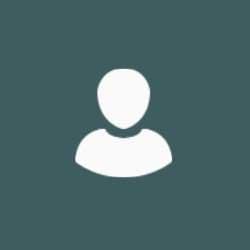
Julian Chan
Postgraduate Research Student
Academic and research departments
ResearchResearch interests
- N-body simulations of galaxy mergers
- Supermassive Black Hole Binaries
- Probabilistic Machine Learning
Research interests
- N-body simulations of galaxy mergers
- Supermassive Black Hole Binaries
- Probabilistic Machine Learning