecture, with 21.8% and 25.5% higher identification accuracy values over the other two networks, VGGNet-16 and ResNet-18. The findings of this study advance the potential application of the proposed approach towards expert-level condition identification based on limited real-world training data.
A steel frame is designed to measure the existing prestressing force in the concrete beams and slabs when embedded inside the concrete members. The steel frame is designed to work on the principles of a vibrating wire strain gauge and in the present study is referred to as a vibrating beam strain gauge (VBSG). The existing strain in the VBSG is evaluated using both frequency data on the stretched member and static strain corresponding to a fixed static load, measured using electrical strain gauges. The evaluated strain in the VBSG corresponds to the existing stain in the concrete surrounding the prestressing strands. The crack reopening load method is used to compute the existing prestressing force in the concrete members and is then compared with the existing prestressing force obtained from the VBSG at that section. Digital image correlation based surface deformation and change in neutral axis monitored by putting electrical strain gauges across the cross section, are used to compute the crack reopening load accurately. (C) 2016 Elsevier Ltd. All rights reserved.
This paper investigates the experimental response of digitally manufactured hollow timber columns incorporating dry mechanical connections (DMC). Timber plates were cut to complementary geometries and mechanically attached to interlock and form an integral joint. For this research, from existing DMC, rectangular tab and slot geometries were considered. Four sets of columns with different aspect ratios, were tested to assess the influence of member slenderness on the structural response, which has not been yet investigated. Complementary compression and tension samples, as well as single tab and slot joint shear and pull-out samples were also tested. After describing the design rationale and manufacturing methodology, the test results from more than forty material and structural tests are given. A full account of the deformational response, stiffness, strength and post-peak characteristics of each configuration is given. The material tests indicate both the compression and tension strengths of the plywood sheet are of similar magnitude in the range of 25 MPa. As expected, the tension failure was relatively brittle whilst crushing in compression was rather gradual due to the inherent properties of the engineered timber. The tab and slot joint shear strength was consistently above 6 MPa, whilst the friction strength around 0.5 MPa. Brittle failure was observed in all the column tests. The predominant failure modes were crushing close to the top or bottom of the columns, buckling of the tab-panels or slot-panels around mid-height, and separation of plies in the failed region. The load-shortening response indicated that the stiffness and strength of the DMC hollow timber columns reduces gradually with increasing slenderness. Relatively slender columns with an aspect ratio of 5.0, had about 22% less strength than stub columns with an aspect ratio of 1.0. These results provide a detailed insight into the structural performance of digitally manufactured hollow timber columns which can be employed in temporary lightweight structures that can be disassembled and reused.
Vibration-based condition identification of bolted connections can benefit the effective maintenance and operation of steel structures. Existing studies show that modal parameters are not sensitive to such damage as loss of preload. In contrast, structural responses in the time domain contain all the information regarding a structural system. Therefore, this study aims to exploit time-domain data directly for condition identification of bolted connection. Finite element (FE) model updating is carried out based on the vibration test data of a steel frame, with various combinations of bolts with loss of preload, representing different damage scenarios. It is shown that the match between the numerically simulated and measured acceleration responses of the steel frame cannot be achieved. The reason is that time-dependent nonlinearity is generated in bolted connections during dynamic excitation of the steel frame. To capture the nonlinearity, a virtual viscous damper is proposed. By using the proposed damper alongside the updated system matrices of the FE model, the time domain acceleration responses are estimated with great consistency with the measured responses. The results demonstrate that the proposed virtual damper is not only effective in estimating the time domain acceleration responses in each damage case, but also has the potential for condition identification of bolted connections with such small damage as just one bolt with loss of preload. It can also be applied to other challenging scenarios of condition identification, where modal parameters are not sensitive to the damage.
