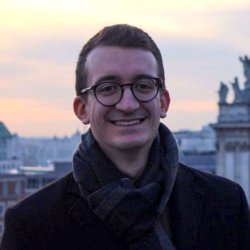
Dr Marco Visca
Academic and research departments
School of Mechanical Engineering Sciences, Centre for Automotive Engineering, Surrey Space Centre.Publications
Driving energy consumption plays a major role in the navigation of mobile robots in challenging environments, especially if they are left to operate unattended under limited on-board power. This paper reports on first results of an energy-aware path planner, which can provide estimates of the driving energy consumption and energy recovery of a robot traversing complex uneven terrains. Energy is estimated over trajectories making use of a self-supervised learning approach, in which the robot autonomously learns how to correlate perceived terrain point clouds to energy consumption and recovery. A novel feature of the method is the use of 1D convolutional neural network to analyse the terrain sequentially in the same temporal order as it would be experienced by the robot when moving. The performance of the proposed approach is assessed in simulation over several digital terrain models collected from real natural scenarios, and is compared with a heuristic inclination-based energy model. We show evidence of the benefit of our method to increase the overall prediction r2 score by 66.8 % and to reduce the driving energy consumption over planned paths by 5.5 %.
Terrain traversability analysis plays a major role in ensuring safe robotic navigation in unstructured environments. However, real-time constraints frequently limit the accuracy of online tests especially in scenarios where realistic robot-terrain interactions are complex to model. In this context, we propose a deep learning framework trained in an end-to-end fashion from elevation maps and trajectories to estimate the occurrence of failure events. The network is first trained and tested in simulation over synthetic maps generated by the OpenSimplex algorithm. The prediction performance of the Deep Learning framework is illustrated by being able to retain over 94% recall of the original simulator at 30% of the computational time. Finally, the network is transferred and tested on real elevation maps collected by the SEEKER consortium during the Martian rover test trial in the Atacama desert in Chile. We show that transferring and fine-tuning of an application-independent pre-trained model retains better performance than training uniquely on scarcely available real data.
Driving energy consumption plays a major role in the navigation of mobile robots in challenging environments, especially if they are left to operate unattended under limited on-board power. This paper reports on first results of an energy-aware path planner, which can provide estimates of the driving energy consumption and energy recovery of a robot traversing complex uneven terrains. Energy is estimated over trajectories making use of a self-supervised learning approach, in which the robot autonomously learns how to correlate perceived terrain point clouds to energy consumption and recovery. A novel feature of the method is the use of 1D convolutional neural network to analyse the terrain sequentially in the same temporal order as it would be experienced by the robot when moving. The performance of the proposed approach is assessed in simulation over several digital terrain models collected from real natural scenarios, and is compared with a heuristic inclination-based energy model. We show evidence of the benefit of our method to increase the overall prediction r2 score by 66.8 % and to reduce the driving energy consumption over planned paths by 5.5 %.
Driving energy consumption plays a major role in the navigation of autonomous mobile robots in off-road scenarios. However, real-time constraints often limit the accuracy of the energy estimations, especially in scenarios where accurate wheel-terrain interactions are complex to model. In this paper, an adaptive deep meta-learning energy-aware path planner is proposed that can provide energy estimates of a mobile robot traversing complex uneven terrains with varying and unknown terrain properties. A novel feature of the method is the integration into the meta-learning framework of a 1D convolutional neural network to analyze the terrain sequentially, in the same temporal order as it would be experienced by the robot when moving, and efficiently adapt its energy estimates to the local terrain conditions based on a small number of local measurements. The performance of the method is assessed in a realistic 3D-body dynamic simulator over several typologies of deformable terrains and unstructured geometries. We provide evidence of the benefit of the proposed approach to retain 83% r2 score of the original simulator at 0.55% of the computing time. Finally, the method is compared with alternative state-of-the-art deep learning solutions. In this way, we show indications of its improved robustness to provide more informed driving energy estimations and energy-efficient paths when navigating over challenging uneven terrains.
This paper presents an adaptive energy-aware prediction and planning framework for vehicles navigating over terrains with varying and unknown properties. A novel feature of the method is the use of a deep meta-learning framework to learn a prior energy model, which can efficiently adapt to the local terrain conditions based on small quantities of exteroceptive and proprioceptive data. A meta-adaptive heuristic function is also proposed for the integration of the energy model into an A* path planner. The performance of the proposed approach is assessed in a 3D-body dynamic simulator over several typologies of deformable terrains and compared with alternative machine learning solutions. We provide evidence of the advantages of the proposed method to adapt to unforeseen terrain conditions, thereby yielding more informed estimations and energy-efficient paths when navigating on unknown terrains.