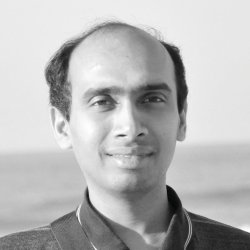
Professor Nishanth Sastry
Academic and research departments
Distributed and Networked Systems Group, Surrey Centre for Cyber Security, Computer Science Research Centre, School of Computer Science and Electronic Engineering.About
Biography
Prof. Nishanth Sastry is the Director of Research of the Department of Computer Science, University of Surrey. His research spans a number of topics relating to social media, content delivery and networking, and online safety and privacy. He is joint Head of the Distributed and Networked Systems Group and co-leads the Pan University Surrey Security Network. He is also a Surrey AI Fellow and a Visiting Researcher at the Alan Turing Institute, where he is a co-lead of the Social Data Science Special Interest Group.
Prof. Sastry holds a Bachelor’s degree (with distinction) from R.V. College of Engineering, Bangalore University, a Master’s degree from University of Texas, Austin, and a PhD from the University of Cambridge, all in Computer Science. Previously, he spent over six years in the Industry (Cisco Systems, India and IBM Software Group, USA) and Industrial Research Labs (IBM TJ Watson Research Center). He has also spent time at the Massachusetts Institute of Technology Computer Science and AI Laboratory.
His honours include a Best Paper Award at SIGCOMM Mobile Edge Computing in 2017, a Best Paper Honorable Mention at WWW 2018, a Best Student Paper Award at the Computer Society of India Annual Convention, a Yunus Innovation Challenge Award at the Massachusetts Institute of Technology IDEAS Competition, a Benefactor’s Scholarship from St. John’s College, Cambridge, a Best Undergraduate Project Award from RV College of Engineering, a Cisco Achievement Program Award and several awards from IBM. He has been granted nine patents in the USA for work done at IBM.
Nishanth has been a keynote speaker, and received media coverage from print media such as The Times UK, New York Times, New Scientist and Nature, as well as Television media such as BBC, Al Jazeera and Sky News. He is a member of the ACM and a Senior Member of the IEEE.
Areas of specialism
News
In the media
ResearchResearch interests
My work focuses on the structures and architectures used for disseminating and consuming content online. This encompasses a range of topics both at the infrastructure (computer networks) and people (social networks) levels. My long-standing approach has been to apply the lens of data analysis to large, real-world data sets of social networks and computer networks, and using the patterns gleaned therein to design and develop better systems and architectures that are "fit-for-purpose" in deployed or deployable systems. This highly empirical approach, which may be termed "Internet Data Science", has allowed me to work with partners such as BBC R&D, Vodafone R&D and Cisco Systems, as well as drive social and public policy impact, with public bodies and organisations such as the UK Parliament, Wikimedia Foundation and the Samaritans:
Peer-Assisted Content Delivery and Edge Networking Architectures
- Using anonymised data from the equivalent of half the UK population accessing BBC iPlayer, a series of papers showed how the load on UK's networks can be decreased by more than half, and how content delivery can be made greener and more sustainable. We also proposed architectures and theoretical evaluations for peer-assisted offloads and cache-aided D2D, based on individual preferences.
- Using ~3TB of data from Facebook Live, we identified a number of opportunities for edge caching and city-level data centres to decrease and cellular data usage for users.
Computational Politics, Partisan News and Filter Bubbles
- We have worked with Facebook, and scholar/activists to quantify biased representations in news and social media during the Russia/Ukraine conflict.
- We contributed to a massive exposé by Buzzfeed News on the effect of hyper-partisan news and filter bubbles in the 2016 US Presidential elections.
- Working with the UK House of Commons Library and the Wikimedia Foundation, we have identified patterns of digital citizen engagement on Twitter and Wikipedia, with an "anti filter-bubble" of significant cross-party conversations between Members of Parliament and citizens.
Web tracking and Privacy
- We have deployed a Chrome extension to understand web tracking which is being used by several thousand users across the globe. Using this, we have launched possibly the largest in-the-wild multi-country study of trackers, developed the 'tangle factor' metric to quantify and compare different efforts (e.g., ad blockers) to improve privacy, and to understand how much GDPR-enforced cookie consents have changed the numbers of third party cookies for real users (Answer: not much!)
- With Telefonica Research, we have developed techniques to understand differential tracking of different demographics, showing that right-leaning partisan websites in the USA track more than left-leaning websites
Harnessing Crowdwork and Cross-Social Network Phenomena
- We have identified several phenomena relating to how users manage their different identities across social platforms. Social bootstrapping showed how social logins help grow nascent social networks such as Pinterest. With Max-Planck Institute, we looked at how to transfer trust across platforms. With Penn State, we identified patterns in how users adapt their profiles to different platforms based on whether they capture formal settings (e.g., LinkedIn) or informal friendships (e.g., Facebook, Instagram).
- We have also developed methods to harness the work of crowds. Predicting Pinterest showed how individual activities on social networks can be treated as a distributed human computation and automated based on crowd work. FaceLift, a collaboration with Bell Labs Cambridge, developed mechanisms to propagate binary rankings of beauty over pairs of images to a whole corpus, and developed a Generative Adversarial Network (GAN) to beautify urban scenes.
In all the above, "we" refers to me and the group of talented hardworking students and postdocs with whom I collaborate. If this sort of research sounds interesting, and you would like to work with me, feel free to send me an email, mentioning "life=42" to indicate you have read through this text.
Research interests
My work focuses on the structures and architectures used for disseminating and consuming content online. This encompasses a range of topics both at the infrastructure (computer networks) and people (social networks) levels. My long-standing approach has been to apply the lens of data analysis to large, real-world data sets of social networks and computer networks, and using the patterns gleaned therein to design and develop better systems and architectures that are "fit-for-purpose" in deployed or deployable systems. This highly empirical approach, which may be termed "Internet Data Science", has allowed me to work with partners such as BBC R&D, Vodafone R&D and Cisco Systems, as well as drive social and public policy impact, with public bodies and organisations such as the UK Parliament, Wikimedia Foundation and the Samaritans:
Peer-Assisted Content Delivery and Edge Networking Architectures
- Using anonymised data from the equivalent of half the UK population accessing BBC iPlayer, a series of papers showed how the load on UK's networks can be decreased by more than half, and how content delivery can be made greener and more sustainable. We also proposed architectures and theoretical evaluations for peer-assisted offloads and cache-aided D2D, based on individual preferences.
- Using ~3TB of data from Facebook Live, we identified a number of opportunities for edge caching and city-level data centres to decrease and cellular data usage for users.
Computational Politics, Partisan News and Filter Bubbles
- We have worked with Facebook, and scholar/activists to quantify biased representations in news and social media during the Russia/Ukraine conflict.
- We contributed to a massive exposé by Buzzfeed News on the effect of hyper-partisan news and filter bubbles in the 2016 US Presidential elections.
- Working with the UK House of Commons Library and the Wikimedia Foundation, we have identified patterns of digital citizen engagement on Twitter and Wikipedia, with an "anti filter-bubble" of significant cross-party conversations between Members of Parliament and citizens.
Web tracking and Privacy
- We have deployed a Chrome extension to understand web tracking which is being used by several thousand users across the globe. Using this, we have launched possibly the largest in-the-wild multi-country study of trackers, developed the 'tangle factor' metric to quantify and compare different efforts (e.g., ad blockers) to improve privacy, and to understand how much GDPR-enforced cookie consents have changed the numbers of third party cookies for real users (Answer: not much!)
- With Telefonica Research, we have developed techniques to understand differential tracking of different demographics, showing that right-leaning partisan websites in the USA track more than left-leaning websites
Harnessing Crowdwork and Cross-Social Network Phenomena
- We have identified several phenomena relating to how users manage their different identities across social platforms. Social bootstrapping showed how social logins help grow nascent social networks such as Pinterest. With Max-Planck Institute, we looked at how to transfer trust across platforms. With Penn State, we identified patterns in how users adapt their profiles to different platforms based on whether they capture formal settings (e.g., LinkedIn) or informal friendships (e.g., Facebook, Instagram).
- We have also developed methods to harness the work of crowds. Predicting Pinterest showed how individual activities on social networks can be treated as a distributed human computation and automated based on crowd work. FaceLift, a collaboration with Bell Labs Cambridge, developed mechanisms to propagate binary rankings of beauty over pairs of images to a whole corpus, and developed a Generative Adversarial Network (GAN) to beautify urban scenes.
In all the above, "we" refers to me and the group of talented hardworking students and postdocs with whom I collaborate. If this sort of research sounds interesting, and you would like to work with me, feel free to send me an email, mentioning "life=42" to indicate you have read through this text.
Supervision
Postgraduate research supervision
I am looking for PhD students. Please read my research and my publications, and if the topics sound like something you might want to work on for 3+ years, please get in touch!
Publications
In the evolving landscape of satellite communications, the deployment of Low-Earth Orbit (LEO) satellite constellations promises to revolutionize global Internet access by providing low-latency, high-bandwidth connectivity to underserved regions. However, the dynamic nature of LEO satellite networks, characterized by rapid orbital movement and frequent changes in Inter-Satellite Links (ISLs), challenges the suitability of existing Internet protocols designed for static terrestrial infrastructures. Testing and developing new solutions and protocols on actual satellite mega-constellations are either too expensive or impractical because some of these constellations are not fully deployed yet. This creates the need for a realistic simulation platform that can accurately simulate this large scale of satellites, and allow end-to-end control over all aspects of LEO constellations. This paper introduces xeoverse, a scalable and realistic network simulator designed to support comprehensive LEO satellite network research and experimentation. By modeling user terminals, satellites, and ground stations as lightweight Linux virtual machines within Mininet and implementing three key strategies - pre-computing topology and routing changes, updating only changing ISL links, and focusing on ISL links relevant to the simulation scenario - xeoverse achieves real- time simulation, where 1 simulated second equals 1 wall-clock second. Our evaluations show that xeoverse outperforms state- of-the-art simulators Hypatia and StarryNet in terms of total simulation time by being 2.9 and 40 times faster, respectively.
Recent studies have outlined the accessibility challenges faced by blind or visually impaired, and less-literate people, in interacting with social networks, in-spite of facilitating technologies such as monotone text-to-speech (TTS) screen readers and audio narration of visual elements such as emojis. Emotional speech generation traditionally relies on human input of the expected emotion together with the text to syn-thesise, with additional challenges around data simplification (causing information loss) and duration inaccuracy, leading to lack of expressive emotional rendering. In real-life communications, the duration of phonemes can vary since the same sentence might be spoken in a variety of ways depending on the speakers' emotional states or accents (referred to as the one-to-many problem of text to speech generation). As a result, an advanced voice synthesis system is required to account for this un-predictability. We propose an end-to-end context-aware Text-to-Speech (TTS) synthesis system that derives the conveyed emotion from text input and synthesises audio that focuses on emotions and speaker features for natural and expressive speech, integrating advanced natural language processing (NLP) and speech synthesis techniques for real-time applications. Our system also showcases competitive inference time performance when benchmarked against the state-of-the-art TTS models, making it suitable for real-time accessibility applications.
Recent studies have outlined the accessibility challenges faced by blind or visually impaired, and less-literate people, in interacting with social networks, in-spite of facilitating technologies such as monotone text-to-speech (TTS) screen readers and audio narration of visual elements such as emojis. Emotional speech generation traditionally relies on human input of the expected emotion together with the text to synthesise, with additional challenges around data simplification (causing information loss) and duration inaccuracy, leading to lack of expressive emotional rendering. In real-life communications, the duration of phonemes can vary since the same sentence might be spoken in a variety of ways depending on the speakers' emotional states or accents (referred to as the one-to-many problem of text to speech generation). As a result, an advanced voice synthesis system is required to account for this unpredictability. We propose an end-to-end context-aware Text-to-Speech (TTS) synthesis system that derives the conveyed emotion from text input and synthesises audio that focuses on emotions and speaker features for natural and expressive speech, integrating advanced natural language processing (NLP) and speech synthesis techniques for real-time applications. Our system also showcases competitive inference time performance when benchmarked against the state-of-the-art TTS models, making it suitable for real-time accessibility applications.
Content Delivery Networks (CDNs) have been pivotal in the dramatic evolution of the Internet, handling the majority of data traffic for billions of connected users. Low-Earth-Orbit (LEO) satellite networks, such as Starlink, aim to revolutionize global connectivity by providing high-speed, low-latency Internet to remote regions. However, LEO satellite networks (LSNs) face challenges integrating with traditional CDNs, which rely on geographical proximity for efficient content delivery - a method that clashes with the operational dynamics of LSNs. In this paper, we scrutinize the operation of CDNs in the context of LSNs, using Starlink as a case study. We develop a browser extension NetMet that performs extensive web browsing experiments from controlled nodes using both Starlink and terrestrial Internet access. Additionally, we analyse crowdsourced speed tests from Starlink users to Cloudflare CDN servers globally. Our results indicate significant performance issues for Starlink users, stemming from the misalignment between terrestrial and satellite infrastructures. We then investigate the potential for SpaceCDNs which integrate CDN infrastructure directly within the LSNs, and show that this approach offers a promising alternative that decreases latencies by over 50%, making them comparable with the CDN experience of users behind terrestrial ISPs. Our aim is to stimulate further research and discussion on overcoming the challenges of effective content delivery with growing LSN offerings.
This paper explores the optimisation of gateway (GW) placements within Low Earth Orbit (LEO) satellite networks, focusing on enhancing network performance metrics such as latency and hop count. Utilising a modified variant of the K-means algorithm termed Geo K-means, we analysed the current Starlink GW distribution and proposed a strategic placement model that aligns GW locations with user density. Results demonstrate that strategic GW placement, informed by user population density and utilising a hybrid architecture of bent-pipe and inter-satellite links (ISLs), significantly improves network performance, decreasing latency by 86% on average for user terminals across the globe, even when using only half the number of gateways deployed by Starlink. Our optimised GW placements connect more user terminals through direct bent-pipe connections, requiring less reliance on ISLs. ISLs increase the reach of Starlink gateways but at the expense of higher hop counts and can therefore increase latency by up to 5x compared to a bent-pipe connection to a nearby GW, highlighting the importance of strategic GW placement in LEO satellite megaconstellations, especially when relying on ISLs.
Social media is recognized as an important source for deriving insights into public opinion dynamics and social impacts due to the vast textual data generated daily and the 'un-constrained' behavior of people interacting on these platforms. However, such analyses prove challenging due to the semantic shift phenomenon , where word meanings evolve over time. This paper proposes an unsupervised dynamic word embedding method to capture longitudinal semantic shifts in social media data without predefined anchor words. The method leverages word co-occurrence statistics and dynamic updating to adapt embed-dings over time, addressing the challenges of data sparseness, imbalanced distributions, and synergistic semantic effects. Evaluated on a large COVID-19 Twitter dataset, the method reveals semantic evolution patterns of vaccine-and symptom-related entities across different pandemic stages, and their potential correlations with real-world statistics. Our key contributions include the dynamic embedding technique , empirical analysis of COVID-19 semantic shifts, and discussions on enhancing semantic shift modeling for computational social science research. This study enables capturing longitudinal semantic dynamics on social media to understand public discourse and collective phenomena.
