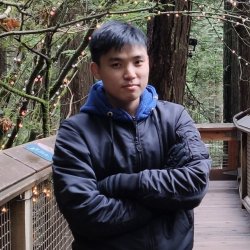
Kam Woh Ng
About
My research project
Deep visual representation learningThe objective of this project is to develop novel gradient based deep visual representation learning methods. An important goal in representation learning is to allow a system (for example, a neural network) to automatically discover the representations or features needed for classification from raw data. A feature or a data representation is often acted as an input to a machine learning method, and to mathematically compute an output. However, real-world data such as images, video, and sensor data pose challenges as input of a machine learning model as these data are huge in size and often contain a lot of non-linear relationships. Deep representation learning is thus important. In this project, the focus will be on supervised visual representation learning with a focus on incorporating gradient information in the learning. Moreover, more theoretical and practical analysis will be carried out to understand deeply about this proposed research.
Supervisors
The objective of this project is to develop novel gradient based deep visual representation learning methods. An important goal in representation learning is to allow a system (for example, a neural network) to automatically discover the representations or features needed for classification from raw data. A feature or a data representation is often acted as an input to a machine learning method, and to mathematically compute an output. However, real-world data such as images, video, and sensor data pose challenges as input of a machine learning model as these data are huge in size and often contain a lot of non-linear relationships. Deep representation learning is thus important. In this project, the focus will be on supervised visual representation learning with a focus on incorporating gradient information in the learning. Moreover, more theoretical and practical analysis will be carried out to understand deeply about this proposed research.
Publications
Recent text-to-image (T2I) generative models allow for high-quality synthesis following either text instructions or visual examples. Despite their capabilities, these models face limitations in creating new, detailed creatures within specific categories (e.g., virtual dog or bird species), which are valuable in digital asset creation and biodiversity analysis. To bridge this gap, we introduce a novel task, Virtual Creatures Generation: Given a set of unlabeled images of the target concepts (e.g., 200 bird species), we aim to train a T2I model capable of creating new, hybrid concepts within diverse backgrounds and contexts. We propose a new method called DreamCreature, which identifies and extracts the underlying sub-concepts (e.g., body parts of a specific species) in an unsupervised manner. The T2I thus adapts to generate novel concepts (e.g., new bird species) with faithful structures and photorealistic appearance by seamlessly and flexibly composing learned sub-concepts. To enhance sub-concept fidelity and disentanglement, we extend the textual inversion technique by incorporating an additional projector and tailored attention loss regularization. Extensive experiments on two fine-grained image benchmarks demonstrate the superiority of DreamCreature over prior methods in both qualitative and quantitative evaluation. Ultimately, the learned sub-concepts facilitate diverse creative applications, including innovative consumer product designs and nuanced property modifications.
This paper propels creative control in generative visual AI by allowing users to "select". Departing from traditional text or sketch-based methods, we for the first time allow users to choose visual concepts by parts for their creative endeavors. The outcome is fine-grained generation that precisely captures selected visual concepts, ensuring a holistically faithful and plausible result. To achieve this, we first parse objects into parts through unsupervised feature clustering. Then, we encode parts into text tokens and introduce an entropy-based normalized attention loss that operates on them. This loss design enables our model to learn generic prior topology knowledge about object's part composition, and further generalize to novel part compositions to ensure the generation looks holistically faithful. Lastly, we employ a bottleneck encoder to project the part tokens. This not only enhances fidelity but also accelerates learning, by leveraging shared knowledge and facilitating information exchange among instances. Visual results in the paper and supplementary material showcase the compelling power of PartCraft in crafting highly customized, innovative creations, exemplified by the "charming" and creative birds. Code is released at https://github.com/kamwoh/partcraft.