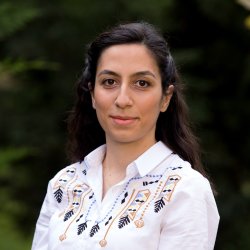
Dr Özge Mercanoğlu Sincan
About
Biography
Özge Mercanoğlu Sincan is a Research Fellow at the Centre for Vision, Speech and Signal Processing (CVSSP) at the University of Surrey. She is currently working with Prof. Richard Bowden on sign language recognition and translation. She received her Ph.D. degree in computer engineering from Ankara University, Turkey, in 2021 under the supervision of Assoc. Prof. Hacer Yalim Keles. Her main research interests include computer vision and deep learning.
My qualifications
Previous roles
Publications
Capturing and annotating Sign language datasets is a time consuming and costly process. Current datasets are orders of magnitude too small to successfully train unconstrained \acf{slt} models. As a result, research has turned to TV broadcast content as a source of large-scale training data, consisting of both the sign language interpreter and the associated audio subtitle. However, lack of sign language annotation limits the usability of this data and has led to the development of automatic annotation techniques such as sign spotting. These spottings are aligned to the video rather than the subtitle, which often results in a misalignment between the subtitle and spotted signs. In this paper we propose a method for aligning spottings with their corresponding subtitles using large spoken language models. Using a single modality means our method is computationally inexpensive and can be utilized in conjunction with existing alignment techniques. We quantitatively demonstrate the effectiveness of our method on the \acf{mdgs} and \acf{bobsl} datasets, recovering up to a 33.22 BLEU-1 score in word alignment.
Sign Language Translation (SLT) is a challenging task that aims to generate spoken language sentences from sign language videos, both of which have different grammar and word/gloss order. From a Neural Machine Translation (NMT) perspective, the straightforward way of training translation models is to use sign language phrase-spoken language sentence pairs. However, human interpreters heavily rely on the context to understand the conveyed information, especially for sign language interpretation, where the vocabulary size may be significantly smaller than their spoken language equivalent. Taking direct inspiration from how humans translate, we propose a novel multi-modal transformer architecture that tackles the translation task in a context-aware manner, as a human would. We use the context from previous sequences and confident predictions to disambiguate weaker visual cues. To achieve this we use complementary transformer encoders, namely: (1) A Video Encoder, that captures the low-level video features at the frame-level, (2) A Spotting Encoder, that models the recognized sign glosses in the video, and (3) A Context Encoder, which captures the context of the preceding sign sequences. We combine the information coming from these encoders in a final transformer decoder to generate spoken language translations. We evaluate our approach on the recently published large-scale BOBSL dataset, which contains ~1.2M sequences, and on the SRF dataset, which was part of the WMT-SLT 2022 challenge. We report significant improvements on state-of-the-art translation performance using contextual information, nearly doubling the reported BLEU-4 scores of baseline approaches.
Sign Language Translation (SLT) is a challenging task that aims to generate spoken language sentences from sign language videos, both of which have different grammar and word/gloss order. From a Neural Machine Translation (NMT) perspective, the straightforward way of training translation models is to use sign language phrase-spoken language sentence pairs. However, human interpreters heavily rely on the context to understand the conveyed information , especially for sign language interpretation, where the vocabulary size may be significantly smaller than their spoken language equivalent. Taking direct inspiration from how humans translate, we propose a novel multi-modal transformer architecture that tackles the translation task in a context-aware manner, as a human would. We use the context from previous sequences and confident predictions to disambiguate weaker visual cues. To achieve this we use complementary transformer encoders, namely: (1) A Video Encoder, that captures the low-level video features at the frame-level, (2) A Spotting Encoder, that models the recognized sign glosses in the video, and (3) A Context Encoder, which captures the context of the preceding sign sequences. We combine the information coming from these encoders in a final transformer decoder to generate spoken language translations. We evaluate our approach on the recently published large-scale BOBSL dataset, which contains ∼1.2M sequences , and on the SRF dataset, which was part of the WMT-SLT 2022 challenge. We report significant improvements on state-of-the-art translation performance using contextual information, nearly doubling the reported BLEU-4 scores of baseline approaches.
