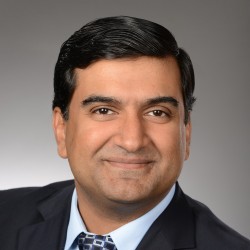
Dr Razi Arshad
Academic and research departments
Computer Science Research Centre, Surrey Centre for Cyber Security.About
Biography
Dr. Razi Arshad is a Research Fellow in Security and Privacy at the University of Surrey, Guilford, United Kingdom. Razi Arshad received his PhD degree in Mathematical Cryptography (with a focus on symmetric key cryptography) from Otto-von-Guericke University, Magdeburg, Germany in 2018. He obtained his MSc degree in Information Security from Sichuan University, Chengdu, China in 2007.
Prior to this position at Surrey, he was working as an Assistant professor at the School of Electrical Engineering and Computer Sciences, National University of Sciences and Technology, Islamabad, Pakistan.
Razi Arshad worked as a consultant in several tech companies that include Xprime Inc., CodeX Inc., SNSKIES Pvt. Ltd, and Graxo Consulting Pvt. Ltd. He is a certified ISO/IEC 17025 lead Assessor and ISO/IEC 27001 ISMS lead auditor.
ResearchResearch interests
His main research interests are applied cryptography, provable security, privacy-enhancing technologies, authentication schemes, secure communication, and post-quantum cryptography. Razi Arshad has published nine research articles in well-reputed venues that include journal articles, conference articles, and book chapters.
Research interests
His main research interests are applied cryptography, provable security, privacy-enhancing technologies, authentication schemes, secure communication, and post-quantum cryptography. Razi Arshad has published nine research articles in well-reputed venues that include journal articles, conference articles, and book chapters.
Publications
The Session Initiation Protocol (SIP) is the most widely used signaling protocol for controlling communication on the internet, establishing, maintaining, and terminating the sessions. The services that are enabled by SIP are equally applicable in the world of multimedia communication. Recently, Tsai proposed an efficient nonce-based authentication scheme for SIP. In this paper, we do a cryptanalysis of Tsai’s scheme and show that Tsai’s scheme is vulnerable to the password guessing attack and stolen-verifier attack. Furthermore, Tsai’s scheme does not provide known-key secrecy and perfect forward secrecy. We also propose a novel and secure mutual authentication scheme based on elliptic curve discrete logarithm problem for SIP which is immune to the presented attacks.
The aim of this comment paper is to identify a technical error in the published article of Nizam Chew et al.
Emotions are a crucial part of our daily lives, and they are defined as an organism’s complex reaction to significant objects or events, which include subjective and physiological components. Human emotion recognition has a variety of commercial applications, including intelligent automobile systems, affect-sensitive systems for customer service and contact centres, and the entertainment sector. In this work, we present a novel deep neural network of the Convolutional Neural Network - Bidirectional Gated Recurrent Unit (CNN-RNN) that can classify six basic emotions with an accuracy of above 95%. The deep model was trained on human gait data captured with body-mounted inertial sensors. We also proposed a reduction in the input space by utilizing 1D magnitudes of 3D accelerations and 3D angular velocities, which not only minimizes the computational complexity but also yields better classification accuracies. We compared the performance of the proposed model with existing methodologies and observed that the model outperforms the state-of-the-art.