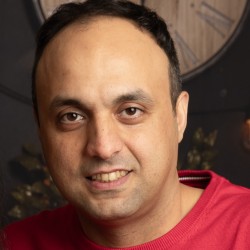
Dr Mohammad Shojafar
Academic and research departments
Institute for Communication Systems, Faculty of Engineering and Physical Sciences, 5G/6G Innovation Centre.About
Biography
Mohammad Shojafar (M'17-SM'19) is an Associate Professor in the Institute for Communication Systems (ICS) at the University of Surrey, United Kingdom. Before joining 5G/6GIC, he was a Marie Curie Individual Fellowship (MSCA-GF-IF) - named 'PRISENODE' at the University of Padua, Italy. Also, he was a Senior Researcher working with UofT and Toronto Metropolitan University on a network security project at TELUS Communications (TELUS), Toronto, Canada. Before that, he was a senior researcher at Italian universities, working on related network and security projects with Telecom Italia Mobile (TIM) for different EU and Italian projects.
Mohammad secured more than £1.9M (as a PI) in various local and international projects, such as 'ORAN-TWIN' (Lead, CHEDDAR HUB/EPSRC), '5G MODE' (UKTIN/DSIT), 'ONE4HDD' (UKTIN/DSIT), 'PRISENODE' (Lead, EU-MSCA-IF), 'TRACE-V2X' (EU-MSCA-SE), 'AUTOTRUST' (European Space Agency), 'Dynamic Response In O-RAN' (UK/DSTL), '6G-SMART' (EU-CELTIC-NEXT), and 'HiPer-RAN' (UKTIN/DSIT), 'D-XPERT' (Innovate UK), 'APTd5G' (UK-EPSRC-UKI-FNI), 'ESKMARALD' (UK-NCSC), 'FIoT4' (Ecuadorian-UK), etc. Mohammad participated in and contributed to some EU projects, such as SUPERFLUIDITY and TagItSmart. In addition, he contributed to some Italian projects like 'PRIN15', 'SAMMClouds', 'S2C', and 'V-FoG', aimed to address some of the open issues related to the SaaS/IaaS systems in Cloud and Fog networking.
He received a PhD in ICT from Sapienza University of Rome, Italy, in 2016 with an ’’Excellent’’ degree. He is an Intel Innovator, ACM Professional Member, Sustainability Fellow at the Institute for Sustainability, Fellow of the Higher Education Academy, and an IEEE Senior Member.
I am always searching for enthusiastic PhD and postdoc candidates in advanced 5G/Open-RAN/6G Security and Cloud/Fog/Edge Networking and Communications. Also, PDRA positions might be available for PhD holders. Interested candidates are encouraged to email me (m.shojafar@surrey.ac.uk) their CV and cover letter.
Areas of specialism
Affiliations and memberships
YouTube Channel
News
In the media
ResearchResearch interests
Research Interest
My research interest is 5G/5G-advanced, Open-RAN Security and Privacy like resource-constrained mobile devices (IoTs and smartphones) in Open-RAN; Cryptography algorithms, e.g., DLT and Blockchain applied in the 5G/5G-advanced network; Green Networking; Cloud and Fog Networks and their security and privacy issues; distributed and networked systems.
Some Research Topics
Privacy and Security in Open RAN
Open Radio Access Network (Open RAN) improves the flexibility and programmability of the 5G network by applying the Software-Defined Network (SDN) principles. O-RAN defines a near-real-time Radio Intelligent Controller (RIC) to decouple the RAN functionalities into the control and user planes. Although the current Open RAN security group offers several countermeasures against threats, RIC is still prone to attacks such as RIC spoofing, network misconfiguration, inconsistency in radio access policies, software vulnerabilities for x/rApps, intrusion attacks of traffic steering over O-DUs/O-CUs, etc. This problem will be complex when intelligent strategies have a pivot role in 5G advanced or 6G.
To mitigate the raised issues, we first studied some of these issues, such as [bearer context migration], and developed and tested real-time intelligent solutions [RLV] and [6G RAN]. More info (including our software, source code, and demo video) can be found [HERE].
Privacy and Security in Cloud/Fog Networking
Cloud Data Centers (CDCs) have become a key aspect of the Internet Information and Communication Technology (IICT) sector. CDCs are facing several issues like energy, electricity, carbon footprint, and some other environmental problems. Researchers often use several techniques to manage the energy inside and outside CDCs, e.g., temperature management technologies, virtualization, consolidations, VM/workload migrations, right-sizing, the use of some complex admission controls or new estimations of the incoming workloads, to avoid spikes or troughs in workload or SLA violations. The problem will be more problematic while the cloud service demands rise by raising mobile applications, leading to utilizing emerging technologies, especially Fog technology in the CDCs. In 2018, several issues were reported for CDCs, including security issues (i.e., switch and controller vulnerabilities and denial of service) and privacy issues (i.e., cloud data breaches, insufficient identity, credential, and access management, and cloud application account hijacking). These problems become more critical when the cloud traffic rate increases by increasing the number of cloud applications on mobile devices. Consequently, security and privacy have become more complicated in CDC assurance.
In this way, to mitigate the raised issues, we introduced the concept of [Fog Data Center] and [Networked Fog Centers]. We also implemented these concepts in various real datasets. We analyzed the CDC challenges, esp., tested on SDNs [with failure security issues] and [without failure security issues], [tested on WSNs], [mutually address cost/electricity of CDCs], tests on [distributed fog structure], and most recent one tested on [smart city network, Fog-IoT]. Recently, we designed a robust crypto mechanism over cloud storage which is tested in various real systems [LEVER]. More info (including our software, source code, and demo video) can be found [HERE].
IoT and Smartphone Security, Privacy, and Forensics
In the world, there is an average of almost one mobile telephone per human being (one for each inhabitant in developed countries and one for every two inhabitants in developing countries). Computational capabilities of mobile devices have increased significantly, and they are commonly used as personal devices to store private data. However, their specific characteristics (user mobility, storage of personal information, and communication features, among others) make the security and privacy of these devices particularly exposed.
Our primary contribution in this field has been the proposal of FeatureAnalytics, a new feature-based solution to cover the Android dataset available in [HERE]. Also, we propose a new adversarial machine learning-aware model to tackle data manipulation and analyze the Android datasets, which are which is available in [FGCS, NCAA] and DDoS attack/detection on IoT devices [RSS]. Recently, we designed a robust model using federate learning and adversarial machine learning techniques on IIoT, which is available in [FED-IIoT]. Also, we design a forward privacy mechanism verifying the correctness of IoT device search results, which is available at [HERE].
Applying Lightweight Cryptography Algorithms
Designing Authentication and Key Agreement (AKA) protocol is essential in several network and communication problems esp., WSN, and Vehicular/mobile networks. Applications of these networks can be found both in the military and local contexts (e.g., environmental monitoring). Due to their strict constraints (e.g., limited battery, computation, and communication), the design of secure and privacy-preserving encryption primitives like blockchain in vehicular/mobile networks is very challenging.
We proposed several fundamental lightweight cryptography protocols for privacy and security in global mobility networks in smart cities [GLOMONET]; Internet of drones to support anonymity, authentication, authorization, accountability [AAA-IoD]; Proactive AKA on IoT [PAKIT], Lightweight Authentication Protocol for IoT Devices [Light-Edge], and minimizing network and communications of eHealth using three-factor access controls [LACO].
For more info on our attack and solutions (including the source code and a demo video) see my GitHub in [my GitHub]
Research projects
European projects- European Commission, Marie Curie Fellowship Project ID: 839255, PRISENODE (PI) (275,209 euro)
- European Commission, H2020-ICT30-2015 Project ID: 688061, TagItSmart! 2016-2019 (Team member)
- European Commission, H2020-ICT-2014-2, Project ID: 671566, SUPERFLUIDITY, 2015-2018 (Team member)
Ecuadorian-British project- Implementation of Fog Computing and Network Architecture for Internet of Things and Industry 4.0, (Co-PI) (20,000 dollars) [March 2020-August 2021]
Spanish project- P18-RT-4046, Jaen University, optimization of energy sustainability in cloud computing centers through expert planning with analysis of interoperability, (95,000 euro) (External Collaborator) [July 2020-June. 2023]
Italian projects- University of Padua, Research Grant B Fellowship - Adaptive Failure and QoS-aware Controller over Cloud Data Center to Preserve Robustness and Integrity of the Incoming Traffic, 2018-2020 (52,500 euro+ 5k euro), (PI)
- Sapienza University of Rome, GAUChO - A Green Adaptive Fog Computing and Networking Architecture), 2017-2020 (Team member)
- Sapienza University of Rome, V-FoG - Vehicular Fog network, 2018-2019 (Team member)
- University of Modena and Reggio Emilia, S2C - Secure, Software-defined Cloud 2017-2018 (Team member)
- University of Modena and Reggio Emilia, SAMMClouds - SAMMClouds: Secure and Adaptive Management of Multi-Clouds, 2015-2016 (Team member)
- Sapienza University of Rome, WISECLOUD - Wireless Internet SEnsing CLOUD, 2014-2016 (Team member)
Industry projects- Huawei Technologies UK, Security and Privacy in Smart Grid and IIoT, (Co-PI) (150,000 GBP) - 1 PhD fellowship [October 2020-October 2023]
- Tidewater, Rahyab Rayaneh Gostar, GCOMS - General Cargo Operations Management System, Iran, 2008-2012 (Team member)
- Tidewater, Rahyab Rayaneh Gostar, TCTS - Terminal System, Iran 2008-2010 (Team member)
Research interests
Research Interest
My research interest is 5G/5G-advanced, Open-RAN Security and Privacy like resource-constrained mobile devices (IoTs and smartphones) in Open-RAN; Cryptography algorithms, e.g., DLT and Blockchain applied in the 5G/5G-advanced network; Green Networking; Cloud and Fog Networks and their security and privacy issues; distributed and networked systems.
Some Research Topics
Privacy and Security in Open RAN
Open Radio Access Network (Open RAN) improves the flexibility and programmability of the 5G network by applying the Software-Defined Network (SDN) principles. O-RAN defines a near-real-time Radio Intelligent Controller (RIC) to decouple the RAN functionalities into the control and user planes. Although the current Open RAN security group offers several countermeasures against threats, RIC is still prone to attacks such as RIC spoofing, network misconfiguration, inconsistency in radio access policies, software vulnerabilities for x/rApps, intrusion attacks of traffic steering over O-DUs/O-CUs, etc. This problem will be complex when intelligent strategies have a pivot role in 5G advanced or 6G.
To mitigate the raised issues, we first studied some of these issues, such as [bearer context migration], and developed and tested real-time intelligent solutions [RLV] and [6G RAN]. More info (including our software, source code, and demo video) can be found [HERE].
Privacy and Security in Cloud/Fog Networking
Cloud Data Centers (CDCs) have become a key aspect of the Internet Information and Communication Technology (IICT) sector. CDCs are facing several issues like energy, electricity, carbon footprint, and some other environmental problems. Researchers often use several techniques to manage the energy inside and outside CDCs, e.g., temperature management technologies, virtualization, consolidations, VM/workload migrations, right-sizing, the use of some complex admission controls or new estimations of the incoming workloads, to avoid spikes or troughs in workload or SLA violations. The problem will be more problematic while the cloud service demands rise by raising mobile applications, leading to utilizing emerging technologies, especially Fog technology in the CDCs. In 2018, several issues were reported for CDCs, including security issues (i.e., switch and controller vulnerabilities and denial of service) and privacy issues (i.e., cloud data breaches, insufficient identity, credential, and access management, and cloud application account hijacking). These problems become more critical when the cloud traffic rate increases by increasing the number of cloud applications on mobile devices. Consequently, security and privacy have become more complicated in CDC assurance.
In this way, to mitigate the raised issues, we introduced the concept of [Fog Data Center] and [Networked Fog Centers]. We also implemented these concepts in various real datasets. We analyzed the CDC challenges, esp., tested on SDNs [with failure security issues] and [without failure security issues], [tested on WSNs], [mutually address cost/electricity of CDCs], tests on [distributed fog structure], and most recent one tested on [smart city network, Fog-IoT]. Recently, we designed a robust crypto mechanism over cloud storage which is tested in various real systems [LEVER]. More info (including our software, source code, and demo video) can be found [HERE].
IoT and Smartphone Security, Privacy, and Forensics
In the world, there is an average of almost one mobile telephone per human being (one for each inhabitant in developed countries and one for every two inhabitants in developing countries). Computational capabilities of mobile devices have increased significantly, and they are commonly used as personal devices to store private data. However, their specific characteristics (user mobility, storage of personal information, and communication features, among others) make the security and privacy of these devices particularly exposed.
Our primary contribution in this field has been the proposal of FeatureAnalytics, a new feature-based solution to cover the Android dataset available in [HERE]. Also, we propose a new adversarial machine learning-aware model to tackle data manipulation and analyze the Android datasets, which are which is available in [FGCS, NCAA] and DDoS attack/detection on IoT devices [RSS]. Recently, we designed a robust model using federate learning and adversarial machine learning techniques on IIoT, which is available in [FED-IIoT]. Also, we design a forward privacy mechanism verifying the correctness of IoT device search results, which is available at [HERE].
Applying Lightweight Cryptography Algorithms
Designing Authentication and Key Agreement (AKA) protocol is essential in several network and communication problems esp., WSN, and Vehicular/mobile networks. Applications of these networks can be found both in the military and local contexts (e.g., environmental monitoring). Due to their strict constraints (e.g., limited battery, computation, and communication), the design of secure and privacy-preserving encryption primitives like blockchain in vehicular/mobile networks is very challenging.
We proposed several fundamental lightweight cryptography protocols for privacy and security in global mobility networks in smart cities [GLOMONET]; Internet of drones to support anonymity, authentication, authorization, accountability [AAA-IoD]; Proactive AKA on IoT [PAKIT], Lightweight Authentication Protocol for IoT Devices [Light-Edge], and minimizing network and communications of eHealth using three-factor access controls [LACO].
For more info on our attack and solutions (including the source code and a demo video) see my GitHub in [my GitHub]
Research projects
- European Commission, Marie Curie Fellowship Project ID: 839255, PRISENODE (PI) (275,209 euro)
- European Commission, H2020-ICT30-2015 Project ID: 688061, TagItSmart! 2016-2019 (Team member)
- European Commission, H2020-ICT-2014-2, Project ID: 671566, SUPERFLUIDITY, 2015-2018 (Team member)
- Implementation of Fog Computing and Network Architecture for Internet of Things and Industry 4.0, (Co-PI) (20,000 dollars) [March 2020-August 2021]
- P18-RT-4046, Jaen University, optimization of energy sustainability in cloud computing centers through expert planning with analysis of interoperability, (95,000 euro) (External Collaborator) [July 2020-June. 2023]
- University of Padua, Research Grant B Fellowship - Adaptive Failure and QoS-aware Controller over Cloud Data Center to Preserve Robustness and Integrity of the Incoming Traffic, 2018-2020 (52,500 euro+ 5k euro), (PI)
- Sapienza University of Rome, GAUChO - A Green Adaptive Fog Computing and Networking Architecture), 2017-2020 (Team member)
- Sapienza University of Rome, V-FoG - Vehicular Fog network, 2018-2019 (Team member)
- University of Modena and Reggio Emilia, S2C - Secure, Software-defined Cloud 2017-2018 (Team member)
- University of Modena and Reggio Emilia, SAMMClouds - SAMMClouds: Secure and Adaptive Management of Multi-Clouds, 2015-2016 (Team member)
- Sapienza University of Rome, WISECLOUD - Wireless Internet SEnsing CLOUD, 2014-2016 (Team member)
- Huawei Technologies UK, Security and Privacy in Smart Grid and IIoT, (Co-PI) (150,000 GBP) - 1 PhD fellowship [October 2020-October 2023]
- Tidewater, Rahyab Rayaneh Gostar, GCOMS - General Cargo Operations Management System, Iran, 2008-2012 (Team member)
- Tidewater, Rahyab Rayaneh Gostar, TCTS - Terminal System, Iran 2008-2010 (Team member)
Supervision
Postgraduate research supervision
Current (ongoing)
- Sotiris Chatzimiltis - University of Surrey, UK (PhD, expected 2025)
- Emmanuel Amachaghi - University of Surrey, UK (P/T PhD, expected December 2024)
- Krupal Byalaiah - University of Surrey, UK (P/T PhD, expected 2028)
Past (graduated)
- Sanaz Soltani, University of Surrey, UK (PhD, August 2024)
- Esmaeil Amiri, University of Surrey, UK (PhD, October 2023)
- Parya HajiMirzaee, University of Surrey, UK (PhD, September 2022)
- Rekha Mundackal Das, University of Surrey, UK (PhD, Jan 2023)
- Sotiris Chatzimiltis, University of Surrey, UK (MSc, September 2022)
- Shabnam Fathima Basheer - University of Surrey, UK (MSc, September 2023)
- Mona Akbari, University of Surrey, UK (MSc, September 2023)
- Neha Gupta, University of Surrey, UK (MSc, September 2022)
- Roshan Baby, University of Surrey, UK (MSc, September 2022)
- Cewen Yang, University of Surrey, UK (MSc, September 2022)
- Bhavitha Tukivakam, University of Surrey, UK (MSc, September 2022)
- Lingyi Zhang, University of Surrey, UK (MSc, September 2021)
- Hingo Chan, University of Surrey, UK (MSc, September 2021)
Teaching
2024/2025
- EEEM048 - INTERNET OF THINGS, University of Surrey, UK (MSc level) - Module leader
- EEEM048 - INTERNET OF THINGS (Short Course), University of Surrey, UK (MSc level) - Module leader
- EEE2036 - LABORATORIES, DESIGN & PROFESSIONAL STUDIES III, University of Surrey, UK (BSc level)
- COM2022 - COMPUTER NETWORKING, University of Surrey, UK (Bsc level) - Module leader
2023/2024
- EEEM048 - INTERNET OF THINGS, University of Surrey, UK (MSc level) - Module leader
- EEEM048 - INTERNET OF THINGS (Short Course), University of Surrey, UK (MSc level) - Module leader
- EEE2036 - LABORATORIES, DESIGN & PROFESSIONAL STUDIES III, University of Surrey, UK (Bsc level) - Module LAB leader
- EEE2040 - COMMUNICATIONS & NETWORKS, University of Surrey, UK (BSc level)
2022/2023
- EEEM048 - INTERNET OF THINGS, University of Surrey, UK (MSc level) - Module leader
- EEEM048 - INTERNET OF THINGS (Short Course), University of Surrey, UK (MSc level) - Module leader
- EEE2036 - LABORATORIES, DESIGN & PROFESSIONAL STUDIES III, University of Surrey, UK (Bsc level) - Module LAB leader
- EEE2040 - COMMUNICATIONS & NETWORKS, University of Surrey, UK (BSc level)
2021/2022
- EEEM048 - INTERNET OF THINGS, University of Surrey, UK (MSc level) - Module leader
- EEEM048 - INTERNET OF THINGS (Short Course), University of Surrey, UK (MSc level) - Module leader
- EEE2036 - LABORATORIES, DESIGN & PROFESSIONAL STUDIES III, University of Surrey, UK (Bsc level) - Module LAB leader
- EEE2040 - COMMUNICATIONS & NETWORKS, University of Surrey, UK (BSc level)
2020/2021
- EEEM048 - INTERNET OF THINGS, University of Surrey, UK (MSc level) - Module leader
- EEE2036 - LABORATORIES, DESIGN & PROFESSIONAL STUDIES III, University of Surrey, UK (BSc level)
2019/2020
- EEE2040 - COMMUNICATIONS & NETWORKS, University of Surrey, UK (BSc level)
- EEE2036 - LABORATORIES, DESIGN & PROFESSIONAL STUDIES III, University of Surrey, UK (BSc level)
Publications
Highlights
M. Shojafar, N. Cordeschi, E. Baccarelli, "Energy-efficient Adaptive Resource Management for Real-time Vehicular Cloud Services", IEEE Transactions on Cloud Computing, (TCC), Vol. 7, Iss. 1, pp. 196-209, March 2019.
R. Taheri, M. Shojafar, M. Alazab, R. Tafazolli, "FED-IIoT: A Robust Federated Malware Detection Architecture in Industrial IoT", IEEE Transactions on Industrial Informatics, (TII), Vol. PP, Iss. 99, pp. 1-11, December 2020.
A. Kose, H. Lee, Ch. H. Foh, M. Shojafar, "Multi-Agent Context Learning Strategy for Interference-Aware Beam Allocation in mmWave Vehicular Communications", IEEE Transactions on Intelligent Transportation Systems (T-ITS), Impact Factor: 7.9, Vol. 25, Issue 7, pp. 7477-7493, July 2024.
— This paper addresses the challenge of fault analysis in the Vehicular Energy Network (VEN) caused by small fault samples due to transient faults and complex disturbances. The proposed generation and diagnosis networks (GDNs) are developed without necessitating prior knowledge or manual intervention. The approach starts with an encoding and diagnosis network that converts multi-dimensional signals into images through supervised learning. A sample enhancement network, improved with module transfer and a relaxation objective function , is then proposed to increase the reliability of convergence and diversity of features for small fault samples. Additionally, a joint iterative training strategy between these two networks improves diagnostic accuracy and generalization through feature feedback. Performance validation on a semi-physical simulation platform demonstrates that the proposed GDNs achieve a 20% improvement in diagnostic accuracy with small datasets (200 samples) and maintain superior performance as sample volume grows. Thus, the proposed approach offers a potent solution for fault diagnosis in VENs with scarce samples, enhancing the analysis of complex systems. Note to Practitioners—This paper delves into fault diagnosis in the vehicular energy network (VEN) using small samples, employing a data-driven and deep learning model. The proposed method is versatile, suitable for analyzing complex systems with multiple and heterogeneous signals. An end-to-end model, named generation and diagnosis networks (GDNs), is introduced for generating small samples and conducting fault diagnosis without requiring prior knowledge or manual input. This method encodes multiple signals into signal images, which are then processed by a specially designed sample enhancement model, improved through the relaxation objective function and module transfer method. The enhanced samples are utilized for accurate analysis within the encoding and diagnosis networks' diagnostic unit. The paper also provides a comparison of diagnosis results for reference. This approach enables researchers and engineers to efficiently augment and analyze small samples in practical applications, offering a practical framework for junior and inexperienced analysts. Preliminary experiments conducted using the RT-Lab semi-physical simulation platform suggest the method's feasibility and effectiveness. Future research will explore the optimization of the model's topology and parameters for lightweight design.