India is experiencing intense political partisanship and sectarian divisions. The paper performs, to the best of our knowledge, the first comprehensive analysis on the Indian online news media with respect to tracking and partisanship. We build a dataset of 103 online, mostly mainstream news websites. With the help of two experts, alongside data from the Media Ownership Monitor, we label these websites according to their partisanship (Left, Right, or Centre). We study and compare user tracking on these sites with different metrics: numbers of cookies, cookie synchronization, device fingerprinting, and invisible pixel-based tracking. We find that Left and Centre websites serve more cookies than Right-leaning websites. However, through cookie synchronization, more user IDs are synchronized in Left websites than Right or Centre. Canvas fingerprinting is used similarly by Left and Right, and less by Centre. Invisible pixel-based tracking is 50% more intense in Centre-leaning websites than Right, and 25% more than Left. Desktop versions of news websites deliver more cookies than their mobile counterparts. A handful of third-parties are tracking users in most websites in this study. This paper demonstrates the intensity of Web tracking happening in Indian news websites and discusses implications for research on overall privacy of users visiting partisan news websites in India.
Decentralising the Web is a desirable but challenging goal. One particular challenge is achieving decentralised content moderation in the face of various adversaries (e.g. trolls). To overcome this challenge, many Decentralised Web (DW) im- plementations rely on federation policies. Administrators use these policies to create rules that ban or modify content that matches specific rules. This, however, can have unintended consequences for many users. In this paper, we present the first study of federation policies on the DW, their in-the-wild usage, and their impact on users. We identify how these poli- cies may negatively impact "innocent" users and outline pos- sible solutions to avoid this problem in the future.
Accurately assigning standardized diagnosis and procedure codes from clinical text is crucial for healthcare applications. However, this remains challenging due to the complexity of medical language. This paper proposes a novel model that incorporates extreme multi-label classification tasks to enhance International Classification of Diseases (ICD) coding. The model utilizes deformable convolutional neural networks to fuse representations from hidden layer outputs of pre-trained language models and external medical knowledge embeddings fused using a multimodal approach to provide rich semantic encodings for each code. A probabilistic label tree is constructed based on the hierarchical structure existing in ICD labels to incorporate ontological relationships between ICD codes and enable structured output prediction. Experiments on medical code prediction on the MIMIC-III database demonstrate competitive performance, highlighting the benefits of this technique for robust clinical code assignment.
In response to the exponential surge in Internet Video on Demand (VOD) traffic, numerous research endeavors have concentrated on optimizing and enhancing infrastructure efficiency. In contrast, this paper explores whether users' demand patterns can be shaped to reduce the pressure on infrastructure. Our main idea is to design a mechanism that alters the distribution of user requests to another distribution which is much more cache-efficient, but still remains 'close enough' (in the sense of cost) to fulfil each individual user's preference. To quantify the cache footprint of VOD traffic, we propose a novel application of Rényi entropy as its proxy, capturing the 'richness' (the number of distinct videos or cache size) and the 'evenness' (the relative popularity of video accesses) of the on-demand video distribution. We then demonstrate how to decrease this metric by formulating a problem drawing on the mathematical theory of optimal transport (OT). Additionally, we establish a key equivalence theorem: minimizing Rényi entropy corresponds to maximizing soft cache hit ratio (SCHR) --- a variant of cache hit ratio allowing similarity-based video substitutions. Evaluation on a real-world, city-scale video viewing dataset reveals a remarkable 83% reduction in cache size (associated with VOD caching traffic). Crucially, in alignment with the above-mentioned equivalence theorem, our approach yields a significant uplift to SCHR, achieving close to 100%.
Computational analyses driven by Artificial Intelligence (AI)/Machine Learning (ML) methods to generate patterns and inferences from big datasets in computational social science (CSS) studies can suffer from biases during the data construction, collection and analysis phases as well as encounter challenges of generalizability and ethics. Given the interdisciplinary nature of CSS, many factors such as the need for a comprehensive understanding of different facets such as the policy and rights landscape, the fast-evolving AI/ML paradigms and dataset-specific pitfalls influence the possibility of biases being introduced. This chapter identifies challenges faced by researchers in the CSS field and presents a taxonomy of biases that may arise in AI/ML approaches. The taxonomy mirrors the various stages of common AI/ML pipelines: dataset construction and collection, data analysis and evaluation. By detecting and mitigating bias in AI, an active area of research, this chapter seeks to highlight practices for incorporating responsible research and innovation into CSS practices.
In response to the exponential surge in Video on Demand (VOD) traffic, numerous research endeavors have concentrated on optimizing and enhancing infrastructure efficiency. In contrast, this paper explores whether users' demand patterns can be shaped to reduce the pressure on infrastructure. Our main idea is to design a mechanism that alters the distribution of user requests to another distribution which is much more cache-efficient, but still remains 'close enough' (in terms of cost) to fulfil individual user's preference.
This paper is concerned with helping people who are vulnerable during important transitions in life, such as 'coming out' as LGBTQIA+, experiencing serious illness, undergoing relationship breakdown etc. Rich sensor streams derived from so-called 'smart' Internet of Things (IoT) devices can be highly beneficial, for example in ensuring the safety of such individuals during their sensitive life transitions, or in providing functionality that can mitigate some of the difficulties faced by them. However, the data that needs to be extracted to provide these benefits can itself be highly sensitive and needs to be processed with safeguards to protect privacy. We develop scenarios that highlight issues arising from having to merge data streams from multiple devices, including data governance issues that are relevant when the sensors are owned by multiple individuals. We propose a "Transition Guardian" architecture that leverages "Smart Experts" written as smart contracts operating on homomorphically encrypted sensor data streams to provide real-time protection without disclosing their sensitive information. We have also implemented a proof-of-concept on the Ethereum protocol to validate our proposed solution.
The rapid growth of satellite network operators (SNOs) has revolutionized broadband communications, enabling global connectivity and bridging the digital divide. As these networks expand, it is important to evaluate their performance and efficiency. This paper presents the first comprehensive study of SNOs. We take an opportunistic approach and devise a methodology which allows to identify public network measurements performed via SNOs. We apply this methodology to both M-Lab and RIPE public datasets which allowed us to characterize low level performance and footprint of up to 18 SNOs operating in different orbits. Finally, we identify and recruit paid testers on three popular SNOs (Starlink, HughesNet, and ViaSat) to evaluate the performance of popular applications like web browsing and video streaming.
Low Power Wide Area Networks (LPWANs) are a subset of IoT transmission technologies that have gained traction in recent years with the number of such devices exceeding 200 million. This paper considers the scalability of one such LPWAN, LoRaWAN, as the number of devices in a network increases. Various existing optimisation techniques target LoRa characteristics such as collision rate, fairness, and power consumption. This paper proposes a machine learning ensemble to reduce the total distance between devices and improve the average received signal strength, resulting in improved network throughput, the scalability of LoRaWAN, and the cost of networks. The ensemble consists of a constrained K-Means clustering algorithm, a regression model to validate new gateway locations and a Neural network to estimate signal strength based on the location of the devices. Results show a mean distance reduction of 51% with an RSSI improvement of 3% when maintaining the number of gateways, also achieving a distance reduction of 27% and predicting an RSSI increase of 1% after clustering with 50% of the number of gateways.
With the advent of 5G+ services, it has become increasingly convenient for mobile users to enjoy high-quality multimedia content from CDN driven streaming and catch-up TV services (Netflix, iPlayer) in the (post-) COVID over-the-top (OTT) content rush. To relieve ISP owned fixed-line networks from CDN streamed multimedia traffic, system ideas (e.g., Wi-Stitch in [45]) have been proposed to (a) leverage 5G services and enable consumers to share cached multimedia content at the edge, and (b) consequently, and more importantly, reduce IP traffic at the core network. Unfortunately, given that contemporary multimedia content might be a monetized asset, these ideas do not take this important fact into account for shared content. We present EdgeMart—a content provider (CP) federated, and computationally sustainable networked (graphical) market economy for paid-sharing of cached licensed (OTT) content with autonomous users of a wireless edge network (WEN). EdgeMart is a unique oligopoly multimedia market (economy) that comprises competing networked sub-markets of non-cooperative content sellers/buyers—each sub-market consisting of a single buyer connected (networked) to only a subset of sellers. We prove that for any WEN-supported supply-demand topology, a pure strategy EdgeMart equilibrium exists that is (a) nearly efficient (in a microeconomic sense) indicating economy sustainability, (b) robust to edge user entry/exit, and (c) can be reached in poly-time (indicating computational sustainability). In addition, we experimentally show that for physical WENs of varying densities, a rationally selfish EdgeMart economy induces similar orders of multimedia IP traffic savings when compared to the ideal (relatively less practical), altruistic, and non-monetized “economy” implemented atop the recently introduced Wi-Stitch WEN-based content trading architecture. Moreover, the EdgeMart concept helps envision a regulated edge economy of opportunistic (pay per licensed file) client services for commercial OTT platforms.
The acquisition of Twitter by Elon Musk has spurred controversy and uncertainty among Twitter users. The move raised both praise and concerns, particularly regarding Musk's views on free speech. As a result, a large number of Twitter users have looked for alternatives to Twitter. Mastodon, a decentralized micro-blogging social network, has attracted the attention of many users and the general media. In this paper, we analyze the migration of 136,009 users from Twitter to Mastodon. We inspect the impact that this has on the wider Mastodon ecosystem, particularly in terms of user-driven pressure towards centralization. We further explore factors that influence users to migrate, highlighting the effect of users' social networks. Finally, we inspect the behavior of individual users, showing how they utilize both Twitter and Mastodon in parallel. We find a clear difference in the topics discussed on the two platforms. This leads us to build classifiers to explore if migration is predictable. Through feature analysis, we find that the content of tweets as well as the number of URLs, the number of likes, and the length of tweets are effective metrics for the prediction of user migration.
Traditional, slow and error-prone human-driven methods to configure and manage Internet service requests are proving unsatisfactory. This is due to an increase in Internet applications with stringent quality of service (QoS) requirements. Which demands faster and fault-free service deployment with minimal or without human intervention. With this aim, intent-driven service management (IDSM) has emerged, where users express their service level agreement (SLA) requirements in a declarative manner as intents . With the help of closed control-loop operations, IDSM performs service configurations and deployments, autonomously to fulfill the intents. This results in a faster deployment of services and reduction in configuration errors caused by manual operations, which in turn reduces the SLA violations. This article is an attempt to provide a systematic review of How the IDSM systems manage and fulfill the SLA requirements specified as intents. As an outcome, the review identifies four intent management activities, which are performed in a closed-loop manner. For each activity, a taxonomy is proposed and used to compare the existing techniques for SLA management in IDSM systems. A critical analysis of all the considered research articles in the review and future research directions are presented in the conclusion.
The introduction of ChatGPT and the subsequent improvement of Large Language Models (LLMs) have prompted more and more individuals to turn to the use of ChatBots, both for information and assistance with decision-making. However, the information the user is after is often not formulated by these ChatBots objectively enough to be provided with a definite, globally accepted answer. Controversial topics, such as "religion", "gender identity", "freedom of speech", and "equality", among others, can be a source of conflict as partisan or biased answers can reinforce preconceived notions or promote disinformation. By exposing ChatGPT to such debatable questions, we aim to understand its level of awareness and if existing models are subject to socio-political and/or economic biases. We also aim to explore how AI-generated answers compare to human ones. For exploring this, we use a dataset of a social media platform created for the purpose of debating human-generated claims on polemic subjects among users, dubbed Kialo. Our results show that while previous versions of ChatGPT have had important issues with controversial topics, more recent versions of ChatGPT (gpt-3.5-turbo) are no longer manifesting significant explicit biases in several knowledge areas. In particular, it is well-moderated regarding economic aspects. However, it still maintains degrees of implicit libertarian leaning toward right-winged ideals which suggest the need for increased moderation from the socio-political point of view. In terms of domain knowledge on controversial topics, with the exception of the "Philosophical" category, ChatGPT is performing well in keeping up with the collective human level of knowledge. Finally, we see that sources of Bing AI have slightly more tendency to the center when compared to human answers. All the analyses we make are generalizable to other types of biases and domains.
India is experiencing intense political partisanship and sectarian divisions. The paper performs, to the best of our knowledge, the first comprehensive analysis on the Indian online news media with respect to tracking and partisanship. We build a dataset of 103 online, mostly mainstream news websites. With the help of two experts, alongside data from the Media Ownership Monitor, we label these websites according to their partisanship (Left, Right, or Centre). We study and compare user tracking on these sites with different metrics: numbers of cookies, cookie synchronization, device fingerprinting, and invisible pixel-based tracking. We find that Left and Centre websites serve more cookies than Right-leaning websites. However, through cookie synchronization, more user IDs are synchronized in Left websites than Right or Centre. Canvas fingerprinting is used similarly by Left and Right, and less by Centre. Invisible pixel-based tracking is 50% more intense in Centre-leaning websites than Right, and 25% more than Left. Desktop versions of news websites deliver more cookies than their mobile counterparts. A handful of third-parties are tracking users in most websites in this study. This paper demonstrates the intensity of Web tracking happening in Indian news websites and discusses implications for research on overall privacy of users visiting partisan news websites in India.
Supervised approaches generally rely on majority-based labels. However, it is hard to achieve high agreement among annotators in subjective tasks such as hate speech detection. Existing neural network models principally regard labels as categorical variables, while ignoring the semantic information in diverse label texts. In this paper, we propose AnnoBERT, a first-of-its-kind architecture integrating annotator characteristics and label text with a transformer-based model to detect hate speech, with unique representations based on each annotator's characteristics via Collaborative Topic Regression (CTR) and integrate label text to enrich textual representations. During training, the model associates annotators with their label choices given a piece of text; during evaluation, when label information is not available, the model predicts the aggregated label given by the participating annotators by utilising the learnt association. The proposed approach displayed an advantage in detecting hate speech, especially in the minority class and edge cases with annotator disagreement. Improvement in the overall performance is the largest when the dataset is more label-imbalanced, suggesting its practical value in identifying real-world hate speech, as the volume of hate speech in-the-wild is extremely small on social media, when compared with normal (non-hate) speech. Through ablation studies, we show the relative contributions of annotator embeddings and label text to the model performance, and tested a range of alternative annotator embeddings and label text combinations.
Supervised machine learning approaches often rely on a "ground truth" label. However, obtaining one label through majority voting ignores the important subjectivity information in tasks such hate speech detection. Existing neural network models principally regard labels as categorical variables, while ignoring the semantic information in diverse label texts. In this paper, we propose AnnoBERT, a first-of-its-kind architecture integrating annotator characteristics and label text with a transformer-based model to detect hate speech, with unique representations based on each annotator's characteristics via Collaborative Topic Regression (CTR) and integrate label text to enrich textual representations. During training, the model associates annotators with their label choices given a piece of text; during evaluation, when label information is not available, the model predicts the aggregated label given by the participating annotators by utilising the learnt association. The proposed approach displayed an advantage in detecting hate speech, especially in the minority class and edge cases with annotator disagreement. Improvement in the overall performance is the largest when the dataset is more label-imbalanced, suggesting its practical value in identifying real-world hate speech, as the volume of hate speech in-the-wild is extremely small on social media, when compared with normal (non-hate) speech. Through ablation studies, we show the relative contributions of annotator embeddings and label text to the model performance, and tested a range of alternative annotator embeddings and label text combinations.
The rapid growth of satellite network operators (SNOs) has revolutionized broadband communications, enabling global connectivity and bridging the digital divide. As these networks expand, it is important to evaluate their performance and efficiency. This paper presents the first comprehensive study of SNOs. We take an opportunistic approach and devise a methodology which allows to identify public network measurements performed via SNOs. We apply this methodology to both M-Lab and RIPE public datasets which allowed us to characterize low level performance and footprint of up to 18 SNOs operating in different orbits. Finally, we identify and recruit paid testers on three popular SNOs (Starlink, HughesNet, and ViaSat) to evaluate the performance of popular applications like web browsing and video streaming.
