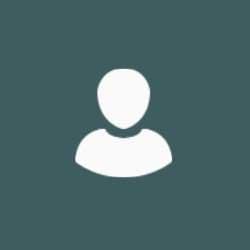
Dr Matthew Bailey
Publications
This landing page contains the datasets presented in the paper "Finite Aperture Stereo". The datasets are intended for defocus-based 3D reconstruction and analysis. Each download link contains images of a static scene, captured from multiple viewpoints and with different focus settings. The captured objects exhibit a range of reflectance properties and are physically small in scale. Calibration images are also available. A CC BY-NC licence is in effect. Use of this data must be for non-commercial research purposes. Acknowledgement must be given to the original authors by referencing the dataset DOI, the dataset web address, and the aforementioned publication. Re-distribution of this data is prohibited. Before downloading, you must agree with these conditions as presented on the dataset webpage.
Multi-view stereo remains a popular choice when recovering 3D geometry, despite performance varying dramatically according to the scene content. Moreover, typical pinhole camera assumptions fail in the presence of shallow depth of field inherent to macro-scale scenes; limiting application to larger scenes with diffuse reflectance. However, the presence of defocus blur can itself be considered a useful reconstruction cue, particularly in the presence of view-dependent materials. With this in mind, we explore the complimentary nature of stereo and defocus cues in the context of multi-view 3D reconstruction; and propose a complete pipeline for scene modelling from a finite aperature camera that encompasses image formation, camera calibration and reconstruction stages. As part of our evaluation, an ablation study reveals how each cue contributes to the higher performance observed over a range of complex materials and geometries. Though of lesser concern with large apertures, the effects of image noise are also considered. By introducing pre-trained deep feature extraction into our cost function, we show a step improvement over per-pixel comparisons; as well as verify the cross-domain applicability of networks using largely in-focus training data applied to defocused images. Finally, we compare to a number of modern multi-view stereo methods, and demonstrate how the use of both cues leads to a significant increase in performance across several synthetic and real datasets.
Reconstruction approaches based on monocular defocus analysis such as Depth from Defocus (DFD) often utilise the thin lens camera model. Despite this widespread adoption, there are inherent limitations associated with it. Coupled with invalid parameterisation commonplace in literature, the overly-simplified image formation it describes leads to inaccurate defocus modelling; especially in macro-scale scenes. As a result, DFD reconstructions based around this model are not geometrically consistent, and are typically restricted to single-view applications. Subsequently, the handful of existing approaches which attempt to include additional viewpoints have had only limited success.In this work, we address these issues by instead utilising a thick lens camera model, and propose a novel calibration procedure to accurately parameterise it. The effectiveness of our model and calibration is demonstrated with a novel DFD reconstruction framework. We achieve highly detailed, geometrically accurate and complete 3D models of real-world scenes from multi-view focal stacks. To our knowledge, this is the first time DFD has been successfully applied to complete scene modelling in this way.
While the accuracy of multi-view stereo (MVS) has continued to advance, its performance reconstructing challenging scenes from images with a limited depth of field is generally poor. Typical implementations assume a pinhole camera model, and therefore treat defocused regions as a source of outlier. In this paper, we address these limitations by instead modelling the camera as a thick lens. Doing so allows us to exploit the complementary nature of stereo and defocus information, and overcome constraints imposed by traditional MVS methods. Using our novel reconstruction framework, we recover complete 3D models of complex macro-scale scenes. Our approach demonstrates robustness to view-dependent materials, and outperforms state-of-the-art MVS and depth from defocus across a range of real and synthetic datasets.