This paper proposes an edge server deployment strategy based on multi-agent reinforcement learning (CKM-MAPPO) to address the multi-objective optimization problem in a vehicle networking environment. CKM-MAPPO focuses on optimizing the load balancing among edge servers and minimizing edge servers’ delay and energy consumption. Firstly, the Canopy and K-means algorithms determine the edge server deployment’s number and initial location. Then, we utilize the multi-agent reinforcement learning algorithm to decide the optimal deployment location of the edge server. We performed a series of tests, and the experimental results show that CKM-MAPPO improves load balancing by 26.5% and reduces delay and energy consumption by 12.4% and 17.9%, respectively.
Software-Defined Networking (SDN) has found applications in different domains, including wired- and wireless networks. The SDN controller has a global view of the network topology, which is vulnerable to topology poisoning attacks, e.g., link fabrication and host-location hijacking. The adversaries can leverage these attacks to monitor the flows or drop them. However, current defence systems such as TopoGuard and TopoGuard+ can detect such attacks. In this paper, we introduce the Link Latency Attack (LLA) that can successfully bypass the systems' defence mechanisms above. In LLA, the adversary can add a fake link into the network and corrupt the controller's view from the network topology. This can be accomplished by compromising the end hosts without the need to attack the SDN-enabled switches. We develop a Machine Learning-based Link Guard (MLLG) system to provide the required defence for LLA. We test the performance of our system using an emulated network on Mininet, and the obtained results show an accuracy of 98.22% in detecting the attack. Interestingly, MLLG improves 16% the accuracy of TopoGuard+.
Fifth generation mobile networks (5G) leverage the power of edge computing to move vital services closer to end users. With critical 5G core network components located at the edge there is a need for detecting malicious signalling traffic to mitigate potential signalling attacks between the distributed Network Functions (NFs). A prerequisite for detecting anomalous signalling is a network traffic dataset for the identification and classification of normal traffic profiles. To this end, we utilise a 5G Core Network (5GC) simulator to execute test scenarios for different 5G procedures and use the captured network traffic to generate a dataset of normalised service interactions in the form of packet captures. We then apply machine learning techniques (supervised learning) and do a comparative analysis on accuracy, which uses three features from the traffic meta-data. Our results show that the identification of 5G service use by applying ML techniques offer a viable solution to classifying normal services from network traffic metadata alone. This has potential advantages in forecasting service demand for resource allocation in the dynamic 5GC environment and provide a baseline for performing anomaly detection of NF communication for detecting malicious traffic within the 5G Service Based Architecture (SBA).
Recent years have witnessed video streaming demands evolve into one of the most popular Internet applications. With the ever-increasing personalized demands for highdefinition and low-latency video streaming services, networkassisted video streaming schemes employing modern networking paradigms have become a promising complementary solution in the HTTP Adaptive Streaming (HAS) context. The emergence of such techniques addresses long-standing challenges of enhancing users' Quality of Experience (QoE), end-to-end (E2E) latency, as well as network utilization. However, designing a cost-effective, scalable, and flexible network-assisted video streaming architecture that supports the aforementioned requirements for live streaming services is still an open challenge. This article leverages novel networking paradigms, i.e., edge computing and Network Function Virtualization (NFV), and promising video solutions, i.e., HAS, Video Super-Resolution (SR), and Distributed Video Transcoding (TR), to introduce A Latency-and cost-aware hybrId P2P-CDN framework for liVe video strEaming (ALIVE). We first introduce the ALIVE multi-layer architecture and design an action tree that considers all feasible resources (i.e., storage, computation, and bandwidth) provided by peers, edge, and CDN servers for serving peer requests with acceptable latency and quality. We then formulate the problem as a Mixed Integer Linear Programming (MILP) optimization model executed at the edge of the network. To alleviate the optimization model's high time complexity, we propose a lightweight heuristic, namely, Greedy-Based Algorithm (GBA). Finally, we (i) design and instantiate a large-scale cloud-based testbed including 350 HAS players, (ii) deploy ALIVE on it, and (iii) conduct a series of experiments to evaluate the performance of ALIVE in various scenarios. Experimental results indicate that ALIVE (i) improves the users' QoE by at least 22%, (ii) decreases incurred cost of the streaming service provider by at least 34%, (iii) shortens clients' serving latency by at least 40%, (iv) enhances edge server energy consumption by at least 31%, and (v) reduces backhaul bandwidth usage by at least 24% compared to baseline approaches.
By harnessing Graphics Processing Unit (GPU), Field-programmable Gate Array (FPGA), and advanced cracking techniques, the success rates of server-side threats on passwords have reached unprecedented levels. Honeywords, also known as decoy passwords, have emerged as a promising detection strategy against this threat scenario. However, existing work falls short in creating a trap to counter targeted guessing attackers (\mathbf{TgA}) who exploit users' personal information to bypass honeyword-based traps. In this paper, we introduce two fundamental honeyword generation modules, namely NSecure-modifiedUI and ESecure-modifiedUI. Building upon these primary modules, we propose a hybrid honeyword-based strategy named NESec, which significantly enhances the ability to detect \mathbf{TgA} 's activities. A comparative analysis showcases the usability advantages and security benefits of the proposed NESec approach.
Millimeter wave (mmWave) has been recognized as one of key technologies for 5G and beyond networks due to its potential to enhance channel bandwidth and network capacity. The use of mmWave for various applications including vehicular communications has been extensively discussed. However, applying mmWave to vehicular communications faces challenges of high mobility nodes and narrow coverage along the mmWave beams. Due to high mobility in dense networks, overlapping beams can cause strong interference which leads to performance degradation. As a remedy, beam switching capability in mmWave can be utilized. Then, frequent beam switching and cell change become inevitable to manage interference, which increase computational and signalling complexity. In order to deal with the complexity in interference control, we develop a new strategy called Multi-Agent Context Learning (MACOL), which utilizes Contextual Bandit to manage interference while allocating mmWave beams to serve vehicles in the network. Our approach demonstrates that by leveraging knowledge of neighbouring beam status, the machine learning agent can identify and avoid potential interfering transmissions to other ongoing transmissions. Furthermore, we show that even under heavy traffic loads, our proposed MACOL strategy is able to maintain low interference levels at around 10%.
—This paper exploits an intelligent reflecting surface (IRS) assisted wireless powered mobile edge computing and caching (WP-MECC) network. In particular, an IRS is utilized to reflect energy signals from a power station (PS) to various IoT devices for energy harvesting during uplink wireless energy transfer (WET). These devices collect energy to support their own partially local computing for computational tasks and their offloading capabilities to an access point (AP), with the help of IRS via time or frequency division multiple access (TDMA or FDMA). The AP is equipped with a local cache connected with a MEC server via a backhaul link, which prefetches the data to facilitate edge computing capabilities. The maximization of a utility function is formulated to evaluate the overall network performance, which is defined as the difference between the sum of computational bits (offloading bits and local computing bits) and total backhaul cost. Due to multiple coupled variables, we first design the optimal caching strategy. Then, an auxiliary vector is introduced to coordinate the energy consumption of local computing and offloading, where its optimal solution can be achieved by an exhaustive search. Moreover, we utilize the Lagrange dual method and the Karush-Kuhn-Tucker (KKT) conditions to derive the optimal time scheduling for the TDMA scheme or the optimal bandwidth allocation for the FDMA counterpart in closed form. The IRS phase shifts are iteratively designed by employing the quadratic transformation (QT) and the Riemannian Manifold Optimization (RMO). Finally, simulation results are demonstrated to validate the network utility performance and confirm the advantage of the employment of IRS, the optimal IRS phase shift design and caching strategy, in comparison to the benchmark schemes. Index Terms—Intelligent reflecting surface (IRS), wireless powered mobile edge computing and caching (WP-MECC), utility
Software-Defined Networking (SDN) has found applications in different domains, including wired- and wireless networks. The SDN controller has a global view of the network topology, which is vulnerable to topology poisoning attacks, e.g., link fabrication and host-location hijacking. The adversaries can leverage these attacks to monitor the flows or drop them. However, current defence systems such as TopoGuard and TopoGuard+ can detect such attacks. In this paper, we introduce the Link Latency Attack (LLA) that can successfully bypass the systems' defence mechanisms above. In LLA, the adversary can add a fake link into the network and corrupt the controller's view from the network topology. This can be accomplished by compromising the end hosts without the need to attack the SDN-enabled switches. We develop a Machine Learning-based Link Guard (MLLG) system to provide the required defence for LLA. We test the performance of our system using an emulated network on Mininet, and the obtained results show an accuracy of 98.22% in detecting the attack. Interestingly, MLLG improves 16% the accuracy of TopoGuard+.
IoT-enabled smart healthcare systems has the characteristics of heterogeneous fusion, cross domain, collaborative autonomy, dynamic change and open interconnection, but they bring huge challenges in privacy issues. We proposed a scheme of forward privacy preserving for IoT-enabled healthcare systems, which mainly includes a searchable encryption scheme to achieve privacy preserving and searchable function. Our scheme uses trapdoor permutation to change the status counter, and it makes the adversary difficult to determine the valid status counter of inserted record with only the public key of the client. Our mechanism can solve the problem of verifying the correctness of the search results in the top-k search scenario with only part of the search results. The formal security analysis proves that our scheme achieves forward privacy preservation which can guarantee the privacy of healthcare data. Besides, performance evaluation shows our scheme are efficient and secure to preserve privacy of IoT-enabled healthcare systems.
This paper designs an efficient distributed intrusion detection system (DIDS) for Internet of Things (IoT) data traffic. The proposed DIDS has been implemented at IoT network gateways and edge sites to detect and alarm on anomalous traffic data. We implement different machine learning (ML) algorithms to classify the traffic as benign or malicious. We perform an in-depth parametric study of the models using multiple real-time IoT datasets to enable the model deployment to be consistent with the demands of the specific IoT network. Specifically, we develop a decentralized method using federated learning (FL) for collecting data from IoT sensor nodes to address the data privacy issues associated with centralizing data at the gateway DIDS. We propose two poisoning attacks on the perception layer of these IoT networks that use generative adversarial networks (GAN) to determine how the threats of unpredictable authenticity of the IoT sensors can be triggered. To address such attacks, we design an appropriate defence algorithm that is implemented at the gateways to help separate anomalous from benign data and preserve the system's robustness. The suggested defence algorithm successfully classifies anomalies with high accuracy, exhibiting the system's immunity against poisoning attacks. We confirm that the Random Forest classifier performs the best across all ML key performance indicators (KPIs) and can be implemented at the edge to reduce false alarm rates.
Modern network applications demand low-latency traffic engineering in the presence of network failure, while preserving the quality of service constraints like delay and capacity. Fast Re-Route (FRR) mechanisms are widely used for traffic re-routing purposes in failure scenarios. Control plane FRR typically computes the backup forwarding rules to detour the traffic in the data plane when the failure occurs. This mechanism could be computed in the data plane with the emergence of programmable data planes. In this paper, we propose a system (called TEL) that contains two FRR mechanisms, namely, and . The first one computes backup forwarding rules in the control plane, satisfying max-min fair allocation. The second mechanism provides FRR in the data plane. Both algorithms require minimal memory on programmable data planes and are well-suited with modern line rate match-action forwarding architectures (e.g., PISA). We implement both mechanisms on P4 programmable software switches (e.g., BMv2 and Tofino) and measure their performance on various topologies. The obtained results from a datacenter topology show that our FRR mechanism can improve the flow completion time up to 4.6xb-7.3x (i.e., small flows) and 3.1x-12x (i.e., large flows) compared to recirculation-based mechanisms, such as F10, respectively.
Most devices in the Internet of Things (IoT) work on unsafe networks and are constrained by limited computing, power, and storage resources. Since the existing centralized signature schemes cannot address the challenges to security and efficiency in IoT identification, this article proposes IdenMultiSig, a decentralized multi-signature protocol that combines identity-based signature (IBS) with Schnorr scheme under discrete logarithms on elliptic curves. First, to solve the problem of offline or faulty devices under unstable networks, we introduce a novel improvement of the existing Schnorr scheme by introducing a threshold Merkle tree for the verification with only m valid signatures among n participants ( m – n tree), while hiding the real identity to protect the data security and privacy of IoT nodes. Furthermore, to prevent dishonest or malicious behavior of the private key generator (PKG), a consortium blockchain is innovatively applied to replace the traditional PKG as a decentralized and trusted private key issuer. Finally, the proposed scheme is proven to be unforgeable against forgery signature attacks in the random oracle model (ROM) under the elliptic curve discrete logarithm (ECDL) assumption. Theoretical analysis and experimental results show that our scheme matches or outperforms existing research studies in privacy protection, offline device support, decentralized PKG, and provable security.
Time-reversal prefiltering (TRP) technique for impulse radio (IR) ultra wide-band (UWB) systems requires a large amount of feedback to transmit the channel impulse response from the receiver to the transmitter. In this paper, we propose a new feedback design based on vector quantization. We use a machine learning algorithm to cluster the estimated channels into several groups and to select the channel cluster heads (CCHs) for feedback. In particular, CCHs and their labels are recorded at both side of the UWB transceivers and the label of the most similar CCH to the estimated channel is fed back to the transmitter. Finally, the TRP is applied using the feedback CCH. The proposed digital feedback provides three main advantages: (1) it significantly reduces the dedicated bandwidth required for feedback; (2) it considerably improves the speed of transceivers; and, (3) it is robust to noise in the feedback channel since few bytes are required to send the codes that can be heavily error protected. Numerical results on standard UWB channel models are discussed, showing the advantage of the proposed solution.
Open vehicle routing problem (OVRP) is one of the most important problems in vehicle routing, which has attracted great interest in several recent applications in industries. The purpose in solving the OVRP is to decrease the number of vehicles and to reduce travel distance and time of the vehicles. In this article, a new meta-heuristic algorithm called OVRP_ICA is presented for the above-mentioned problem. This is a kind of combinatorial optimization problem that can use a homogeneous fleet of vehicles that do not necessarily return to the initial depot to solve the problem of offering services to a set of customers exploiting the imperialist competitive algorithm. OVRP_ICA is compared with some well-known state-of-the-art algorithms and the results confirmed that it has high efficiency in solving the above-mentioned problem.
Due to the growing interest for multimedia contents by mobile users, designing bandwidth and delay-efficient distributed algorithms for data searching over wireless (possibly, mobile) “ad hoc” Peer-to-Peer (P2P) content Delivery Networks (CDNs) is a topic of current interest. This is mainly due to the limited computing-plus-communication resources featuring state-of-the-art wireless P2P CDNs. In principle, an effective means to cope with this limitation is to empower traditional P2P CDNs by distributed Fog nodes. Motivated by this consideration, the goal of this paper is twofold. First, we propose and describe the main building blocks of a hybrid (e.g., mixed infrastructure and “ad hoc”) Fog-supported P2P architecture for wireless content delivery, namely, the Fog-Caching P2P architecture. It exploits the topological (possibly, time varying) information locally available at the serving Fog nodes, in order to speed up the data searching operations performed by the served peers. Second, we propose a bandwidth and delay-efficient, distributed and adaptive probabilistic search algorithm, that relies on the learning automata paradigm, e.g., the Fog-supported Learning Automata Adaptive Probabilistic Search (FLAPS) algorithm. The main feature of the FLAPS algorithm is the exploitation of the local topology information provided by the serving Fog nodes and the current status of the collaborating peers, in order to run a suitably distributed reinforcement algorithm for the adaptive discovery of peer-to-peer and peer-to-fog minimum-hop routes. The performance of the proposed FLAPS algorithm is numerically evaluated in terms of Success Rate, Hit-per-Query, Message-per-Query, Response Delay and Message Duplication Factor over a number of randomly generated benchmark CDN topologies. Furthermore, in order to corroborate the actual effectiveness of the FLAPS algorithm, extensive performance comparisons are carried out with some state-of-the-art searching algorithms, namely the Adaptive Probabilistic Search, Improved Adaptive Probabilistic Search and the Random Walk algorithms.
Wireless Body Area Network (WBAN) is a new trend in the technology that provides remote mechanism to monitor and collect patient’s health record data using wearable sensors. It is widely recognized that a high level of system security and privacy play a key role in protecting these data when being used by the healthcare professionals and during storage to ensure that patient’s records are kept safe from intruder’s danger. It is therefore of great interest to discuss security and privacy issues in WBANs. In this paper, we reviewed WBAN communication architecture, security and privacy requirements and security threats and the primary challenges in WBANs to these systems based on the latest standards and publications. This paper also covers the state-of-art security measures and research in WBAN. Finally, open areas for future research and enhancements are explored.
Big data stream mobile computing is proposed as a paradigm that relies on the convergence of broadband Internet mobile networking and real-time mobile cloud computing. It aims at fostering the rise of novel self-configuring integrated computing-communication platforms for enabling in real time the offloading and processing of big data streams acquired by resource-limited mobile/wireless devices. This position article formalizes this paradigm, discusses its most significant application opportunities, and outlines the major challenges in performing real-time energy-efficient management of the distributed resources available at both mobile devices and Internet-connected data centers. The performance analysis of a small-scale prototype is also included in order to provide insight into the energy vs. performance tradeoff that is achievable through the optimized design of the resource management modules. Performance comparisons with some state-of-the-art resource managers corroborate the discussion. Hints for future research directions conclude the article.
•A study on convergence of Blockchain-AI for sustainable smart city.•Presents the security issues and challenges based on various dimensions.•Discusses the blockchain security enhancement solutions, and summarizing key points.•Summarize the open issues and research direction: new security suggestions, future guidelines. In the digital era, the smart city can become an intelligent society by utilizing advances in emerging technologies. Specifically, the rapid adoption of blockchain technology has led a paradigm shift to a new digital smart city ecosystem. A broad spectrum of blockchain applications promise solutions for problems in areas ranging from risk management and financial services to cryptocurrency, and from the Internet of Things (IoT) to public and social services. Furthermore, the convergence of Artificial Intelligence (AI) and blockchain technology is revolutionizing the smart city network architecture to build sustainable ecosystems. However, these advancements in technologies bring both opportunities and challenges when it comes to achieving the goals of creating a sustainable smart cities. This paper provides a comprehensive literature review of the security issues and problems that impact the deployment of blockchain systems in smart cities. This work presents a detailed discussion of several key factors for the convergence of Blockchain and AI technologies that will help form a sustainable smart society. We discuss blockchain security enhancement solutions, summarizing the key points that can be used for developing various blockchain-AI based intelligent transportation systems. Also, we discuss the issues that remain open and our future research direction, this includes new security suggestions and future guidelines for a sustainable smart city ecosystem.
A clear trend in the evolution of network-based services is the ever-increasing amount of multimedia data involved. This trend towards big-data multimedia processing finds its natural placement together with the adoption of the cloud computing paradigm, that seems the best solution to cope with the demands of a highly fluctuating workload that characterizes this type of services. However, as cloud data centers become more and more powerful, energy consumption becomes a major challenge both for environmental concerns and for economic reasons. An effective approach to improve energy efficiency in cloud data centers is to rely on traffic engineering techniques to dynamically adapt the number of active servers to the current workload. Towards this aim, we propose a joint computing-plus-communication optimization framework exploiting virtualization technologies, called MMGreen . Our proposal specifically addresses the typical scenario of multimedia data processing with computationally intensive tasks and exchange of a big volume of data. The proposed framework not only ensures users the Quality of Service (through Service Level Agreements), but also achieves maximum energy saving and attains green cloud computing goals in a fully distributed fashion by utilizing the DVFS-based CPU frequencies. To evaluate the actual effectiveness of the proposed framework, we conduct experiments with MMGreen under real-world and synthetic workload traces. The results of the experiments show that MMGreen may significantly reduce the energy cost for computing, communication and reconfiguration with respect to the previous resource provisioning strategies, respecting the SLA constraints.
The fifth-generation (5G) mobile communication technology with higher capacity and data rate, ultra-low device to device (D2D) latency, and massive device connectivity will greatly promote the development of vehicular ad hoc networks (VANETs). Meantime, new challenges such as security, privacy and efficiency are raised. In this article, a hybrid D2D message authentication (HDMA) scheme is proposed for 5G-enabled VANETs, in which a novel group signature-based algorithm is used for mutual authentication between vehicle to vehicle (V2V) communication. In addition, a pre-computed lookup table is adopted to reduce the computation overhead of modular exponentiation operation. Security analysis shows that HDMA is robust to resist various security attacks, and performance analysis also points out that, the authentication overhead of HDMA is more efficient than some traditional schemes with the help of the pre-computed lookup table in V2V and vehicle to infrastructure (V2I) communication.
Smart city vision brings emerging heterogeneous communication technologies such as Fog Computing (FC) together to substantially reduce the latency and energy consumption of Internet of Everything (IoE) devices running various applications. The key feature that distinguishes the FC paradigm for smart cities is that it spreads communication and computing resources over the wired/wireless access network (e.g., proximate access points and base stations) to provide resource augmentation (e.g., cyberforaging) for resource- and energy-limited wired/wireless (possibly mobile) things. Motivated by these considerations, this paper presents a Fog-supported smart city network architecture called Fog Computing Architecture Network (FOCAN), a multi-tier structure in which the applications are running on things thatjointly compute, route, and communicate with one another through the smart city environment. FOCAN decreases latency and improves energy provisioning and the efficiency of services among things with different capabilities. In particular, three types of communications are defined between FOCAN devices – interprimary, primary, and secondary communication –to manage applications in a way that meets the quality of service standards for the Internet of Everything. One of the main advantages of the proposed architecture is that the devices can provide the services with low energy usage and in an efficient manner. Simulation results for a selected case study demonstrate the tremendous impact of the FOCAN energy-efficient solution on the communication performance of various types of things in smart cities. •Present a generalized multi-tiered smart city architecture utilizes FC for devices.•Develop an FC-supported resource allocation model to cover FNs/device components.•Provide various types of communications between the components.•Evaluate the performance of the solution for an FC platform on real datasets.
The use of the Internet of Things (IoT) in the electronic health (e-health) management systems brings with it many challenges, including secure communications through insecure radio channels, authentication and key agreement schemes between the entities involved, access control protocols and also schemes for transferring ownership of vital patient information. Besides, the resource-limited sensors in the IoT have real difficulties in achieving this goal. Motivated by these considerations, in this work we propose a new lightweight authentication and ownership transfer protocol for e-health systems in the context of IoT (LACO in short). The goal is to propose a secure and energy-efficient protocol that not only provides authentication and key agreement but also satisfies access control and preserves the privacy of doctors and patients. Moreover, this is the first time that the ownership transfer of users is considered. In the ownership transfer phase of the proposed scheme, the medical server can change the ownership of patient information. In addition, the LACO protocol overcomes the security flaws of recent authentication protocols that were proposed for e-health systems, but are unfortunately vulnerable to traceability, de-synchronization, denial of service (DoS), and insider attacks. To avoid past mistakes, we present formal (i.e., conducted on ProVerif language) and informal security analysis for the LACO protocol. All this ensures that our proposed scheme is secure against the most common attacks in IoT systems. Compared to the predecessor schemes, the LACO protocol is both more efficient and more secure to use in e-health systems. •We present several serious security attacks against Zhang et al. scheme (called ZZTL). Our proposed attacks include user traceability, de-synchronization, DoS and insider attacks.•In order to increase the security level offered by ZZTL protocol, we fix all security faults found in this scheme.•We propose a new architecture involving three main entities. We also provide the access control mechanism during the authentication phase.•We also consider the situation where the current doctor of the patient wants to transfer her/his privileges to a new doctor (ownership transfer).•The security of the proposed scheme is examined from a formal (ProVerif language) and informal point of view.•The efficiency of our proposal is higher than the predecessor schemes. Therefore our scheme can be used for resource-constrained sensors in IoT systems.