We present a comprehensive theoretical framework for window-based congestion control protocols that are designed to converge to fairness and efficiency. We first derive a sufficient condition for convergence to fairness. Using this, we show how fair window increase/decrease policies can be constructed from suitable pairs of monotonically non-decreasing functions. We show that well-studied protocols such as TCP, GAIMD (general additive-increase multiplicative-decrease) and binomial congestion control can be constructed using this method. Thus we provide a common framework for the analysis of such window-based protocols. To validate our approach, we present experimental results for a new TCP-friendly protocol, LOG, designed using this framework with the objective of reconciling the smoothness requirement of streaming media-like applications with the need for a fast dynamic response to congestion.
The Internet has evolved into a huge video delivery infrastructure, with websites such as YouTube and Netflix appearing at the top of most traffic measurement studies. However, most traffic studies have largely kept silent about an area of the Internet that (even today) is poorly understood: adult media distribution. Whereas ten years ago, such services were provided primarily via peer-to-peer file sharing and bespoke websites, recently these have converged towards what is known as ``Porn 2.0''. These popular web portals allow users to upload, view, rate and comment videos for free. Despite this, we still lack even a basic understanding of how users interact with these services. This paper seeks to address this gap by performing the first large-scale measurement study of one of the most popular Porn 2.0 websites: YouPorn. We have repeatedly crawled the website to collect statistics about 183k videos, witnessing over 60 billion views. Through this, we offer the first characterisation of this type of corpus, highlighting the nature of YouPorn's repository. We also inspect the popularity of objects and how they relate to other features such as the categories to which they belong. We find evidence for a high level of flexibility in the interests of its user base, manifested in the extremely rapid decay of content popularity over time, as well as high susceptibility to browsing order. Using a small-scale user study, we validate some of our findings and explore the infrastructure design and management implications of our observations.
The viability of new mission-critical networked applications such as connected cars or remote surgery is heavily dependent on the availability of truly customized network services at a Quality of Service (QoS) level that both the network operator and the customer can agree on. This is difficult to achieve in today's mainly "best effort" Internet. Even if a level of service were to be agreed upon between a consumer and an operator, it is important for both parties to be able to scalably and impartially monitor the quality of service delivered in order to enforce the service level agreement (SLA). Building upon a recently proposed architecture for automated negotiation of SLAs using smart contracts, we develop a low overhead solution for monitoring these SLAs and arranging automated payments based on the smart contracts. Our solution uses cryptographically secure bloom filters to create succinct summaries of the data exchanged over fine-grained epochs. We then use a state channel-based design for both parties to quickly and scalably agree and sign off on the data that was delivered in each epoch, making it possible to monitor and enforce at run time the agreed upon QoS levels.
TV White Spaces technology is a means of allowing wireless devices to opportunistically use locally-available TV channels (TV White Spaces), enabled by a geolocation database. The geolocation database informs the device of which channels can be used at a given location, and in the UK/EU case, which transmission powers (EIRPs) can be used on each channel based on the technical characteristics of the device, given an assumed interference limit and protection margin at the edge of the primary service coverage area(s). The UK regulator, Ofcom, has initiated a large-scale Pilot of TV White Spaces technology and devices. The ICT-ACROPOLIS Network of Excellence, teaming up with the ICT-SOLDER project and others, is running an extensive series of trials under this effort. The purpose of these trials is to test a number of aspects of white space technology, including the white space device and geolocation database interactions, the validity of the channel availability/powers calculations by the database and associated interference effects on primary services., and the performances of the white spaces devices, among others. An additional key purpose is to undertake a number of research investigations such as into aggregation of TV White Space resources with conventional (licensed/unlicensed) resources, secondary coexistence issues and means to mitigate such issues, and primary coexistence issues under challenging deployment geometries, among others. This paper describes our trials, their intentions and characteristics, objectives, and some early observations.
The innovation challenge seeks to design and develop a communications system that can operate anywhere globally with limited reliance on local infrastructure while ensuring support for discrete messaging. We propose building a satellite-based secure communication system that supports all modern mobile services, such as SMS and video calls with support for discreet communications using 5G 3GPP technology. To develop this novel communication solution, the project creatively builds on three distinct technologies: satellite-based transport/backhaul network, private 5G, and privacy protocols. It targets solutions to technology challenges in addition to those described in the challenge.
This paper aims to understand how third-party ecosystems have developed in four different countries: UK, China, AU, US. We are interested in how wide a view a given third-party player may have, of an individual user's browsing history over a period of time, and of the collective browsing histories of a cohort of users in each of these countries. We study this by utilizing two complementary approaches: the first uses lists of the most popular websites per country, as determined by Alexa.com. The second approach is based on the real browsing histories of a cohort of users in these countries. Our larger continuous user data collection spans over a year. Some universal patterns are seen, such as more third parties on more popular websites, and a specialization among trackers, with some trackers present in some categories of websites but not others. However, our study reveals several unexpected country-specific patterns: China has a home-grown ecosystem of third-party operators in contrast with the UK, whose trackers are dominated by players hosted in the US. UK trackers are more location sensitive than Chinese trackers. One important consequence of these is that users in China are tracked lesser than users in the UK. Our unique access to the browsing patterns of a panel of users provides a realistic insight into third party exposure, and suggests that studies which rely solely on Alexa top ranked websites may be over estimating the power of third parties, since real users also access several niche interest sites with lesser numbers of many kinds of third parties, especially advertisers.
Mobile data offloading can greatly decrease the load on and usage of current and future cellular data networks by exploiting opportunistic and frequent access to Wi-Fi connectivity. Unfortunately, Wi-Fi access from mobile devices can be difficult during typical work commutes, e.g., via trains or cars on highways. In this paper, we propose a new approach: to preload the mobile device with content that a user might be interested in, thereby avoiding the need for cellular data access. We demonstrate the feasibility of this approach by developing a supervised machine learning model that learns from user preferences for different types of content, and propensity to be guided by the user interface of the player, and predictively preload entire TV shows. Testing on a data set of nearly 3.9 million sessions from all over the U.K. to BBC TV shows, we find that predictive preloading can save over 71% of the mobile data for an average user.
On many social media and user---generated content sites, users can not only upload content but also create links with other users to follow their activities. It is interesting to ask whether the resulting user---user Followers' Network is based more on social ties, or shared interests in similar content. This paper reports our preliminary progress in answering this question using around five years of data from social video---sharing site vimeo. Many links in the Followers' Network are between users who do not have any videos in common, which would imply the network is not interest---based, but rather has a social character. However, the Followers' Network also exhibits properties unlike other social networks, for instance, clustering co---efficient is low, links are frequently not reciprocated, and users form links across vast geographical distances. In addition, analysis of the relationship strength, calculated as the number of commonly liked videos, people who follow each other and share some "likes" have more video likes in common than the general population. We conclude by speculating on the reasons for these differences and proposals for further work.
Online debates typically possess a large number of argumentative comments. Most readers who would like to see which comments are winning arguments often only read a part of the debate. Many platforms that host such debates allow for the comments to be sorted, say from the earliest to latest. How can argumentation theory be used to evaluate the effectiveness of such policies of sorting comments, in terms of the actually winning arguments displayed to a reader who may not have read the whole debate? We devise a pipeline that captures an online debate tree as a bipolar argumentation framework (BAF), which is sorted depending on the policy, giving a sequence of induced sub-BAFs representing how and how much of the debate has been read. Each sub-BAF has its own set of winning arguments, which can be quantitatively compared to the set of winning arguments of the whole BAF. We apply this pipeline to evaluate policies on Kialo debates, where it is shown that reading comments from most to least liked, on average, displays more winners than reading comments earliest first. Therefore, in Kialo, reading comments from most to least liked is on average more effective than reading from the earliest to the most recent.
The "Decentralised Web" (DW) is an evolving concept, which encompasses technologies aimed at providing greater transparency and openness on the web. The DW relies on independent servers (aka instances) that mesh together in a peer-to-peer fashion to deliver a range of services (e.g. micro-blogs, image sharing, video streaming). However, toxic content moderation in this decentralised context is challenging. This is because there is no central entity that can define toxicity, nor a large central pool of data that can be used to build universal classifiers. It is therefore unsurprising that there have been several high-profile cases of the DW being misused to coordinate and disseminate harmful material. Using a dataset of 9.9M posts from 117K users on Pleroma (a popular DW microblogging service), we quantify the presence of toxic content. We find that toxic content is prevalent and spreads rapidly between instances. We show that automating per-instance content moderation is challenging due to the lack of sufficient training data available and the effort required in labelling. We therefore propose and evaluate ModPair, a model sharing system that effectively detects toxic content, gaining an average per-instance macro-F1 score 0.89.
Decentralising the Web is a desirable but challenging goal. One particular challenge is achieving decentralised content moderation in the face of various adversaries (e.g. trolls). To overcome this challenge, many Decentralised Web (DW) implementations rely on federation policies. Administrators use these policies to create rules that ban or modify content that matches specific rules. This, however, can have unintended consequences for many users. In this paper, we present the first study of federation policies on the DW, their in-the-wild usage, and their impact on users. We identify how these policies may negatively impact "innocent"users and outline possible solutions to avoid this problem in the future.
We proposed a novel method to generate a secret between two people using a smartphone gyroscope assisted by the Fast Fourier Transform~(FFT) without communicating between two smartphones for a secret agreement. The secret generation process requires natural smartphone movements while performing day-to-day activities. Our evaluation by implementing it on Android smartphones shows a success rate above 90% with entropy above 6/8 bits. The code implements the secret generation method and its evaluation in Python.
The code implements the Android App to generate a secret using smartphone gyroscope data. It uses the publically available FFT library and is written in Android Java.
Content Delivery Networks aim at delivering the desired content to each user at minimum delay and cost. To tackle this problem, the content placement problem considering available cache locations has been widely studied. However, this paper addresses this problem by taking advantage of using existing but still underused Wi-Fi links. Our study considers to cache content in user homes and sharing it among neighbours via Wi-Fi links. To maximizee energy savings and reduce delays, content should be intelligently placed at the caches distributed in different users' homes. We propose using a `game theoretic centrality' metric, which models the sharing of content among neighbours as a co-operative coalition game. We apply this metric to study the energy savings and evaluate how close the contents are placed to the interested user(s).
Online forums that allow for participatory engagement between users have been transformative for the public discussion of many important issues. However, such conversations can sometimes escalate into full-blown exchanges of hate and misinformation. Existing approaches in natural language processing (NLP), such as deep learning models for classification tasks, use as inputs only a single comment or a pair of comments depending upon whether the task concerns the inference of properties of the individual comments or the replies between pairs of comments, respectively. But in online conversations, comments and replies may be based on external context beyond the immediately relevant information that is input to the model. Therefore, being aware of the conversations’ surrounding contexts should improve the model’s performance for the inference task at hand. We propose GraphNLI 1 , a novel graph-based deep learning architecture that uses graph walks to incorporate the wider context of a conversation in a principled manner. Specifically, a graph walk starts from a given comment and samples “nearby” comments in the same or parallel conversation threads, which results in additional embeddings that are aggregated together with the initial comment’s embedding. We then use these enriched embeddings for downstream NLP prediction tasks that are important for online conversations. We evaluate GraphNLI on two such tasks - polarity prediction and misogynistic hate speech detection - and find that our model consistently outperforms all relevant baselines for both tasks. Specifically, GraphNLI with a biased root-seeking random walk performs with a macro- F 1 score of 3 and 6 percentage points better than the best-performing BERT-based baselines for the polarity prediction and hate speech detection tasks, respectively. We also perform extensive ablative experiments and hyperparameter searches to understand the efficacy of GraphNLI. This demonstrates the potential of context-aware models to capture the global context along with the local context of online conversations for these two tasks.
In the missile borne monopulse radar system, effectiveness of jamming the receiver in presence of internal and external noise is much significant. In this paper, jamming of such radar receiver in frequency domain is studied when White Gaussian Noise (WGN) and Phase Noise (PN) signals are injected into the receiver in two separate cases. The missile radar receiver operates on unmodulated continuous wave sinusoidal echo signal and the jammer is assumed to be a WGN source which generates Gaussian noise samples with zero mean. The Gaussian noise signal is injected into the receiver along with the radar echo signal and the noise power required for breaking the frequency lock in the receiver is reported. Initially, it is assumed that receiver is locked onto the desired radar echo signal frequency as the noise power is too less to break the frequency lock of the receiver. It is verified that Gaussian noise power required for jamming the receiver depends upon how the power is interpreted. For our simulation, the noise power is interpreted in symbol rate bandwidth, sampling frequency bandwidth, and in single-sided and double-sided power spectral density. The break-lock in the radar receiver is presented. In the case of phase noise, the noise is added to phase of the radar echo signal and the phase noise mask required for break-lock in the receiver is studied. The phase noise is specified through a phase noise mask consisting of frequency and dBc/Hz values. It is verified that phase noise mask required for jamming the receiver is less when frequency offset from echo signal is large. The effects of windowing techniques when implemented in the phase noise measurement are presented. It is shown that the windowing technique reduces the phase noise required for breaking the frequency lock in the receiver. The effectiveness of noise jamming is carried out through computer simulation using AWR (Visual System Simulator) software. The receiver response is observed online in the frequency spectrum of the signal.
Using nine months of access logs comprising 1.9 Billion sessions to BBC iPlayer, we survey the UK ISP ecosystem to understand the factors affecting adoption and usage of a high bandwidth TV streaming application across different providers. We find evidence that connection speeds are important and that external events can have a huge impact for live TV usage. Then, through a temporal analysis of the access logs, we demonstrate that data usage caps imposed by mobile ISPs significantly affect usage patterns, and look for solutions. We show that product bundle discounts with a related fixed-line ISP, a strategy already employed by some mobile providers, can better support user needs and capture a bigger share of accesses. We observe that users regularly split their sessions between mobile and fixed-line connections, suggesting a straightforward strategy for offloading by speculatively pre-fetching content from a fixed-line ISP before access on mobile devices.
Wi-Fi, the most commonly used access technology at the very edge, supports download speeds that are orders of magnitude faster than the average home broadband or cellular data connection. Furthermore, it is extremely common for users to be within reach of their neighbours' Wi-Fi access points. Given the skewed nature of interest in content items, it is likely that some of these neighbours are interested in the same items as the users. We sketch the design of Wi-Stitch, an architecture that exploits these observations to construct a highly efficient content sharing infrastructure at the very edge and show through analysis of a real workload that it can deliver substantial (up to 70%) savings in network traffic. The Wi-Stitch approach can be used both by clients of fixed-line broadband, as well as mobile devices obtaining indoors access in converged networks.
Peer-assisted content delivery networks have recently emerged as an economically viable alternative to traditional content delivery approaches: the feasibility studies conducted for several large content providers suggested a remarkable potential of peer-assisted content delivery networks to reduce the burden of user requests on content delivery servers and several commercial peer-assisted deployments have been recently introduced. Yet there are many technical and commercial challenges which question the future of peer-assisted solutions in industrial settings. This includes among others unreliability of peer to-peer networks, the lack of incentives for peers' participation, and copyright issues. In this paper, we carefully review and systematize this ongoing debate around the future of peer-assisted networks and propose a novel taxonomy to characterize the research and industrial efforts in the area. To this end, we conduct a comprehensive survey of the last decade in the peer-assisted content delivery research and devise a novel taxonomy to characterize the identified challenges and the respective proposed solutions in the literature. Our survey includes a thorough review of the three very large scale feasibility studies conducted for BBC iPlayer, MSN Video and Conviva, five large commercial peer-assisted CDNs - Kankan, LiveSky, Akamai NetSession, Spotify, Tudou - and a vast scope of technical papers. We focus both on technical challenges in deploying peer-assisted solutions and also on non-technical challenges caused due to heterogeneity in user access patterns and distribution of resources among users as well as commercial feasibility related challenges attributed to the necessity of accounting for the interests and incentives of Internet Service Providers, End-Users and Content Providers. The results of our study suggest that many of technical challenges for implementing peer-assisted content delivery networks on an industrial scale have been already addressed in the literature, whereas a problem of finding economically viable solutions to incentivize participation in peer-assisted schemes remains an open issue to a large extent. Furthermore, the emerging Internet of Things (IoT) is expected to enable expansion of conventional CDNs to a broader network of connected devices through machine to machine communication. (C) 2017 The Authors. Published by Elsevier B.V.