A finite element model updating technique based on measured strain responses is used to monitor the health of prestressed concrete structures. In this work health corresponds to the existing prestressing force present and the associated stiffness properties of the structure. Measurements are taken on post-tensioned beams, both bonded and un-bonded. The measured response is the total strain of the beams at predefined locations and the natural frequencies of the structures. In this work uncertainties in the measured data and those in the stiffness parameters are considered. The uncertainties are modeled using both Bayesian technique and interval analysis. The uncertain parameters in the finite element model are then estimated using Markov chain Monte Carlo technique in Bayesian framework and set inversion via interval analysis in interval analysis framework. The existing prestressing force estimated by both the mentioned techniques are then compared with the measured actual prestressing force present in the structure. To check the robustness of the proposed algorithm tests are conducted at different time spans corresponding to different prestress loss and different material stiffness.
Imprecise probability based methods are developed in this study for the parameter estimation, in finite element model updating for concrete structures, when the measurements are imprecisely defined. Bayesian analysis using Metropolis Hastings algorithm for parameter estimation is generalized to incorporate the imprecision present in the prior distribution, in the likelihood function, and in the measured responses. Three different cases are considered (i) imprecision is present in the prior distribution and in the measurements only, (ii) imprecision is present in the parameters of the finite element model and in the measurement only, and (iii) imprecision is present in the prior distribution, in the parameters of the finite element model, and in the measurements. Procedures are also developed for integrating the imprecision in the parameters of the finite element model, in the finite element software Abaqus. The proposed methods are then verified against reinforced concrete beams and prestressed concrete beams tested in our laboratory as part of this study. (C) 2017 Elsevier Ltd. All rights reserved.
The deep learning technologies have transformed many research areas with accuracy levels that the traditional methods are not comparable with. Recently, they have received increasing attention in the structural health monitoring (SHM) domain. In this paper, we aim to develop a new deep learning algorithm for structural condition monitoring and to evaluate its performance in a challenging case, bolt loosening damage in a frame structure. First, the design of a one-Dimensional Convolutional Neural Network (1DCNN) is introduced. Second, a series of impact hammer tests are conducted on a steel frame in the laboratory under ten scenarios, with bolts loosened at different locations and quantities. For each scenario, ten repeated tests are performed to provide enough training data for the algorithm. Third, the algorithm is trained with different quantities of training data (from one to seven test data for each scenario), and then is tested with the rest test data. The results show that the proposed 1D-CNN with three convolutional layers provide reliable identification results (over 95% accuracy) with sufficient training data sets. It has the potential to transform the SHM practice.
Large scale offshore wind farms are relatively new infrastructures and are being deployed in regions prone to earthquakes. Offshore wind farms comprise of both offshore wind turbines (OWTs) and balance of plants (BOP) facilities, such as inter-array and export cables, grid connection etc. An OWT structure can be either grounded systems (rigidly anchored to the seabed) or floating systems (with tension legs or catenary cables). OWTs are dynamically-sensitive structures made of a long slender tower with a top-heavy mass, known as Nacelle, to which a heavy rotating mass (hub and blades) is attached. These structures, apart from the variable environmental wind and wave loads, may also be subjected to earthquake related hazards in seismic zones. The earthquake hazards that can affect offshore wind farm are fault displacement, seismic shaking, subsurface liquefaction, submarine landslides, tsunami effects and a combination thereof. Procedures for seismic designing OWTs are not explicitly mentioned in current codes of practice. The aim of the paper is to discuss the seismic related challenges in the analysis and design of offshore wind farms and wind turbine structures. Different types of grounded and floating systems are considered to evaluate the seismic related effects. However, emphasis is provided on Tension Leg Platform (TLP) type floating wind turbine. Future research needs are also identified.