Smart city is an important concept in urban development. The use of information and communication technology to promote quality of life and the management of natural resources is one of the main goals in smart cities. On the other hand, at any time, thousands of mobile users send a variety of information on the network, and this is the main challenge in smart cities. To overcome this challenge and collect data from roaming users, the global mobility network (GLOMONET) is a good approach for information transfer. Consequently, designing a secure protocol for GLOMONET is essential. The main intention of this paper is to provide a secure protocol for GLOMONET in smart cities. To do this, we design a protocol that is based on Li et al.’s protocol, which is not safe against our proposed attacks. Our protocol inherits all the benefits of the previous one; it is entirely secure and does not impose any more communication overhead. We formally analyze the protocol using BAN logic and compare it to similar ones in terms of performance and security, which shows the efficiency of our protocol. Our proposed protocol enables mobile users and foreign agents to share a secret key in 6.1 ms with 428 bytes communication overhead, which improves the time complexity of the previous protocol to 53%.
Middleboxes have become a vital part of modern networks by providing services such as load balancing, optimization of network traffic, and content filtering. A sequence of middleboxes comprising a logical service is called a Service Function Chain (SFC). In this context, the main issues are to maintain an acceptable level of network path survivability and a fair allocation of the resource between different demands in the event of faults or failures. In this paper, we focus on the problems of traffic engineering, failure recovery, fault prevention, and SFC with reliability and energy consumption constraints in Software Defined Networks (SDN). These types of deployments use Fog computing as an emerging paradigm to manage the distributed small-size traffic flows passing through the SDN-enabled switches (possibly Fog Nodes). The main aim of this integration is to support service delivery in real-time, failure recovery, and fault-awareness in an SFC context. Firstly, we present an architecture for Failure Recovery and Fault Prevention called FRFP; this is a multi-tier structure in which the real-time traffic flows pass through SDN-enabled switches to jointly decrease the network side-effects of flow rerouting and energy consumption of the Fog Nodes. We then mathematically formulate an optimization problem called the Optimal Fog-Supported Energy-Aware SFC rerouting algorithm (OFES) and propose a near-optimal heuristic called Heuristic OFES (HFES) to solve the corresponding problem in polynomial time. In this way, the energy consumption and the reliability of the selected paths are optimized, while the Quality of Service (QoS) constraints are met and the network congestion is minimized. In a reliability context, the focus of this work is on fault prevention; however, since we use a reallocation technique, the proposed scheme can be used as a failure recovery scheme. We compare the performance of HFES and OFES in terms of energy consumption, average path length, fault probability, network side-effects, link utilization, and Fog Node utilization. Additionally, we analyze the computational complexity of HFES. We use a real-world network topology to evaluate our algorithm. The simulation results show that the heuristic algorithm is applicable to large-scale networks.
Providing real-time cloud services to Vehicular Clients (VCs) must cope with delay and delay-jitter issues. Fog computing is an emerging paradigm that aims at distributing small-size self-powered data centers (e.g., Fog nodes) between remote Clouds and VCs, in order to deliver data-dissemination real-time services to the connected VCs. Motivated by these considerations, in this paper, we propose and test an energy-efficient adaptive resource scheduler for Networked Fog Centers (NetFCs). They operate at the edge of the vehicular network and are connected to the served VCs through Infrastructure-to-Vehicular (I2V) TCP/IP-based single-hop mobile links. The goal is to exploit the locally measured states of the TCP/IP connections, in order to maximize the overall communication-plus-computing energy efficiency, while meeting the application-induced hard QoS requirements on the minimum transmission rates, maximum delays and delay-jitters. The resulting energy-efficient scheduler jointly performs: (i) admission control of the input traffic to be processed by the NetFCs; (ii) minimum-energy dispatching of the admitted traffic; (iii) adaptive reconfiguration and consolidation of the Virtual Machines (VMs) hosted by the NetFCs; and, (iv) adaptive control of the traffic injected into the TCP/IP mobile connections. The salient features of the proposed scheduler are that: (i) it is adaptive and admits distributed and scalable implementation; and, (ii) it is capable to provide hard QoS guarantees, in terms of minimum/maximum instantaneous rates of the traffic delivered to the vehicular clients, instantaneous rate-jitters and total processing delays. Actual performance of the proposed scheduler in the presence of: (i) client mobility; (ii) wireless fading; and, (iii) reconfiguration and consolidation costs of the underlying NetFCs, is numerically tested and compared against the corresponding ones of some state-of-the-art schedulers, under both synthetically generated and measured real-world workload traces.
We propose a novel model, called joint computing, data transmission and migration energy costs (JCDME), for the allocation of virtual elements (VEs), with the goal of minimizing the energy consumption in a software-defined cloud data center (SDDC). More in detail, we model the energy consumption by considering the computing costs of the VEs on the physical servers, the costs for migrating VEs across the servers, and the costs for transferring data between VEs. In addition, JCDME introduces a weight parameter to avoid an excessive number of VE migrations. Specifically, we propose three different strategies to solve the JCDME problem with an automatic and adaptive computation of the weight parameter for the VEs migration costs. We then evaluate the considered strategies over a set of scenarios, ranging from a small sized SDDC up to a medium-sized SDDC composed of hundreds of VEs and hundreds of servers. Our results demonstrate that JCDME is able to save up to an additional 7% of energy with respect to previous energy-aware algorithms, without a substantial increase in the solution complexity.
The Internet of Everything paradigm is being rapidly adopted in developing applications for different domains like smart agriculture, smart city, big data streaming, and so on. These IoE applications are leveraging cloud computing resources for execution. Fog computing, which emerged as an extension of cloud computing, supports mobility, heterogeneity, geographical distribution, context awareness, and services such as storage, processing, networking, and analytics on nearby fog nodes. The resource-limited, heterogeneous, dynamic, and uncertain fog environment makes task scheduling a great challenge that needs to be investigated. The article is motivated by this consideration and presents a systematic, comprehensive, and detailed comparative study by discussing the merits and demerits of different scheduling algorithms, focused optimization metrics, and evaluation tools in the fog computing and IoE environment. The goal of this survey article is fivefold. First, we review the fog computing and IoE paradigms. Second, we delineate the optimization metric engaged with fog computing and IoE environment. Third, we review, classify, and compare existing scheduling algorithms dealing with fog computing and IoE environment paradigms by leveraging some examples. Fourth, we rationalize the scheduling algorithms and point out the lesson learned from the survey. Fifth, we discuss the open issues and future research directions to improve scheduling in fog computing and the IoE environment.
Presents the introductory welcome message from the conference proceedings. May include the conference officers' congratulations to all involved with the conference event and publication of the proceedings record.
The vehicular ad hoc network (VANET) is a platform for exchanging information between vehicles and everything to enhance driver's driving experience and improve traffic conditions. The reputation system plays an essential role in judging whether to communicate with the target vehicle based on other vehicles' feedback. However, existing reputation systems ignore the privacy protection of feedback providers. Additionally, traditional VANET based on wireless sensor networks (WSNs) has limited power, storage, and processing capabilities, which cannot meet the real-world demands in a practical VANET deployment. Thus, we attempt to integrate cloud computing with VANET and proposes a privacy-preserving protocol of vehicle feedback (PPVF) for cloud-assisted VANET. In cloud-assisted VANET, we integrate homomorphic encryption and data aggregation technology to design the scheme PPVF, in which with the assistance of the roadside units (RSU), cloud service provider (CSP) obtains the total number of vehicles with the corresponding parameters in the feedback for reputation calculation without violating individual feedback privacy. Simulation results and security analysis confirm that PPVF achieves effective privacy protection for vehicle feedback with acceptable computational and communication burden. Besides, the RSU is capable of handling 1999 messages for every 300ms, so as the number of vehicles in the communication domain increases, the PPVF has a lower message loss rate.
This letter highlights the combined advantages of Open Radio Access Network (O-RAN) and distributed Artificial Intelligence (AI) in network slicing. O-RAN's virtualization and disaggregation techniques enable efficient resource allocation, while AI-driven networks optimize performance and decision-making. We propose a federated Deep Reinforcement Learning (DRL) approach to offload dynamic RAN disaggregation to edge sites to enable local data processing and faster decision-making. Our objective is to optimize dynamic RAN disaggregation by maximizing resource utilization and minimizing reconfiguration overhead. Through performance evaluation, our proposed approach surpasses the distributed DRL approach in the training phase. By modifying the learning rate, we can influence the variance of rewards and enhance the convergence of training. Moreover, fine-tuning the reward function's weighting factor enables us to attain the targeted network Key Performance Indicators (KPIs).
Cloud computing platforms support the Internet of Vehicles, but the main bottlenecks are high latency and massive data transmission in cloud-based processing. Vehicular fog computing has emerged as a promising paradigm to accommodate the increasing computational needs of vehicles. It provides low latency network services that are most important for latency-sensitive tasks. The dynamic nature of VFC, having vehicles with heterogeneous computing resources, vehicle mobility, and diverse tasks with different priorities are the main challenges in vehicular fog networks. In VFC, vehicles can share their idle compute resources with other task-generating vehicles. So, scheduling the tasks on the idle resources of resource-limited vehicles is very important. Existing solutions use a heuristic approach to solve this issue but lack generalizability and adaptability. In this paper, we describe a PPO-based intelligent, priority and deadline-aware online and distributed resource allocation and task scheduling algorithm, called IRATS, in vehicular fog networks. IRATS formulates the resource allocation problem as a Markov decision process to minimize the waiting time and delay of tasks. For vehicles sharing their idle resources, we design a task scheduler for the orderly execution of received tasks according to their priorities using multi-level queues. We conducted extensive simulations using SUMO, OMNeT++, Veins, and veins-gym to validate the effectiveness of the presented algorithm. The simulation results confirm that the proposed algorithm improves the percentage of in-time completed tasks and decreases the packet loss, waiting time, and end-to-end delay as compared to random, A2C, and DQN algorithms considering the task priority and link duration of vehicles.
Integrating information and communication technologies into the power generation, transmission and distribution system provides a new concept called Smart Grid (SG). The wide variety of devices connected to the SG communication infrastructure generates heterogeneous data with different Quality of Service (QoS) requirements and communication technologies. An intrusion Detection System (IDS) is a surveillance system monitoring the traffic flow over the network, seeking any abnormal behaviour to detect possible intrusions or attacks against the SG system. Distributed fashion of power and data in SG leads to an increase in the complexity of analysing the QoS and user requirements. Thus, we require a Big Data-aware distributed IDS dealing with the malicious behaviour of the network. Motivated by this, we design a distributed IDS dealing with anomaly big data and impose the proper defence algorithm to alert the SG. This paper proposes a new smart meter (SM) architecture, including a distributed IDS model (SM-IDS). Secondly, we implement SM-IDS using supervised ML algorithms. Finally, a distributed IDS model is introduced using federated learning. Numerical results approve that Neighbourhood Area Network IDS (NAN-IDS) can help decrease smart meters' energy and resource consumption. Thus, SM-IDS achieves an accuracy of 84.31% with a detection rate of 74.69%. Also, NAN-IDS provides an accuracy of 87.40% and a detection rate of 86.73%.
A multi-frame Neighbor Discovery Protocol over a DTN network with RFID Devices is proposed. This protocol is based on a Sift-distribution (s-Persistent) in order to differentiate the access probability to slots among RFID devices. Moreover, our approach has been considered in a mono-frame and multi-frame scenario. Our s-Persistent approach is applied in a simulated test and in a real test-bed in order to see the effectiveness of the proposal in comparison with a well-known technique such as p-Persistent protocol. Performance Evaluation has been evaluated in terms of number discovered neighbors under different numbers of nodes and frames. Obtained results shows that s-Persistent performs better than p-Persistent increasing the number of discovered neighbors.
Fog computing is a paradigm to overcome the cloud computing limitations which provides low latency to the users’ applications for the Internet of Things (IoT). Software-defined networking (SDN) is a practical networking infrastructure that provides a great capability in managing network flows. SDN switches are powerful devices, which can be used as fog devices/fog gateways simultaneously. Hence, fog devices are more vulnerable to several attacks. TCP SYN flood attack is one of the most common denial of service attacks, in which a malicious node produces many half-open TCP connections on the targeted computational nodes so as to break them down. Motivated by this, in this paper, we apply SDN concepts to address TCP SYN flood attacks in IoT–fog networks . We propose FUPE, a security-aware task scheduler in IoT–fog networks. FUPE puts forward a fuzzy-based multi-objective particle swarm Optimization approach to aggregate optimal computing resources and providing a proper level of security protection into one synthetic objective to find a single proper answer. We perform extensive simulations on IoT-based scenario to show that the FUPE algorithm significantly outperforms state-of-the-art algorithms. The simulation results indicate that, by varying the attack rates, the number of fog devices, and the number of jobs, the average response time of FUPE improved by 11% and 17%, and the network utilization of FUPE improved by 10% and 22% in comparison with Genetic and Particle Swarm Optimization algorithms, respectively.
Resource scheduling approaches (RSA) are the core component of mobile cloud computing (MCC) systems that aim to optimally allocate cloud-based remote resources to resource-intensive components of mobile applications. The ultimate goal of RSA is to reduce execution time and energy consumption of resource-intensive mobile applications which contributes to successful MCC adoption. Role of RSA is critical in efficiently executing resource-intensive mobile applications in the cloud. Although several aspects of MCC have been extensively reviewed, analysis of RSAs in MCC is overlooked. Therefore, it is important to provide a comprehensive review of RSA to complement existing literature in MCC. In this paper, we conduct a survey to review the state-of-the-art RSA approaches in MCC and present the taxonomy of existing RSA approaches. We present a brief tutorial on resource scheduling in MCC followed by a critical review of some of the most credible approaches to highlight their advantages and disadvantages. We then discuss the open challenges in this area and point out future research directions.
We design and test a distributed and adaptive resource management controller in Vehicular Access Networks, allowing energy and computing-limited car smart phones to opportunistically accede to a spectral-limited wireless backbone. We cast the resource management problem into a suitable constrained stochastic Network Utility Maximization problem and derive the optimal cognitive resource management controller, which dynamically allocates the access time-windows at the serving Roadside Units (i.e., the primary users) and the access rates and traffic flows at the served Vehicular Clients (i.e., the secondary users), allowing hard reliability guarantees to Roadside Units. We validated the controller performances in real-word application scenarios.
This paper studies the impact of an intelligent reflecting surface (IRS) on computational performance in a mobile edge computing (MEC) system. Specifically, an access point (AP) equipped with an edge server provides MEC services to multiple internet of thing (IoT) devices that choose to offload a portion of their own computational tasks to the AP with the remaining portion being locally computed. We deploy an IRS to enhance the computational performance of the MEC system by intelligently adjusting the phase shift of each reflecting element. A joint design problem is formulated for the considered IRS assisted MEC system, aiming to optimize its sum computational bits and taking into account the CPU frequency, the offloading time allocation, transmit power of each device as well as the phase shifts of the IRS. To deal with the non-convexity of the formulated problem, we conduct our algorithm design by finding the optimized phase shifts first and then achieving the jointly optimal solution of the CPU frequency, the transmit power and the offloading time allocation by considering the Lagrange dual method and Karush-Kuhn-Tucker (KKT) conditions. Numerical evaluations highlight the advantage of the IRS-assisted MEC system in comparison with the benchmark schemes.
Protecting large-scale networks, especially Software-Defined Networks (SDNs), against distributed attacks in a cost effective manner plays a prominent role in cybersecurity. One of the pervasive approaches to plug security holes and prevent vulnerabilities from being exploited is Moving Target Defense (MTD), which can be efficiently implemented in SDN as it needs comprehensive and proactive network monitoring. The critical key in MTD is to shuffle the least number of hosts with an acceptable security impact and keep the shuffling frequency low. In this paper, we have proposed an SDN-oriented Cost-effective Edge-based MTD Approach (SCEMA) to mitigate Distributed Denial of Service (DDoS) attacks at a lower cost by shuffling an optimized set of hosts that have the highest number of connections to the critical servers. These connections are named edges from a graph-theoretical point of view. We have designed a system based on SCEMA and simulated it in Mininet. The results show that SCEMA has lower (52.58%) complexity than the previous related MTD methods improving the security level by 14.32%.
In this contribution, we design and test the performance of a distributed and adaptive resource management controller, which allows the optimal exploitation of Cognitive Radio and soft-input/soft-output data fusion in Vehicular Access Networks. The ultimate goal is to allow energy and computing-limited car smartphones to utilize the available Vehicular-to-Infrastructure WiFi connections for performing traffic offloading towards local or remote Clouds by opportunistically acceding to a spectral-limited wireless backbone built up by multiple Roadside Units. For this purpose, we recast the afforded resource management problem into a suitable constrained stochastic Network Utility Maximization problem. Afterwards, we derive the optimal cognitive resource management controller, which dynamically allocates the access time-windows at the serving Roadside Units (i.e., the access points) together with the access rates and traffic flows at the served Vehicular Clients (i.e., the secondary users of the wireless backbone). Interestingly, the developed controller provides hard reliability guarantees to the Cloud Service Provider (i.e., the primary user of the wireless backbone) on a per-slot basis. Furthermore, it is also capable to self-acquire context information about the currently available bandwidth-energy resources, so as to quickly adapt to the mobility-induced abrupt changes of the state of the vehicular network, even in the presence of fadings, imperfect context information and intermittent Vehicular-to-Infrastructure connectivity. Finally, we develop a related access protocol, which supports a fully distributed and scalable implementation of the optimal controller. (C) 2014 Elsevier Inc. All rights reserved.
A grid computing environment provides a type of distributed computation that is unique because it is not centrally managed and it has the capability to connect heterogeneous resources. A grid system provides location-independent access to the resources and services of geographically distributed machines. An essential ingredient for supporting location-independent computations is the ability to discover resources that have been requested by the users. Because the number of grid users can increase and the grid environment is continuously changing, a scheduler that can discover decentralized resources is needed. Grid resource scheduling is considered to be a complicated, NP-hard problem because of the distribution of resources, the changing conditions of resources, and the unreliability of infrastructure communication. Various artificial intelligence algorithms have been proposed for scheduling tasks in a computational grid. This paper uses the imperialist competition algorithm (ICA) to address the problem of independent task scheduling in a grid environment, with the aim of reducing the makespan. Experimental results compare ICA with other algorithms and illustrate that ICA finds a shorter makespan relative to the others. Moreover, it converges quickly, finding its optimum solution in less time than the other algorithms.
Commodity HardWare (CHW) is currently used in the Internet to deploy large data centers or small computing nodes. Moreover, CHW will be also used to deploy future telecommunication networks, thanks to the adoption of the forthcoming network softwarization paradigm. In this context, CHW machines can be put in Active Mode (AM) or in Sleep Mode (SM) several times per day, based on the traffic requirements from users. However, the transitions between the power states may introduce fatigue effects, which may increase the CHW maintenance costs. In this paper, we perform a measurement campaign of a CHW machine subject to power state changes introduced by SM. Our results show that the temperature change due to power state transitions is not negligible, and that the abrupt stopping of the fans on hot components (such as the CPU) tends to spread the heat over the other components of the CHW machine. In addition, we also show that the CHW failure rate is reduced by a factor of 5 when the number of transitions between AM and SM states is more than 20 per day and the SM duration is around 800 [s].
Volunteer computing is an Internet-based distributed computing in which volunteers share their extra available resources to manage large-scale tasks. However, computing devices in a Volunteer Computing System (VCS) are highly dynamic and heterogeneous in terms of their processing power, monetary cost, and data transferring latency. To ensure both of the high Quality of Service (QoS) and low cost for different requests, all of the available computing resources must be used efficiently. Task scheduling is an NP-hard problem that is considered as one of the main critical challenges in a heterogeneous VCS. Due to this, in this article, we design two task scheduling algorithms for VCSs, named Min-CCV and Min-V. The main goal of the proposed algorithms is jointly minimizing the computation, communication, and delay violation cost for the Internet of Things (IoT) requests. Our extensive simulation results show that proposed algorithms are able to allocate tasks to volunteer fog/cloud resources more efficiently than the state-of-the-art. Specifically, our algorithms improve the deadline satisfaction task rates around 99.5% and decrease the total cost between 15 to 53% in comparison with the genetic-based algorithm.
The distinguishing feature of the Fog Computing (FC) paradigm is that FC spreads communication and computing resources over the wireless access network, so as to provide resource augmentation to resource and energy-limited wireless (possibly mobile) devices. Since FC would lead to substantial reductions in energy consumption and access latency, it will play a key role in the realization of the Fog of Everything (FoE) paradigm. The core challenge of the resulting FoE paradigm is to materialize the seamless convergence of three distinct disciplines, namely, broadband mobile communication, cloud computing, and Internet of Everything (IoE). In this paper, we present a new IoE architecture for FC in order to implement the resulting FoE technological platform. Then, we elaborate the related Quality of Service (QoS) requirements to be satisfied by the underlying FoE technological platform. Furthermore, in order to corroborate the conclusion that advancements in the envisioned architecture description, we present: (i) the proposed energy aware algorithm adopt Fog data center; and, (ii) the obtained numerical performance, for a real-world case study that shows that our approach saves energy consumption impressively in the Fog data Center compared with the existing methods and could be of practical interest in the incoming Fog of Everything (FoE) realm.
In this paper, we propose a dynamic resource provisioning scheduler to maximize the application throughput and minimize the computing-plus-communication energy consumption in virtualized networked data centers. The goal is to maximize the energy-efficiency, while meeting hard QoS requirements on processing delay. The resulting optimal resource scheduler is adaptive, and jointly performs: i) admission control of the input traffic offered by the cloud provider; ii) adaptive balanced control and dispatching of the admitted traffic; iii) dynamic reconfiguration and consolidation of the Dynamic Voltage and Frequency Scaling (DVFS)-enabled virtual machines instantiated onto the virtualized data center. The proposed scheduler can manage changes of the workload without requiring server estimation and prediction of its future trend. Furthermore, it takes into account the most advanced mechanisms for power reduction in servers, such as DVFS and reduced power states. Performance of the proposed scheduler is numerically tested and compared against the corresponding ones of some state-of-the-art schedulers, under both synthetically generated and measured real-world workload traces. The results confirm the delay-vs.-energy good performance of the proposed scheduler.