TV White Spaces (TVWS) and associated spectrum sharing mechanisms represent key means of realizing necessary prime-frequency spectrum for future wireless communication systems. We have been leading a major trial of TVWS technology within the Ofcom TV White Spaces Pilot. As one aspect of the work of our trial, we have investigated solutions for aggregation in TVWS and as part of that the performance of InterDigital White Space Devices (WSDs), capable of aggregating a IEEE 802.11 enabled technology for operation in up to 4 TVWS channels, non-contiguously as well as contiguously. This paper reports on some of our assessment of aggregation in TVWS, as well as our assessment of the InterDigital WSDs. It reports on the white space channel availabilities that can be achieved through aggregation, based on a real implementation of a WSD exhaustively testing a large area of England with a high resolution. The considerable benefit that is achieved through allowing non-contiguous aggregation as compared with contiguous-only aggregation is shown. Further, this paper assesses the TCP and UDP throughput performances of the InterDigital WSDs against the number of channels aggregated and received signal powers, in highly controlled scenarios. Statistics on performance of the WSDs for the studied large area of England are derived based on this. These results are compared with theoretical similar WSDs with one major difference that they can only achieve contiguous channel aggregation. Results show almost a doubling of capacity through non-contiguous aggregation with the InterDigital WSDs; this performance benefit would be increased significantly if more than 4 channels were supported for aggregation.
Caching of video files at the wireless edge, i.e., at the base stations or on user devices, is a key method for improving wireless video delivery. While global popularity distributions of video content have been investigated in the past and used in a variety of caching algorithms, this paper investigates the statistical modeling of the individual user preferences . With individual preferences being represented by probabilities, we identify their critical features and parameters and propose a novel modeling framework by using a genre-based hierarchical structure as well as a parameterization of the framework based on an extensive real-world data set. Besides, the correlation analysis between parameters and critical statistics of the framework is conducted. With the framework, an implementation recipe for generating practical individual preference probabilities is proposed. By comparing with the underlying real data, we show that the proposed models and generation approach can effectively characterize the individual preferences of users for video content.
IoT-driven smart societies are modern service-networked ecosystems, whose proper functioning is hugely based on the success of supply chain relationships. Robust security is still a big challenge in such ecosystems, catalyzed primarily by naive cyber-security practices (e.g., setting default IoT device passwords) on behalf of the ecosystem managers, i.e., users and organizations. This has recently led to some catastrophic malware-driven DDoS and ransomware attacks (e.g., the Mirai and WannaCry attacks). Consequently, markets for commercial third-party cyber-risk management (CRM) services (e.g., cyber-insurance) are steadily but sluggishly gaining traction with the rapid increase of IoT deployment in society, and provides a channel for ecosystem managers to transfer residual cyber-risk post attack events. Current empirical studies have shown that such residual cyber-risks affecting smart societies are often heavy-tailed in nature and exhibit tail dependencies . This is both, a major concern for a profit-minded CRM firm that might normally need to cover multiple such dependent cyber-risks from different sectors (e.g., manufacturing and energy) in a service-networked ecosystem, and a good intuition behind the sluggish market growth of CRM products. In this article, we provide: 1) a rigorous general theory to elicit conditions on (tail-dependent) heavy-tailed cyber-risk distributions under which a risk management firm might find it (non)sustainable to provide aggregate cyber-risk coverage services for smart societies and 2) a real-data-driven numerical study to validate claims made in theory assuming boundedly rational cyber-risk managers, alongside providing ideas to boost markets that aggregate dependent cyber-risks with heavy-tails. To the best of our knowledge, this is the only complete general theory till date on the feasibility of aggregate CRM.
The physics and economics of cellular networks often means that there is a need to treat some services differently. This reality has spawned several technical mechanisms in the industry (e.g. DiffServ, QCI) and lured policymakers to promulgate, sometimes, unclear service classes (e.g. FCC's non-BIAS in the US). Yet, in the face of Net Neutrality expectations, this mixture of technical and policy toolkit has had little commercial impact, with no clear roadmap on how cellular operators should differentiate between services. Worse, the lack of clarity has disincentivised innovations that would increase the utilisation of the network or improve its operational efficiency. It has also discouraged the introduction of more customer choice on how to manage the priority of their own services. As policymakers begin the process of crafting the rules that will guide the 5G era, our contribution in this position paper is to bring better clarity on the nature and treatment of differentiated services in the industry. We introduce a clarifying framework of seven differentiated service classes (statutory, critical, best effort, commercially-preferred, discounted, delayed and blocked). Our framework is designed to shape discussions, provide guidance to stakeholders and inform policymaking on how to define, design, implement and enforce differentiated service classes in the 5G era.
The Pocket Switched Network (PSN) is a radical proposal to take advantage of short-range connectivity afforded by human face-to-face contacts, and create longer paths by having intermediate nodes ferry data on behalf of the sender. The Pocket Switched Network creates paths over time using transient social contacts. This chapter explores the achievable connectivity properties of this dynamically changing mileu, and gives a community-based heuristic to find efficient routes. We first employ empirical traces to examine the effect of the human contact process on data delivery. Contacts between a few node pairs are found to occur too frequently, leading to inadequate mixing of data, while the majority of contacts occur rarely, but are essential for global connectivity. We then examine all successful paths found by flooding and show that though delivery times vary widely, randomly sampling a small number of paths between each source and destination is sufficient to yield a delivery time distribution close to that of flooding over all paths. Thus, despite the apparent fragility implied by the reliance on rare edges, the rate at which the network can deliver data is remarkably robust to path failures. We then give a natural heuristic that finds routes by exploiting the latent social structure. Previous methods relied on building and updating routing tables to cope with dynamic network conditions. This has been shown to be cost ineffective due to the partial capture of transient network behavior. A more promising approach would be to capture the intrinsic characteristics of such networks and utilize them for routing decsions. We design and evaluate BUBBLE, a novel social-based forwarding algorithm, that utilizes the centrality and community metrics to enhance delivery performance. We empirically show that BUBBLE can efficiently identify good paths using several real mobility datasets.
Technology-facilitated Intimate Partner Violence (IPV) is especially pernicious because it is common for one person (assumed to be an abusive partner) to be responsible for setting up the household's technical infrastructure, which can be used to snoop over the victim. In this paper, we proposed a novel method to generate a secret between the victim and an external supportive agent using a smartphone gyroscope assisted by the Fast Fourier Transform (FFT) without any communication between two smartphones for secret agreement. The secret generation process requires natural smart-phone movements while performing day-today activities. Our evaluation by implementing it on Android smartphones shows a success rate between 90 − −99%. We proved the resilience of the generated secret under spoofing and brute-force attacks. Thus, the method allows IPV victims to generate a secret to encrypt their communication with an external supporting agent over conventional communication services in the presence of a powerful IPV adversary.
In this paper, we are interested in understanding the interrelationships between mainstream and social media in forming public opinion during mass crises, specifically in regards to how events are framed in the mainstream news and on social networks and to how the language used in those frames may allow to infer political slant and partisanship. We study the lingual choices for political agenda setting in mainstream and social media by analyzing a dataset of more than 40M tweets and more than 4M news articles from the mass protests in Ukraine during 2013–2014—known as “Euromaidan”—and the post-Euromaidan conflict between Russian, pro-Russian and Ukrainian forces in eastern Ukraine and Crimea. We design a natural language processing algorithm to analyze at scale the linguistic markers which point to a particular political leaning in online media and show that political slant in news articles and Twitter posts can be inferred with a high level of accuracy. These findings allow us to better understand the dynamics of partisan opinion formation during mass crises and the interplay between mainstream and social media in such circumstances.
In a battle engagement scenario, while missile interception and hard kill options can be exercised, soft kill options are less expensive and elegant. In this paper, optimum positioning of an active decoy which is fired in the form of a cartridge from the platform of the target is reported. Various radar and jammer parameters for effective luring away of the missile are studied. Computer simulations are carried out and it is shown that miss distances of the order of half a Kilo meter or more can be obtained for typical monopulse radars.
The rapid growth of online health communities and the increasing availability of relational data from social media provide invaluable opportunities for using network science and big data analytics to better understand how patients and caregivers can benefit from online conversations. Here, we outline a new network-based theory of social medical capital that will open up new avenues for conducting large-scale network studies of online health communities and devising effective policy interventions aimed at improving patients' self-care and health.
In search of scalable solutions, CDNs are exploring P2P support. However, the benefits of peer assistance can be limited by various obstacle factors such as ISP friendliness - requiring peers to be within the same ISP, bitrate stratification - the need to match peers with others needing similar bitrate, and partial participation - some peers choosing not to redistribute content. This work relates potential gains from peer assistance to the average number of users in a swarm, its capacity, and empirically studies the effects of these obstacle factors at scale, using a month-long trace of over 2 million users in London accessing BBC shows online. Results indicate that even when P2P swarms are localised within ISPs, up to 88% of traffic can be saved. Surprisingly, bitrate stratification results in 2 large sub-swarms and does not significantly affect savings. However, partial participation, and the need for a minimum swarm size do affect gains. We investigate improvements to gain from increasing content availability through two well-studied techniques: content bundling-combining multiple items to increase availability, and historical caching of previously watched items. Bundling proves ineffective as increased server traffic from larger bundles outweighs benefits of availability, but simple caching can considerably boost traffic gains from peer assistance.
Copying, sharing and linking have always been important for the functioning and the growth of the World Wide Web. Two recent copying trends which have emerged are social content curation, and social logins. Social curation involves the copying, categorization and sharing of links and images from third party websites on the social curation website. Social logins enable the copying of user identities and their friends from an established social network such as Facebook or Twitter, onto third party websites. In this article, we chronicile our ongoing work on Pinterest, a popular image sharing website and social network. The highly active user community on Pinterest has been instrumental in making social curation a mainstream phenomenon. Interestingly, a large fraction (nearly 60%) of the users have also linked their Pinterest accounts with Facebook and have copied their Facebook friends over onto the new website. Thus, using a large dataset crawled from Pinterest, we uncover both the practices used for sharing content, as well as how the copying of friends has helped the content sharing. We find that social curation tends to copy and share hard-to-find niche interest content from websites with a low Alexa Rank or Google Page Rank, and curators with consistent updates and a diversity of interests are popular and attract more followers. On the other hand, Pinterest users can also copy friends from Faceebook, or Twitter. We find that this copying of friends create a community with higher levels of social interaction; thus social logins serve as a social bootstrapping tool. But beyond bootstrapping, we also find a weaning process, where active and influential users tend to form more links natively on Pinterest interact with native friends rather than copied friends.
On most current websites untrustworthy or spammy identities are easily created. Existing proposals to detect untrustworthy identities rely on reputation signals obtained by observing the activities of identities over time within a single site or domain; thus, there is a time lag before which websites cannot easily distinguish attackers and legitimate users. In this paper, we investigate the feasibility of leveraging information about identities that is aggregated across multiple domains to reason about their trustworthiness. Our key insight is that while honest users naturally maintain identities across multiple domains (where they have proven their trustworthiness and have acquired reputation over time), attackers are discouraged by the additional effort and costs to do the same. We propose a flexible framework to transfer trust between domains that can be implemented in today's systems without significant loss of privacy or significant implementation overheads. We demonstrate the potential for inter-domain trust assessment using extensive data collected from Pinterest, Facebook, and Twitter. Our results show that newer domains such as Pinterest can benefit by transferring trust from more established domains such as Facebook and Twitter by being able to declare more users as likely to be trustworthy much earlier on (approx. one year earlier).
This paper looks at optimising the energy costs for storing user-generated content when accesses are highly skewed towards a few "popular" items, but the popularity ranks vary dynamically. Using traces from a video-sharing website and a social news website, it is shown that the non-popular content, which constitute the majority by numbers, tend to have accesses which spread locally in the social network, in a viral fashion. Based on the proportion of viral accesses, popular data is separated onto a few disks on storage. The popular disks receive the majority of accesses, allowing other disks to be spun down when there are no requests, saving energy. Our technique, SpinThrift, improves upon Popular Data Concentration (PDC), which, in contrast with our binary separation between popular and unpopular items, directs the majority of accesses to a few disks by arranging data according to popularity rank. Disregarding the energy required for data reorganisation, SpinThrift and PDC display similar energy savings. However, because of the dyamically changing popularity ranks, SpinThrift requires less than half the number of data reorderings compared to PDC.
This work presents a comprehensive theoretical framework for memoryless window-based congestion control protocols that are designed to converge to fairness and efficiency. We first derive a necessary and sufficient condition for stepwise convergence to fairness. Using this, we show how fair window increase/decrease policies can be constructed from suitable pairs of monotonically nondecreasing functions. We generalize this to smooth protocols that converge over each congestion epoch. The framework also includes a simple method for incorporating TCP-friendliness.Well-studied congestion control protocols such as TCP, GAIMD, and Binomial congestion control can be constructed using this method. Thus, we provide a common framework for the analysis of such window-based protocols. We also present two new congestion control protocols for streaming media-like applications as examples of protocol design in this framework: The first protocol, LOG, has the objective of reconciling the smoothness requirement of an application with the need for a fast dynamic response to congestion.The second protocol, SIGMOID, guarantees a minimum bandwidth for an application but behaves exactly like TCP for large windows.
Conference Title: 2022 IEEE International Conference on Pervasive Computing and Communications Workshops and other Affiliated Events (PerCom Workshops) Conference Start Date: 2022, March 21 Conference End Date: 2022, March 25 Conference Location: Pisa, ItalyPresents the introductory welcome message from the conference proceedings. May include the conference officers' congratulations to all involved with the conference event and publication of the proceedings record.
Service liability interconnections among networked IT and IoT-driven service organizations create potential channels for cascading service disruptions due to modern cybercrimes such as DDoS, APT, and ransomware attacks. These attacks are known to inflict cascading catastrophic service disruptions worth billions of dollars across organizations and critical infrastructure around the globe. Cyber-insurance is a risk management mechanism that is gaining increasing industry popularity to cover client (organization) risks after a cyberattack. However, there is a certain likelihood that the nature of a successful attack is of such magnitude that an organizational client's insurance provider is not able to cover the multi-party aggregate losses incurred upon itself by its clients and their descendants in the supply chain, thereby needing to re-insure itself via other cyber-insurance firms. To this end, one question worth investigating in the first place is whether an ecosystem comprising a set of profit-minded cyber-insurance companies, each capable of providing re-insurance services for a service-networked IT environment, is economically feasible to cover the aggregate cyber-losses arising due to a cyber-attack. Our study focuses on an empirically interesting case of extreme heavy tailed cyber-risk distributions that might be presenting themselves to cyber-insurance firms in the modern Internet age in the form of catastrophic service disruptions, and could be a possible standard risk distribution to deal with in the near IoT age. Surprisingly, as a negative result for society in the event of such catastrophes, we prove via a game-theoretic analysis that it may not be economically incentive compatible, even under i.i.d. statistical conditions on catastrophic cyber-risk distributions, for limited liability-taking risk-averse cyber-insurance companies to offer cyber re-insurance solutions despite the existence of large enough market capacity to achieve full cyber-risk sharing. However, our analysis theoretically endorses the popular opinion that spreading i.i.d. cyber-risks that are not catastrophic is an effective practice for aggregate cyber-risk managers, a result established theoretically and empirically in the past. A failure to achieve a working re-insurance market in critically demanding situations after catastrophic cyber-risk events strongly calls for centralized government regulatory action/intervention to promote risk sharing through re-insurance activities for the benefit of service-networked societies in the IoT age.
