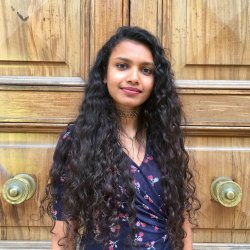
Dr Ishanki Anjana De Mel
Academic and research departments
School of Chemistry and Chemical Engineering, Faculty of Engineering and Physical Sciences.About
My research project
Optimisation of distributed energy systemsDistributed Energy Systems (DES) have been increasingly investigated as a viable alternative to ageing centralised energy networks. Optimisation models presented in literature for the design and operation of distributed energy systems often exclude the inherent nonlinearities, related to power flow and generation and storage units, to maintain an accuracy-complexity balance. Such models may provide sub-optimal or even infeasible designs and dispatch schedules. In DES, optimal power flow (OPF) is often treated as a standalone problem, consisting of highly nonlinear, nonconvex constraints related to the underlying distribution network. This aspect of the optimisation problem has often been overlooked by researchers in the process systems and optimisation area.
This project aims to address the disparity between OPF and DES models, highlighting the importance of including elements of OPF in DES design and operational models to obtain feasible designs and operational schedules. The literature review identified key works that have attempted to do so, and highlights several gaps that have remained despite these efforts. A methodology is proposed to develop new models that are capable of maintaining the accuracy-complexity balance, while consolidating DES and OPF and including detailed representations of key components (such as batteries). It involves three modelling routes, each designed to investigate how detailed modelling can impact DES objectives, designs, and operating schedules. Current model formulations are implemented and tested, where preliminary results shed light on the multi-faceted nature of DES and the need to make detailed optimisation models available to stakeholders who are interested in consolidating them.
Research theme: Digital and Process Innovation
Supervisors
Distributed Energy Systems (DES) have been increasingly investigated as a viable alternative to ageing centralised energy networks. Optimisation models presented in literature for the design and operation of distributed energy systems often exclude the inherent nonlinearities, related to power flow and generation and storage units, to maintain an accuracy-complexity balance. Such models may provide sub-optimal or even infeasible designs and dispatch schedules. In DES, optimal power flow (OPF) is often treated as a standalone problem, consisting of highly nonlinear, nonconvex constraints related to the underlying distribution network. This aspect of the optimisation problem has often been overlooked by researchers in the process systems and optimisation area.
This project aims to address the disparity between OPF and DES models, highlighting the importance of including elements of OPF in DES design and operational models to obtain feasible designs and operational schedules. The literature review identified key works that have attempted to do so, and highlights several gaps that have remained despite these efforts. A methodology is proposed to develop new models that are capable of maintaining the accuracy-complexity balance, while consolidating DES and OPF and including detailed representations of key components (such as batteries). It involves three modelling routes, each designed to investigate how detailed modelling can impact DES objectives, designs, and operating schedules. Current model formulations are implemented and tested, where preliminary results shed light on the multi-faceted nature of DES and the need to make detailed optimisation models available to stakeholders who are interested in consolidating them.
Research theme: Digital and Process Innovation
University roles and responsibilities
- Associate Tutor - Personal Awareness and Development Module (Jan 2020 - Present)
- Teaching Assistant - ENG1083 Transferable and Laboratory Skills (May - June 2020)
- Teaching Assistant - ENG2120 Engineering Systems and Dynamics (Oct - Dec 2019)
My qualifications
Royal Academy of Engineering crowns Surrey students world champions
A team of chemical engineering students from the University of Surrey has been crowned winners of a prestigious international competition hosted by the Royal Academy of Engineering.