This letter proposes an innovative energy-efficient Radio Access Network (RAN) disaggregation and virtualization method for Open RAN (O-RAN) that effectively addresses the challenges posed by dynamic traffic conditions. In this case, the energy consumption is primarily formulated as a multi-objective optimization problem and then solved by integrating Advantage Actor-Critic (A2C) algorithm with a sequence-to-sequence model due to sequentially of RAN disaggregation and long-term dependencies. According to the results, our proposed solution for dynamic Virtual Network Functions (VNF) splitting outperforms approaches that do not involve VNF splitting, significantly reducing energy consumption. The solution achieves up to 56% and 63% for business and residential areas under traffic conditions, respectively.
Recent telecommunication paradigms, such as big data, Internet of Things (IoT), ubiquitous edge computing (UEC), and machine learning, are encountering with a tremendous number of complex applications that require different priorities and resource demands. These applications usually consist of a set of virtual machines (VMs) with some predefined traffic load between them. The efficiency of a cloud data center (CDC) as prominent component in UEC significantly depends on the efficiency of its VM placement algorithm applied. However, VM placement is an NP-hard problem and thus there exist practically no optimal solution for this problem. In this paper, motivated by this, we propose a priority, power and traffic-aware approach for efficiently solving the VM placement problem in a CDC. Our approach aims to jointly minimize power consumption, network consumption and resource wastage in a multi-dimensional and heterogeneous CDC. To evaluate the performance of the proposed method, we compared it to the state-of-the-art on a fat-tree topology under various experiments. Results demonstrate that the proposed method is capable of reducing the total network consumption up to 29%, the consumption of power up to 18%, and the wastage of resources up to 68%, compared to the second-best results.
Label manipulation attacks are a subclass of data poisoning attacks in adversarial machine learning used against different applications, such as malware detection. These types of attacks represent a serious threat to detection systems in environments having high noise rate or uncertainty, such as complex networks and Internet of Thing (IoT). Recent work in the literature has suggested using the K-nearest neighboring algorithm to defend against such attacks. However, such an approach can suffer from low to miss-classification rate accuracy. In this paper, we design an architecture to tackle the Android malware detection problem in IoT systems. We develop an attack mechanism based on silhouette clustering method, modified for mobile Android platforms. We proposed two convolutional neural network-type deep learning algorithms against this Silhouette Clustering-based Label Flipping Attack. We show the effectiveness of these two defense algorithms—label-based semi-supervised defense and clustering-based semi-supervised defense—in correcting labels being attacked. We evaluate the performance of the proposed algorithms by varying the various machine learning parameters on three Android datasets: Drebin, Contagio, and Genome and three types of features: API, intent, and permission. Our evaluation shows that using random forest feature selection and varying ratios of features can result in an improvement of up to 19% accuracy when compared with the state-of-the-art method in the literature.
Summary In the last decade, the rising trend in the popularity of smartphones motivated software developers to increase application functionality. However, increasing application functionality demands extra power budget that as a result, decreases smartphone battery lifetime. Optimizing energy critical sections of an application creates an opportunity to increase battery lifetime. Smartphone application energy estimation helps investigate energy consumption behavior of an application at diversified granularity (eg, coarse and fine granular) for optimal battery resource use. This study explores energy estimation and modeling schemes to highlight their advantages and shortcomings. It classifies existing smartphone application energy estimation and modeling schemes into 2 categories, ie, code analysis and mobile components power model–based estimation owing to their architectural designs. Moreover, it further classifies code analysis–based modeling and estimation schemes in simulation‐based and profiling‐based categories. It compares existing energy estimation and modeling schemes based on a set of parameters common in most literature to highlight the commonalities and differences among reported literature. Existing application energy estimation schemes are low‐accurate, resource expensive, or non‐scalable, as they consider marginally accurate smart battery's voltage/current sensors, low‐rate power capturing tools, and labor‐driven lab‐setting environment to propose power models for smartphone application energy estimation. Besides, the energy estimation overhead of the components power model–based estimation schemes is very high as they physically run the application on a smartphone for energy profiling. To optimize smartphone application energy estimation, we have highlighted several research issues to help researchers of this domain to understand the problem clearly. As shown in figure, this paper discusses energy estimation methods and techniques for energy estimation of smartphone applications. It estimates energy consumption of applications based on smartphone components power models or source code energy models. It proposes taxonomies and highlights open research issues. It concludes that energy estimation is a resource expensive task owing to high profiling overhead.
Fog computing (FC) and Internet of Everything (IoE) are two emerging technological paradigms that, to date, have been considered standing-alone. However, because of their complementary features, we expect that their integration can foster a number of computing and network-intensive pervasive applications under the incoming realm of the future Internet. Motivated by this consideration, the goal of this position paper is fivefold. First, we review the technological attributes and platforms proposed in the current literature for the standing-alone FC and IoE paradigms. Second, by leveraging some use cases as illustrative examples, we point out that the integration of the FC and IoE paradigms may give rise to opportunities for new applications in the realms of the IoE, Smart City, Industry 4.0, and Big Data Streaming, while introducing new open issues. Third, we propose a novel technological paradigm, the Fog of Everything (FoE) paradigm, that integrates FC and IoE and then we detail the main building blocks and services of the corresponding technological platform and protocol stack. Fourth, as a proof-of-concept, we present the simulated energy-delay performance of a small-scale FoE prototype, namely, the V-FoE prototype. Afterward, we compare the obtained performance with the corresponding one of a benchmark technological platform, e.g., the V-D2D one. It exploits only device-to-device links to establish inter-thing "ad hoc" communication. Last, we point out the position of the proposed FoE paradigm over a spectrum of seemingly related recent research projects.
The emerging utilization of Software-as-a-Service (SaaS) Fog computing centers as an Internet virtual computing commodity is raising concerns over the energy consumptions of networked data centers for the support of delay-sensitive applications. In addition to the energy consumed by the servers, the energy wasted by the network devices that support TCP/IP reliable inter-Virtual Machines (VMs) connections is becoming a significant challenge. In this paper, we propose and develop a framework for the joint characterization and optimization of TCP/IP SaaS Fog data centers that utilize a bank of queues for increasing the fraction of the admitted workload. Our goal is two-fold: (i) we maximize the average workload admitted by the data center; and, (ii) we minimize the resulting networking-plus-computing average energy consumption. For this purpose, we exploit the Lyapunov stochastic optimization approach, in order to design and analyze an optimal (yet practical) online joint resource management framework, which dynamically performs: (i) admission control; (ii) dispatching of the admitted workload; (iii) flow control of the inter-VM TCP/IP connections; (iv) queue control; (v) up/down scaling of the processing frequencies of the instantiated VMs; and, (vi) adaptive joint consolidation of both physical servers and TCP/IP connections. The salient features of the resulting scheduler (e.g., the Q* scheduler) are that: (i) it admits distributed and scalable implementation; (ii) it provides deterministic bounds on the instantaneous queue backlogs; (iii) it avoids queue overflow phenomena; and, (iv) it effectively tracks the (possibly unpredictable) time-fluctuations of the input workload, in order to perform joint resource consolidation without requiring any a prioriinformation and/or forecast of the input workload. Actual energy and delay performances of the proposed scheduler are numerically evaluated and compared against the corresponding ones of some competing and state-of-the-art schedulers, under: (i) Fast - Giga - 10Giga Ethernet switching technologies; (ii) various settings of the reconfiguration-consolidation costs; and, (iii) synthetic, as well as real-world workloads. The experimental results support the conclusion that the proposed scheduler can achieve over 30% energy savings.
The sheer volume of IIOT malware is one of the most serious security threats in today's interconnected world, with new types of advanced persistent threats and advanced forms of obfuscations. This paper presents a robust Federated Learning-based architecture called Fed-IIoT for detecting Android malware applications in IIoT. Fed-IIoT consists of two parts: i) participant side, where the data are triggered by two dynamic poisoning attacks based on a generative adversarial network (GAN) and Federated Generative Adversarial Network (FedGAN). While ii) server-side, aim to monitor the global model and shape a robust collaboration training model, by avoiding anomaly in aggregation by GAN network (A3GAN) and adjust two GAN-based countermeasure algorithms. One of the main advantages of Fed-IIoT is that devices can safely participate in the IIoT and efficiently communicate with each other, with no privacy issues. We evaluate our solution through experiments on various features using three IoT datasets. The results confirm the high accuracy rates of our attack and defence algorithms and show that the A3GAN defensive approach preserves the robustness of data privacy for Android mobile users and is about 8% higher accuracy with existing state-of-the-art solutions.
6G technology enables AI-based massive IoT to manage network resources and data with ultra high speed, responsive network and wide coverage. However, many artificial intelligence-enabled internet of things (AIoT) systems are vulnerable to adversarial example attacks. Therefore, designing robust deep learning models that can be deployed on resource-constrained devices has become an important research topic in the field of 6G-enabled AIoT. In this paper, we propose a method for automatically searching for robust and efficient neural network structures for AIoT systems. By introducing a skip connection structure, a feature map with reduced front-end influence can be used for calculations during the classification process. Additionally, a novel type of of dense connected search space is proposed. By relaxing this space, it is possible to search for network structures efficiently. In addition, combined with adversarial training and model delay constraints, we propose a multi-objective gradient optimization method to realize the automatic searching of network structures. Experimental results demonstrate that our method is effective for AIoT systems and superior to state-of-the-art neural architecture search algorithms.
Cloud envisioned Cyber-Physical Systems (CCPS) is a practical technology that relies on the interaction among cyber elements like mobile users to transfer data in cloud computing. In CCPS, cloud storage applies data deduplication techniques aiming to save data storage and bandwidth for real-time services. In this infrastructure, data deduplication eliminates duplicate data to increase the performance of the CCPS application. However, it incurs security threats and privacy risks. In this area, several types of research have been done. Nevertheless, they are suffering from a lack of security, high performance, and applicability. Motivated by this, we propose a message Lock Encryption with neVer-decrypt homomorphic EncRyption (LEVER) protocol between the uploading CCPS user and cloud storage to reconcile the encryption and data deduplication. Interestingly, LEVER is the first brute-force resilient encrypted deduplication with only cryptographic two-party interactions
Monitoring a set of targets and extending network lifetime is a critical issue in wireless sensor networks (WSNs). Various coverage scheduling algorithms have been proposed in the literature for monitoring deployed targets in WSNs. These algorithms divide the sensor nodes into cover sets, and each cover set can monitor all targets. It is proven that finding the maximum number of disjointed cover sets is an NP-complete problem. In this paper we present a novel and efficient cover set algorithm based on Imperialist Competitive Algorithm (ICA). The proposed algorithm taking advantage of ICA determines the sensor nodes that must be selected in different cover sets. As the presented algorithm proceeds, the cover sets are generated to monitor all deployed targets. In order to evaluate the performance of the proposed algorithm, several simulations have been conducted and the obtained results show that the proposed approach outperforms similar algorithms in terms of extending the network lifetime. Also, our proposed algorithm has a coverage redundancy that is about 1-2 % close to the optimal value.
At present, specific voice control has gradually become an important means for 5G-IoT-aided industrial control systems. However, the security of specific voice control system needs to be improved, because the voice cloning technology may lead to industrial accidents and other potential security risks. In this paper, we propose a transductive voice transfer learning method to learn the predictive function from the source domain and fine-tune in the target domain adaptively. The target learning task and source learning task are both synthesizing speech signals from the given audio while the data sets of both domains are different. By adding different penalty values to each instances and minimizing the expected risk, an optimal precise model can be learned. Many details of the experimental results show that our method can effectively synthesize the speech of the target speaker with small samples.
Cloud computing efficiency greatly depends on the efficiency of the virtual machines (VMs) placement strategy used. However, VM placement has remained one of the major challenging issues in cloud computing mainly because of the heterogeneity in both virtual and physical machines (PMs), the multidimensionality of the resources, and the increasing scale of the cloud data centers (CDCs). An inefficiency in VM placement strategy has a significant influence on the quality of service provided, the amount of energy consumed, and the running costs of the CDCs. To address these issues, in this article, we propose a greedy randomized VM placement (GRVMP) algorithm in a large-scale CDC with heterogeneous and multidimensional resources. GRVMP inspires the "power of two choices" model and places VMs on the more power-efficient PMs to jointly optimize CDC energy usage and resource utilization. The performance of GRVMP is evaluated using synthetic and real-world production scenarios (Amazon EC2) with several performance matrices. The results of the experiment confirm that GRVMP jointly optimizes power usage and the overall wastage of resource utilization. The results also show that GRVMP significantly outperforms the baseline schemes in terms of the performance metrics used.
Fog computing is a decentralised model which can help cloud computing for providing high quality-of-service (QoS) for the Internet of Things (IoT) application services. Service placement problem (SPP) is the mapping of services among fog and cloud resources. It plays a vital role in response time and energy consumption in fog–cloud environments. However, providing an efficient solution to this problem is a challenging task due to difficulties such as different requirements of services, limited computing resources, different delay, and power consumption profile of devices in fog domain. Motivated by this, in this study, we propose an efficient policy, called MinRE, for SPP in fog–cloud systems. To provide both QoS for IoT services and energy efficiency for fog service providers, we classify services into two categories: critical services and normal ones. For critical services, we propose MinRes, which aims to minimise response time, and for normal ones, we propose MinEng, whose goal is reducing the energy consumption of fog environment. Our extensive simulation experiments show that our policy improves the energy consumption up to 18%, the percentage of deadline satisfied services up to 14% and the average response time up to 10% in comparison with the second-best results.
In this paper, we develop four malware detection methods using Hamming distance to find similarity between samples which are first nearest neighbors (FNN), all nearest neighbors (ANN), weighted all nearest neighbors (WANN), and k-medoid based nearest neighbors (KMNN). In our proposed methods, we can trigger the alarm if we detect an Android app is malicious. Hence, our solutions help us to avoid the spread of detected malware on a broader scale. We provide a detailed description of the proposed detection methods and related algorithms. We include an extensive analysis to asses the suitability of our proposed similaritybased detection methods. In this way, we perform our experiments on three datasets, including benign and malware Android apps like Drebin, Contagio, and Genome. Thus, to corroborate the actual effectiveness of our classifier, we carry out performance comparisons with some state-of-the-art classification and malware detection algorithms, namely Mixed and Separated solutions, the program dissimilarity measure based on entropy (PDME) and the FalDroid algorithms. We test our experiments in a different type of features: API, intent, and permission features on these three datasets. The results confirm that accuracy rates of proposed algorithms are more than 90% and in some cases (i.e., considering API features) are more than 99%, and are comparable with existing state-of-the-art solutions.
Summary The ever increasing number of Android malware has always been a concern for cybersecurity professionals. Even though plenty of anti‐malware solutions exist, we hypothesize that the performance of existing approaches can be improved by deriving relevant attributes through effective feature selection methods. In this paper, we propose a novel two‐step feature selection approach based on Rough Set and Statistical Test named as RSST to extract refined system calls, which can effectively discriminate malware from benign apps. By refined set of system call, we mean the existence of highly relevant calls that are uniformly distributed thought target classes. Moreover, an optimal attribute set is created, which is devoid of redundant system calls. To address the problem of higher dimensional attribute set, we derived suboptimal system call space by applying the proposed feature selection method to maximize the separability between malware and benign samples. Comprehensive experiments conducted on three datasets resulted in an accuracy of 99.9%, Area Under Curve (AUC) of 1.0, with 1% False Positive Rate (FPR). However, other feature selectors (Information Gain, CFsSubsetEval, ChiSquare, FreqSel, and Symmetric Uncertainty) used in the domain of malware analysis resulted in the accuracy of 95.5% with 8.5% FPR. Moreover, the empirical analysis of RSST derived system calls outperformed other attributes such as permissions, opcodes, API, methods, call graphs, Droidbox attributes, and network traces.
Middleboxes have become a vital part of modern networks by providing services such as load balancing, optimization of network traffic, and content filtering. A sequence of middleboxes comprising a logical service is called a Service Function Chain (SFC). In this context, the main issues are to maintain an acceptable level of network path survivability and a fair allocation of the resource between different demands in the event of faults or failures. In this paper, we focus on the problems of traffic engineering, failure recovery, fault prevention, and SFC with reliability and energy consumption constraints in Software Defined Networks (SDN). These types of deployments use Fog computing as an emerging paradigm to manage the distributed small‐size traffic flows passing through the SDN‐enabled switches (possibly Fog Nodes). The main aim of this integration is to support service delivery in real‐time failure recovery in an SFC context. First, we present an architecture for Failure Recovery called FRFP; this is a multi‐tier structure in which the real‐time traffic flows pass through SDN‐enabled switches to jointly decrease the network side‐effects of flow rerouting and energy consumption of the Fog Nodes. We then mathematically formulate an optimization problem called the Optimal Fast Failure Recovery algorithm (OFFR) and propose a near‐optimal heuristic called Heuristic HFFR to solve the corresponding problem in polynomial time. In this way, the reliability of the selected paths are optimized, while the network congestion is minimized.
Wireless mesh networks (WMNs) consist of static nodes that usually have one or more radios or media. Optimal channel assignment (CA) for nodes is a challenging problem in WMNs. CA aims to minimize interference in the overall network and thus increase the total capacity of the network. This paper proposes a new method for solving the CA problem that comparatively performs more efficient than existing methods. The link layer in the TCP/IP model is a descriptive realm of networking protocols that operates on the local network link in routers discovery and neighboring hosts. TCP/IP employs the link-layer protocol (LLP) that is included among the hybrid states in CA methods, and learning automata are used to complete the algorithm with an intelligent method for suitable CA. We call this algorithm LLLA, which are short for LLP and learning automata. Our simulation results show that LLLA performs more efficient than ad hoc on-demand distance vector (AODV) types with respect to parameters such as packet drop, end-to-end delay, average goodput, jitter in special applications, and energy usage.
In recent years, we have witnessed tremendous advances in cloud data centers (CDCs) from the point of view of the communication layer. A recent report from Cisco Systems Inc demonstrates that CDCs, which are distributed across many geographical locations, will dominate the global data center traffic flow for the foreseeable future. Their importance is highlighted by a top‐line projection from this forecast that by 2019, more than four‐fifths of total data center traffic will be Cloud traffic. The geographical diversity of the computing resources in CDCs provides several benefits, such as high availability, effective disaster recovery, uniform access to users in different regions, and access to different energy sources. Although Cloud technology is currently predominant, it is essential to leverage new agile software technologies, agile processes, and agile applications near to both the edge and the users; hence, the concept of Fog has been developed.Fog computing (FC) has emerged as an alternative to traditional Cloud computing to support geographically distributed latency‐sensitive and QoS‐aware IoT applications while reducing the burden on data centers used in traditional Cloud computing. In particular, FC with features that can support heterogeneity and real‐time applications (eg, low latency, location awareness, and the capacity to process a large number of nodes with wireless access) is an attractive solution for delay‐ and resource‐constrained large‐scale applications. The distinguishing feature of the FC paradigm is that a set of Fog nodes (FNs) spreads communication and computing resources over the wireless access network to provide resource augmentation to resource‐limited and energy‐limited wireless (possibly mobile) devices. The joint management of Fog and Internet of Technology (IoT) paradigms can reduce the energy consumption and operating costs of state‐of‐the‐art Fog‐based data centers (FDCs). An FDC is dedicated to supervising the transmission, distribution, and communication of FC. As a vital component of the Internet of Everything (IoE) environment, an FDC is capable of filtering and processing a considerable amount of incoming data on edge devices, by making the data processing architecture distributed and thereby scalable. An FDC therefore provides a platform for filtering and analyzing the data generated by sensors utilizing the resources of FNs.Increasing interest is emerging in FDCs and CDCs that allow the delivery of various kinds of agile services and applications over telecommunication networks and the Internet, including resource provisioning, data streaming/transcoding, analysis of high‐definition videos across the edge of the network, IoE application analysis, etc. Motivated by these issues, this special section solicits original research and practical contributions that advance the use of CDCs/FDCs in new technologies such as IoT, edge networks, and industries. Results obtained from simulations are validated in terms of their boundaries by experiments or analytical results. The main objectives of this special issue are to provide a discussion forum for people interested in Cloud and Fog networking and to present new models, adaptive tools, and applications specifically designed for distributed and parallel on‐demand requests received from (mobile) users and Cloud applications.These papers presented in this special issue provide insights in fields related to Cloud and Fog/edge architecture, including parallel processing of Cloudlets/Foglets, the presentation of new emerging models, performance evaluation and improvements, and developments in Cloud/Fog applications. We hope that readers can benefit from the insights in these papers, and contribute to these rapidly growing areas.
Service function chaining (SFC) allows the forwarding of traffic flows along a chain of virtual network functions (VNFs). Software defined networking (SDN) solutions can be used to support SFC to reduce both the management complexity and the operational costs. One of the most critical issues for the service and network providers is the reduction of energy consumption, which should be achieved without impacting the Quality of Service. In this paper, we propose a novel resource allocation architecture which enables energy-aware SFC for SDN-based networks, considering also constraints on delay, link utilization, server utilization. To this end, we formulate the problems of VNF placement, allocation of VNFs to flows, and flow routing as integer linear programming (ILP) optimization problems. Since the formulated problems cannot be solved (using ILP solvers) in acceptable timescales for realistic problem dimensions, we design a set of heuristic to find near-optimal solutions in timescales suitable for practical applications. We numerically evaluate the performance of the proposed algorithms over a real-world topology under various network traffic patterns. Our results confirm that the proposed heuristic algorithms provide near-optimal solutions (at most 14% optimality-gap) while their execution time makes them usable for real-life networks.
Summary We consider the problem of managing a 5G network composed of virtualized entities, called reusable functional blocks (RFBs), as proposed by the Horizon 2020 SUPERFLUIDITY project. The RFBs are used to decompose network functions and services and are deployed on top of physical nodes, in order to realize the 5G functionalities. After formally modeling the RFBs in a 5G network, as well as the physical nodes hosting them, we formulate the problem of managing the 5G network through the RFBs, in order to satisfy different key performance indicators to users. In particular, we focus either on the maximization of the amount of downlink throughput sent to users or on the minimization of the number of powered‐on physical nodes. We then consider different scenarios to evaluate the proposed formulations. Our results show that, when an RFB‐based approach is put into place, a high level of flexibility and dynamicity is achieved. In particular, the RFBs can be shared, moved, and rearranged based on the network conditions. As a result, the downlink throughput can be extremely high, ie, more than 150 Mbps per user on average when the throughput maximization is pursued and more than 100 Mbps on average when the goal is the minimization of the number of powered‐on physical nodes. We consider the management of a 5G network composed of virtualized entities, called reusable functional blocks (RFBs), which are used to decompose network functions and services and are deployed on top of physical nodes, in order to realize the 5G functionalities. We then evaluate the performance of an RFB‐based 5G network, showing that the downlink throughput can be extremely high, ie, more than 150 [Mbps] per user on average when the throughput maximization is pursued.