Introduction In the UK, approximately 4.3 million adults have asthma, with one-third experiencing poor asthma control, affecting their quality of life, and increasing their healthcare use. Interventions promoting emotional/behavioural self-management can improve asthma control and reduce comorbidities and mortality. Integration of online peer support into primary care services to foster self-management is a novel strategy. We aim to co-design and evaluate an intervention for primary care clinicians to promote engagement with an asthma online health community (OHC). Our protocol describes a ‘survey leading to a trial’ design as part of a mixed-methods, non-randomised feasibility study to test the feasibility and acceptability of the intervention. Methods and analysis Adults on the asthma registers of six London general practices (~3000 patients) will be invited to an online survey, via text messages. The survey will collect data on attitudes towards seeking online peer support, asthma control, anxiety, depression, quality of life, information on the network of people providing support with asthma and demographics. Regression analyses of the survey data will identify correlates/predictors of attitudes/receptiveness towards online peer support. Patients with troublesome asthma, who (in the survey) expressed interest in online peer support, will be invited to receive the intervention, aiming to reach a recruitment target of 50 patients. Intervention will involve a one-off, face-to-face consultation with a practice clinician to introduce online peer support, sign patients up to an established asthma OHC, and encourage OHC engagement. Outcome measures will be collected at baseline and 3 months post intervention and analysed with primary care and OHC engagement data. Recruitment, intervention uptake, retention, collection of outcomes, and OHC engagement will be assessed. Interviews with clinicians and patients will explore experiences of the intervention. Ethics and dissemination Ethical approval was obtained from a National Health Service Research Ethics Committee (reference: 22/NE/0182). Written consent will be obtained before intervention receipt and interview participation. Findings will be shared via dissemination to general practices, conference presentations and peer-reviewed publications. Trial registration number NCT05829265 .
In online debates, as in offline ones, individual utterances or arguments support or attack each other, leading to some subset of arguments (potentially from different sides of the debate) being considered more relevant than others. However, online conversations are much larger in scale than offline ones, with often hundreds of thousands of users weighing in, collaboratively forming large trees of comments by starting from an original post and replying to each other. In large discussions, readers are often forced to sample a subset of the arguments being put forth. Since such sampling is rarely done in a principled manner, users may not read all the relevant arguments to get a full picture of the debate from a sample. This article is interested in answering the question of how users should sample online conversations to selectively favour the currently justified or accepted positions in the debate. We apply techniques from argumentation theory and complex networks to build a model that predicts the probabilities of the normatively justified arguments given their location in idealised online discussions of comments and replies, which we represent as trees. Our model shows that the proportion of replies that are supportive, the distribution of the number of replies that comments receive, and the locations of comments that do not receive replies (i.e., the “leaves” of the reply tree) all determine the probability that a comment is a justified argument given its location. We show that when the distribution of the number of replies is homogeneous along the tree length, for acrimonious discussions (with more attacking comments than supportive ones), the distribution of justified arguments depends on the parity of the tree level, which is the distance from the root expressed as number of edges. In supportive discussions, which have more supportive comments than attacks, the probability of having justified comments increases as one moves away from the root. For discussion trees that have a non-homogeneous in-degree distribution, for supportive discussions we observe the same behaviour as before, while for acrimonious discussions we cannot observe the same parity-based distribution. This is verified with data obtained from the online debating platform Kialo. By predicting the locations of the justified arguments in reply trees, we can therefore suggest which arguments readers should sample, to grasp the currently accepted opinions in such discussions. Our models have important implications for the design of future online debating platforms.
The acquisition of Twitter by Elon Musk has spurred controversy and uncertainty among Twitter users. The move raised as many praises as concerns, particularly regarding Musk's views on free speech. As a result, a large number of Twitter users have looked for alternatives to Twitter. Mastodon, a decentralized micro-blogging social network, has attracted the attention of many users and the general media. In this paper, we track and analyze the migration of 136,009 users from Twitter to Mastodon. Our analysis sheds light on the user-driven pressure towards centralization in a decentralized ecosystem and identifies the strong influence of the social network in platform migration. We also characterize the activity of migrated users on both Twitter and Mastodon.
Edge computing is considered a key enabler to deploy Artificial Intelligence platforms to provide real-time applications such as AR/VR or cognitive assistance. Previous works show computing capabilities deployed very close to the user can actually reduce the end-to-end latency of such interactive applications. Nonetheless, the main performance bottleneck remains in the machine learning inference operation. In this paper, we question some assumptions of these works, as the network location where edge computing is deployed, and considered software architectures within the framework of a couple of popular machine learning tasks. Our experimental evaluation shows that after performance tuning that leverages recent advances in deep learning algorithms and hardware, network latency is now the main bottleneck on end-to-end application performance. We also report that deploying computing capabilities at the first network node still provides latency reduction but, overall, it is not required by all applications. Based on our findings, we overview the requirements and sketch the design of an adaptive architecture for general machine learning inference across edge locations.
Society is increasingly reliant on digital services for its proper functioning. Yet, going into the 5G era, the prevailing paradigm for data treats all traffic as equal regardless of how critical they are to the proper functioning of society. We argue that this is a suboptimal scenario and that services such as driverless cars and road/rail traffic updates are too important for society to be treated the same way as entertainment services. Our contribution in this paper is to propose the CLASP (Critical, Localized, Authorized, Specific, Perishable) framework to guide regulators and policymakers in deciding and managing 999-style priority lanes for critical data services during atypical scenarios in the 5G era. Our evaluation shows that reserving a 100kbps `lane' for CLASP-prioritised traffic for all users does not lead to an overall statistically significant deterioration in atypical scenarios.
We describe the design and implementation of PEG, a networked system of distributed sensor nodes that detects an uncooperative agent called the evader and assists an autonomous robot called the pursuer in capturing the evader. PEG requires embedded network services such as leader election, routing, network aggregation, and closed loop control. Instead of using general purpose distributed system solutions for these services, we employ whole-system analysis and rely on spatial and physical properties to create simple and efficient mechanisms. We believe this approach advances sensor network design, yielding pragmatic solutions that leverage physical properties to simplify design of embedded distributed systems. We deployed PEG on a 400 square meter field using 100 sensor nodes, and successfully intercepted the evader in all runs. We confronted practical issues such as node breakage, packaging decisions, in situ debugging, network reprogramming, and system reconfiguration. We discuss the approaches we took to cope with these issues and share our experiences in deploying a realistic outdoor sensor network system.
The Decentralised Web (DW) has recently seen a renewed momentum, with a number of DW platforms like Mastodon, PeerTube, and Hubzilla gaining increasing traction. These offer alternatives to traditional social networks like Twitter, YouTube, and Facebook, by enabling the operation of web infrastructure and services without centralised ownership or control. Although their services differ greatly, modern DW platforms mostly rely on two key innovations: first, their open source software allows anybody to setup independent servers ("instances") that people can sign-up to and use within a local community; and second, they build on top of federation protocols so that instances can mesh together, in a peer-to-peer fashion, to offer a globally integrated platform. In this paper, we present a measurement-driven exploration of these two innovations, using a popular DW microblogging platform (Mastodon) as a case study. We focus on identifying key challenges that might disrupt continuing efforts to decentralise the web, and empirically highlight a number of properties that are creating natural pressures towards re-centralisation. Finally, our measurements shed light on the behaviour of both administrators (i.e., people setting up instances) and regular users who sign-up to the platforms, also discussing a few techniques that may address some of the issues observed.
This work introduces IARank, a novel, simple and accurate model to continuously rank influential Twitter users in real-time. Our model is based on the information amplification potential of a user, the capacity of the user to increase the audience of a tweet or another username that they find interesting, by retweets or mentions. We incorporate information amplification using two factors, the first of which indicates the tendency of a user to be retweeted or mentioned, and the second of which is proportional to the size of the audience of the retweets or mentions. We distinguish between cumulative influence acquired by a user over time, and an important tweet made by an otherwise not-important user, which deserves attention instantaneously, and devise our ranking scheme based on both notions of influence. We show that our methods produce rankings similar to PageRank, which is the basis for several other successful rankings of Twitter users. However, as opposed to PageRank-like algorithms, which take non-trivial time to converge, our method produces rankings in near-real time. We validate our results with a user-study, which shows that our method ranks top users similar to a manual ranking produced by the users themselves. Further, our ranking marginally outperformed PageRank, with 80% of the Top 5 most important users being classified as relevant to the event, whereas, PageRank had 60% of the Top 5 users marked as relevant. However, PageRank produces slightly better rankings, which correlates better with the user-produced rankings, when considering users beyond the top 5.
Monopulse technique is widely used in modern tracking radars and missile seekers for precise angle (frequency) tracking. In this paper, the break-lock behavior of phase locked loop (PLL) in monopulse radar receiver in presence of linear frequency modulated (LFM) repeater jamming signal is presented. The radar echo and LFM signals are injected into the PLL simultaneously with an assumption that initially, the PLL locks onto the echo signal frequency. The frequency deviation required for breaking the frequency lock as a function of jamming signal power and modulation rate is reported. The results show that break-lock is achieved at frequency deviation of 0.35 MHz for a typical jammer power of -14 dBm and 200 kHz modulation rate when the radar echo power at the PLL input is -14 dBm. The break-lock is also studied for different modulation rate (200, 300, 400 kHz and so) and echo signal power (-14, -10 dBm) at the input of the PLL. For the computer simulation, the radar echo and centre frequency of LFM signals are assumed at an intermediate frequency (IF) of 50 MHz such that the LFM signal closely replicate the actual radar echo signal. The PLL containing charge pump phase detector and passive loop filter is designed with a typical bandwidth of 200 kHz. The simulation is carried out using visual system simulator AWR software and potential conclusions are demonstrated. (C) 2015 Published by Elsevier B.V.
When users browse to a so-called "First Party" website, other third parties are able to place cookies on the users' browsers. Although this practice can enable some important use cases, in practice, these third party cookies also allow trackers to identify that a user has visited two or more first parties which both share the second party. This simple feature been used to bootstrap an extensive tracking ecosystem that can severely compromise user privacy. In this paper, we develop a metric called "tangle factor" that measures how a set of first party websites may be interconnected or tangled with each other based on the common third parties used. Our insight is that the interconnectedness can be calculated as the chromatic number of a graph where the first party sites are the nodes, and edges are induced based on shared third parties. We use this technique to measure the interconnectedness of the browsing patterns of over 100 users in 25 different countries, through a Chrome browser plugin which we have deployed. The users of our plugin consist of a small carefully selected set of 15 test users in UK and China, and 1000+ in-the-wild users, of whom 124 have shared data with us. We show that different countries have different levels of interconnectedness, for example China has a lower tangle factor than the UK. We also show that when visiting the same sets of websites from China, the tangle factor is smaller, due to blocking of major operators like Google and Facebook. We show that selectively removing the largest trackers is a very effective way of decreasing the interconnectedness of third party websites. We then consider blocking practices employed by privacy-conscious users (such as ad blockers) as well as those enabled by default by Chrome and Firefox, and compare their effectiveness using the tangle factor metric we have defined. Our results help quantify for the first time the extent to which one ad blocker is more effective than others, and how Firefox defaults also greatly help decrease third party tracking compared to Chrome.
This paper looks at optimising the energy costs for data storage when the work load is highly skewed by a large number of accesses from a few popular articles, but whose popularity varies dynamically. A typical example of such a work load is news article access, where the most popular is highly accessed, but which article is most popular keeps changing. The properties of dynamically changing popular content are investigated using a trace drawn from a social news web site. It is shown that a) popular content have a much larger window of interest than non-popular articles. i.e. popular articles typically have a more sustained interest rather than a brief surge of interest. b) popular content are accessed by multiple unrelated users. In contrast, articles whose accesses spread only virally, i.e. from friend to friend, are shown to have a tendency not to be popular. Using this data, we improve upon Popular Data Concentration (PDC), a technique which is used to save energy by spinning down disks that do not contain popular data. PDC requires keeping the data ordered by their popularity, which involves significant amount of data migration, when the most popular articles keep changing. In contrast, our technique, SpinThrift, detects popular data by the proportion of non-viral accesses made, and results in lesser data migration, whilst using a similar amount of energy as PDC.
Time-lapse microscopy movies have transformed the study of subcellular dynamics. However, manual analysis of movies can introduce bias and variability, obscuring important insights. While automation can overcome such limitations, spatial and temporal discontinuities in time-lapse movies render methods such as 3D object segmentation and tracking difficult. Here, we present SpinX, a framework for reconstructing gaps between successive image frames by combining deep learning and mathematical object modeling. By incorporating expert feedback through selective annotations, SpinX identifies subcellular structures, despite confounding neighbor-cell information, non-uniform illumination, and variable fluorophore marker intensities. The automation and continuity introduced here allows the precise 3D tracking and analysis of spindle movements with respect to the cell cortex for the first time. We demonstrate the utility of SpinX using distinct spindle markers, cell lines, microscopes, and drug treatments. In summary, SpinX provides an exciting opportunity to study spindle dynamics in a sophisticated way, creating a framework for step changes in studies using time-lapse microscopy.
Several content-driven platforms have adopted the ‘micro video’ format, a new form of short video that is constrained in duration, typically at most 5–10 s long. Micro videos are typically viewed through mobile apps, and are presented to viewers as a long list of videos that can be scrolled through. How should micro video creators capture viewers’ attention in the short attention span? Does quality of content matter? Or do social effects predominate, giving content from users with large numbers of followers a greater chance of becoming popular? To the extent that quality matters, what aspect of the video – aesthetics or affect – is critical to ensuring user engagement? We examine these questions using a snapshot of nearly all (\documentclass[12pt]{minimal} \usepackage{amsmath} \usepackage{wasysym} \usepackage{amsfonts} \usepackage{amssymb} \usepackage{amsbsy} \usepackage{mathrsfs} \usepackage{upgreek} \setlength{\oddsidemargin}{-69pt} \begin{document}$${>}120,000$$\end{document}) videos uploaded to globally accessible channels on the micro video platform Vine over an 8 week period. We find that although social factors do affect engagement, content quality becomes equally important at the top end of the engagement scale. Furthermore, using the temporal aspects of video, we verify that decisions are made quickly, and that first impressions matter more, with the first seconds of the video typically being of higher quality and having a large effect on overall user engagement. We verify these data-driven insights with a user study from 115 respondents, confirming that users tend to engage with micro videos based on “first sight”, and that users see this format as a more immediate and less professional medium than traditional user-generated video (e.g., YouTube) or user-generated images (e.g., Flickr).
Wikipedia is a major source of information providing a large variety of content online, trusted by readers from around the world. Readers go to Wikipedia to get reliable information about different subjects, one of the most popular being living people, and especially politicians. While a lot is known about the general usage and information consumption on Wikipedia, less is known about the life-cycle and quality of Wikipedia articles in the context of politics. The aim of this study is to quantify and qualify content production and consumption for articles about politicians, with a specific focus on UK Members of Parliament (MPs). First, we analyze spatio-temporal patterns of readers' and editors' engagement with MPs' Wikipedia pages, finding huge peaks of attention during election times, related to signs of engagement on other social media (e.g. Twitter). Second, we quantify editors' polarisation and find that most editors specialize in a specific party and choose specific news outlets as references. Finally we observe that the average citation quality is pretty high, with statements on 'Early life and career' missing citations most often (18%).
