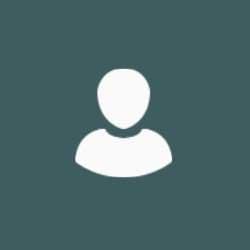
Dr Robert Pell
Publications
This article discusses how the gap between early 5G network threat assessments and an adversarial Tactics, Techniques, Procedures (TTPs) knowledge base for future use in the MITRE ATT&CK threat modelling framework can be bridged. We identify knowledge gaps in the existing framework for key 5G technology enablers such as SDN, NFV, and 5G specific signalling protocols of the core network. We adopt a pre-emptive approach to identifying adversarial techniques which can be used to launch attacks on the 5G core network (5GCN) and map these to its components. Using relevant 5G threat assessments along with industry reports, we study how the domain specific techniques can be employed by APTs in multi-stage attack scenarios based on historic telecommunication network attacks and motivation of APT groups. We emulate this mapping in a pre-emptive fashion to facilitate a rigorous cyber risk assessment, support intrusion detection, and design defences based on common APT TTPs in a 5GCN.
The fifth generation of mobile networks (5G) promises a range of new capabilities including higher data rates and more connected users. To support the new capabilities and use cases the 5G Core Network (5GCN) will be dynamic and reconfigurable in nature to deal with demand. It is these improvements which also introduce issues for traditional security monitoring methods and techniques which need to adapt to the new network architecture. The increased data volumes and dynamic network architecture mean an approach is required to focus security monitoring resources where it is most needed and react to network changes in real time. When considering multi-stage threat scenarios a coordinated, centralised approach to security monitoring is required for the early detection of attacks which may affect different parts of the network. In this chapter we identify potential solutions for overcoming these challenges which begins by identifying the threats to the 5G networks to determine suitable security monitoring placement in the 5GCN.
The threat landscape is evolving with tremendous speed. We are facing an extremely fast-growing attack surface with a diversity of attack vectors, a clear asymmetry between attackers and defenders, billions of connected IoT devices, mostly reactive detection and mitigation approaches, and finally big data challenges. The clear asymmetry of attacks and the enormous amount of data are additional arguments to make it necessary to rethink cybersecurity approaches in terms of reducing the attack surface, to make the attack surface dynamic, to automate the detection, risk assessment, and mitigation, and to investigate the prediction and prevention of attacks with the utilization of emerging technologies like blockchain, artificial intelligence and machine learning.This book contains eleven chapters dealing with different Cybersecurity Issues in Emerging Technologies. The issues that are discussed and analyzed include smart connected cars, unmanned ships, 5G/6G connectivity, blockchain, agile incident response, hardware assisted security, ransomware attacks, hybrid threats and cyber skills gap. Both theoretical analysis and experimental evaluation of state-of-the-art techniques are presented and discussed. Prospective readers can be benefitted in understanding the future implications of novel technologies and proposed security solutions and techniques. Graduate and postgraduate students, research scholars, academics, cybersecurity professionals, and business leaders will find this book useful, which is planned to enlighten both beginners and experienced readers.