We target the problem of managing the power states of the servers in a Cloud Data Center (CDC) to jointly minimize the electricity consumption and the maintenance costs derived from the variation of power (and consequently of temperature) on the servers' CPU. More in detail, we consider a set of virtual machines (VMs) and their requirements in terms of CPU and memory across a set of Time Slot (TSs). We then model the consumed electricity by taking into account the VMs processing costs on the servers, the costs for transferring data between the VMs, and the costs for migrating the VMs across the servers. In addition, we employ a material-based fatigue model to compute the maintenance costs needed to repair the CPU, as a consequence of the variation over time of the server power states. After detailing the problem formulation, we design an original algorithm, called Maintenance and Electricity Costs Data Center (MECDC), to solve it. Our results, obtained over several scenarios from a real CDC, show that MECDC largely outperforms two reference algorithms, which instead either target the load balancing or the energy consumption of the servers.
The proliferation of cloud data center applications and network function virtualization (NFV) boosts dynamic and QoS dependent traffic into the data centers network. Currently, lots of network routing protocols are requirement agnostic, while other QoS-aware protocols are computationally complex and inefficient for small flows. In this paper, a computationally efficient congestion avoidance scheme, called CECT, for software-defined cloud data centers is proposed. The proposed algorithm, CECT, not only minimizes network congestion but also reallocates the resources based on the flow requirements. To this end, we use a routing architecture to reconfigure the network resources triggered by two events: 1) the elapsing of a predefined time interval, or, 2) the occurrence of congestion. Moreover, a forwarding table entries compression technique is used to reduce the computational complexity of CECT. In this way, we mathematically formulate an optimization problem and define a genetic algorithm to solve the proposed optimization problem. We test the proposed algorithm on real-world network traffic. Our results show that CECT is computationally fast and the solution is feasible in all cases. In order to evaluate our algorithm in term of throughput, CECT is compared with ECMP (where the shortest path algorithm is used as the cost function). Simulation results confirm that the throughput obtained by running CECT is improved up to 3x compared to ECMP while packet loss is decreased up to 2x.
Summary The hierarchical routing algorithm is categorized as a kind of routing method using node clustering to create a hierarchical structure in large‐scale mobile ad hoc network (LMANET). In this paper, we proposed a new hierarchical clustering algorithm (HCAL) and a corresponded protocol for hierarchical routing in LMANET. The HCAL is designed based on a cost metric in the form of the link expiration time and node's relative degree. Correspondingly, the routing protocol for HCAL adopts a reactive protocol to control the existing cluster head (CH) nodes and handle proactive nodes to be considered as a cluster in LMANET. Hierarchical clustering algorithm jointly utilizes table‐driven and on‐demand routing by using a combined weight metric to search dominant set of nodes. This set is composed by link expiration time and node's relative degree to establish the intra/intercommunication paths in LMANET. The performance of the proposed algorithm and protocol is numerically evaluated in average end‐to‐end delay, number of CH per round, iteration count between the CHs, average CH keeping time, normalized routing overhead, and packet delivery ratio over a number of randomly generated benchmark scenarios. Furthermore, to corroborate the actual effectiveness of the HCAL algorithm, extensive performance comparisons are carried out with some state‐of‐the‐art routing algorithms, namely, Dynamic Doppler Velocity Clustering, Signal Characteristic‐Based Clustering, Dynamic Link Duration Clustering, and mobility‐based clustering algorithms. In this paper, we proposed a hybrid hierarchical clustering algorithm (HCAL) for large‐scale ad hoc networks (LMANET) and a protocol for hierarchical routing related to it (HCAL‐R) based on the cost metric in the forms of the link expiration time and node's relative degree. Remarkable features of the HCAL algorithm are that (1) its implementation is distributed over the available mobile nodes and (2) it is capable to adapt to the (possibly, complex) network size with the high‐speed nodes over the LMANET. Both these features are attained by equipping each routing path by a cost metric function in cluster head (CH) election that acquires context information by the environment (eg, current state of the CHs and the keeping time of the CHs).
Feature weighting is a technique used to approximate the optimal degree of influence of individual features. This paper presents a feature weighting method for Document Image Retrieval System (DIRS) based on keyword spotting. In this method, we weight the features using Weighted Principal Component Analysis (PCA). The purpose of PCA is to reduce the dimensionality of the data space to the smaller intrinsic dimensionality of feature space (independent variables), which are needed to describe the data economically. This is the case when there is a strong correlation between variables. The aim of this paper is to show feature weighting effect on increasing the performance of DIRS. After applying the feature weighting method to DIRS the average precision is 92.1% and average recall become 97.7% respectively.
A P2P grid is a special case of grid networks in which P2P communications are used for communication between nodes and trust management. This technology allows creation of a network with greater distribution and scalability. Semantic grids have appeared as an expansion of grid networks in which rich resource metadata are revealed and clearly handled. In a semantic P2P grid, nodes are clustered into different groups based on the semantic similarities between their services. This paper proposes a reputation model for trust management in a semantic P2P grid. We use fuzzy theory, in a trust overlay network named FR TRUST that models the network structure and the storage of reputation information. We present a reputation collection and computation system for semantic P2P grids. The system uses fuzzy theory to compute a peer trust level, which can be either: low, medium, or high. Experimental results demonstrate that FR TRUST combines desirable good computational complexity with high-ranking accuracy.
We consider the problem of evaluating the performance of a 5G network based on reusable components, called Reusable Functional Blocks (RFBs), proposed by the Horizon 2020 SUPERFLUIDITY project. RFBs allow a high level of flexibility, agility, portability and high performance. After formally modelling the RFB entities and the network physical nodes, we optimally formulate the problem of maximizing different Key Performance Indicators (KPIs) on an RFB-based network architecture, in which the RFBs are shared among the nodes, and deployed only where and when they are really needed. Our results, obtained by solving the proposed optimization problem over a simple yet representative scenario, show that the network can be managed in a very efficient way. More in depth, the RFBs are placed into the nodes in accordance with the amount of requested traffic from users and the specific pursued KPI, e.g., maximization of user throughput or minimization of the number of used nodes. Moreover, we evaluate the relationship between the capacity of each node and the number of RFBs deployed on it.
Open Radio Access Networks (O-RANs) have revolutionized the telecom ecosystem by bringing intelligence into disaggregated RAN and implementing functionalities as Virtual Network Functions (VNF) through open interfaces. However, dynamic traffic conditions in real-life O-RAN environments may require necessary VNF reconfigurations during run-time, which introduce additional overhead costs and traffic instability. To address this challenge, we propose a multi-objective optimization problem that minimizes VNF computational costs and overhead of periodical reconfigurations simultaneously. Our solution uses constrained combinatorial optimization with deep reinforcement learning, where an agent minimizes a penalized cost function calculated by the proposed optimization problem. The evaluation of our proposed solution demonstrates significant enhancements, achieving up to 76% reduction in VNF reconfiguration overhead, with only a slight increase of up to 23% in computational costs. In addition, when compared to the most robust O-RAN system that doesn't require VNF reconfigurations, which is Centralized RAN (C-RAN), our solution offers up to 76% savings in bandwidth while showing up to 27% overprovisioning of CPU.
—In this paper, we propose an intelligent reflecting surface (IRS) enabled wireless powered caching system. In the proposed IRS model, a power station (PS) provides wireless energy to multiple Internet of Things (IoT) devices, delivering their information to an access point (AP) by utilizing the harvested power. The AP, equipped with a local cache, stores the IoT data to avoid waking up the IoT devices frequently. Meanwhile, we deploy the IRS involving in the wireless energy and information transfer process for performance enhancements. In this practical system, the PS and the AP could belong to different service providers. Also, the AP requires to incentivize the PS to offer a provisional energy service. We model the interaction between the PS and the AP as a Stackelberg game that jointly optimizes the transmit power of the PS, the energy price, the phase shifts of the wireless energy transfer (WET) and wireless information transfer (WIT) phases, as well as wireless caching strategies of the AP. In this way, we first derive the optimal solutions of the phase shifts and the transmit power of the PS in closed-form. We propose an alternating optimization (AO) algorithm to optimize the wireless caching strategies and the energy price iteratively. Finally, we present various numerical evaluations to validate the beneficial role of the IRS and the wireless caching strategies and the performance of the proposed scheme compared with the existing benchmark schemes.
The detection and prevention of cyber-attacks is one of the main challenges in Vehicle-to-Everything (V2X) autonomous platooning scenarios. A key tool in this activity is the measurement report that is generated by User Equipment (UE), containing received signal strength and location information. Such data is effective in techniques to detect Rogue Base Stations (RBS) or Subscription Permanent Identifier SUPI/5G-GUTI catchers. An undetected RBS could result in unwanted consequences such as Denial of Service (DoS) attacks and subscriber privacy attacks on the network and UE. Motivated by this, this paper presents the novel simulation of a 5G cellular system to generate a realistic dataset of signal strength measurements that can later be used in the development of techniques to identify and prevent RBS interventions. The results show that the tool can create a large dataset of realistic measurement reports which can be used to develop and validate RBS detection techniques.
With increased wireless connectivity and embedded sensors, vehicles are becoming more intelligent, offering Internet access, telematics, and advanced driver assistance systems. Along with all benefits, connectivity to the public network and automotive control systems introduces new threats and security risks to connected and autonomous driving systems. Therefore, it is highly critical to design robust security mechanisms to protect the system from potential attacks and security vulnerabilities. An intrusion detection system (IDS) is a promising solution to detect and identify attacks and malicious behaviour within the network. This paper proposes a two-layer IDS mechanism that exploits machine learning (ML) solutions for collaborative attack detection between an on-vehicle IDS module and a developed IDS platform at a mobile edge computing (MEC) server. The results illustrate that the proposed solution can significantly reduce communication latency and energy consumption up to 80% while maintaining a high level of detection accuracy.
Mobile solutions give businesses a unique opportunity to re-think the way they interact with customers, employees and partners. We propose a new method called rapid way application on the mobile devices to handle and control the connection of the mobiles with GPS while mobile devices or sensor has mobility. This paper explores the relationship between the five depends and independent variable on Rapid Way mobile application such as Perceived usefulness, perceived ease of use, time saving, cost saving and intention to use Rapid Way application in Wireless sensor network for managing the sensors. A sample of 100 respondents is selected using a purposive sampling method whereby all segments of society to be included in the survey. A structured, self-administered as hard copy questionnaire is used to find data from these respondents. The findings indicate that the perceived usefulness (β = 0.307, p
Smart Grid (SG) is the revolutionised power network characterised by a bidirectional flow of energy and information between customers and suppliers. The integration of power networks with information and communication technologies enables pervasive control, automation and connectivity from the energy generation power plants to the consumption level. However, the development of wireless communications, the increased level of autonomy, and the growing sofwarisation and virtualisation trends have expanded the attack susceptibility and threat surface of SGs. Besides, with the real-time information flow, and online energy consumption controlling systems, customers' privacy and preserving their confidential data in SG is critical to be addressed. In order to prevent potential attacks and vulnerabilities in evolving power networks, the need for additional studying security and privacy mechanisms is reinforced. In addition, recently, there has been an ever-increasing use of machine intelligence and Machine Learning (ML) algorithms in different components of SG. ML models are currently the mainstream for attack detection and threat analysis. However, despite these algorithms' high accuracy and reliability, ML systems are also vulnerable to a group of malicious activities called adversarial ML (AML) attacks. Throughout this paper, we survey and discuss new findings and developments in existing security issues and privacy breaches associated with the SG and the introduction of novel threats embedded within power systems due to the development of ML-based applications. Our survey builds multiple taxonomies and tables to express the relationships of various variables in the field. Our final section identifies the implications of emerging technologies, future communication systems, and advanced industries on the security and privacy issues of SG.
Intrusion Detection Systems (IDSs) can identify the malicious activities and anomalies in networks and present robust protection for these systems. Clustering of attacks plays an important role in defining IDS defense policies. A key challenge in clustering has been finding the optimal value for the number of clusters. In this paper, we propose an automatic clustering algorithm as part of an IDS architecture. This algorithm is based on concepts of coherence and separation. Our automatic clustering algorithms find clusters with the most similarity between the proposed cluster elements and the least similarity with other clusters. The proposed clustering is further optimized by considering two types of objective index functions, and Artificial Bee Colony (ABC), Particle Swarm Optimization (PSO), and Differential Evolution (DE) methods. Comparison of the results obtained with other work in the literature shows improvements in terms of the low average number of evaluations functions, high accuracy, and low computation cost.
Open Radio Access Network (O-RAN) improves the flexibility and programmability of the 5G network by applying the Software-Defined Network (SDN) principles. O-RAN defines a near-real time Radio Intelligent Controller (RIC) to decouple the RAN functionalities into the control and user planes. Although the O-RAN security group offers several countermeasures against threats, RIC is still prone to attacks. In this letter, we introduce a novel attack, named Bearer Migration Poisoning (BMP), that misleads the RIC into triggering a malicious bearer migration procedure. The adversary aims to change the user plane traffic path and causes significant network anomalies such as routing blackholes. BMP has a remarkable feature that even a weak adversary with only two compromised hosts could launch the attack without compromising the RIC, RAN components, or applications. Based on our numerical results, the attack imposes a dramatic increase in signalling cost by approximately 10 times. Our experiment results show that the attack significantly degrades the downlink and uplink throughput to nearly 0 Mbps, seriously impacting the service quality and end-user experience.
Current technological advancements in Software Defined Networks (SDN) can provide efficient solutions for smart grids (SGs). An SDN-based SG promises to enhance the efficiency, reliability and sustainability of the communication network. However, new security breaches can be introduced with this adaptation. A layer of defence against insider attacks can be established using machine learning based intrusion detection system (IDS) located on the SDN application layer. Conventional centralised practises, violate the user data privacy aspect, thus distributed or collaborative approaches can be adapted so that attacks can be detected and actions can be taken. This paper proposes a new SDN-based SG architecture, highlighting the existence of IDSs in the SDN application layer. We implemented a new smart meter (SM) collaborative intrusion detection system (SM-IDS), by adapting the split learning methodology. Finally, a comparison of a federated learning and split learning neighbourhood area network (NAN) IDS was made. Numerical results showed, a five class classification accuracy of over 80.3% and F1-score 78.9 for a SM-IDS adapting the split learning technique. Also, the split learning NAN-IDS exhibit an accuracy of over 81.1% and F1-score 79.9.
In this paper, a primary-secondary resource-management controller on vehicular networks is designed and tested. We formulate the resource-management problem as a constrained stochastic network utility maximization problem and derive the optimal resource management controller, which dynamically allocates the access time-windows to the secondary-users. We provide the optimal steady-state controllers under hard and soft primary-secondary collision constraints, showing as the hard controller does not present any optimality gap in the average utility respect to the soft one, while, on the contrary, it is able to make the outage-probability vanishing. Then, we present as a particular case the subset of memoryless controller, that are unable to exploit the system statistics, derive the throughput-gain of the general controllers with respect to the memoryless ones and discuss conditions of applicability and advantages of each subclass.
Peer-to-peer (P2P) networks are gaining increased attention from both the scientific community and the larger Internet user community. Data retrieval algorithms lie at the center of P2P networks, and this paper addresses the problem of efficiently searching for files in unstructured P2P systems. We propose an Improved Adaptive Probabilistic Search (IAPS) algorithm that is fully distributed and bandwidth efficient. IAPS uses ant-colony optimization and takes file types into consideration in order to search for file container nodes with a high probability of success. We have performed extensive simulations to study the performance of IAPS, and we compare it with the Random Walk and Adaptive Probabilistic Search algorithms. Our experimental results show that IAPS achieves high success rates, high response rates, and significant message reduction.
In this paper, we propose a traffic engineering-based adaptive approach to dynamically reconfigure the computing-plus-communication resources of networked data centers which support in real-time the service requirements of mobile clients connected by TCP/IP energy-limited wireless backbones. The goal is to maximize the energy-efficiency, while meeting hard QoS requirements on the delivered transmission rate and processing delay. In order to cope with the (possibly, unpredictable) fluctuations of the offered workload, the proposed optimal cross-layer resource controller is adaptive. It jointly performs: i) the balanced control and dispatching of the admitted workload; ii) the dynamic reconfiguration of the Virtual Machines (VMs) instantiated onto the parallel computing platform at the data center; and iii) the rate control of the traffic injected into the wireless backbone for delivering the service to the requiring clients. Our experimental results show that the proposed technique improves energy consumption of servers by 25% compared to state of the art improvement on average in the entire data center.
In Cloud computing environments, computing resources are available for users, and they only pay for used resources The most important issues in cloud computing are scheduling and energy consumption which many researchers worked on them. In these systems a scheduling mechanism has two phases: task prioritization and processor selection. Different priorities may cause to different makespan and for each processor which assigned to the task, the energy consumption is different. So a good scheduling algorithm must assign priority to each task and select the best processor for them, in such a way that makespan and energy consumption be minimized. In this paper, we proposed a two phase's algorithm for scheduling, named TETS, the first phase is task prioritization and the second phase is processor assignment. We use three prioritization methods for prioritize the tasks and produce optimized initial chromosomes and assign the tasks to processors which is an energy-aware model. Simulation results indicate that our algorithm is better than previous algorithms in terms of energy consumption and makespan. It can improve the energy consumption by 20 % and makespan by 4 %.
Vehicle routing is considered the basic issue in distribution management. In real-world problems, customer demand for some commodities increases on special situations. On the one hand, one of the factors that are very important for customers is the timely delivery of the demanded commodities. In this research, customers had several different kinds of demands. Therefore, a new routing model was introduced in the form of integer linear programming by combining the concepts of time windows and multiple demands and by considering the two contradictory goals of minimizing travel cost and maximizing demand coverage. Moreover, two approaches were designed for the problem-solving model based on the NSGA-II algorithm with diversification of the mutation operator structure. The two criteria of spread and coverage of non-dominated solutions were used to compare algorithms. Study of some typical created problems indicated the validity of the model and the computational efficiency of the proposed algorithm. The proposed algorithm could increase the criterion of solution spread by about 10%, and increased the number of obtained solutions on the Pareto border compared to other algorithms, which indicated its high efficiency.
Because of the powerful computing and storage capability in cloud computing, machine learning as a service (MLaaS) has recently been valued by the organizations for machine learning training over some related representative datasets. When these datasets are collected from different organizations and have different distributions, multi-task learning (MTL) is usually used to improve the generalization performance by scheduling the related training tasks into the virtual machines in MLaaS and transferring the related knowledge between those tasks. However, because of concerns about privacy breaches (e.g., property inference attack and model inverse attack), organizations cannot directly outsource their training data to MLaaS or share their extracted knowledge in plaintext, especially the organizations in sensitive domains. In this article, we propose a novel privacy-preserving mechanism for distributed MTL, namely NOInfer, to allow several task nodes to train the model locally and transfer their shared knowledge privately. Specifically, we construct a single-server architecture to achieve the private MTL, which protects task nodes’ local data even if n-1 out of n nodes colluded. Then, a new protocol for the Alternating Direction Method of Multipliers (ADMM) is designed to perform the privacy-preserving model training, which resists the inference attack through the intermediate results and ensures that the training efficiency is independent of the number of training samples. When releasing the trained model, we also design a differentially private model releasing mechanism to resist the membership inference attack. Furthermore, we analyze the privacy preservation and efficiency of NOInfer in theory. Finally, we evaluate our NOInfer over two testing datasets and evaluation results demonstrate that NOInfer efficiently and effectively achieves the distributed MTL.
Data Dissemination is the distribution of data/statistics to the end users. With the adoption of Internet of Drones (IoD) environment for data dissemination, an efficient scheme is proposed which provides data integrity, identity anonymity, authentication, authorization, accountability (AAA) to the system model. We propose a system model having Ethereum based public blockchain distributed network in order to secure drone communication for the data collection and transmission. The proposed model provides secure communication between the drones and the users in a decentralized way. In this paper, blockchain technology is used for the storage of collected data from the drones and update the information into the distributed ledgers to reduce the burden of drones. It also provides integrity, authentication, and authorization to the collected data by the drones in the system model. Motivated by this consideration, the goal of this paper is threefold. First, we select a forger node from the number of drones. Second, we create blocks and validate their processes. Third, we provide secure data dissemination by applying Proof-of-Stake consensus mechanism. Afterward, we evaluate the security of the presented system model compared against the corresponding ones of some state-of-the-art in terms of communication time/cost. The results confirm that our system model is reliable and scalable for data dissemination in the IoD environment.
One of the important and challenging matters in sensor network is energy of life span of nodes in the network. Directed routing algorithm is one of propounded methods in sensor networks which are a data-oriented algorithm. This algorithm focuses on saving energy within life span of network nodes. One of problems of directed diffusion method is existence of multiple routes. Now, consider that some sinks from the same origin request the same data who's Data Volume is very much. Directed routing algorithm establishes one route toward targeted route for each query. The problem of this algorithm is multiplicity of routes for the same data. Therefore, if we can establish a route which has the most common feature with regards to nodes which forms the route, we have prevented wasting energy. In this paper, it is tried to remove problem of multiplicity of routes for the same data by learning automata. We named this algorithm RDDLA. RDDLA decrease overhead and energy in the network Considerable against with some others methods.
In this paper with the aid of genetic algorithm and fuzzy theory, we present a hybrid job scheduling approach, which considers the load balancing of the system and reduces total execution time and execution cost. We try to modify the standard Genetic algorithm and to reduce the iteration of creating population with the aid of fuzzy theory. The main goal of this research is to assign the jobs to the resources with considering the VM MIPS and length of jobs. The new algorithm assigns the jobs to the resources with considering the job length and resources capacities. We evaluate the performance of our approach with some famous cloud scheduling models. The results of the experiments show the efficiency of the proposed approach in term of execution time, execution cost and average Degree of Imbalance (DI).