Intelligent Personal Assistants (IPAs) such as Apple's Siri, Google Now, and Amazon Alexa are becoming an increasingly important class of web-service application. In contrast to keyword-oriented web search, IPAs provide a rich query interface that enables user interaction through images, audio, and natural language queries. However, supporting this interface involves compute-intensive machine-learning inference. To achieve acceptable performance, ML-driven IPAs increasingly depend on specialized hardware accelerators (e.g. GPUs, FPGAs or TPUs), increasing costs for IPA service providers. For end-users, IPAs also present considerable privacy risks given the sensitive nature of the data they capture. In this paper, we present Privacy Preserving Intelligent Personal Assistant at the EdGEx (PAIGE), a hybrid edge-cloud architecture for privacy-preserving Intelligent Personal Assistants. PAIGE's design is founded on the assumption that recent advances in low-cost hardware for machine-learning inference offer an opportunity to offload compute-intensive IPA ML tasks to the network edge. To allow privacy-preserving access to large IPA databases for less compute-intensive pre-processed queries, PAIGE leverages trusted execution environments at the server side. PAIGE's hybrid design allows privacy-preserving hardware acceleration of compute-intensive tasks, while avoiding the need to move potentially large IPA question-answering databases to the edge. As a step towards realising PAIGE, we present a first systematic performance evaluation of existing edge accelerator hardware platforms for a subset of IPA workloads, and show they offer a competitive alternative to existing datacenter alternatives.
Everyday, millions of users save content items for future use on sites like Pinterest, by "pinning" them onto carefully categorised personal pinboards, thereby creating personal taxonomies of the Web. This paper seeks to understand Pinterest as a distributed human computation that categorises images from around the Web. We show that despite being categorised onto personal pinboards by individual actions, there is a generally a global agreement in implicitly assigning images into a coarse-grained global taxonomy of 32 categories, and furthermore, users tend to specialise in a handful of categories. By exploiting these characteristics, and augmenting with image-related features drawn from a state-of-the-art deep convolutional neural network, we develop a cascade of predictors that together automate a large fraction of Pinterest actions. Our end-to-end model is able to both predict whether a user will repin an image onto her own pinboard, and also which pinboard she might choose, with an accuracy of 0.69 (Accuracy@5 of 0.75).
Vehicular CrowdSensing (VCS) is an emerging solution designed to remotely collect data from smart vehicles. It enables a dynamic and large-scale phenomena monitoring just by exploring the variety of technologies which have been embedded in modern cars. However, VCS applications might generate a huge amount of data traffic between vehicles and the remote monitoring center, which tends to overload the LTE networks. In this paper, we describe and analyze a gEo-clUstering approaCh for Lte vehIcular crowDsEnsing dAta offloadiNg (EUCLIDEAN). It takes advantage of opportunistic vehicle-to-vehicle (V2V) communications to support the VCS data upload process, preserving, as much as possible, the cellular network resources. In general, it is shown from the presented results that our proposal is a feasible and an effective scheme to reduce up to 92.98 % of the global demand for LTE transmissions while performing vehicle-based sensing tasks in urban areas. The most encouraging results were perceived mainly under high-density conditions (i.e., above 125 vehicles/km(2)), where our solution provides the best benefits in terms of cellular network data offloading.
In this paper we propose an Enhanced Mobile SET (EMSET) protocol with formal verification using Mobile Agent technology and Digital Signature with Message Recovery based on ECDSA mechanism. Mobile Agent technology and Digital Signature with Message Recovery (DSMR) based on ECDSA mechanism provides in proposing EMSET protocol in Mobile Networks. Mobile Agent technology has many benefits such as bandwidth conservation, reduction of latency, reduction of completion time, Asynchronous (disconnected) communications. Digital Signature with Message Recovery based on ECDSA eliminates the need of adopting PKI cryptosystems. Our proposed protocol EMSET ensures Authentication, Integrity, Confidentiality and Non Repudiation, achieves Identity protection from merchant and Eavesdropper, achieves Transaction privacy from Eavesdropper and Payment Gateway, achieves Payment Secrecy, Order Secrecy, forward secrecy, and prevents Double Spending, Overspending and Money laundering. In addition to these our proposed protocol withstands Replay, Man in the Middle and Impersonation attacks. The security properties of the proposed protocol have been verified using Scyther Tool and presented with results.
How does one develop a new online community that is highly engaging to each user and promotes social interaction? A number of websites offer friend-finding features that help users bootstrap social networks on the website by copying links from an established network like Facebook or Twitter. This paper quantifies the extent to which such social bootstrapping is effective in enhancing a social experience of the website. First, we develop a stylised analytical model that suggests that copying tends to produce a giant connected component (i.e., a connected community) quickly and preserves properties such as reciprocity and clustering, up to a linear multiplicative factor. Second, we use data from two websites, Pinterest and Last.fm, to empirically compare the subgraph of links copied from Facebook to links created natively. We find that the copied subgraph has a giant component, higher reciprocity and clustering, and confirm that the copied connections see higher social interactions. However, the need for copying diminishes as users become more active and influential. Such users tend to create links natively on the website, to users who are more similar to them than their Facebook friends. Our findings give new insights into understanding how bootstrapping from established social networks can help engage new users by enhancing social interactivity.
Online conversation understanding is an important yet challenging NLP problem which has many useful applications (e.g., hate speech detection). However, online conversations typically unfold over a series of posts and replies to those posts, forming a tree structure within which individual posts may refer to semantic context from higher up the tree. Such semantic cross-referencing makes it difficult to understand a single post by itself; yet considering the entire conversation tree is not only difficult to scale but can also be misleading as a single conversation may have several distinct threads or points, not all of which are relevant to the post being considered. In this paper, we propose a Graph-based Attentive Semantic COntext Modeling (GASCOM) framework for online conversation understanding. Specifically, we design two novel algorithms that utilise both the graph structure of the online conversation as well as the semantic information from individual posts for retrieving relevant context nodes from the whole conversation. We further design a token-level multi-head graph attention mechanism to pay different attentions to different tokens from different selected context utterances for fine-grained conversation context modeling. Using this semantic conversational context, we re-examine two well-studied problems: polarity prediction and hate speech detection. Our proposed framework significantly outperforms state-of-the-art methods on both tasks, improving macro-F1 scores by 4.5% for polarity prediction and by 5% for hate speech detection. The GASCOM context weights also enhance interpretability.
In this paper we introduce LENS, a novel spam protection system based on the recipient's social network, which allows correspondence within the social circle to directly pass to the mailbox and further mitigates spam beyond social circles. The key idea in LENS is to select legitimate and authentic users, called Gatekeepers (GKs), from outside the recipients social circle and within pre-defined social distances. Unless a GK vouches for the emails of potential senders from outside the social circle of a particular recipient, those e-mails are prevented from transmission. In this way LENS drastically reduces the consumption of Internet bandwidth by spam. Using extensive evaluations, we show that LENS provides each recipient reliable email delivery from a large fraction of the social network. We also evaluate the computational complexity of email processing with LENS deployed on two Mail Servers (MSs) and compared it with the most popular content-based filter i.e SpamAssassin. LENS proved to be fast in processing emails (around 2-3 orders of magnitude better than SpamAssassin) and scales efficiently with increasing community size and GKs.
In essence, an information-centric network (ICN) is one which supports a content request/reply model. One proposed benefit of this is improved mobility. This can refer to provider, consumer or content mobility . Despite this, little specific research has looked into the effectiveness of ICN in this regard. This paper presents a survey of some of the key ICN technologies, alongside their individual approaches to mobility. Through this, we highlight some of the promising benefits of ICN, before discussing important future research questions that must be answered.
Following the rollout of the first 5G networks in 2018, press reports in the US began to emerge that the 5G icon on smartphones was not depicting 5G connectivity. Such reports about 'fake' 5G icon reverberated across the industry, exposing a mismatch between the icon on the phone and the actual experience of users. Between 2018 - early 2020, 3GPP and the GSMA sought to provide industry guidance on what and when the 5G icon should be used and how 5G performance can differ from 4G. In this paper, we introduce an intuitive four stage investigation framework to explore the technical considerations that ultimately confirm the veracity of the 5G connectivity. Then, following the launch of 5G in the UK in late 2019, we set out to explore if there were similar confusion on 5G notification and performance in the country. We conducted field measurements at the five busiest train stations in the UK, during rush hour, using a Samsung 5G S10 and a Samsung S6 Edge+ 4G device to compare 5G notifications and perceived network performance on 4G and 5G networks. We observe confusing messages to the user - device icon says 5G but Android's TelephonyManager API says 4G; worst cases for latency and uplink/downlink speeds were minimised but best case performance was the same on 4G and 5G devices. Based on our observations, and while we expect any lingering concerns to be ironed out as 5G deployment and adoption matures, we draw lessons that should guide the industry to avoid doubts about the icon and connectivity in 6G.
Social media has been on the vanguard of political information diffusion in the 21st century. Most studies that look into disinformation, political influence and fake-news focus on mainstream social media platforms. This has inevitably made English an important factor in our current understanding of political activity on social media. As a result, there has only been a limited number of studies into a large portion of the world, including the largest, multilingual and multi-cultural democracy: India. In this paper we present our characterisation of a multilingual social network in India called ShareChat. We collect an exhaustive dataset across 72 weeks before and during the Indian general elections of 2019, across 14 languages. We investigate the cross lingual dynamics by clustering visually similar images together, and exploring how they move across language barriers. We find that Telugu, Malayalam, Tamil and Kannada languages tend to be dominant in soliciting political images (often referred to as memes), and posts from Hindi have the largest cross-lingual diffusion across ShareChat (as well as images containing text in English). In the case of images containing text that cross language barriers, we see that language translation is used to widen the accessibility. That said, we find cases where the same image is associated with very different text (and therefore meanings). This initial characterisation paves the way for more advanced pipelines to understand the dynamics of fake and political content in a multi-lingual and non-textual setting. Comment: Accepted at ICWSM 2020, please cite the ICWSM version
On-demand video streaming dominates today's Internet traffic mix. For instance, Netflix constitutes a third of the peak time traffic in the USA. Nearly half of UK online households have accessed BBC's shows through its on-demand streaming interface, BBC iPlayer. Using UK-wide traces from BBC iPlayer as a case study, this talk will characterise users' content consumption at scale and discuss techniques that can be deployed at the edge by users to substantially decrease the load on the Internet. We will survey both well-known techniques such as peer-assisted VoD, studying whether it works at scale, as well as new edge-caching mechanisms that can potentially be deployed today. We will conclude by exploring new directions for content-centric network architectures, to address the roots of the pain points observed in our user workload, in a "clean" fashion.
The specialness of New Year eve traffic is a telecoms industry fable. But how true is it, and what's the impact on user experience? We investigate this on the four UK cellular networks, in London, on New Year eve in 2016/17, 2017/18, 2018/19 and 2019/20 (covid cancelled 2020/21 & 2021/22). Overall, we captured 544,560 readings across 14 categories using 3G/4G/5G devices. This paper summarises our longitudinal readings into 10 observations on the nature of network performance, from a user's perspective, on special days such as New Year eve. Based on these, we confirm that mature 3G/4G networks are unable to deliver a consistent user experience, especially on atypical days. For example, on 4G, a user had a 60% chance to get a latency below 50 ms and 90% chance for 500ms. If repeated in mature 5G networks, it suggests that it is inadequate to support safety-critical 5G use cases.
This editorial article introduces the OSNEM special issue on Detecting, Understanding and Countering Online Harms. Whilst online social networks and media have revolutionised society, leading to unprecedented connectivity across the globe, they have also enabled the spread of hazardous and dangerous behaviours. Such ‘online harms’ are now a pressing concern for policymakers, regulators and big tech companies. Building deep knowledge about the scope, nature, prevalence, origins and dynamics of online harms is crucial for ensuring we can clean up online spaces. This, in turn, requires innovation and advances in methods, data, theory and research design – and developing multi-domain and multi-disciplinary approaches. In particular, there is a real need for methodological research that develops high-quality methods for detecting online harms in a robust, fair and explainable way. With this motivation in mind, the present special issue attracted 20 submissions, of which 8 were ultimately accepted for publication in the journal. These submissions predominantly revolve around online misinformation and abusive language, with an even distribution between the two topics. In what follows, we introduce and briefly discuss the contributions of these accepted submissions.
Online forums that allow participatory engagement between users have been transformative for public discussion of important issues. However, debates on such forums can sometimes escalate into full blown exchanges of hate or misinformation. An important tool in understanding and tackling such problems is to be able to infer the argumentative relation of whether a reply is supporting or attacking the post it is replying to. This so called polarity prediction task is difficult because replies may be based on external context beyond a post and the reply whose polarity is being predicted. We propose GraphNLI, a novel graph-based deep learning architecture that uses graph walk techniques to capture the wider context of a discussion thread in a principled fashion. Specifically, we propose methods to perform root-seeking graph walks that start from a post and captures its surrounding context to generate additional embeddings for the post. We then use these embeddings to predict the polarity relation between a reply and the post it is replying to. We evaluate the performance of our models on a curated debate dataset from Kialo, an online debating platform. Our model outperforms relevant baselines, including S-BERT, with an overall accuracy of 83%.
As adoption of connected cars (CCs) grows, the expectation is that 5G will better support safety-critical vehicle-to-everything (V2X) use cases. Operationally, most relationships between cellular network providers and car manufacturers or users are exclusive, providing a single network connectivity, with at best an occasional option of a back-up plan if the single network is unavailable. We question if this setup can provide QoS assurance for V2X use cases. Accordingly, in this paper, we investigate the role of redundancy in providing QoS assurance for cellular connectivity for CCs. Using our bespoke Android measurement app, we did a drive-through test on 380 kilometers of major and minor roads in South East England. We measured round trip times, jitter, page load times, packet loss, network type, uplink speed and downlink speeds on the four UK networks for 14 UK-centric websites every five minutes. In addition, we did the same measurement using a much more expensive universal SIM card provider that promises to fall back on any of the four UK networks to assure reliability. By comparing actual performance on the best performing network versus the universal SIM, and then projected performance of a two/three/four multi-operator setup, we make three major contributions. First, the use of redundant multi-connectivity, especially if managed by the demand-side, can deliver superior performance (up to 28 percentage points in some cases). Second, despite costing 95x more per GB of data, the universal SIM performed worse than the best performing network except for uplink speed, highlighting how the choice of parameter to monitor can influence operational decisions. Third, any assessment of CC connectivity reliability based on availability is sub-optimal as it can hide significant under-performance.
Websites with hyper-partisan, left or right-leaning focus offer content that is typically biased towards the expectations of their target audience. Such content often polarizes users, who are repeatedly primed to specific (extreme) content, usually reflecting hard party lines on political and socio-economic topics. Though this polarization has been extensively studied with respect to content, it is still unknown how it associates with the online tracking experienced by browsing users, especially when they exhibit certain demographic characteristics. For example, it is unclear how such websites enable the ad-ecosystem to track users based on their gender or age. In this paper, we take a first step to shed light and measure such potential differences in tracking imposed on users when visiting specific party-line's websites. For this, we design and deploy a methodology to systematically probe such websites and measure differences in user tracking. This methodology allows us to create user personas with specific attributes like gender and age and automate their browsing behavior in a consistent and repeatable manner. Thus, we systematically study how personas are being tracked by these websites and their third parties, especially if they exhibit particular demographic properties. Overall, we test 9 personas on 556 hyper-partisan websites and find that right-leaning websites tend to track users more intensely than left-leaning, depending on user demographics, using both cookies and cookie synchronization methods and leading to more costly delivered ads.
