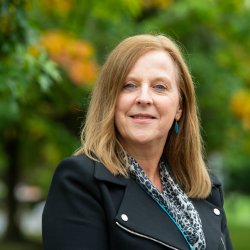
Dr Kim Starr
About
Biography
Kim began her academic studies at Queen Mary, University of London graduating with a BSc (Econ) in Politics and Law. She subsequently spent a decade in the finance industry working for a City brokerage where she provided legal consultancy services to blue-chip corporate clients.This was followed by fifteen years in the commercial television sector, during which time she received sponsorship from her employer Independent Television (ITV) to pursue an MA in Journalism at the University of Westminster. She subsequently worked in the press team at Independent Television News (ITN) where she held the position of Head of Internal Communications. Kim returned to academia in 2010, studying for an MA in audiovisual translation at the University of Surrey's Centre for Translation Studies.
Kim was awarded a full doctoral studentship by the AHRC/UKRI, gaining her PhD in intralingual translation. Her doctoral research focused on the use of audio description for the purposes of conveying emotions in film content to children on the autism spectrum.
She has since held two research fellow posts, the first working on the human analysis of machine-generated AV captioning data, under the umbrella of the EU-funded MeMAD (Methods for Managing Audiovisual Data) project, and currently as a researcher across translation and multimodal technologies.
In 2022, Kim was granted the UK-US Fulbright-Smithsonian Scholar's Award.
University roles and responsibilities
- Research Fellow
ResearchResearch interests
Audiovisual translation, intralingual translation, media accessibility, universal design, digital humanities.
Research projects
MeMAD is an European Union Horizon 2020 research project (2018-2020) led by Aalto University (Finland).
The project will develop novel methods and models for managing and accessing digital audiovisual information in multiple languages and for various use contexts and audiences, based on a combination of computer vision technologies, human input and machine learning approaches to derive enhanced descriptions of audio-visual content.
These descriptions will benefit the creative industries, especially television broadcasters and on-demand media service providers, as well as people using their services, by enabling them to access audiovisual information in novel ways.
Research interests
Audiovisual translation, intralingual translation, media accessibility, universal design, digital humanities.
Research projects
MeMAD is an European Union Horizon 2020 research project (2018-2020) led by Aalto University (Finland).
The project will develop novel methods and models for managing and accessing digital audiovisual information in multiple languages and for various use contexts and audiences, based on a combination of computer vision technologies, human input and machine learning approaches to derive enhanced descriptions of audio-visual content.
These descriptions will benefit the creative industries, especially television broadcasters and on-demand media service providers, as well as people using their services, by enabling them to access audiovisual information in novel ways.
Teaching
Kim has taught the Academic Writing series of seminars for the past three years at the Centre for Translation Studies.
Publications
Highlights
Starr, K. (2022) ‘Audio Description for the Non-Blind’, in Taylor, C. and Perego, E. (eds.) The Routledge Handbook of Audio Description. Abingdon: Routledge.
Braun, S. and Starr, K. (2022) ‘Automation in Audio Description’, in Taylor, C. and Perego, E. (eds.) The Routledge Handbook of Audio Description. Abingdon: Routledge.
Braun, S. and Starr, K. (2020) Innovation in Audio Description Research. Abingdon: Routledge.
According to Snyder, “AD is about democracy” (2005: 16 in Mazur & Chmeil, 2012), yet audio description (AD) research and practice remains fundamentally focused on optimising accessibility to multimodal texts by reference to those affected by physical (visual) impairment.
Nevertheless, recent evidence suggests audiences requiring cognitive assistance, including individuals on the autism spectrum experiencing emotion-recognition difficulties (ERDs), may also benefit from supplementary audio description (Fellowes, 2012).
While previous studies have considered the AD of emotions, emotional lexicon and describing gestures and facial expression for visually impaired audiences (Ramos, 2015; Salway & Graham, 2003; Mazur & Chmeil, 2012), emotion-centric AD has not been considered in relation to audiences with emotion recognition difficulties.
This study employs a functionalist, skopos-based approach (Reiss and Vermeer, 1984 in Nord, 1997:29) to AD creation in order to examine the potential for extending the reach of audio description into the domain of supplemental cognitive narrative through the adoption of bespoke, dianoic (‘between minds’) translation strategies.