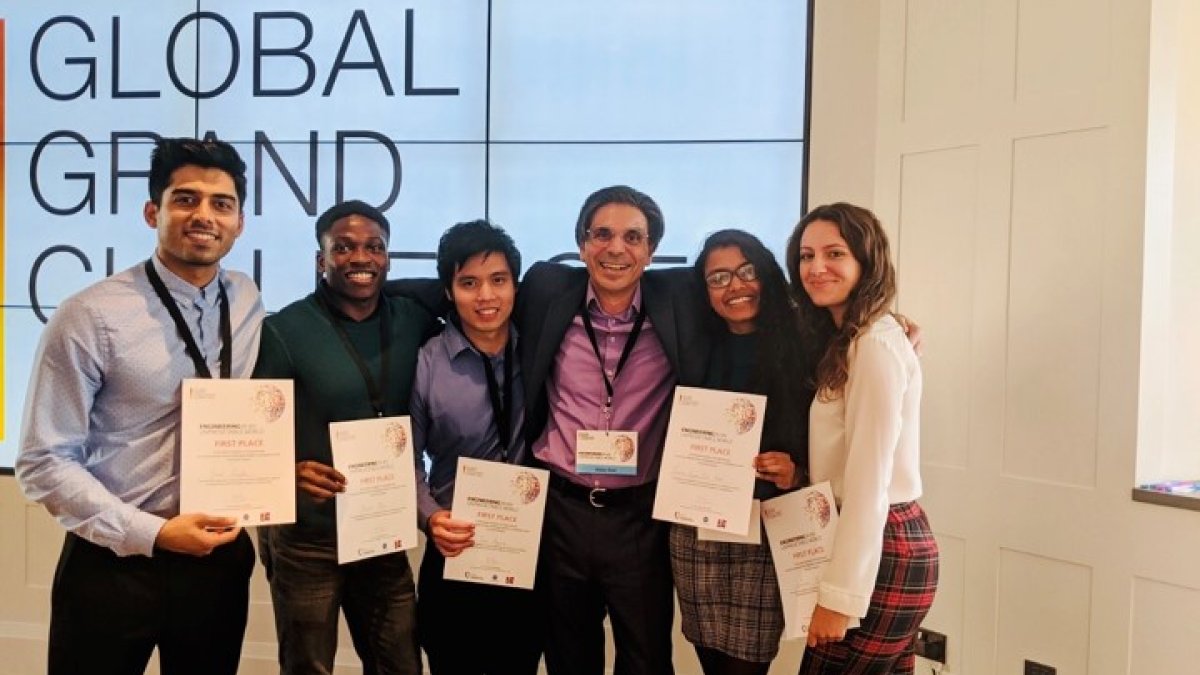
From the left: Zarif Shafiei, Clement Ibeh, John Azurin, Prof. Esat Alpay, Ishanki De Mel, and Nicolina Pieri.
News
In the media
ResearchResearch interests
Ishanki enjoys using optimisation (mathematical programming) techniques to model energy systems and consolidate the multi-faceted nature of these systems. She uses Mixed-Integer Linear Programming (MILP) and Mixed-Integer Nonlinear Programming (MINLP) to develop algorithms and tools, and hopes to make these freely available to the research community and stakeholders interested in designing and operating low-carbon and small-scale energy systems.
She has been involved in several M-level research projects, either as a co-supervisor or contributor, focusing on:
- Distributed Energy Systems
- Multi-period Heat Exchanger Design
- Supply Chain Optimisation
- Personal Awareness and Development
During the COVID-19 pandemic, she volunteered in the Rapid Assistance in Modelling the Pandemic (RAMP) initiative and continues to support a project on modelling fomite transmission, which resulted from this initiative.
Her passion project involves modelling a storage network to minimise post-harvest grain storage losses (Global Grand Challenges 2019 winning idea) with her teammates, which is currently a work-in-progress.
Research interests
Ishanki enjoys using optimisation (mathematical programming) techniques to model energy systems and consolidate the multi-faceted nature of these systems. She uses Mixed-Integer Linear Programming (MILP) and Mixed-Integer Nonlinear Programming (MINLP) to develop algorithms and tools, and hopes to make these freely available to the research community and stakeholders interested in designing and operating low-carbon and small-scale energy systems.
She has been involved in several M-level research projects, either as a co-supervisor or contributor, focusing on:
- Distributed Energy Systems
- Multi-period Heat Exchanger Design
- Supply Chain Optimisation
- Personal Awareness and Development
During the COVID-19 pandemic, she volunteered in the Rapid Assistance in Modelling the Pandemic (RAMP) initiative and continues to support a project on modelling fomite transmission, which resulted from this initiative.
Her passion project involves modelling a storage network to minimise post-harvest grain storage losses (Global Grand Challenges 2019 winning idea) with her teammates, which is currently a work-in-progress.