Video accounts for a large proportion of traffic on the Internet. Understanding its geographical viewing patterns is extremely valuable for the design of Internet ecosystems for content delivery, recommendation and ads. While previous works have addressed this problem at coarse-grain scales (e.g., national), the urban-scale geographical patterns of video access have never been revealed. To this end, this article aims to investigate the problem that whether there exists distinct viewing patterns among the neighborhoods of a large-scale city. To achieve this, we need to address several challenges including unknown of patterns profiles, complicate urban neighborhoods, and comprehensive viewing features. The contributions of this article include two aspects. First, we design a framework to automatically identify geographical video viewing patterns in urban neighborhoods. Second, by using a dataset of two months real video requests in Shanghai collected from one major ISP of China, we make a rigorous analysis of video viewing patterns in Shanghai. Our study reveals the following important observations. First, there exists four prevalent and distinct patterns of video access behavior in urban neighborhoods, which are corresponding to four different geographical contexts: downtown residential, office, suburb residential and hybrid regions. Second, there exists significant features that distinguish different patterns, e.g., the probabilities of viewing TV plays at midnight, and viewing cartoons at weekends can distinguish the two viewing patterns corresponding to downtown and suburb regions.
The plane of cell division is defined by the final position of the mitotic spindle. The spindle is pulled and rotated to the correct position by cortical dynein. However, it is unclear how the spindle's rotational center is maintained and what the consequences of an equatorially off centered spindle are in human cells. We analyzed spindle movements in 100s of cells exposed to protein depletions or drug treatments and uncovered a novel role for MARK2 in maintaining the spindle at the cell's geometric center. Following MARK2 depletion, spindles glide along the cell cortex, leading to a failure in identifying the correct division plane. Surprisingly, spindle off centering in MARK2-depleted cells is not caused by excessive pull by dynein. We show that MARK2 modulates mitotic microtubule growth and length and that codepleting mitotic centromere-associated protein (MCAK), a microtubule destabilizer, rescues spindle off centering in MARK2-depleted cells. Thus, we provide the first insight into a spindle-centering mechanism needed for proper spindle rotation and, in turn, the correct division plane in human cells.
Web 2.0 sites have made networked sharing of user generated content increasingly popular. Serving rich-media content with strict delivery constraints requires a distribution infrastructure. Traditional caching and distribution algorithms are optimised for globally popular content and will not be efficient for user generated content that often show a heavy-tailed popularity distribution. New algorithms are needed. This paper shows that information encoded in social network structure can be used to predict access patterns which may be partly driven by viral information dissemination, termed as a social cascade. Specifically, knowledge about the number and location of friends of previous users is used to generate hints that enable placing replicas of the content closer to future accesses.
5G promises unprecedented levels of network connectivity to handle diverse applications, including life-critical applications such as remote surgery. However, to enable the adoption of such applications, it is important that customers trust the service quality provided. This can only be achieved through transparent Service Level Agreements (SLAs). Current resource provisioning systems are too general to handle such variety in applications. Moreover, service agreements are often opaque to customers, which can be an obstacle for 5G adoption for mission-critical services.In this work, we advocate short-term and specialised rather than long-term general service contracts and propose an end-to-end Permissioned Distributed Ledger (PDL) focused architecture; which allows operators to advertise their service contracts on a public portal backed by a PDL. These service contracts with clear Service Level Agreement (SLA) offers are deployed as smart contracts to enable transparent, automatic and immutable SLAs. To justify our choice of using a permissioned ledger instead of permissionless, we evaluated and compared contract execution times on both permissioned (i.e. Quorum and Hyperledger Fabric) and permissionless (i.e. Ropsten testnet) ledgers.
Today, the Internet is a large multimedia delivery infrastructure, with websites such as YouTube appearing at the top of most measurement studies. However, most traffic studies have ignored an important domain: adult multimedia distribution. Whereas, traditionally, such services were provided primarily via bespoke websites, recently these have converged towards what is known as "Porn 2.0". These services allow users to upload, view, rate, and comment on videos for free (much like YouTube). Despite their scale, we still lack even a basic understanding of their operation. This article addresses this gap by performing a large-scale study of one of the most popular Porn 2.0 websites: YouPorn. Our measurements reveal a global delivery infrastructure that we have repeatedly crawled to collect statistics (on 183k videos). We use this data to characterise the corpus, as well as to inspect popularity trends and how they relate to other features, for example, categories and ratings. To explore our discoveries further, we use a small-scale user study, highlighting key system implications.
The recently introduced General Data Protection Regulation (GDPR) requires that when obtaining information online that could be used to identify individuals, their consents must be obtained. Among other things, this affects many common forms of cookies, and users in the EU have been presented with notices asking their approvals for data collection. This paper examines the prevalence of third party cookies before and after GDPR by using two datasets: accesses to top 500 websites according to Alexa.com, and weekly data of cookies placed in users' browsers by websites accessed by 16 UK and China users across one year. We find that on average the number of third parties dropped by more than 10% after GDPR, but when we examine real users' browsing histories over a year, we find that there is no material reduction in long-term numbers of third party cookies, suggesting that users are not making use of the choices offered by GDPR for increased privacy. Also, among websites which offer users a choice in whether and how they are tracked, accepting the default choices typically ends up storing more cookies on average than on websites which provide a notice of cookies stored but without giving users a choice of which cookies, or those that do not provide a cookie notice at all. We also find that top non-EU websites have fewer cookie notices, suggesting higher levels of tracking when visiting international sites. Our findings have deep implications both for understanding compliance with GDPR as well as understanding the evolution of tracking on the web.
With the rise of social media, a vast amount of new primary research material has become available to social scientists, but the sheer volume and variety of this make it difficult to access through the traditional approaches: close reading and nuanced interpretations of manual qualitative coding and analysis. This paper sets out to bridge the gap by developing semi-automated replacements for manual coding through a mixture of crowdsourcing and machine learning, seeded by the development of a careful manual coding scheme from a small sample of data. To show the promise of this approach, we attempt to create a nuanced categorisation of responses on Twitter to several recent high profile deaths by suicide. Through these, we show that it is possible to code automatically across a large dataset to a high degree of accuracy (71%), and discuss the broader possibilities and pitfalls of using Big Data methods for Social Science.
Mapathons and hackathons are short-lived events with different purposes. A mapathon is a collaborative effort for collecting geographic data in unmapped areas, while hackathons are focused on application development. Mapathon outputs need to be high quality to be reusable, but often, when applications are later built on top of map data, there is a mismatch between data collected and application requirements. We conducted an international collaboration project aiming to address this situation by creating a circular process where geographic information is collected in a mapathon and later used in a hackathon. Based on user feedback this cycle can be repeated so the collected data and developed applications can be improved. In this event report, we describe the two mapathon-hackathon cycles that were part of our pilot to validate that process. We present their outcomes and some lessons learned. We focused on the so-called "blue economy" (i.e. the sustainable use of marine and ocean resources for economic growth and improved livelihoods in coastal areas.) as the target domain for this pilot. Data for carefully selected areas of the South African coast was collected through in mapathons and later use in hackathons. The mapathons were held in South Africa, and the hackathons took place in Brazil.
Online misogyny is a pernicious social problem that risks making online platforms toxic and unwelcoming to women. We present a new hierarchical taxonomy for online misogyny, as well as an expert labelled dataset to enable automatic classification of misogynistic content. The dataset consists of 6,567 labels for Reddit posts and comments. As previous research has found untrained crowdsourced annotators struggle with identifying misogyny, we hired and trained annotators and provided them with robust annotation guidelines. We report baseline classification performance on the binary classification task, achieving accuracy of 0.93 and F1 of 0.43. The codebook and datasets are made freely available for future researchers.
New applications such as remote surgery and connected cars, which are being touted as use cases for 5G and beyond, are mission-critical. As such, communications infrastructure needs to support and enforce stringent and guaranteed levels of service before such applications can take off. However, from an operator's perspective, it can be difficult to provide uniformly high levels of service over long durations or large regions. As network conditions change over time, or when a mobile end point goes to regions with poor coverage, it may be difficult for the operator to support previously agreed upon service agreements that are too stringent. Second, from a consumer's perspective, purchasing a stringent service level agreement with an operator can also be expensive. Finally, failures in mission critical applications can lead to disasters, so infrastructure should support assignment of liabilities when a guaranteed service level is reneged upon - this is a difficult problem because both the operator and the customer have an incentive to lay the blame on each other to avoid liabilities of poor service. To address the above problems, we propose AJIT, an architecture that allows creating fine-grained short-term contracts between operator and consumer. AJIT uses smart contracts to allow dynamically changing service levels so that more expensive and stringent levels of service need only be requested by a customer for short durations when the application needs it, and operator agrees to the SLA only when the infrastructure is able to support the demand. Second, AJIT uses trusted enclaves to do the accounting of packet deliveries such that neither the customer requesting guaranteed service levels for mission-critical applications, nor the operator providing the infrastructure support, can cheat.
It is evident that noise jamming is one of the several active jamming techniques employed against tracking radars and missile seekers. The noise jamming mainly aims at completely masking the desired radar signal by the externally injected noise signal. Of several parameters to be considered for the analysis of noise jamming problem, the noise jammer power is one of the most critical parameter. In this paper, emphasis is given for estimation and quantitative analyses of the effectiveness of break-lock in a missile borne phase locked loop (PLL) based monopulse radar receiver using external noise signal. The analyses involve estimating the jamming signal power required to break-lock as a function of radar echo signal power through computer simulation and experimental measurements. The simulation plots representing the receiver PLL output are presented for selected echo signal powers from -14 dBm to -2 dBm. The simulation results are compared and verified with experimental results and it is established that these results are close approximate within 2 dB. It is noted that the measured values of jamming signal power at break-lock using HMC702LP6CE, HMC703LP4E and HMC830LP6GE PLL synthesizers are -19.5 dBm, -18.1 dBm and -17.6 dBm, respectively, while the simulated value is -18.8 dBm for a typical radar echo signal power of -10 dBm. The fairly good and consistent agreement between these results validates the simulation data.
P2P sharing amongst consumers has been proposed as a way to decrease load on Content Delivery Networks. This paper develops an analytical model that shows an additional benefit of sharing content locally: Selecting close by peers to share content from leads to shorter paths compared to traditional CDNs, decreasing the overall carbon footprint of the system. Using data from a month-long trace of over 3 million monthly users in London accessing TV shows online, we show that local sharing can result in a decrease of 24-48% in the system-wide carbon footprint of online video streaming, despite various obstacle factors that can restrict swarm sizes. We confirm the robustness of the savings by using realistic energy parameters drawn from two widely used settings. We also show that if the energy savings of the CDN servers are transferred as carbon credits to the end users, over 70% of users can become carbon positive, i.e., are able to support their content consumption without incurring any carbon footprint, and are able to offset their other carbon consumption. We suggest carbon credit transfers from CDNs to end users as a novel way to incentivise participation in peer-assisted content delivery.
The exploding volumes of mobile video traffic call for deploying content caches inside mobile operators network. With in-network caching, users' requests for popular content can be served from a content cache deployed at mobile gateways in vicinity to the end user, therefore considerably reducing the load on the content servers and the backbone of operator's network. In practice, content caches can be installed at multiple levels inside an operator's network (e.g., serving gateway, packet data network gateway, RAN, etc.), leading to an idea of hierarchical in-network video caching. In order to evaluate the pros and cons of hierarchical caching, in this paper we formulate a cache provisioning problem which aims to find the best trade-off between the cost of cache storage and bandwidth savings from hierarchical caching. More specifically, we aim to find the optimal size of video caches at different layers of a hierarchical in-network caching architecture which minimizes the ratio of transmission bandwidth cost to storage cost. We overcome the complexity of our problem which is formulated as a binary-integer programming (BIP) by using canonical duality theory (CDT). Numerical results obtained using the invasive weed optimization (IWO) show that important gains can be achieved, with benefit-cost ratio and cost efficiency improvements of more than 43% and 38%, respectively.
The last 5 years have seen a dramatic shift in media distribution. For decades, TV and radio were solely provisioned using push-based broadcast technologies, forcing people to adhere to fixed schedules. The introduction of catch-up services, however, has now augmented such delivery with online pull-based alternatives. Typically, these allow users to fetch content for a limited period after initial broadcast, allowing users flexibility in accessing content. Whereas previous work has investigated both of these technologies, this paper explores and contrasts them, focusing on the network consequences of moving towards this multifaceted delivery model. Using traces from nearly 6 million users of BBC iPlayer, one of the largest catch-up TV services, we study this shift from push-to pull-based access. We propose a novel technique for unifying both push-and pull-based delivery: the Speculative Content Offloading and Recording Engine (SCORE). SCORE operates as a set-top box, which interacts with both broadcast push and online pull services. Whenever users wish to access media, it automatically switches between these distribution mechanisms in an attempt to optimize energy efficiency and network resource utilization. SCORE also can predict user viewing patterns, automatically recording certain shows from the broadcast interface. Evaluations using our BBC iPlayer traces show that, based on parameter settings, an oracle with complete knowledge of user consumption can save nearly 77% of the energy, and over 90% of the peak bandwidth, of pure IP streaming. Optimizing for energy consumption, SCORE can recover nearly half of both traffic and energy savings.
Since the inception of the first web page three decades back, the Web has evolved considerably, from static HTML pages in the beginning to the dynamic web pages of today, from mainly the text-based pages of the 1990s to today's multimedia rich pages, etc.. Although much of this is known anecdotally, to our knowledge, there is no quantitative documentation of the extent and timing of these changes. This paper attempts to address this gap in the literature by looking at the top 100 Alexa websites for over 25 years from the Internet Archive or the "Wayback Machine", archive.org. We study the changes in popularity, from Geocities and Yahoo! in the mid-to-late 1990s to the likes of Google, Facebook, and Tiktok of today. We also look at different categories of websites and their popularity over the years and find evidence for the decline in popularity of news and education-related websites, which have been replaced by streaming media and social networking sites. We explore the emergence and relative prevalence of different MIME-types (text vs. image vs. video vs. javascript and json) and study whether the use of text on the Internet is declining.
Online presence is becoming unavoidable for politicians worldwide. In countries such as the UK, Twitter has become the platform of choice, with over 85% (553 of 650) of the Members of Parliament (MPs) having an active online presence. Whereas this has allowed ordinary citizens unprecedented and immediate access to their elected representatives, it has also led to serious concerns about online hate towards MPs. This work attempts to shed light on the problem using a dataset of conversations between MPs and non-MPs over a two month period. Deviating from other approaches in the literature, our data captures entire threads of conversations between Twitter handles of MPs and citizens in order to provide a full context for content that may be flagged as 'hate'. By combining widely-used hate speech detection tools trained on several widely available datasets, we analyse 2.5 million tweets to identify hate speech against MPs and we characterise hate across multiple dimensions of time, topics and MPs' demographics. We find that MPs are subject to intense 'pile on' hate by citizens whereby they get more hate when they are already busy with a high volume of mentions regarding some event or situation. We also show that hate is more dense with regard to certain topics and that MPs who have an ethnic minority background and those holding positions in Government receive more hate than other MPs. We find evidence of citizens expressing negative sentiments while engaging in cross-party conversations, with supporters of one party (e.g. Labour) directing hate against MPs of another party (e.g. Conservative).
WhatsApp is a popular messaging app used by over a billion users around the globe. Due to this popularity, understanding misbehavior on WhatsApp is an important issue. The sending of unwanted junk messages by unknown contacts via WhatsApp remains understudied by researchers, in part because of the end-to-end encryption offered by the platform. We address this gap by studying junk messaging on a multilingual dataset of 2.6M messages sent to 5K public WhatsApp groups in India. We characterise both junk content and senders. We find that nearly 1 in 10 messages is unwanted content sent by junk senders, and a number of unique strategies are employed to reflect challenges faced on WhatsApp, e.g., the need to change phone numbers regularly. We finally experiment with on-device classification to automate the detection of junk, whilst respecting end-to-end encryption.
