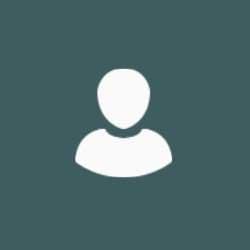
Łukasz Tomaszewski
About
My research project
Optimisation of radiation health monitoring through machine learning and data fusionThis research project seeks to build on prior studies in radiation dosimetry, focusing specifically on the relationship between glow curve mathematical modelling and the fundamental physics. Previous research has highlighted the use of silica beads and fibres for delayed thermoluminescence dosimetry (TLD), giving access to new ways to detect and monitor ionising radiation.
This study will compare real-time dosimetry with that obtained through delayed in-situ material measurements obtained via thermoluminescence dosimetry. Machine learning techniques will be employed to model the glow curve response across various bead and fibre materials, potentially drawing from experimental seed datasets. Approaches such as neural networks will then explore the capability of artificial intelligence to distinguish material composition characteristics (bead colour, fibre core type), to determine the received radiation dose and understand the kinematics of the trapped electrons. This will produce an enhanced analysis of their structure and behaviour to predict and aid radiation dosimetry, detection and monitoring and, in turn, improve safety.
To further optimise this project, data fusion approaches will assess the diagnostic information obtained when retrospective and real-time radiation monitoring methods are combined. This project’s scope will inform an assessment of delayed material dosimetry within the radiation health monitoring landscape, supporting a diverse array of detection and monitoring methods. Benefitting an array of disciplines that handle ionising radiation, focusing on security and safety applications and where retrospective monitoring may be required in cases of natural disasters or human accidents.
Supervisors
This research project seeks to build on prior studies in radiation dosimetry, focusing specifically on the relationship between glow curve mathematical modelling and the fundamental physics. Previous research has highlighted the use of silica beads and fibres for delayed thermoluminescence dosimetry (TLD), giving access to new ways to detect and monitor ionising radiation.
This study will compare real-time dosimetry with that obtained through delayed in-situ material measurements obtained via thermoluminescence dosimetry. Machine learning techniques will be employed to model the glow curve response across various bead and fibre materials, potentially drawing from experimental seed datasets. Approaches such as neural networks will then explore the capability of artificial intelligence to distinguish material composition characteristics (bead colour, fibre core type), to determine the received radiation dose and understand the kinematics of the trapped electrons. This will produce an enhanced analysis of their structure and behaviour to predict and aid radiation dosimetry, detection and monitoring and, in turn, improve safety.
To further optimise this project, data fusion approaches will assess the diagnostic information obtained when retrospective and real-time radiation monitoring methods are combined. This project’s scope will inform an assessment of delayed material dosimetry within the radiation health monitoring landscape, supporting a diverse array of detection and monitoring methods. Benefitting an array of disciplines that handle ionising radiation, focusing on security and safety applications and where retrospective monitoring may be required in cases of natural disasters or human accidents.