Publications
Distributed Energy Systems (DES) can play a vital role as the energy sector faces unprecedented changes to reduce carbon emissions by increasing renewable and low-carbon energy generation. However, current operational DES models do not adequately reflect the influence of uncertain inputs on operational outputs, resulting in poor planning and performance. This paper details a methodology to analyse the effects of uncertain model inputs on the primary output, the total daily cost, of an operational model of a DES. Global Sensitivity Analysis (GSA) is used to quantify these effects, both individually and through interactions, on the variability of the output. A Mixed-Integer Linear Programming model for the DES design is presented, followed by the operational model, which incorporates Rolling Horizon Model Predictive Control. A subset of model inputs, which include electricity and heating demand, and solar irradiance, is treated as uncertain using data from a case study. Results show reductions of minimum 25% in the total annualised cost compared to a traditional design that purchases electricity from the centralised grid and meets heating demand using boilers. In terms of carbon emissions, the savings are much smaller, although the dependency on the national grid is drastically reduced. Limitations and suggestions for improving the overall DES design and operation are also discussed in detail, highlighting the importance of incorporating GSA into the DES framework. Introduction The energy sector worldwide has taken on a transformative approach to meet current and future demand by increasing the proportion of renewable and low-carbon energy resources. In its wake, Distributed Energy Systems (DES) have attracted both large and small-scale customers , seeking to take advantage of government incentives whilst reducing greenhouse gas (GHG) emissions, distribution costs, and energy losses associated with transmission over long distances from power plants to consumers [1]. DES are the building block of holistic smart energy systems [2], as they can be connected and integrated into the national grid, with capabilities of introducing internal utility networks. Optimisation-based models are often employed for the design and operation of such systems, where the main aim is the minimization of its total annual cost considering the utilization of renewable energy generation technologies, such as solar panels, wind turbines, fuel cells, electrolysers, hydrogen tanks, etc. [3,4]. In this paper, a two-stage approach, which involves the optimisation of a DES design for a specified location or scale, and the subsequent optimisation of its operation based on the recommended structure [5], is implemented. The design includes the types, capacity and potential location for installation of the various generation and storage technologies to be used during the operation. DES, like most energy systems, have nonlinear characteristics, complex to model and time-consuming to solve [6]. This often demands a trade-off between complexity, accuracy, and solution time [7,8] for the models to be suitable for real-time operation. Therefore DES models are commonly linearized (or formulated as approximate linear models in the first place), to benefit from the efficiency of Mixed-Integer Linear Programming (MILP) solvers [9]. Although arguably more accurate, Mixed-Integer Nonlinear Programs (MINLP) are computationally very expensive and cannot ensure global optimality [7], guaranteed by MILPs. Linearization inevitably introduces differences between prediction and actual system performance for both the design and operational
Optimisation models for the design of distributed energy systems (DES) often exclude inherent nonlinearities and constraints associated with alternating current (AC) power flow and the underlying distribution network. This study aims to assess this gap by comparing the performance of linear and nonlinear formulations of DES design models, connected to and trading with an AC grid. The inclusion of the optimal power flow (OPF) constraints within the DES design framework is demonstrated in the methodology. A residential case study is used to test both models and compare the designs obtained from the two formulations. The results highlight that DES designs obtained are different when constraints related to the underlying distribution network are added, particularly when electricity storage is not considered. Overall, this study highlights the need for future modelling efforts to include OPF within DES optimisation frameworks to obtain practically feasible designs, rather than considering them as standalone problems.
The optimal selection, sizing, and location of small-scale technologies within a grid-connected distributed energy system (DES) can contribute to reducing carbon emissions, consumer costs, and network imbalances. There is a significant lack of studies on how DES designs, especially those with electrified heating systems, impact unbalanced low-voltage distribution networks to which most DES are connected. This is the first study to present an optimisation framework for obtaining discrete technology sizing and selection for grid-connected DES design, while simultaneously considering multiphase optimal power flow (MOPF) constraints to accurately represent unbalanced low-voltage distribution networks. An algorithm is developed to solve the resulting Mixed-Integer Nonlinear Programming (MINLP) formulation. It employs a decomposition based on Mixed-Integer Linear Programming (MILP) and Nonlinear Programming (NLP) and uses integer cuts and complementarity reformulations to obtain discrete designs that are also feasible with respect to the network constraints. A heuristic modification to the original algorithm is also proposed to improve computational speed. Improved formulations for selecting feasible combinations of air source heat pumps (ASHPs) and hot water storage tanks are also presented. Two networks of varying size are used to test the optimisation methods. Designs with electrified heating (ASHPs and tanks) are compared to those with conventional gas boilers. The algorithms outperform one of the existing state-of-the-art commercial deterministic MINLP solvers, which fails to find any solutions in two instances within specified time limits. While feasible solutions were obtained for all cases, convergence was not achieved for all, especially for those involving the larger network. Where converged, the algorithm with the heuristic modification has achieved results up to 70% faster than the original algorithm. Results for case studies suggest that including ASHPs can support up to 16% higher renewable generation capacity compared to gas boilers, albeit with higher ASHP investment costs, as local generation and consumption minimises network violations associated with excess power export. The results also show the importance of including nonlinear power flow constraints in DES design problems. The optimisation framework and results can be used to inform stakeholders such as policymakers and network operators, to increase renewable energy capacity and aid the decarbonisation of domestic heating systems. •The selection of discrete technologies in the presence of MOPF constraints is enabled.•An improved formulation selects feasible ASHP - thermal storage combinations.•The algorithm finds feasible solutions where the state-of-the-art MINLP solver fails.•The proposed heuristic modification decreases computational time.•ASHPs with local renewable energy generation can help minimise network violations.