Caching of video files at the wireless edge, i.e., at the base stations or on user devices, is a key method for improving wireless video delivery. While global popularity distributions of video content have been investigated in the past, and used in a variety of caching algorithms, this paper investigates the statistical modeling of the individual user preferences. With individual preferences being represented by probabilities, we identify their critical features and parameters and propose a novel modeling framework as well as a parameterization of the framework based on an extensive real-world data set. Besides, an implementation recipe for generating practical individual preference probabilities is proposed. By comparing with the underlying real data, we show that the proposed models and generation approach can effectively characterize individual preferences of users for video content.
This chapter explores a collaboration between computer scientists, who take a primarily quantitative approach, and qualitative researchers in sociology and international relations. It aims to investigate how online platforms support or hinder the sharing of empathy and trust among people in extreme and vulnerable circumstances. The chapter introduces the benefits of interdisciplinary work within the emerging field of computational social science. It explored how computer and social scientists can work together to investigate these themes in relation to two different spheres: emotional distress and humanitarian and disaster-linked crises. The computer scientists in the team have devised a simple tool that finds replies to an initial data set achieved through key word searching. Identifying the potential and limits of social media research is important for international researchers and policymakers in the context of situations where access on the ground and traditional field analysis may be difficult.
ln-app advertising is a multi-billion dollar industry that is an essential part of the current digital ecosystem, and is amenable to sensitive consumer information often being sold downstream without the knowledge of consumers, and in many cases to their annoyance. While this practice, in cases, may result in long-term benefits for the consumers, it can result in serious information privacy (IP) breaches of very significant impact (e.g., breach of genetic data) in the short term. The question we raise through this article is: does the type of information being traded downstream play a role in the degree of IP risks generated? We investigate two general (one-many) information trading market structures between a single data aggregating seller (e.g., enterprise app) and multiple competing buyers (e.g., ad-networks, retailers), distinguished by mutually exclusive and privacy sanitized aggregated consumer data (information) types: (i) data entailing strategically complementary actions among buyers and (ii) data entailing strategically substituting actions among buyers. Our primary question of interest here is: trading which type of data might pose less information privacy risks for society? To this end, we show that at market equilibrium IP trading markets exhibiting strategic substitutes between buying firms pose lesser risks for IP in society, primarily because the 'substitutes' setting, in contrast to the 'complements' setting, economically incentivizes appropriate consumer data distortion by the seller in addition to restricting the proportion of buyers to which it sells. Moreover, we also show that irrespective of the data type traded by the seller, the likelihood of improved IP in society is higher if there is purposeful or free-riding based transfer/leakage of data between buying firms. This is because the seller finds itself economically incentivized to restrict the release of sanitized consumer data with respect to the span of its buyer space, as well as in improved data quality.
This paper aims to shed light on alternative news media ecosystems that are believed to have influenced opinions and beliefs by false and/or biased news reporting during the 2016 US Presidential Elections. We examine a large, professionally curated list of 668 hyper-partisan websites and their corresponding Facebook pages, and identify key characteristics that mediate the traffic flow within this ecosystem. We uncover a pattern of new websites being established in the run up to the elections, and abandoned after. Such websites form an ecosystem, creating links from one website to another, and by 'liking' each others' Facebook pages. These practices are highly effective in directing user traffic internally within the ecosystem in a highly partisan manner, with right-leaning sites linking to and liking other right-leaning sites and similarly left-leaning sites linking to other sites on the left, thus forming a filter bubble amongst news producers similar to the filter bubble which has been widely observed among consumers of partisan news. Whereas there is activity along both left- and right-leaning sites, right-leaning sites are more evolved, accounting for a disproportionate number of abandoned websites and partisan internal links. We also examine demographic characteristics of consumers of hyper-partisan news and find that some of the more populous demographic groups in the US tend to be consumers of more right-leaning sites.
Many adult content websites incorporate social networking features. Although these are popular, they raise significant challenges, including the potential for users to "catfish", i.e., to create fake profiles to deceive other users. This paper takes an initial step towards automated catfish detection. We explore the characteristics of the different age and gender groups, identifying a number of distinctions. Through this, we train models based on user profiles and comments, via the ground truth of specially verified profiles. When applying our models for age and gender estimation to unverified profiles, 38% of profiles are classified as lying about their age, and 25% are predicted to be lying about their gender. The results suggest that women have a greater propensity to catfish than men. Our preliminary work has notable implications on operators of such online social networks, as well as users who may worry about interacting with catfishes.
We argue for network slicing as an efficient solution that addresses the diverse requirements of 5G mobile networks, thus providing the necessary flexibility and scalability associated with future network implementations. We elaborate on the challenges that emerge when we design 5G networks based on network slicing. We focus on the architectural aspects associated with the coexistence of dedicated as well as shared slices in the network. In particular, we analyze the realization options of a flexible radio access network with focus on network slicing and their impact on the design of 5G mobile networks. In addition to the technical study, this paper provides an investigation of the revenue potential of network slicing, where the applications that originate from such concept and the profit capabilities from the network operator's perspective are put forward.
The recently proposed Pocket Switched Network paradigm takes advantage of human social contacts to opportunistically create data paths over time. Our goal is to examine the effect of the human contact process on data delivery. We find that the contact occurrence distribution is highly uneven: contacts between a few node-pairs occur too frequently, leading to inadequate mixing in the network, while the majority of contacts are rare, and essential for connectivity. This distribution of contacts leads to a significant variation in performance over short time windows. We discover that the formation of a large clique core during the window is correlated with the fraction of data delivered, as well as the speed of delivery. We then show that the clustering co-efficient of the contact graph over a time window is a good predictor of performance during the window. Taken together, our findings suggest new directions for designing forwarding algorithms in ad-hoe or delay-tolerant networking schemes using humans as data mules.
TV White Spaces (TVWS) technology allows wireless devices to opportunistically use locally-available TV channels enabled by a geolocation database. The UK regulator Ofcom has initiated a pilot of TVWS technology in the UK. This paper concerns a large-scale series of trials under that pilot. The purposes are to test aspects of white space technology, including the white space device and geolocation database interactions, the validity of the channel availability/powers calculations by the database and associated interference effects on primary services, and the performances of the white space devices, among others. An additional key purpose is to perform research investigations such as on aggregation of TVWS resources with conventional resources and also aggregation solely within TVWS, secondary coexistence issues and means to mitigate such issues, and primary coexistence issues under challenging deployment geometries, among others. This paper provides an update on the trials, giving an overview of their objectives and characteristics, some aspects that have been covered, and some early results and observations.
The nature of information gathering and dissemination has changed dramatically over the past 20 years as traditional media sources are increasingly being replaced by a cacaphony of social media channels. Despite this, society still expects to disseminate its critical information via traditional news sources. Public Warning Systems (PWS) exist, but concerns about spamming users with irrelevant warnings mean that mostly only life threatening emergency warnings are delivered via PWS. We argue that it is time for society to upgrade its infrastructure for critical information services (CIS) and that a smartphone app system can provide a standardised, less-intrusive user interface to deliver CIS, especially if the traffic for the app is prioritised during congestion periods. Accordingly, we make three contributions in this paper. Firstly, using network parameters from our longitudinal measurements of network performance in Central London (an area of high user traffic), we show, with simulations, that reserving some bandwidth exclusively for CIS could assure QoS for CIS without significant degradation for other services. Secondly, we provide a conceptual design of a 999 CIS app, which can mimic the current 999 voice system and can be built using 3GPP defined systems. Thirdly, we identify the stakeholder relationships with industry partners and policymakers that can help to deliver a CIS system that is fit for purpose for an increasingly smartphone-based society.
Virtualization, containerization and softwarization technologies enable telecommunication systems to realize multitenancy, multi-network slicing and multi-level services. However, the use of these technologies to such ends requires a redesign of the telecommunications network architecture that goes beyond the current long term evolution-advanced (LTE-A). This paper proposes a novel hierarchical and distributed Virtualized Authentication, Authorization and Accounting (V-AAA) architecture for fifth-generation (5G) telecommunications systems, conceived to handle multi-tenancy, multi-network slicing and multi-level services. It also contemplates a new hierarchical and distributed database architecture to inter-work with our 5G V-AAA, able to cope with the network flexibility, elasticity and traffic fluctuation implied in 5G. The sum achievement is the design of a new approach that can provide fast billing and multiple network services for authentication and authorization at the edge cloud.
On-demand video accounts for the majority of wireless data traffic. Video distribution schemes based on caching combined with device-to-device (D2D) communications promise order-of-magnitude greater spectral efficiency for video delivery, but hinge on the principle of "concentrated demand distributions." This paper presents, for the first time, the analysis and evaluations of the throughput-outage tradeoff of such schemes based on measured cellular demand distributions. In particular, we use a dataset with more than 100 million requests from the BBC iPlayer, a popular video streaming service in the U.K., as the foundation of the analysis and evaluations. We present an achievable scaling law based on the practical popularity distribution, and show that such scaling law is identical to those reported in the literature. We find that also for the numerical evaluations based on a realistic setup, order-of-magnitude improvements can be achieved. Our results indicate that the benefits promised by the caching-based D2D in the literature could be retained for cellular networks in practice.
In the area of computer vision, deep learning techniques have recently been used to predict whether urban scenes are likely to be considered beautiful: it turns out that these techniques are able to make accurate predictions. Yet they fall short when it comes to generating actionable insights for urban design. To support urban interventions, one needs to go beyond predicting beauty, and tackle the challenge of recreating beauty. Unfortunately, deep learning techniques have not been designed with that challenge in mind. Given their 'black-box nature', these models cannot be directly used to explain why a particular urban scene is deemed to be beautiful. To partly fix that, we propose a deep learning framework (which we name FaceLift(1)) that is able to both beautify existing urban scenes (Google Street Views) and explain which urban elements make those transformed scenes beautiful. To quantitatively evaluate our framework, we cannot resort to any existing metric (as the research problem at hand has never been tackled before) and need to formulate new ones. These new metrics should ideally capture the presence (or absence) of elements that make urban spaces great. Upon a review of the urban planning literature, we identify five main metrics: walkability, green spaces, openness, landmarks and visual complexity. We find that, across all the five metrics, the beautified scenes meet the expectations set by the literature on what great spaces tend to be made of. This result is further confirmed by a 20-participant expert survey in which FaceLift has been found to be effective in promoting citizen participation. All this suggests that, in the future, as our framework's components are further researched and become better and more sophisticated, it is not hard to imagine technologies that will be able to accurately and efficiently support architects and planners in the design of the spaces we intuitively love.
In this paper, the break-lock phenomenon of phase locked loop (PLL) in missile borne monopulse radar receiver is presented. The continuous wave (CW) frequency modulated (FM) signal is used as jamming signal which is injected into the PLL along with the desired radar echo signal. The effects of key parameters in the FM CW jammer platform such as frequency sensitivity (k f ), modulating signal amplitude (v m ) and modulation frequency (f m ) on break-lock are reported. The value of k f at which the PLL loses the frequency lock to the radar echo signal as a function of modulating signal amplitude and modulation frequency is presented. It is shown that break-lock is achieved at 3.511×10 9 Hz/V for a typical modulating signal amplitude of 5 mV and modulation frequency of 200 kHz, when the radar echo amplitude at the PLL input is 1 volt. The break-lock is also studied by injecting radar echo signal with different amplitude at the PLL input and the value of k f required for break-lock is reported. From these results, the frequency deviation and modulation index required for break-lock are computed and conclusions are demonstrated. The PLL with a third order passive loop filter is designed by exact method and simulation is carried out using visual system simulator (VSS) AWR software for performance evaluation.
Disparate algorithms are being designed to decide certain basic questions in opportunistic networks. This position paper describes a nascent idea that aims to provide a single framework to answer such questions. Inspired by the concept of a generic knowledge plane, we propose to study whether the information embodied in folksonomies can be used to make network decisions in opportunistic networks.
The aim of this article is to provide an understanding of social networks as a useful addition to the standard toolbox of techniques used by system designers. To this end, we give examples of how data about social links have been collected and used in different application contexts. We develop a broad taxonomy-based overview of common properties of social networks, review how they might be used in different applications, and point out potential pitfalls where appropriate. We propose a framework, distinguishing between two main types of social network-based user selection-personalised user selection, which identifies target users who may be relevant for a given source node, using the social network around the source as a context, and generic user selection or group delimitation, which filters for a set of users who satisfy a set of application requirements based on their social properties. Using this framework, we survey applications of social networks in three typical kinds of application scenarios: recommender systems, content-sharing systems (e.g., P2P or video streaming), and systems that defend against users who abuse the system (e.g., spam or sybil attacks). In each case, we discuss potential directions for future research that involve using social network properties.
In this paper, we propose to leverage social graphs from Online Social Networks (OSN) to improve the forwarding efficiency of mobile networks, more particularly Delay Tolerant Networks (DTN). We extract community structures from three popular OSNs, Flickr, LiveJournal,and YouTube, and quantify the clustering features of each network at different levels of hierarchical resolution. We then show how community information can be used for forwarding using hints small enough to store on a mobile device. We also provide a first comparison study of the topological community structures for different types of OSNs with millions of users.
Caching of video files on user devices, combined with file exchange through device-to-device (D2D) communications is a promising method for increasing the throughput of wireless networks. Previous theoretical investigations showed that throughput can be increased by orders of magnitude, but assumed a Zipf distribution for modeling the popularity distribution, which was based on observations in wired networks. Thus the question whether cache-aided D2D video distribution can provide in practice the benefits promised by existing theoretical literature remains open. To answer this question, we provide new results specifically for popularity distributions of video requests of mobile users. Based on an extensive real-world dataset, we adopt a generalized distribution, known as Mandelbrot-Zipf (MZipf) distribution. We first show that this popularity distribution can fit the practical data well. Using this distribution, we analyze the throughput-outage tradeoff of the cache-aided D2D network and show that the scaling law is identical to the case of Zipf popularity distribution when the MZipf distribution is sufficiently skewed, implying that the benefits previously promised in the literature could indeed be realized in practice. To support the theory, practical evaluations using numerical experiments are provided, and show that the cache-aided D2D can outperform the conventional unicasting from base stations.
Service liability interconnections among globally networked IT- and IoT-driven service organizations create potential channels for cascading service disruptions worth billions of dollars, due to modern cyber-crimes such as DDoS, APT, and ransomware attacks. A natural question that arises in this context is: What is the likelihood of a cyber-blackout?, where the latter term is defined as the probability that all (or a major subset of) organizations in a service chain become dysfunctional in a certain manner due to a cyber-attack at some or all points in the chain. The answer to this question has major implications to risk management businesses such as cyber-insurance when it comes to designing policies by risk-averse insurers for providing coverage to clients in the aftermath of such catastrophic network events. In this article, we investigate this question in general as a function of service chain networks and different cyber-loss distribution types. We show somewhat surprisingly (and discuss the potential practical implications) that, following a cyber-attack, the effect of (a) a network interconnection topology and (b) a wide range of loss distributions on the probability of a cyber-blackout and the increase in total service-related monetary losses across all organizations are mostly very small. The primary rationale behind these results are attributed to degrees of heterogeneity in the revenue base among organizations and the Increasing Failure Rate property of popular (i.i.d/non-i.i.d) loss distributions, i.e., log-concave cyber-loss distributions. The result will enable risk-averse cyber-riskmanagers to safely infer the impact of cyber-attacks in a worst-case network and distribution oblivious setting.