In this paper, we develop the optimal minimum-energy scheduler for the dynamic online joint allocation of the task sizes, computing rates, communication rates and communication powers in virtualized Networked Data Centers (NetDCs) that operates under hard per-job delay-constraints. The referred NetDC's infrastructure is composed by multiple frequency-scalable Virtual Machines (VMs), that are interconnected by a bandwidth and power-limited switched Local Area Network (LAN). Due to the nonlinear power-vs.-communication rate relationship, the resulting Computing-Communication Optimization Problem (CCOP) is inherently nonconvex. In order to analytically compute the exact solution of the CCOP, we develop a solving approach that relies on the following two main steps: (i) we prove that the CCOP retains a loosely coupled structure, that allows us to perform the loss-less decomposition of the CCOP into the cascade of two simpler sub-problems; and, (ii) we prove that the coupling between the aforementioned sub-problems is provided by a (scalar) constraint, that is linear in the offered workload. The resulting optimal scheduler is amenable of scalable and distributed online implementation and its analytical characterization is in closed-form. After numerically testing its actual performance under randomly time-varying synthetically generated and real-world measured workload traces, we compare the obtained performance with the corresponding ones of some state-of-the-art static and sequential schedulers. (C) 2013 Published by Elsevier B.V.
Authentication protocols are powerful tools to ensure confidentiality as an important feature of Internet of Things (IoT). The Denial-of-Service (DoS) attack is one of the significant threats to availability , as another essential feature of IoT, which deprives users of services by consuming the energy of IoT nodes. On the other hand, computational intelligence algorithms can be applied to solve such issues in the network and cyber domains. Motivated by this, this article links these concepts. To do so, we analyze two lightweight authentication protocols, present a DoS attack inspired by users' misbehavior and suggest a solution called received signal strength, which is easy to compute, applicable for resisting against different kinds of vulnerabilities in Internet protocols, and feasible for practical implementations. We implement it on two scenarios for locating attackers, investigate the effects of IoT devices' internal error on locating, and propose an optimization problem to finding the exact location of attackers, which is efficiently solvable for computational intelligence algorithms, such as TLBO. Besides, we analyze the solutions for unreliable results of accurate devices and provide a solution to detect attackers with less than 12-cm error and the false alarm probability of 0.7%.
Service Function Chaining (SFC) is a service deployment concept that promises cost efficiency and increases flexibility for computer networks. On the other hand, Software Defined Networking (SDN) provides a powerful infrastructure to implement SFC. In this paper, we mathematically formulate the SFC problem in SDN-based networks. In this way, the energy consumption of the network is minimized while the traffic congestion is controlled through network reconfiguration. Additionally, a low complex heuristic algorithm is proposed to find a near-optimal solution for the mentioned problem. Simulation results show that the proposed heuristic reconfigures the network in a way that the energy consumption is near-optimal while the SFC requirements are met. Besides, the computational complexity is very low which makes it applicable for real-world networks.
Fog computing is a novel, decentralized and heterogeneous computing environment that extends the traditional cloud computing systems by facilitating task processing near end‐users on computing resources called fog nodes. These diverse and resource‐constrained fog devices process a large volume of tasks generated by various fog applications. These tasks are generated by various applications, some of which may be latency‐sensitive, while others may tolerate some degree of delay in their normal functions. Task scheduling determines when a task should be allocated to a computing resource and how long that task can occupy the assigned resource. The majority of task scheduling algorithms focus on prioritizing the latency‐sensitive tasks only, which results in the long waiting time for the other type of tasks. Hence, these priority‐based schedulers cause task starvation for less important tasks while achieving delay‐optimal results for latency‐sensitive tasks. As a result, in this paper, we propose MQP, a multi‐queue priority‐based preemptive task scheduling approach that achieves a balanced task allocation for those applications that can tolerate a certain amount of processing delay and the latency‐sensitive fog applications. At run‐time, the MQP algorithm categorizes tasks as short and long based on their burst time. MQP algorithm maintains a separate task queue for each task category and dynamically updates the time slot value for preemption. The proposed technique's major purpose is to reduce response time for those data‐intensive applications in the fog computing environment, which include both latency‐sensitive tasks and tasks which are less latency‐sensitive, thereby addressing the starvation problem for less latency‐sensitive tasks. A smart traffic management case study is created to model a scenario with both latency‐sensitive short and less latency‐sensitive long tasks. We implement the MQP algorithm using iFogSim and confirm that it reduces the service latencies for long tasks. Simulation results show that the MQP algorithm allocates tasks to a fog device more efficiently and reduces the service latencies for long tasks. The average value of percentage reduction in the latency across all experimental configurations achieved is 22.68% and 38.45% in comparison to First Come‐First Serve and shortest job first algorithms.
In this article an algorithm is presented for edges coloring of the graph. In this algorithm the node that we discuss represented by an Agent and then all the nodes of a graph like multi-agent system. Each node independently colours its edges with respect to cellular automata using distributed and paralleling of the process. Innovation of this method is on distributing of the process for each node so that each node updates its edges color only with the use of its neighbors in some steps until all graphs are colored completely. At the end the results are being tested on some Standard graphs and the results are presented. In this method, each graph is colored using one rule repetition for each node with high speed on its edges.
In this work, we deploy a one-day-ahead prediction algorithm using a deep neural network for a fast-response BESS in an intelligent energy management system (I-EMS) that is called SIEMS. The main role of the SIEMS is to maintain the state of charge at high rates based on the one-day-ahead information about solar power, which depends on meteorological conditions. The remaining power is supplied by the main grid for sustained power streaming between BESS and end-users. Considering the usage of information and communication technology components in the microgrids, the main objective of this paper is focused on the hybrid microgrid performance under cyber-physical security adversarial attacks. Fast gradient sign, basic iterative, and DeepFool methods, which are investigated for the first time in power systems e.g. smart grid and microgrids, in order to produce perturbation for training data.
In this research a two dimensional, single phase, and isothermal model is developed to investigate the effects of eccentric catheterization on blood flow characteristics in a tapered and stenosis artery which is complex system. The model conducted by assuming that the blood is as Newtonian and incompressible fluid and the temperature effects are also neglected. The results clearly show that the axial velocity and the magnitude of the wall shear stress distribution are higher for eccentric catheter than that for concentric one. Also, the resistance impedance gives the reverse trend of the wall shear stress with respect to the taper angle where blood can flow freely through diverging vessel but in the case of eccentric catheter is less than that of the concentric one when the radius of catheter is considered. In addition, the trapping appears near the wall of catheter and the trapped bolus increases in size as the radius of catheter increases.
The Multiple Traveling Salesmen Problem (mTSP) is of the famous and classical problems of research in operations and is accounted as one of the most famous and widely used problems of combinational optimization. Most of the complex problems can be modeled as the mTSP and then be solved. The mTSP is a NP-Complete one; therefore, it is not possible to use the exact algorithms for solving it instead the heuristics methods are often applied for solving such problems. In this paper, a new hybrid algorithm, called GELS-GA, has been presented for solving the mTSP. The utility of GELS-GA is compared with some related works such as GA and ACO and achieves optimality even in highly complex scenarios. Although, the proposed algorithm is simple, it includes an appropriate time of completion and the least traversed distance among existing algorithms.
Designers of smart environments based on radio frequency identification devices have a challenging task to build secure mutual authentication protocols. These systems are classified into two major factions which are traditional closed-loop systems, and open-loop systems. To the best of our knowledge, all of the mutual authentication protocols previously introduced for these two categories rely on a centralized database but they fail to address decentralized mutual authentication and their related attacks. Thanks to the blockchain technology, which is a novel distributed technology, in this paper, we propose two decentralized mutual authentication protocols for IoT systems. Our first scheme is utilized for traditional closed-loop RFID systems (called CLAB), and the second one applies to open-loop RFID systems (called OLAB). Meanwhile, we examine the security of the Chebyshev chaotic map-based authentication algorithm and confirm that this algorithm is unprotected against tag and reader impersonation attacks. Likewise, we present a denial of service (DoS), tag impersonation, and reader impersonation attacks against the Chebyshev chaotic-map based protocol when employed in open-loop IoT networks. Moreover, we discover a full secret recovery attack against a recent RFID mutual authentication protocol which is based on blockchain. Finally, we use the BAN-logic method to approve the security characteristics of our CLAB and OLAB proposals.
The utilization of the Internet of Things (IoT) has burst in recent years. Fog computing is a notion that solves cloud computing’s limitations by offering low latency to IoT network user applications. However, the significant number of networked IoT devices, the large scale of the IoT, security concerns, users’ critical data, and heterogeneity in this extensive network significantly complicate the implementation. The IoT-Fog architecture consists of fog devices (servers) at the fog layer, which decreases network utilization and response time due to their closeness to IoT devices. However, as the number of IoT and fog devices under the IoT-Fog architecture grows, new security concerns and requirements emerge. Because incorporating fog computing into IoT networks introduces some vulnerabilities to IoT-Fog networks, the nodes in the fog layer are the target of security threats. Software-Defined Networking (SDN) is a novel paradigm that decouples the data plane from control plane, resulting in better programmability and manageability. Attack defense mechanisms can be implemented in the IoT-Fog network without SDN. But SDN paradigm provides the IoT-Fog with some characteristics that facilitate counterattacks. This survey briefly explains some works that utilized the SDN features in the IoT-Fog network for security threats in the IoT-Oriented fog layer. To this end, we examine IoT-Fog, SDN, and SDN-based IoT-Fog networks. We describe security threats in IoT-Fog networks and briefly explain the vulnerabilities and attacks in the fog layer. Then, we describe the fog layer’s most common IoT-Fog security defense mechanisms. Following that, we present the SDN features, explore how SDN can help defensive mechanisms in IoT-Fog networks, and categorize the works based on the SDN features they use. We explain their features and present a comparison between them. Finally, we discuss the disadvantages of SDN in IoT-Fog networks.
Conference Title: 2021 20th International Conference on Ubiquitous Computing and Communications (IUCC/CIT/DSCI/SmartCNS) Conference Start Date: 2021, Dec. 20 Conference End Date: 2021, Dec. 22 Conference Location: London, United KingdomPresents the introductory welcome message from the conference proceedings. May include the conference officers' congratulations to all involved with the conference event and publication of the proceedings record.
Internet of Things (IoT) holds great promise for many life-improving applications like health-care systems. In IoT systems, providing secure authentication and key agreement scheme that considers compromised entities is an important issue. State-of-the-arts tackle this problem, but they fail to address compromised entity attack and have high computation cost. Motivated by these considerations, in this paper, we propose an energy-efficient proactive authentication and key agreement scheme called PAKIT for IoT systems. The security of PAKIT scheme is validated using the ProVerif tool. Moreover, the efficiency of PAKIT is compared with the predecessor schemes proposed for IoT systems. The results of the experiments show that PAKIT is efficient and suitable for real-world IoT applications by utilizing lightweight functions, such as hash and XOR.
Selected procedures in [1] and additional simulation results are presented in detail in this report. We first present the IoT device registration in Section I, and we provide the details of fuzzy-based trust computation in Section II. In the end, we show some additional simulation results for formal validation of the Light-Edge under On-the-Fly Model Checker (OFMC) and Constraint-Logic-based ATtack SEarcher (CLAtse) tools in Section III. See the original paper [1] for more detail.
This book is the result of years of research and redrafting based on materials in the libraries of the Hoover Institution (Stanford), the British Museum and in particular the Bibliothèque de Documentation et Information Contemporaine (BDIC, Paris Nanterre).During the initial research I was fortunate to have received comments on my drafts from Professors B. Ward, G. Grossman and M. Lewin. In the final stages, the stimulating remarks of Professors R.W. Davies, O. Crisp, L. Szamuely and L. Sire were of considerable help. The initial English editing was undertaken with much sensitivity and respect for the content by Mr Jacob Miller, without whose aid and enthusiasm the script would never have been ready for printing.My gratitude goes also to Dr J.M. Cooper and Mrs Bo Richter, who have in different degrees but with the same spirit of friendship helped me in the editing of the final version. In this they displayed much patience and a cheerful disregard for my intermittent objections to their justified corrections. I am also indebted to Dr Cooper for bringing my attention to additional sources and information contained in his remarkable collection of early Soviet literature.I wish to express my thanks to all these scholars and at the same time to apologize for any weaknesses which may remain; I assume full responsibility for them.Miss Jean Fyfe performed the valuable task of decoding my illegible handwriting and translating it into a neatly typed text.
In a critical infrastructure such as Smart Grid (SG), providing security of the system and privacy of consumers are significant challenges to be considered. The SG developers adopt Machine Learning (ML) algorithms within the Intrusion Detection System (IDS) to monitor traffic data and network performance. This visibility safeguards the SG from possible intrusions or attacks that may trigger the system. However, it requires access to residents' consumption information which is a severe threat to their privacy. In this paper, we present a novel method to detect abnormalities on a large scale SG while preserving the privacy of users. We design a Federated IDS (FIDS) architecture using Federated Learning (FL) in a 5G environment for the SG metering network. In this way, we design Federated Deep Neural Network (FDNN) model that protects customers' information and provides supervisory management for the whole energy distribution network. Simulation results for a real-time dataset demonstrate the reasonable improvement of the proposed FDNN model compared with the state-of-the-art algorithms. The FDNN achieves approximately 99.5% accuracy, 99.5% precision/recall, and 99.5% f1-score when comparing with classification algorithms.
Wireless interfaces, remote control schemes, and increased autonomy have raised the attacks surface of vehicular networks. As powerful monitoring entities, intrusion detection systems (IDS) must be updated and customised to respond to emerging networks' requirements. As server-based monitoring schemes were prone to significant privacy concerns, new privacy constrained learning methods such as federated learning (FL) have received considerable attention in designing IDS. However, to alleviate the efficiency and enhance the scalability of the original FL, this paper proposes a novel collaborative hierarchical federated IDS, named CHFL for the vehicular network. In the CHFL model, a group of vehicles assisted by vehicle-to-everything (V2X) communication technologies can exchange intrusion detection information collaboratively in a private format. Each group nominates a leader, and the leading vehicle serves as the intermediate in the second level detection system of the hierarchical federated model. The leader communicates directly with the server to transmit and receive model updates of its nearby end vehicles. By reducing the number of direct communications to the server, our proposed system reduces network uplink traffic and queuing-processing latency. In addition, CHFL improved the prediction loss and the accuracy of the whole system. We are achieving an accuracy of 99.10% compared with 97.01% accuracy of the original FL.
This study addresses the need for developing new frameworks to monitor and detect sensor failures in connected commercial vehicles (CCV)s. The CCV's sensor health is more important when performance predictions and other communication-related errors (e.g. cyber-physical attacks) can manipulate the sensory network's resiliency. We developed a novel machine learning (ML)-based framework, AutoDetect, to equip the cloud-tied operators with tools for understanding the abnormal sensor data streaming from the vehicle on the cloud level which explains the sensor data errors due to sensor failures only. We developed an innovative autoencoder (AE) neural network algorithm coupled with K-means clustering to create patterns. To learn the relationship between operating samples and features, when streaming sensor data over high-dimensional datasets is collected in the United Kingdom (UK). Different profiles of sensor data are collected under various driving conditions to monitor the ground truth of the sensor's confidence levels in CCVs. The new AutoDetect tracked real-time sensor failures with a minimum accuracy of 90%.
The book aims to promote high-quality research by bringing together researchers and experts in cyber-physical system security and privacy from around the world to share their knowledge of the different aspects of CPS security and we have integrated these chapters into a comprehensive book. This book will present the state of the art and state of practice on how to address the following unique challenges in cybersecurity and privacy in CPS. This book is ideally suited for policymakers, Industrial Engineers, researchers, academics and professionals seeking a thorough understanding of the principles of security and privacy in Cyber-physical Systems. They will learn about promising solutions to these research problems and identify an unresolved and challenging problem for their own research. Readers of this book will have an overview of CPS cyber security and privacy design.
Cloud Data Centers (CDCs) have become a vital computing infrastructure for enterprises. However, CDCs consume substantial energy due to the increased demand for computing power, especially for the Internet of Things (IoT) applications. Although a great deal of research in green resource allocation algorithms have been proposed to reduce the energy consumption of the CDCs, existing approaches mostly focus on minimizing the number of active Physical Machines (PMs) and rarely address the issue of load fluctuation and energy efficiency of the Virtual Machine (VM) provisions jointly. Moreover, existing approaches lack mechanisms to consider and redirect the incoming traffics to appropriate resources to optimize the Quality of Services (QoSs) provided by the CDCs. We propose a novel adaptive energy-aware VM allocation and deployment mechanism called AFED-EF for IoT applications to handle these problems. The proposed algorithm can efficiently handle the fluctuation of load and has good performance during the VM allocation and placement. We carried out extensive experimental analysis using a real-world workload based on more than a thousand PlanetLab VMs. The experimental results illustrate that AFED-EF outperforms other energy-aware algorithms in energy consumption, Service Level Agreements (SLA) violation, and energy efficiency.
Due to the ever-growing use of active Internet devices, the Internet has achieved good popularity at present. The smart devices could connect to the Internet and communicate together that shape the Internet of Things (IoT). Such smart devices are generating data and are connecting to each other through edge-cloud infrastructure. Authentication of the IoT devices plays a critical role in the success of the integration of IoT, edge, and cloud computing technologies. The complexity and attack resistance of the authentication protocols are still the main challenges. Motivated by this, this paper introduces a lightweight authentication protocol for IoT devices named Light-Edge using a three-layer scheme, including IoT device layer, trust center at the edge layer, and cloud service providers. The results show the superiority of the proposed protocol against other approaches in terms of attack resistance, communication cost, and time cost.
One of the current key challenges in wireless sensor networks is the development of routing protocols that provide stable cluster-head election, while prolonging network lifetime by saving energy. In this contribution, a new Stable Election Protocol (SEP), named New-SEP (N-SEP), is presented to prolong the stable period of Fog-supported sensor networks by maintaining balanced energy consumption. N-SEP takes into account some features of sensor nodes (e.g., distance from base station, network heterogeneity ratio, residual/consumed energy, distance between cluster heads (CHs)) in order to elect the best CHs. For this purpose, it exploits heterogeneous energy thresholds, in order to select CHs and prolong the time interval of the system. Simulation results support the capability of the proposed algorithm to maximize the network lifetime and preserve more energy as compared to the results obtained by using current heuristics, such as, Low Energy Adaptive Clustering Hierarchy (LEACH) and SEP protocols. Additionally, we found that N-SEP outperforms LEACH and SEP in prolonging the stability period of the network by 50% and 25%, respectively.
Recent advances in the context of Internet of Things (IoT) have led to the emergence of many useful IoT applications with different Quality of Service (QoS) requirements. The fog-cloud computing systems offer a promising environment to provision resources for IoT application services. However, providing an efficient solution to service placement problem in such systems is a critical challenge. To address this challenge, in this paper, we propose a QoS-aware service placement policy for fog-cloud computing systems that places the most delay-sensitive application services as closer to the clients as possible. We validate our proposed algorithm in the iFogSim simulator. Results demonstrate that our algorithm achieves significant improvement in terms of service latency and execution cost compared to simulators built-in policies.
5G and 6G networks are expected to support various novel emerging adaptive video streaming services (e.g., live, VoD, immersive media, and online gaming) with versatile Quality of Experience (QoE) requirements such as high bitrate, low latency, and sufficient reliability. It is widely agreed that these requirements can be satisfied by adopting emerging networking paradigms like Software-Defined Networking (SDN), Network Function Virtualization (NFV), and edge computing. Previous studies have leveraged these paradigms to present network-assisted video streaming frameworks, but mostly in isolation without devising chains of Virtualized Network Functions (VNFs) that consider the QoE requirements of various types of Multime-dia Services (MS). To bridge the aforementioned gaps, we first introduce a set of multimedia VNFs at the edge of an SDN-enabled network, form diverse Service Function Chains (SFCs) based on the QoE requirements of different MS services. We then propose SARENA, an _S_FC-enabled ArchitectuRe for adaptive VidEo StreamiNg Applications. Next, we formulate the problem as a central scheduling optimization model executed at the SDN controller. We also present a lightweight heuristic solution consisting of two phases that run on the SDN controller and edge servers to alleviate the time complexity of the optimization model in large-scale scenarios. Finally, we design a large-scale cloud-based testbed including 250 HTTP Adaptive Streaming (HAS) players requesting two popular MS applications (i.e., live and VoD), conduct various experiments, and compare its effectiveness with baseline systems. Experimental results illustrate that SARENA outperforms baseline schemes in terms of users' QoE by at least 39.6%, latency by 29.3%, and network utilization by 30% in both MS services.
The expected pervasive use of mobile cloud computing and the growing number of Internet data centers have brought forth many concerns, such as, energy costs and energy saving management of both data centers and mobile connections. Therefore, the need for adaptive and distributed resource allocation schedulers for minimizing the communication-plus-computing energy consumption has become increasingly important. In this paper, we propose and test an efficient dynamic resource provisioning scheduler that jointly minimizes computation and communication energy consumption, while guaranteeing user Quality of Service (QoS) constraints. We evaluate the performance of the proposed dynamic resource provisioning algorithm with respect to the execution time, goodput and bandwidth usage and compare the performance of the proposed scheduler against the exiting approaches. The attained experimental results show that the proposed dynamic resource provisioning algorithm achieves much higher energy-saving than the traditional schemes.
5G is expected to become the dominant technology in the forthcoming years. In this work, we consider a 5G Super-fluid network, as an outcome of the H2020 project SUPERFLUIDITY. The project exploits the concept of Reusable Functional Block (RFB), a virtual resource that can be deployed on top of 5G physical nodes. Specifically, we focus on the management of the RFBs in a Superfluid network to deliver a high definition video to the users. We design an efficient algorithm, called P5G, which is based on Particle Swarm Optimization (PSO). Our solution targets different Key Performance Indicators (KPIs), including the maximization of user throughput, or the minimization of the number of used 5G nodes. Results, obtained over a representative scenario, show that P5G is able to wisely manage the RFBs, while always guaranteeing a large throughput to the users.
The increasing popularity of Software-Defined Network technologies is shaping the characteristics of present and future data centers. This trend, leading to the advent of Software-Defined Data Centers, will have a major impact on the solutions to address the issue of reducing energy consumption in cloud systems. As we move towards a scenario where network is more flexible and supports virtualization and softwarization of its functions, energy management must take into account not just computation requirements but also network related effects, and must explicitly consider migrations throughout the infrastructure of Virtual Elements (VEs), that can be both Virtual Machines and Virtual Routers. Failing to do so is likely to result in a sub-optimal energy management in current cloud data centers, that will be even more evident in future SDDCs. In this chapter, we propose a joint computation-plus-communication model for VEs allocation that minimizes energy consumption in a cloud data center. The model contains a threefold contribution. First, we consider the data exchanged between VEs and we capture the different connections within the data center network. Second, we model the energy consumption due to VEs migrations considering both data transfer and computational overhead. Third, we propose a VEs allocation process that does not need to introduce and tune weight parameters to combine the two (often conflicting) goals of minimizing the number of powered-on servers and of avoiding too many VE migrations. A case study is presented to validate our proposal. We apply our model considering both computation and communication energy contributions even in the migration process, and we demonstrate that our proposal outperforms the existing alternatives for VEs allocation in terms of energy reduction.
