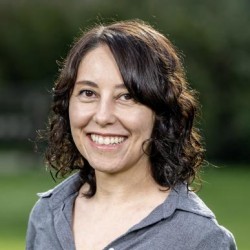
Dr Nimet Kaygusuz
Academic and research departments
Faculty of Engineering and Physical Sciences, Centre for Vision, Speech and Signal Processing (CVSSP).About
My research project
Vehicle State Estimation for Autonomous DrivingVehicle State Estimation for Autonomous Driving
Vehicle State Estimation for Autonomous Driving
Publications
Visual Odometry (VO) estimation is an important source of information for vehicle state estimation and autonomous driving. Recently, deep learning based approaches have begun to appear in the literature. However, in the context of driving, single sensor based approaches are often prone to failure because of degraded image quality due to environmental factors, camera placement, etc. To address this issue, we propose a deep sensor fusion framework which estimates vehicle motion using both pose and uncertainty estimations from multiple on-board cameras. We extract spatio-temporal feature representations from a set of consecutive images using a hybrid CNN-RNN model. We then utilise a Mixture Density Network (MDN) to estimate the 6-DoF pose as a mixture of distributions and a fusion module to estimate the final pose using MDN outputs from multi-cameras. We evaluate our approach on the publicly available, large scale autonomous vehicle dataset, nuScenes. The results show that the proposed fusion approach surpasses the state-of-the-art, and provides robust estimates and accurate trajectories compared to individual camera-based estimations.
Visual Odometry (VO) is used in many applications including robotics and autonomous systems. However, traditional approaches based on feature matching are computationally expensive and do not directly address failure cases, instead relying on heuristic methods to detect failure. In this work, we propose a deep learning-based VO model to efficiently estimate 6-DoF poses, as well as a confidence model for these estimates. We utilise a CNN-RNN hybrid model to learn feature representations from image sequences. We then employ a Mixture Density Network (MDN) which estimates camera motion as a mixture of Gaussians, based on the extracted spatio-temporal representations. Our model uses pose labels as a source of supervision, but derives uncertainties in an unsupervised manner. We evaluate the proposed model on the KITTI and nuScenes datasets and report extensive quantitative and qualitative results to analyse the performance of both pose and uncertainty estimation. Our experiments show that the proposed model exceeds state-of-the-art performance in addition to detecting failure cases using the predicted pose uncertainty.
Motion estimation approaches typically employ sensor fusion techniques, such as the Kalman Filter, to handle individual sensor failures. More recently, deep learning-based fusion approaches have been proposed, increasing the performance and requiring less model-specific implementations. However, current deep fusion approaches often assume that sensors are synchronised, which is not always practical, especially for low-cost hardware. To address this limitation, in this work, we propose AFT-VO, a novel transformer-based sensor fusion architecture to estimate VO from multiple sensors. Our framework combines predictions from asynchronous multi-view cameras and accounts for the time discrepancies of measurements coming from different sources. Our approach first employs a Mixture Density Network (MDN) to estimate the probability distributions of the 6-DoF poses for every camera in the system. Then a novel transformer-based fusion module, AFT-VO, is introduced, which combines these asynchronous pose estimations, along with their confidences. More specifically, we introduce Discretiser and Source Encoding techniques which enable the fusion of multi-source asynchronous signals. We evaluate our approach on the popular nuScenes and KITTI datasets. Our experiments demonstrate that multi-view fusion for VO estimation provides robust and accurate trajectories, outperforming the state of the art in both challenging weather and lighting conditions.