Consideration will be given to the results of an empirical study trialling prototype cognitive AD outputs alongside standard audio description with young autistic spectrum audiences who typically experience difficulty identifying emotions and ‘states of mind’ in others. For this purpose, three discrete AD orientations were created from audiovisual source texts:
(i) standard, blind and visually-impaired (BVI) AD, designed to be visually restorative; (ii) bespoke descriptive AD, created for audiences experiencing difficulty reading emotions and ‘states of mind’, and characterised by the identification and labelling of emotive-markers (EMO); and (iii), bespoke interpretative AD, created for audiences requiring additional assistance with assigning causality and consequence to emotions, and characterised by the contextualisation of emotive-markers (CXT).
Findings from the study are used to support the case for a fundamental reappraisal of AD as an accessibility tool. It will be argued that by developing dianoic translation strategies it should be possible to employ AD to enhance access to audiovisual materials for a range of audiences with atypical cognitive needs. To this end, examples of coincidence and divergence between standard BVI and cognitive EMO/CXT target texts will be given particular consideration for their potential to serve both types of audience simultaneously and independently.
The presentation will conclude with a brief discussion of the manner in which dianoic translation might be further developed to deliver competing AD channels in ‘multiplex’/ ‘red button’ television environments.
This project will develop novel methods of analysing and describing audiovisual content based on a combination of computer vision techniques, human input and machine learning approaches to derive enhanced automatic descriptions. These descriptions will enable people working in the creative industries as well as people using their services to access, use and find audiovisual information in novel ways.
There is broad consensus that audio description (AD) is a modality of intersemiotic translation, but there are different views in relation to how AD can be more precisely conceptualised. While Benecke (2014) characterises AD as ‘partial translation’, Braun (2016) hypothesises that what audio describers appear to ‘omit’ from their descriptions can normally be inferred by the audience, drawing on narrative cues from dialogue, mise-en-scène, kinesis, music or sound effects. This presentation reports on a study that is testing these hypotheses empirically.
Conducted as part of the EU-funded MeMAD project, our research aims to improve access to, and management of, audiovisual (AV) content through various methods, including by enhancing the automation of AV content description through a combination of approaches from computer vision, machine learning and human approaches to describing AV material. To this end, one of the MeMAD workstreams analyses how human audio descriptions approach the rendition of visually salient cues. We use a corpus of approx. 500 audio described film extracts to identify substantive visual elements, i.e. elements that can be considered essential for the construction of the filmic narrative, and analyse the corresponding audio descriptions in terms of how these elements are verbally represented. Where omissions in the audio description appear to occur, we conduct a qualitative analysis to establish whether the ‘omitted’ elements can be inferred from the co-text of the AD and/or from other cues that are accessible to visually impaired audiences (e.g. the film dialogue). Where possible, we establish the most likely source of these inferences.
In this presentation we outline the findings of the study and discuss their relevance, which we show to be twofold. Firstly, the study provides novel insights into a crucial aspect of AD practice and can inform approaches to training. Secondly, by highlighting how human audiences use their ability to draw inferences to build a coherent interpretation of what they perceive, the results of the study can also inform machine-based approaches to developing human-like descriptions of AV material.
Media For All 8 Conference, Stockholm, 17th - 19th June, 2019.
The recent proliferation of (audio)visual content on the Internet, including increased user-generated content, intersects with European-wide legislative efforts to make (audio)visual content more accessible for diverse audiences. As far as access to visual content is concerned, audio description (AD) is an established method for making content accessible to audiences with visual impairment. However, AD is expensive to produce and its coverage remains limited. This applies particularly to the often ephemeral user-generated (audio)visual content on social media, but the Internet more broadly remains less accessible for people with sight loss, despite its high social relevance for people’s everyday lives.
Advances in computer vision, machine learning and AI have led to increasingly accurate automatic image description. Although currently focused on still images, attempts at automating moving image description have also begun to emerge (Huang et al. 2015, Rohrbach et al. 2017). One obvious question arising from these developments is how machine-generated descriptions compare with their human-made counterparts. Initial examination reveals stark differences between the two methods. A more immediate question is where human endeavour might prove most fruitful in the development of effective approaches to automating moving image description.
This presentation reports on an initial study comparing human and machine-generated descriptions of moving images, aimed at identifying the key characteristics and patterns of each method. The study draws on corpus-based and discourse-based approaches to analyse, for example, lexical choices, focalisation and consistency of description. In particular, we will discuss human techniques and strategies which can inform and guide the automation of description. The broader aim of this work is to advance current understanding of multimodal content description and contribute to enhancing content description services and technologies.
This presentation is supported by an EU H2020 grant (MeMAD: Methods for Managing Audiovisual Data: Combining Automatic Efficiency with Human Accuracy).