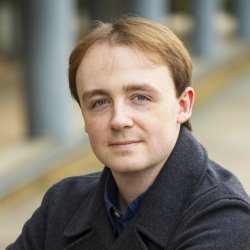
Dr Luke Lee-Brewin
About
My research project
Predicting Gamma Dose Rates from Buried Pipelines based on Limited InformationUse gamma spectroscopy to develop a non-invasive methodology to identify the contents of contaminated structures and determine the dose rate as a function of depth.
Supervisors
Use gamma spectroscopy to develop a non-invasive methodology to identify the contents of contaminated structures and determine the dose rate as a function of depth.
My qualifications
ResearchResearch interests
Gamma Spectroscopy and Signal Processing
Sensor Optimisation
Nuclear Decomissioning
Research interests
Gamma Spectroscopy and Signal Processing
Sensor Optimisation
Nuclear Decomissioning
Teaching
Demonstrating python to undergraduates their Essential Maths module (PHY1034)
Publications
Identifying radioisotopes can facilitate risk-informed management decisions in nuclear security and nuclear site decommissioning applications. An artificial neural network based algorithm capable of identifying multiple radioisotopes across a range of shielded environments is presented. Five isotopes were selected to create this algorithm. Small sets of single-isotope gamma spectra were collected using a sodium iodide detector in three different shielding configurations. Each of these sets of spectra was independently augmented by combining spectra to create multi isotope spectra. The three sets were then combined to form a training set containing 38500 spectra. Four types of neural networks were explored, optimised, and compared. Based on the results of this comparison, a multi-label convolutional neural network with five hidden layers was chosen to test on a large set of 2132 spectra using sources of the same isotopes with different activities. These testing spectra were collected experimentally and independently of the training set. Subsets of spectra were taken from the testing set and used to assess the ability of the algorithm in four different ways: to identify multiple spectra simultaneously, to adapt to different shielding configurations, to explore the decrease in performance when analysing count-starved spectra, and to analyse spectra from a different NaI detector. The precision, recall, and F1 scores were calculated on all tests. Results show a high performance with F1 scores that are typically above 0.85. Recall values were generally higher than precision when analysing shielded spectra, indicating that the algorithm tends to produce more false positives than negatives.