Sign language recognition is a challenging problem where signs are identified by simultaneous local and global articulations of multiple sources, i.e. hand shape and orientation, hand movements, body posture, and facial expressions. Solving this problem computationally for a large vocabulary of signs in real life settings is still a challenge, even with the state-of-the-art models. In this study, we present a new large-scale multi-modal Turkish Sign Language dataset (AUTSL) with a benchmark and provide baseline models for performance evaluations. Our dataset consists of 226 signs performed by 43 different signers and 38,336 isolated sign video samples in total. Samples contain a wide variety of backgrounds recorded in indoor and outdoor environments. Moreover, spatial positions and the postures of signers also vary in the recordings. Each sample is recorded with Microsoft Kinect v2 and contains color image (RGB), depth, and skeleton modalities. We prepared benchmark training and test sets for user independent assessments of the models. We trained several deep learning based models and provide empirical evaluations using the benchmark; we used Convolutional Neural Networks (CNNs) to extract features, unidirectional and bidirectional Long Short-Term Memory (LSTM) models to characterize temporal information. We also incorporated feature pooling modules and temporal attention to our models to improve the performances. We evaluated our baseline models on AUTSL and Montalbano datasets. Our models achieved competitive results with the state-of-the-art methods on Montalbano dataset, i.e. 96.11% accuracy. In AUTSL random train-test splits, our models performed up to 95.95% accuracy. In the proposed user-independent benchmark dataset our best baseline model achieved 62.02% accuracy. The gaps in the performances of the same baseline models show the challenges inherent in our benchmark dataset. AUTSL benchmark dataset is publicly available at https://cvml.ankara.edu.tr .
Capturing and annotating Sign language datasets is a time consuming and costly process. Current datasets are orders of magnitude too small to successfully train unconstrained Sign Language Translation (SLT) models. As a result, research has turned to TV broadcast content as a source of large-scale training data, consisting of both the sign language interpreter and the associated audio subtitle. However, lack of sign language annotation limits the usability of this data and has led to the development of automatic annotation techniques such as sign spotting. These spottings are aligned to the video rather than the subtitle, which often results in a misalignment between the subtitle and spotted signs. In this paper we propose a method for aligning spottings with their corresponding subtitles using large spoken language models. Using a single modality means our method is computationally inexpensive and can be utilized in conjunction with existing alignment techniques. We quantitatively demonstrate the effectiveness of our method on the Meine DGS-Annotated (MeineDGS) and BBC-Oxford British Sign Language (BOBSL) datasets, recovering up to a 33.22 BLEU-1 score in word alignment.
Sign language recognition using computational models is a challenging problem that requires simultaneous spatio-temporal modeling of the multiple sources, i.e. faces, hands, body, etc. In this paper, we propose an isolated sign language recognition model based on a model trained using Motion History Images (MHI) that are generated from RGB video frames. RGB-MHI images represent spatio-temporal summary of each sign video effectively in a single RGB image. We propose two different approaches using this RGB-MHI model. In the first approach, we use the RGB-MHI model as a motion-based spatial attention module integrated into a 3D-CNN architecture. In the second approach, we use RGB-MHI model features directly with the features of a 3D-CNN model using a late fusion technique. We perform extensive experiments on two recently released large-scale isolated sign language datasets, namely AUTSL and BosphorusSign22k. Our experiments show that our models, which use only RGB data, can compete with the state-of-the-art models in the literature that use multi-modal data.
The performances of Sign Language Recognition (SLR) systems have improved considerably in recent years. However, several open challenges still need to be solved to allow SLR to be useful in practice. The research in the field is in its infancy in regards to the robustness of the models to a large diversity of signs and signers, and to fairness of the models to performers from different demographics. This work summarises the ChaLearn LAP Large Scale Signer Independent Isolated SLR Challenge, organised at CVPR 2021 with the goal of overcoming some of the aforementioned challenges. We analyse and discuss the challenge design, top winning solutions and suggestions for future research. The challenge attracted 132 participants in the RGB track and 59 in the RGB+Depth track, receiving more than 1.5K submissions in total. Participants were evaluated using a new large-scale multi-modal Turkish Sign Language (AUTSL) dataset, consisting of 226 sign labels and 36,302 isolated sign video samples performed by 43 different signers. Winning teams achieved more than 96% recognition rate, and their approaches benefited from pose/hand/face estimation, transfer learning, external data, fusion/ensemble of modalities and different strategies to model spatio-temporal information. However, methods still fail to distinguish among very similar signs, in particular those sharing similar hand trajectories.