In fog computing, end-users can offload the computation-intensive tasks to the fog node in the proximity. Additionally, the fog nodes also offload these tasks to the cloud and neighboring fog node to seek additional computational resources. In this paper, we propose an offloading strategy in fog computing to minimize the cost that is a weighted sum of energy consumption and total delay for the task processing per end-user. We take the heterogeneous nature of the fog computing nodes that have different CPU frequency to process the tasks. We aim to find an optimal amount of task data to be either locally processed or offloaded to the preferable fog node and the remote cloud under the energy and delay constraints. We then formulate the optimization problem into a non-convex quadratically constrained quadratic program. We further provide an efficient solution to this problem by semidefinite relaxation. Finally, our proposed offloading scheme is evaluated by the simulation to demonstrate the offloading profile and optimal cost of the offloading with a wide range of parameter settings.
In this paper, we develop the optimal minimum-energy scheduler for the adaptive joint allocation of the task sizes, computing rates, communication rates and communication powers in virtualized networked data centers (VNetDCs) that operate under hard per-job delay-constraints. The considered VNetDC platform works at the Middleware layer of the underlying protocol stack. It aims at supporting real-time stream service (such as, for example, the emerging big data stream computing (BDSC) services) by adopting the software-as-a-service (SaaS) computing model. Our objective is the minimization of the overall computing-plus-communication energy consumption. The main new contributions of the paper are the following ones: (i) the computing-plus-communication resources are jointly allotted in an adaptive fashion by accounting in real-time for both the (possibly, unpredictable) time fluctuations of the offered workload and the reconfiguration costs of the considered VNetDC platform; (ii) hard per-job delay-constraints on the overall allowed computing-plus-communication latencies are enforced; and, (iii) to deal with the inherently nonconvex nature of the resulting resource optimization problem, a novel solving approach is developed, that leads to the lossless decomposition of the afforded problem into the cascade of two simpler sub-problems. The sensitivity of the energy consumption of the proposed scheduler on the allowed processing latency, as well as the peak-to-mean ratio (PMR) and the correlation coefficient (i.e., the smoothness) of the offered workload is numerically tested under both synthetically generated and real-world workload traces. Finally, as an index of the attained energy efficiency, we compare the energy consumption of the proposed scheduler with the corresponding ones of some benchmark static, hybrid and sequential schedulers and numerically evaluate the resulting percent energy gaps.
Provisioning services for Internet of Things (IoT) devices leads to several challenges: heterogeneity of IoT devices, varying Quality of Services requirements, and increasing availability of both Cloud and Fog resources. The last of these is most significant to cope with Cloud infrastructure providers (CIPs) limitations for latency-sensitive services. Many Fog infrastructure providers (FIPs) have recently emerged, and their number is increasing continually. Selecting a suitable provider for each service involves considering multiple factors such as the provider's available resources, geographic location, quality of service, and cost. Motivated by this, FLEX is proposed in this work as a platform for service placement in a multi-Fog and multi-Cloud environment. For each service, FLEX broadcasts service requirements to the resource managers (RMs) of the available Fog and Cloud service providers and then selects the most suitable provider for that service. FLEX is scalable and flexible as it leaves it up to the RMs to have their own policy for the placement of submitted services. Also, the problem of service placement in multi-provider environments has been formulated as an optimization problem to jointly minimize the total weighted delay and cost of services. Next, a heuristic algorithm, namely minimum cost and delay first (MCD1), is proposed to map services to FIPs and CIPs efficiently.To evaluate the performance of the proposed algorithm, extensive experiments are conducted to analyze the behavior of the algorithm under different scenarios, such as a varying number of services, providers, and the ratio of FIPs. Results show that MCD1 significantly performs better than baseline methods and genetic algorithms. In particular, the proposed algorithm can reduce the objective function value up to 26.8%.
A web operating system is an operating system that users can access from any hardware at any location. A peer-to-peer (P2P) grid uses P2P communication for resource management and communication between nodes in a grid and manages resources locally in each cluster, and this provides a proper architecture for a web operating system. Use of semantic technology in web operating systems is an emerging field that improves the management and discovery of resources and services. In this paper, we propose PGSW-OS (P2P grid semantic Web OS), a model based on a P2P grid architecture and semantic technology to improve resource management in a web operating system through resource discovery with the aid of semantic features. Our approach integrates distributed hash tables (DHTs) and semantic overlay networks to enable semantic-based resource management by advertising resources in the DHT based upon their annotations to enable semantic-based resource matchmaking. Our model includes ontologies and virtual organizations. Our technique decreases the computational complexity of searching in a web operating system environment. We perform a simulation study using the Gridsim simulator, and our experiments show that our model provides enhanced utilization of resources, better search expressiveness, scalability, and precision.
One of the important and challenging matters in sensor network is energy of life span of nodes in the network. Node's movement, specifically movement of central node (sink) in these networks cause to increase routing updating overhead and consequently to increase power consumption and to decrease network life span. Directed Diffusion algorithm is one of methods in sensor network which is a data-oriented algorithm. One of the important definitions of basic algorithm is not supporting central node's movement. In case of movement of central node, data packs pass on unreliable rout toward central node. In fact, they pass a rout on which the central node is not present at the time being and it has moved to another place. Therefore, route of data is out of order and there is the need to build new routes. This problem causes to create lots of overhead and waste energy. In this article, it is tried to solve mentioned problem of central node's movement by learning automata. In suggested algorithm by learning automata a route amendment tree is built which prevents from creation of the whole route and its overhead.
Job scheduling is one of the most important research problems in distributed systems, particularly cloud environments/computing. The dynamic and heterogeneous nature of resources in such distributed systems makes optimum job scheduling a non-trivial task. Maximal resource utilization in cloud computing demands/necessitates an algorithm that allocates resources to jobs with optimal execution time and cost. The critical issue for job scheduling is assigning jobs to the most suitable resources, considering user preferences and requirements. In this paper, we present a hybrid approach called FUGE that is based on fuzzy theory and a genetic algorithm (GA) that aims to perform optimal load balancing considering execution time and cost. We modify the standard genetic algorithm (SGA) and use fuzzy theory to devise a fuzzy-based steady-state GA in order to improve SGA performance in term of makespan. In details, the FUGE algorithm assigns jobs to resources by considering virtual machine (VM) processing speed, VM memory, VM bandwidth, and the job lengths. We mathematically prove our optimization problem which is convex with well-known analytical conditions (specifically, Karush-Kuhn-Tucker conditions). We compare the performance of our approach to several other cloud scheduling models. The results of the experiments show the efficiency of the FUGE approach in terms of execution time, execution cost, and average degree of imbalance.
The Quality of Service (QoS) routing protocol plays a vital role in enabling a mobile network to interconnect wired networks with the QoS support. It has become quite a challenge in mobile networks, like mobile ad-hoc networks, to identify a path that fulfils the QoS requirements, regarding their topology and applications. The QoS routing feature can also function in a stand-alone multi hop mobile network for real-time applications. The chief aim of the QoS aware protocol is to find a route from the source to the destination that fulfils the QoS requirements. In this paper we present a new energy and delay aware routing method which combines Cellular automata (CA) with the Genetic algorithm (GA). Here, two QoS parameters are used for routing; energy and delay. The routing algorithm based on CA is used to identify a set of routes that can fulfill the delay constraints and then select a reasonably good one using GAs. The results of Simulation show that the method proposed produces a higher degree of performance than the AODV and another QoS method in terms of network lifetime and end-to-end delay.
Fog-Cloud computing has become a promising platform for executing Internet of Things (IoT) tasks with different requirements. Although the fog environment provides low latency due to its proximity to IoT devices, it suffers from resource constraints. This is vice versa for the cloud environment. Therefore, efficiently utilizing the fog-cloud resources for executing tasks offloaded from IoT devices is a fundamental issue. To cope with this, in this paper, we propose a novel scheduling algorithm in fog-cloud computing named PGA to optimize the multi-objective function that is a weighted sum of overall computation time, energy consumption, and percentage of deadline satisfied tasks (PDST). We take the different requirements of the tasks and the heterogeneous nature of the fog and cloud nodes. We propose a hybrid approach based on prioritizing tasks and a genetic algorithm to find a preferable computing node for each task. The extensive simulations evaluate our proposed algorithm to demonstrate its superiority over the state-of-the-art strategies.
Differential evolution (DE) algorithm is utilized to find an optimized solution in multidimensional real applications like 5G/6G networked devices and support unlimited connectivity for terrestrial networks due to high efficiency, robustness, and easy achievements. With the development of new emerging networks and the rise of big data, the DE algorithm encounters a series of challenges, such as the slow convergence rate in late iteration, strong parameter dependence, and easiness to fall into local optimum. These issues exponentially increase the energy and power consumption of communications and computing technologies in 5G/6G network like a networked data center. To address this and leverage a practical solution, this paper introduces IADE, an improved adaptive DE algorithm, to solve the problems mentioned earlier. IADE improves the scaling factor, crossover probability, variation, and selection strategy of the DE algorithm. In IADE, the parameters adaptively adjusted with the population's iterative evolution to meet the parameter's different requirements values of network steering traffic in each period. Numerous experiments are carried out through the benchmark function to evaluate the performance of IADE, and the results obtained from the experiment illustrate that IADE surpasses the benchmark algorithms in terms of solution accuracy and convergence speed for large tasks around 10%, respectively.
This special section solicits original research and practical contributions which advance the security and privacy of federated learning solutions for industrial IoT applications.
Portfolio optimization is a serious challenge for financial engineering and has pulled down special attention among investors. It has two objectives: to maximize the reward that is calculated by expected return and to minimize the risk. Variance has been considered as a risk measure. There are many constraints in the world that ultimately lead to a non-convex search space such as cardinality constraint. In conclusion, parametric quadratic programming could not be applied and it seems essential to apply multi-objective evolutionary algorithm (MOEA). In this paper, a new efficient multi-objective portfolio optimization algorithm called 2-phase NSGA II algorithm is developed and the results of this algorithm are compared with the NSGA II algorithm. It was found that 2-phase NSGA II significantly outperformed NSGA II algorithm.
Traditional Open Vehicle Routing Problem (OVRP) methods take account to definite responding to the all requests of customers whiles the main goal of proposed approach in OVRP is decreasing the vehicle numbers time and path traveled by vehicles. Therefore, in the present paper, a new optimization algorithm based on Gravity law and mass interactions is introduced to solve the problem. This algorithm being proposed based on random search concepts utilizes two of the four major parameters in physics including speed and Gravity and its researcher agents are a set of masses which are in connection with each other based on Newton’s Gravity and motion laws. The proposed approach is compared with various algorithms and results approve its high effectiveness in solving the above problem.
With the recent development in the Internet of Things (IoT), big data, and machine learning, the number of services has dramatically increased. These services are heterogeneous in terms of the amount of resources and quality of service (QoS) requirements. To cope with the limitations of Cloud infrastructure providers (CIPs) for latency-sensitive services, many Fog infrastructure providers (FIPs) have recently emerged and their numbers are increasing continually. Due to difficulties such as the different requirements of services, location of end-users, and profile cost of IPs, distributing services across multiple FIPs and CIPs has become a fundamental challenge. Motivated by this, a flexible and scalable platform, FLEX, is proposed in this work for the service placement problem (SPP) in multi-Fog and multi-Cloud computing. For each service, FLEX broadcasts the service's requirements to the resource managers (RMs) of all providers and then based on the RMs' responses, it selects the most suitable provider for that service. The proposed platform is flexible and scalable as it leaves it up to the RMs to have their own policy for service placement. The problem is formulated as an optimization problem and an efficient heuristic algorithm is proposed to solve it. Our simulation results show that the proposed algorithm can meet the requirements of services.
Computational offloading becomes an important and essential research issue for the delay-sensitive task completion at resource-constraint end-users. Fog computing that extends the computing and storage resources of the cloud computing to the network edge emerges as a potential solution towards low-latency task provisioning via computational offloading. In our offloading scenario, each end-user will first offload the task to its primary fog node. When the primary fog node cannot meet the tolerable latency, it has the possibility to offload to the cloud and/or assisting fog node to obtain extra computing resource to shorten the computing latency at the expense of additional transmission latency. Therefore, a trade-off needs to be carefully made in the offloading decision. At the same time, in addition to the task data from the end-users under its primary coverage, the primary fog node receives the tasks from other end-users via its neighbor fog nodes. Thus, to jointly optimize the computing and communication resources in the fog node, we formulate a delay-sensitive data offloading problem that mainly considers the local task execution delay and transmission delay. An approximate solution is obtained via Quadratically Constraint Quadratic Programming (QCQP). Finally, the extensive simulation results demonstrate the effectiveness of the proposed solution, while guaranteeing minimum end-to-end latency for various task processing densities and traffic intensity levels.
Offloading task to roadside units (RSUs) provides a promising solution for enhancing the real-time data processing capacity and reducing energy consumption of vehicles in the vehicular ad-hoc network (VANET). Recently, multi-agent deep reinforcement learning (MADRL)-based offloading approaches have been widely used for task offloading in VANET. However, existing MADRL-based approaches suffer from offloading preference inference (OPI) attack, which utilizes the vulnerability in the policy learning process of MADRL to mislead vehicles to offload tasks to malicious RSUs. In this paper, we first formulate a joint optimization of offloading action and transmitting power with the objective of minimizing the system cost, including local and edge costs, under the privacy requirement of protecting offloading preference during offloading policy learning process in VANET. Despite the non-convexity and centralized of this joint optimization problem, we propose a privacy-aware MADRL (PA-MADRL) approach to solve it, which can allow the offload decision of each vehicle to reach the Nash Equilibrium (NE) without leaking offloading preference. The key to resisting the OPI attack is to protect the offloading preference by 1)elaborately constructing the noise based on (beta, Phi)-differential privacy mechanism and 2) adding it to the action selection and policy updating process of vanilla MADRL. We conduct a detailed theoretical analysis of the convergence and privacy guarantee of the proposed PA-MADRL, and extensive simulations are conducted to demonstrate the effectiveness, privacy-protecting capacity, and cost-efficiency of PA-MADRL approach.
Open radio access network (Open-RAN) is becoming a key component of cellular networks, and therefore optimizing its architecture is vital. The Open-RAN is a distributed architecture that lets the virtualized networking functions be split between Distributed Units (DU) and Centralized Units (CUs); as a result, there is a wide range of design options. We propose an optimization problem to choose the split points. The objective is to balance the load across CUs as well as midhaul links by considering delay requirements. The resulting formulation is an NP-hard problem that is solved with a novel heuristic algorithm. Performance evaluation shows that the gap between optimal and heuristic solutions does not exceed 2%. An in-depth analysis of different centralization levels shows that using multi-CUs could reduce the total bandwidth usage by up to 20%. Moreover, multipath routing can improve the result of load balancing between midhaul links while increasing bandwidth usage.
Barrier coverage in wireless sensor networks has been used in many applications such as intrusion detection and border surveillance. Barrier coverage is used to monitor the network borders to prevent intruders from penetrating the network. In these applications, it is critical to find optimal number of sensor nodes to prolong the network lifetime. Also, increasing the network lifetime is one of the important challenges in these networks. Various algorithms have been proposed to extend the network lifetime while guaranteeing barrier coverage requirements. In this paper, we use the imperialist competitive algorithm ( ICA ) for selecting sensor nodes to do barrier coverage monitoring operations called ICABC . The main objective of this work is to improve the network lifetime in a deployed network. To investigate the performance of ICABC , several simulations were conducted and the results of the experiments show that the ICABC significantly improves the performance than other state-of-art methods.
One of the major challenges in the area of wireless sensor networks is simultaneously reducing energy consumption and increasing network lifetime. Efficient routing algorithms have received considerable attention in previous studies for achieving the required efficiency, but these methods do not pay close attention to coverage, which is one of the most important Quality of Service parameters in wireless sensor networks. Suitable route selection for transferring information received from the environment to the sink plays crucial role in the network lifetime. The proposed method tries to select an efficient route for transferring the information. This paper reviews efficient routing algorithms for preserving k-coverage in a sensor network and then proposes an effective technique for preserving k-coverage and the reliability of data with logical fault tolerance. It is assumed that the network nodes are aware of their residual energy and that of their neighbors. Sensors are first categorized into two groups, coverage and communicative nodes, and some are then re-categorized as clustering and dynamic nodes. Simulation results show that the proposed method provides greater efficiency energy consumption.
This paper presents an innovative Intrusion Detection System (IDS) architecture using Deep Reinforcement Learning (DRL). To accomplish this, we started by analysing the DRL issue for IoT devices, followed by designing intruder attacks using Label Flipping Attack (LFA). We propose an artificial intelligence DRL model to imitate IoT attack detection, along with two defence strategies: Label-based Semi-supervised Defence (LSD) and Clustering-based Semi-supervised Defence (CSD). Finally, we provide the evaluation results of the adaptive attack and defence models on multiple IoT scenarios with the NSL-KDD, IoT-23, and NBaIoT datasets. The research proves that DRL functions effectively with dynamically produced traffic in contrast to existing conventional techniques.
With the maturation of Artificial Intelligence of Things, many countries have promoted the smart city concept to improve citizens’ living quality, encouraging many technology developments on the Internet of Behavior (IoB) that utilizes Internet of Things (IoT) to analyze behavioral patterns. For example, during the epidemic of COVID-19, a face-mask detection system and thermal imaging camera can identify if employees fulfill the standards; the equipment can also check if people keep social distances in public gatherings. Smart Care Systems can utilize IoT to analyze older adults’ behaviors, which understand elders’ living and health conditions or track their diets, heartbeats, and sleep through wearable watches. After collecting and analyzing the data, the system will provide feedback regarding personal health suggestions. IoB is at its initial stage that requires combinations from diverse techniques, such as IoT, big data, and artificial intelligence. These technologies analyze behavioral patterns and benefit enterprises to conduct marketing activities or transfer harmful user behaviors. IoB also requires sensor networks to exchange and share data, which makes it essential to consider the energy consumption issue of the sensors. With the development of large-scale sensors and data collection, it is predictable that there will be more and more IoB applications and framework proposed. IoB needs scholars to involve in-depth researches and present more frameworks that are effective, enabling IoB to achieve real-time behavioral analysis. Given IoB's importance and rich applications, it is a very worthwhile topic of research. For this special issue, the goal is to address more than just IoB algorithms; we hope to explore IoB applications and researches in more areas of study and see how IoB models can take a vast amount of available data and help us uncover undiscovered phenomena, retrieve useful knowledge, and draw conclusions and reasoning.
In society today, mobile communication and mobile computing have a significant role in every aspect of our lives, both personal and public communication. However, the growth in mobile computing usage can be enhanced by integrating mobile computing into cloud computing. This will result in emerging a new model called Mobile Cloud Computing (MCC) that has recently attracted much attention in academic sector. In this work, the main challenges and issues related to MCC are outlined. We also present the recent work and countermeasure solutions that are proposed by researchers to counter the challenges and lastly, crucial open research and issues that direct future research are highlighted.
With the construction of intelligent transportation, big data with heterogeneous, multi-source and massive characteristics has become an important carrier of cooperative intelligent transportation systems (C-ITS) and plays an important role. Big data in C-ITS can break through the restrictions between regions and entities and then learning cooperatively by sharing data. In addition, the combined efficiency and information integration advantages of big data are conducive to the construction of a comprehensive and three-dimensional traffic information system and can enhance traffic prediction. However, such substantial sensitive data, mainly on the cloud infrastructure, exposes several vulnerabilities like data leakages and privacy breaks, especially when data is shared for cooperative learning purposes. To address this, this paper proposes a forward privacy-preserving scheme, named AFFIRM, for multi-party encrypted sample alignment adopting cooperative learning in C-ITS. By introducing the searchable encryption method, we realize the sample alignment of cooperative learning in the multi-party encrypted data space. AFFIRM ensures encrypted sample alignment under the condition of forward privacy security. We have formally proved that the proposed scheme satisfies both forward security and validity. We have assessed AFFIRM by validating the potential threat of malicious tampering by privacy attackers and malicious personnel search for the aligned sample data and verify it. Finally, we numerically tested and compared AFFIRM against the corresponding ones of some state-of-the-art schemes under various record sizes, servers and processing.
In recent years, malware detection has become an active research topic in the area of Internet of Things (IoT) security. The principle is to exploit knowledge from large quantities of continuously generated malware. Existing algorithms practice available malware features for IoT devices and lack real-time prediction behaviors. More research is thus required on malware detection to cope with real-time misclassification of the input IoT data. Motivated by this, in this paper we propose an adversarial self-supervised architecture for detecting malware in IoT networks, SETTI, considering samples of IoT network traffic that may not be labeled. In the SETTI architecture, we design three self-supervised attack techniques, namely Self-MDS, GSelf-MDS and ASelf-MDS. The Self-MDS method considers the IoT input data and the adversarial sample generation in real-time. The GSelf-MDS builds a generative adversarial network model to generate adversarial samples in the self-supervised structure. Finally, ASelf-MDS utilizes three well-known perturbation sample techniques to develop adversarial malware and inject it over the self-supervised architecture. Also, we apply a defence method to mitigate these attacks, namely adversarial self-supervised training to protect the malware detection architecture against injecting the malicious samples. To validate the attack and defence algorithms, we conduct experiments on two recent IoT datasets: IoT23 and NBIoT. Comparison of the results shows that in the IoT23 dataset, the Self-MDS method has the most damaging consequences from the attacker's point of view by reducing the accuracy rate from 98% to 74%. In the NBIoT dataset, the ASelf-MDS method is the most devastating algorithm that can plunge the accuracy rate from 98% to 77%.
With the increasing popularity of mobile edge computing (MEC) for processing intensive and delay sensitive IoT applications, the problem of high energy consumption of MEC has become a significant concern. Energy consumption prediction and monitoring of edge servers are crucial for reducing MEC's carbon footprint in accordance with green computing and sustainable development. However, predicting energy consumption of edge servers is a nontrivial problem due to the fluctuation and variation of different loads. To address this problem, we propose ECMS, a new edge intelligent energy modeling approach that jointly adopts Elman Neural Network (ENN) and feature selection to optimize the consumption of energy on edge servers. ECMS considers 29 parameters relevant to edge server energy consumption and uses the ENN to develop an energy consumption model. Unlike other energy consumption models, ECMS can successfully deal with load fluctuation and various sorts of tasks, such as CPU-intensive, online transaction-intensive, and I/O-intensive. We have validated ECMS through extensive experiments and compared its performance in terms of accuracy and training time to several baseline approaches. The experimental results show the superiority of ECMS to the baseline models. We believe that the proposed model can be used by the MEC resource providers to forecast and optimize energy use.