Vibration modal testing was carried out on a 130-year-old metallic riveted bridge, which was taken out of service, to estimate its modal properties under two different boundary conditions. A crane was used to lift the bridge, allowing it to move freely under the excitation load. An impulse hammer was used to excite the structure and a total of nine accelerometers were used to record the acceleration time histories. The response of the bridge, which is comprised of two main girders and seven cross girders, was estimated with a roving hammer technique, where one impulse hammer was used to excite the bridge at multiple locations, thus leading to the estimation of modal properties for the whole bridge, as well as individual girders. The set of modal properties obtained from free-free testing was compared with that estimated from identical testing of the bridge while resting on end supports. This comparison allows the quantification of the effect of the support conditions both on the estimates of the modal properties and on the associated noise levels. Ultimately, the results of this study are aimed at the refinement of bridge numerical (typically Finite Element based) models.
The major sources of error in the measurements of concrete structures are the gauge sensitivities, calibration accuracies, amplitude linearities, and temperature corrections to the gauge sensitivities, which are given in terms of plus-minus ranges, and the round off errors in the measured responses, which are better represented by interval bounds. An algorithm is proposed adapting the existing modified Metropolis Hastings algorithm for estimating the posterior probability of the damage indices as well as the posterior probability of the bounds of the interval parameters, when the measurements are given in terms of interval bounds. A damage index is defined for each element of the finite element model considering the parameters of each element are intervals. Methods are developed for evaluating response bounds in the finite element software ABAQUS, when the parameters of the finite element model are intervals. The proposed method is validated against reinforced concrete beams with three damage scenarios mainly (1) loss of stiffness, (2) loss of mass, and (3) loss of bond between concrete and reinforcement steel, which have been tested in our laboratory. Comparison of the prediction from the proposed method with those obtained from Bayesian analysis and interval optimization technique show improved accuracy and computational efficiency in addition to better representation of measurement uncertainties through interval bounds.
Failure of bolted connections in steel structures may result in catastrophic effects. Many algorithms in existing literature use modal information of a structure to identify damage in that structure, based on the data acquired from accelerometers which record the vibration time histories at different points on the structure. The location of these points may have significant effects on the quality of the acquired data, and thus the identified modal information. In this paper, a distance measure based Markov chain Monte Carlo algorithm is proposed to determine the optimal locations for the accelerometers, and the optimal location of the impact hammer if need. Different damage cases with various combinations of bolt failures are considered in this study. Failures at various levels are simulated by loosening the bolts in a predefined order. To compare the efficiency of the proposed method, the total effect of various damage cases on the accelerations at the optimal locations are calculated for the proposed method and a state-of-the-art method from the existing literature. The results demonstrate the efficiency of the proposed strategy in locating the accelerometers, which can produce data that are more sensitive to the bolted connection failures.
Offshore Wind Farms have established themselves as a matured technology to decarbonize energy sources to achieve net-zero. Offshore wind farms are currently being constructed in many seismic-prone zones and the codes/best practice guidelines are not fully developed. The aim of the paper (which is based on the keynote lecture presented at the 7th International Conference on Recent Advances in Geotechnical Earthquake Engineering) is as follows: (a) discuss the potential seismic risks to the offshore wind farm system and its components such as turbines, cables, and offshore substations; (b) present the different analysis and design methods for offshore wind turbine foundations; (c) highlight the performance-based design considerations and how to assess risks. Future research needs are highlighted.
The deep learning technologies have transformed many research areas with accuracy levels that the traditional methods are not comparable with. Recently, they have received increasing attention in the structural health monitoring (SHM) domain. In this paper, we aim to develop a new deep learning algorithm for structural condition monitoring and to evaluate its performance in a challenging case, bolt loosening damage in a frame structure. First, the design of a one-Dimensional Convolutional Neural Network (1DCNN) is introduced. Second, a series of impact hammer tests are conducted on a steel frame in the laboratory under ten scenarios, with bolts loosened at different locations and quantities. For each scenario, ten repeated tests are performed to provide enough training data for the algorithm. Third, the algorithm is trained with different quantities of training data (from one to seven test data for each scenario), and then is tested with the rest test data. The results show that the proposed 1D-CNN with three convolutional layers provide reliable identification results (over 95% accuracy) with sufficient training data sets. It has the potential to transform the SHM practice.