With the intelligentization of Maritime Transportation System (MTS), Internet of Thing (IoT) and machine learning technologies have been widely used to achieve the intelligent control and routing planning for ships. As an important branch of machine learning, federated learning is the first choice to train an accurate joint model without sharing ships' data directly. However, there are still many unsolved challenges while using federated learning in IoT-enabled MTS, such as the privacy preservation and Byzantine attacks. To surmount the above challenges, a novel mechanism, namely DisBezant, is designed to achieve the secure and Byzantine-robust federated learning in IoT-enabled MTS. Specifically, a credibility-based mechanism is proposed to resist the Byzantine attack in non-iid (not independent and identically distributed) dataset which is usually gathered from heterogeneous ships. The credibility is introduced to measure the trustworthiness of uploaded knowledge from ships and is updated based on their shared information in each epoch. Then, we design an efficient privacy-preserving gradient aggregation protocol based on a secure two-party calculation protocol. With the help of a central server, we can accurately recognise the Byzantine attackers and update the global model parameters privately. Furthermore, we theoretically discussed the privacy preservation and efficiency of DisBezant. To verify the effectiveness of our DisBezant, we evaluate it over three real datasets and the results demonstrate that DisBezant can efficiently and effectively achieve the Byzantine-robust federated learning. Although there are 40% nodes are Byzantine attackers in participants, our DisBezant can still recognise them and ensure the accurate model training.
Security in Industrial Internet of Things (IIoT) is of vital importance as there are some cases where IIoT devices collect sensory information for crucial social production and life. Thus, designing secure and efficient communication channels is always a research hotspot. However, end devices have limitations in memory, computation, and power-supplying capacities. Moreover, perfect forward secrecy (PFS), which means that long-term key exposure cannot disclose previous session keys, is a critical security property for authentication and key exchange (AKE). In this paper, we propose an AKE protocol named SAKE* for the IIoT environment, where PFS is provided by two types of keys (i.e., a master key and an evolution key). In addition, the SAKE* protocol merely uses concatenation, XOR, and hash function operations to achieve lightweight authentication, key exchange, and message integrity. We also compare the SAKE* protocol with seven recent and IoT-related authentication protocols in terms of security properties and performance. Comparison results indicate that the SAKE* protocol consumes the least computation resource and third least communication cost among eight AKE protocols while equipping with twelve security properties.
Differential evolution (DE) algorithm can be used in edge/cloud cyberspace to find an optimal solution due to its effectiveness and robustness}. With the rapid increase of the mobile traffic data and resources in a cybertwin-driven 6G network, the DE algorithm faces some problems such as premature convergence and search stagnation. To deal with the problems mentioned above, an improved DE algorithm based on hierarchical multi-strategy in a cybertwin-driven 6G network (denoted by DEHM) is proposed. Based on the fitness value of the population, DEHM classifies the population into three sub-population. Regarding each sub-population, DEHM adopts different mutation strategies to achieve a tradeoff between convergence speed and population diversity. In addition, a new selection strategy is presented to ensure that the potential individual with good genes is not lost. Experimental results suggest that the DEHM algorithm surpasses other benchmark algorithms in the field of convergence speed and accuracy.
Virtualized networked datacenters (VNDCs) are gaining considerable attention for stochastic task execution under real-time constraints. However, the problem of efficiently minimizing the high energy consumption while ensuring high quality of service (QoS) in VNDCs has not been fully addressed. Although many solutions have been proposed to address this challenge, they are not efficient and only consider one or two of the energy consuming resources of VNDCs. To this end, an adaptive energy-aware algorithm, MCEC, that efficiently reduces the energy consumption of VNDCs while ensuring high QoS is proposed. Different from the existing approaches, the MCEC algorithm considers energy consumed by computing resources, virtual machine (VM) reconfiguration, communication resources and storage media resources while meeting user QoS requirements defined in the service level agreement (SLA). To validate the effectiveness of our algorithm, we carried out extensive experiments and compared the performance of our algorithm with existing baseline algorithms. The results of the experiments show that our algorithm substantially outperforms the baseline algorithms with respect to reducing energy consumption while respecting the service level agreement.
Over the last decade, the Internet of Things (IoT) had impressive growth and became the new direction of information technology. Also, the energy consumption has reached distressing rates due to the large scale of digital context, a number of subscribers, and the number of smart devices.1 By capturing and processing sensitive information in human life, the IoT devices and cloud data centers are increasing energy consumption with a high carbon emission phenomenon. In the IoT ecosystem, intelligent applications require to select smart devices with low energy consumption and battery saving because all smart devices have limited battery life and may lead to disconnect data transmission. However, it is challenging to design a fully optimized framework due to the interconnected nature of smart devices with different technologies. On the other hand, green energy-efficient computing has become a potential research focus in the IoT environment.2 Finally, energy consumption techniques are incoming a more advanced stage in the IoT communications. Also, green energy-efficient techniques can use on-demand protocols, machine learning, deep learning, and artificial intelligence methods to manage cost-effective and power-saving methods on smart devices in IoT communications. To this point, green energy-efficient computing solutions in IoT systems have emerging efforts and high potential to evaluate the critical points and safety conditions. The goal of this special issue is to highlight the latest research focusing on green energy-efficient computing solutions in IoT systems to address the challenges and critical points. We also aim to invite researchers to publish selected original articles presenting intelligent trends to solve new challenges of new problems. We are also interested in review articles as the state-of-the-art of this topic, showing recent major advances and discoveries, significant gaps in the research, and new future issues. This special issue provides a new platform for researchers and scientific experts to share and analyze existing technical case studies to the field of energy-efficient computing solutions in the IoT environments. Our special issue has attracted 35 manuscripts. After a peer review process, 10 papers have been selected for publication in this special issue. Details of these selected papers are presented in the next section.
Software-defined data centers (SDDC) are an emerging softwarized model that can monitor the virtual machines' allocation atop the cloud servers. SDDC consists of softwarized entities like Virtual Machine (VM) and hardware entities like servers and connected switches. SDDCs apply VM deployment algorithms to preserve efficient placement and processing data traffic generated from the Connected and Autonomous Vehicles (CAV). To enhance user satisfaction, SDDC providers are always looking for an intellectual model to monitor large-scale incoming traffics, such as the Internet of Things (IoT) and CAV applications, by optimizing service quality and service level agreement (SLA). This paper is motivated by this, raising an energy-efficient VM cluster placement algorithm named EVCT to handle service quality and SLA issues in an SDDC in a CAV environment. EVCT algorithm leverages the similarity between VMs and models the problem of VM deployment into a weighted directed graph. Based on the amount of traffic between VM, EVCT adopts the "maximum flow and minimum cut theory" to cut the directed graph and achieve high energy-efficient placement for VMs. The proposed algorithm can efficiently reduce the energy consumption cost, provide a high quality of services (QoS) to users, and have good scalability for the variable workload. We have also carried out a series of experiments to use the real-world workload to evaluate the performance of the EVCT. The results illustrate that the EVCT surpasses the state-of-the-art algorithms in terms of energy consumption cost and efficiency.
The high computational capability provided by a data centre makes it possible to solve complex manufacturing issues and carry out large-scale collaborative cloud manufacturing. Accurate, real-time estimation of the power required by a data centre can help resource providers predict the total power consumption and improve resource utilisation. To enhance the accuracy of server power models, we propose a real-time energy consumption prediction method called IECL that combines the support vector machine, random forest, and grid search algorithms. The random forest algorithm is used to screen the input parameters of the model, while the grid search method is used to optimise the hyperparameters. The error confidence interval is also leveraged to describe the uncertainty in the energy consumption by the server. Our experimental results suggest that the average absolute error for different workloads is less than 1.4% with benchmark models.
The Internet of Things (IoT) plays a crucial role in the new generation of smart cities, in which developing Internet of Energy (IoE) in the energy sector is a necessity also. Several schemes have been proposed so far and in this paper we analyze the security of a recently proposed authentication and key agreement framework for smart grid named PALK. Our security analysis demonstrates that an attacker can extract the user permanent identifier and password, which are enough to do any other attacks. To remedy the weaknesses and amend PALK, we propose an improved protocol based on Physical Unclonable Function(PUF) to provide desired security at a reasonable cost. We also prove the semantic security of constructed scheme by using the widely-accepted real and synthetic model, under the computationally hard Diffie-Hellman assumption. Computational and communication cost analysis of the improved protocol versus PALK, based on identical parameter sets on our experimental results on an Arduino UNO R3 board having microcontroller ATmega328P, shows 46% and 23% enhancements, respectively. We also provide, the energy consumption of the proposed protocol and each session of the protocol consumes almost 24 mJ energy. It shows that it is an appropriate choice for constrained environments, such as IoE.
With the ever-increasing demands for high-definition and low-latency video streaming applications, network-assisted video streaming schemes have become a promising complementary solution in the HTTP Adaptive Streaming (HAS) context to improve users’ Quality of Experience (QoE) as well as network utilization. Edge computing is considered one of the leading networking paradigms for designing such systems by providing video processing and caching close to the end-users. Despite the wide usage of this technology, designing network-assisted HAS architectures that support low-latency and high-quality video streaming, including edge collaboration is still a challenge. To address these issues, this article leverages the Software-Defined Networking (SDN), Network Function Virtualization (NFV), and edge computing paradigms to propose A collabo R ative edge- A ssisted framewo R k for HTTP A daptive video s T reaming ( ARARAT ). Aiming at minimizing HAS clients’ serving time and network cost, besides considering available resources and all possible serving actions, we design a multi-layer architecture and formulate the problem as a centralized optimization model executed by the SDN controller. However, to cope with the high time complexity of the centralized model, we introduce three heuristic approaches that produce near-optimal solutions through efficient collaboration between the SDN controller and edge servers. Finally, we implement the ARARAT framework, conduct our experiments on a large-scale cloud-based testbed including 250 HAS players, and compare its effectiveness with state-of-the-art systems within comprehensive scenarios. The experimental results illustrate that the proposed ARARAT methods ( i ) improve users’ QoE by at least 47%, (ii) decrease the streaming cost, including bandwidth and computational costs, by at least 47%, and (iii) enhance network utilization, by at least 48% compared to state-of-the-art approaches.
Protecting large-scale networks, especially Software-Defined Networks (SDNs), against distributed attacks in a cost-effective manner plays a prominent role in cybersecurity. One of the pervasive approaches to plug security holes and prevent vulnerabilities from being exploited is Moving Target Defense (MTD), which can be efficiently implemented in SDN as it needs comprehensive and proactive network monitoring. The critical key in MTD is to shuffle the least number of hosts with an acceptable security impact and keep the shuffling frequency low. In this paper, we have proposed an SDN-oriented Cost-effective Edge-based MTD Approach (SCEMA) to mitigate Distributed Denial of Service (DDoS) attacks at a lower cost by shuffling an optimized set of hosts that have the highest number of connections to the critical servers. These connections are named edges from a graph-theoretical point of view. We have proposed a three-layer mathematical model for the network that can easily calculate the attack cost. We have also designed a system based on SCEMA and simulated it in Mininet. The results show that SCEMA has lower complexity than the previous related MTD field with acceptable performance.
Fog computing aims to provide resources to cloud data centers at the network’s edge to support time-critical Internet of Things (IoT) applications with low-latency requirements. Protecting the IoT-Fog resources and the scheduling services from the treats is critical for executing the users’ requests in the IoT-Fog network. Proper scheduling algorithms are essential to fulfill the requirements of users’ applications properly and fully harness the potential of IoT-Fog resources. Software-Defined Networking (SDN) is a structure that decouples the control plane from the data plane, resulting in more flexible management. That eases the implementation of security mechanisms in the IoT-Fog networks. In SDN-based IoT-Fog networks, SDN switches and controllers can serve as fog gateways/cloud gateways. SDN switches and controllers, on the other hand, are more susceptible to a variety of assaults, making the SDN controller a bottleneck and thus easy to control plane saturation. IoT devices are inherently insecure, making the IoT-Fog network vulnerable to a variety of attacks. This paper presents S-FoS, an SDN-based security-aware workflow scheduler for IoT-Fog networks. The proposed approach defends scheduling services against distributed denial of service (DDoS) and port scanning assaults. S-FoS is a joint security and performance optimization approach that uses fuzzy-based anomaly detection algorithms to identify the source of attacks and block malicious requestors. It also uses a NSGA-III multi-objective scheduler optimization approach to consider load balancing and delay simultaneously. We show that the S-FoS outperforms state-of-the-art algorithms in IoT-based scenarios through comprehensive simulations. The experiments indicate that by varying the attack rates, the number of IoT devices, and the number of fog devices, the response time of S-FoS could be improved by 31% and 18%, and the network utilization of S-FoS could be improved by 9% and 4%, respectively, compared to the NSGA-II and MOPSO algorithms.
With the rapid advancement of Internet of Things (IoT) devices, a variety of IoT applications that require a real-time response and low latency have emerged. Fog computing has become a viable platform for processing emerging IoT applications. However, fog computing devices tend to be highly distributed, dynamic, and resource-constrained, so deploying fog computing resources effectively for executing heterogeneous and delay-sensitive IoT tasks is a fundamental challenge. In this paper, we mathematically formulate the task scheduling problem to minimize the total energy consumption of fog nodes (FNs) while meeting the quality of service (QoS) requirements of IoT tasks. We also consider the minimization of the deadline violation time in our model. Next, we propose two semi-greedy based algorithms, namely priority-aware semi-greedy (PSG) and PSG with multistart procedure (PSG-M), to efficiently map IoT tasks to FNs. We evaluate the performance of the proposed task scheduling approaches with respect to the percentage of IoT tasks that meet their deadline requirement, total energy consumption, total deadline violation time, and the system’s makespan. Compared with existing algorithms, the experiment results confirm that the proposed algorithms improve the percentage of tasks meeting their deadline requirement up to 1.35x and decrease the total deadline violation time up to 97.6% compared to the second-best results, respectively, while the energy consumption of fog resources and makespan of the system are optimized.
Artificial Intelligence (AI) and Data Analytics play a crucial role in building a digitalized society that is ethical and inclusive. AI is a simulation that is trained to learn and mimic human behaviour. These AI algorithms are capable of learning from their mistakes and doing tasks that are comparable to those performed by humans. AI will have a significant impact on our quality of life as it develops. The main aim of any tool and approach is to simplify human effort and aid us in making better decisions. Data Analytics helps in analyzing raw data in order to draw inferences from it. These techniques and processes have been automated in order to deal with raw data, which is intended for human consumption. The combination of both these techniques will help humans to evolve further in field of research and will enhance the decision making process... Byline: Mamoun Alazab, Ameer Al-Nemrat, Mohammad Shojafar, Shahd Al-Janabi
Password is one of the most well-known authentication methods in accessing many Internet of Things (IoT) devices. The usage of passwords, however, inherits several drawbacks and emerging vulnerabilities in the IoT platform. However, many solutions have been proposed to tackle these limitations. Most of these defense strategies suffer from a lack of computational power and memory capacity and do not have immediate cover in the IoT platform. Motivated by this consideration, the goal of this paper is fivefold. First, we analyze the feasibility of implementing a honeyword-based defense strategy to prevent the latest developed server-side threat on the IoT domain's password. Second, we perform thorough cryptanalysis of a recently developed honeyword-based method to evaluate its advancement in preventing the threat and explore the best possible way to incorporate it in the IoT platform. Third, we verify that we can add a honeyword-based solution to the IoT infrastructure by ensuring specific guidelines. Forth, we propose a generic attack model, namely matching attack utilizing the compromised password-file to perform the security check of any legacy-UI approach for meeting the all essential flatness security criterion. Last, we compare the matching attack's performance with the corresponding one of a benchmark technological methods over the legacy-UI model and confirm that our attack has 5%~22% more vulnerable than others.
This book presents the latest advances in machine intelligence and big data analytics to improve early warning of cyber-attacks, for cybersecurity intrusion detection and monitoring, and malware analysis. Cyber-attacks have posed real and wide-ranging threats for the information society. Detecting cyber-attacks becomes a challenge, not only because of the sophistication of attacks but also because of the large scale and complex nature of today’s IT infrastructures. It discusses novel trends and achievements in machine intelligence and their role in the development of secure systems and identifies open and future research issues related to the application of machine intelligence in the cybersecurity field. Bridging an important gap between machine intelligence, big data, and cybersecurity communities, it aspires to provide a relevant reference for students, researchers, engineers, and professionals working in this area or those interested in grasping its diverse facets and exploring the latest advances on machine intelligence and big data analytics for cybersecurity applications. .
Blockchain technology is defined as a decentralized system of distributed registers that are used to record data transactions on multiple computers. The reason this technology has gained popularity is that you can put any digital asset or transaction in the blocking chain, the industry does not matter. Blockchain technology has infiltrated all areas of our lives, from manufacturing to healthcare and beyond. Cybersecurity is an industry that has been significantly affected by this technology and may be more so in the future. Blockchain for Cybersecurity and Privacy: Architectures, Challenges, and Applications is an invaluable resource to discover the blockchain applications for cybersecurity and privacy. The purpose of this book is to improve the awareness of readers about blockchain technology applications for cybersecurity and privacy. This book focuses on the fundamentals, architectures, and challenges of adopting blockchain for cybersecurity. Readers will discover different applications of blockchain for cybersecurity in IoT and healthcare. The book also includes some case studies of the blockchain for e-commerce online payment, retention payment system, and digital forensics. The book offers comprehensive coverage of the most essential topics, including: Blockchain architectures and challenges Blockchain threats and vulnerabilities Blockchain security and potential future use cases Blockchain for securing Internet of Things Blockchain for cybersecurity in healthcare Blockchain in facilitating payment system security and privacy This book comprises a number of state-of-the-art contributions from both scientists and practitioners working in the fields of blockchain technology and cybersecurity. It aspires to provide a relevant reference for students, researchers, engineers, and professionals working in this particular area or those interested in grasping its diverse facets and exploring the latest advances on the blockchain for cybersecurity and privacy.
This title encourages both researchers and practitioners to share and exchange their experiences and recent studies between academia and industry to highlight and discuss the recent development and emerging trends cybercrime and computer digital forensics in the Cloud of Things; to propose new models, practical solutions, and technological advances related to cybercrime and computer digital forensics in the Cloud of Things; and to discuss new cybercrime and computer digital forensics models, prototypes, and protocols for the Cloud of Things environment.
The widespread adoption of smartphones dramatically increases the risk of attacks and the spread of mobile malware, especially on the Android platform. Machine learning-based solutions have been already used as a tool to supersede signature-based anti-malware systems. However, malware authors leverage features from malicious and legitimate samples to estimate statistical difference in-order to create adversarial examples. Hence, to evaluate the vulnerability of machine learning algorithms in malware detection, we propose five different attack scenarios to perturb malicious applications (apps). By doing this, the classification algorithm inappropriately fits the discriminant function on the set of data points, eventually yielding a higher misclassification rate. Further, to distinguish the adversarial examples from benign samples, we propose two defense mechanisms to counter attacks. To validate our attacks and solutions, we test our model on three different benchmark datasets. We also test our methods using various classifier algorithms and compare them with the state-of-the-art data poisoning method using the Jacobian matrix. Promising results show that generated adversarial samples can evade detection with a very high probability. Additionally, evasive variants generated by our attack models when used to harden the developed anti-malware system improves the detection rate up to 50% when using the generative adversarial network (GAN) method.
Massive multidimensional health data collected from Internet of Things (IoT) devices are driving a new era of smart health, and with it come privacy concerns. Privacy-preserving data aggregation (PDA) is a proven solution providing statistics while hiding raw data. However, existing PDA schemes ignore the willingness of data owners to share, so data owners may refuse to share data. To increase their willingness to contribute data, we propose an OPtional dimEnsional pRivacy-preserving data Aggregation scheme, OPERA , to provide data contributors with options on sharing dimensions while keeping their choices and data private. OPERA uses selection vectors to represent the decisions of users and count participants dimensionally and achieves data privacy and utility based on a multi-secret sharing method and symmetric homomorphic cryptography. Analyses show that in OPERA, the probability of adversaries breaching privacy is less than 4.68e-97. Performance evaluations demonstrate that OPERA is outstanding in computation and practical communication.
Critical infrastructures (CIs) include the vital resources for the country’s economic and health systems and should be kept secure. We face improvements in the Internet of Things which brings benefits and, at the same time, dependency for CIs. Internet of Medical Things (IoMT) is among the CI sectors that gather health-related information from patients via sensors and provide healthcare services accordingly. However, research has highlighted that this large-scale system opens the door to the patients’ private data disclosure. Recent work has concentrated on proposing authentication schemes to address this challenge. Motivated by this, in this paper, we introduce a secure and lightweight authentication and key agreement model named Slight. We informally prove Slight’s security and robustness against attacks and formally by using the Scyther tool. We analyze Slight’s performance to show it causes minimal computational overhead (0.0076 ms) and comparable communication overhead (1632 bits), making it suitable for IoMT. [Display omitted] •We propose secure and lightweight authentication and key agreement model named Slight for IoMT environments to provide privacy and security of patients’ health-related data.•The security of the proposed protocol is validated formally through the Scyther tool.•The proposed protocol provides resistance against well-known potential security attacks.•The performance analysis showed that proposed protocol is more efficient than other competing protocols in terms of computational and communication overhead.
Industrial Internet of Things (IIoT) devices have been widely used for monitoring and controlling the process of the automated manufacturing. Due to limited computing capacity of the IIoT sensors in the production line, the scheduling task in production line needs to be offloaded to the edge computing servers (ECS). To obtain desired quality of service (QoS) during offloading scheduling tasks, {the precise interaction information between production line and ECSs have to be uploaded to the} cloud platform, which poses privacy issues. Existing works mostly assume all interaction information, i.e., the offloading decision for the subtask in a scheduling task, have same privacy level, which cannot meet the various privacy requirements of the offloading decision for the subtask. Hence, we propose a local differential privacy-based deep reinforcement learning (LDP-DRL) approach in edge-cloud-assisted IIoT to provide personalized privacy guarantee. The LDP mechanism can generate different level of noise to satisfy various privacy requirements of the offloading decision for the subtask. The prioritized experience replay (PER) is integrated in DRL to reduce the impact of noise on the QoS performance of task offloading. The formal analysis of the LDP-DRL is provided in terms of privacy level and convergence. Finally, the extensive experiments are conducted to evaluate the effectiveness, capacity of privacy protection, the impact of discount factor on the convergence, and cost efficiency of the LDP-DRL approach.