Distributed Energy Systems (DES) are set to play a vital role in achieving emission targets and meeting higher global energy demand by 2050. However, implementing these systems has been challenging, particularly due to uncertainties in local energy demand and renewable energy generation, which imply uncertain operational costs. In this work we are implementing a Mixed-Integer Linear Programming (MILP) model for the operation of a DES, and analysing impacts of uncertainties in electricity demand, heating demand and solar irradiance on the main model output, the total daily operational cost, using Global Sensitivity Analysis (GSA). Representative data from a case study involving nine residential areas at the University of Surrey are used to test the model for the winter season. Distribution models for uncertain variables, obtained through statistical analysis of raw data, are presented. Design results show reduced costs and emissions, whilst GSA results show that heating demand has the largest influence on the variance of total daily operational cost. Challenges and design limitations are also discussed. Overall, the methodology can be easily applied to improve DES design and operation.
The optimal design of grid-connected distributed energy systems (DES) has been previously investigated, but most studies exclude nonlinear multiphase optimal power flow (MOPF) constraints which capture imbalances in alternating current (AC) distribution networks. Previous DES design studies considering AC power flow assume balanced loads and networks, which is an assumption that does not hold for distribution networks that are often inherently unbalanced. This is the first study to present an optimisation framework for designing grid-connected DES while simultaneously considering multiphase optimal power flow (MOPF) constraints to accurately represent unbalanced low-voltage distribution. This study proposes a new algorithm for obtaining DES designs subject to nonlinear multiphase power flow constraints with regularised complementarity reformulations for operational constraints containing binary variables. DES design models with either MOPF or OPF are tested using an unbalanced distribution network, where rooftop solar, gas boilers, and battery storage are considered as distributed resources. Despite the increased complexity, DES design with MOPF obtains the best solution, with 81% greater solar capacity and 6% lower total annualised cost when compared to DES with OPF. Designs from a commonly used linear DES design framework based on direct current (DC) approximations produce the highest costs (8% more than initially predicted) when tested with MOPF. The proposed algorithm with complementarity reformulations achieves a 19% improvement in the objective value over a previous two-stage decomposition for DES with OPF, where the entire binary topology in the nonlinear model is fixed. The study therefore enables the acquisition of DES designs that can work symbiotically within unbalanced distribution networks.
The decarbonisation of residential heating systems has become increasingly important to meet the global goals of minimising carbon emissions and combating climate change. However, with rising energy costs, this can be a significant challenge for low-income households. This study presents a novel optimisation framework to aid the decarbonisation of residential heating in the United Kingdom by combining technology-related decision-support with policy decisions. The framework can recommend the optimal retrofit of low-carbon heating technologies and fabric improvement measures such as insulation upgrades for improving energy efficiency. Concurrently, the optimal financial contributions towards investment costs from grants supporting low-income households and social housing is determined. It also includes piecewise linearisations to capture the detailed operation of air source heat pumps, which are set to replace natural gas-based heating systems, and assesses the eligibility of each dwelling for grant funding. A large case study consisting of social housing stock in Woking, UK, has been used to test the framework. Three scenarios are used to assess the efficacy of existing technology and policy combinations to meet local emissions reduction targets, which are benchmarked against emissions from existing gas-based heating systems and insulation measures. Results highlight the limitations of existing UK grants, as these can only achieve an emissions reduction of 33.5% without incurring significant additional investment costs to the local council. The lack of support towards installing hot water tanks, which are required for the operation of heat pumps, is another major limitation in existing grants. A proposed scenario, which introduces a fictional grant with unlimited funding, sheds light on the much larger grant contributions expected to achieve an emissions reduction of 66.8%, which surpasses local targets. These results also suggest the need for operational support to cope with much higher energy bills, especially for low-income and/or fuel-poor households, due to the electrification of heating systems. Overall, the framework is a useful tool for local councils, policy makers, and other stakeholders to make informed decisions on the affordable decarbonisation of residential heating systems.
In many developing nations, the carbon intensity of the grid is high while capacity is also constrained. Local distributed energy resources are expensive to deploy and own by private households. This work presents an optimisation-based approach to optimally locate renewable technologies at both local prosumer’s dwellings and at community-level locations, incorporating the addition of distribution lines in a distributed energy system (DES) model to implement a solar photovoltaic (PV) energy farm with energy storage at the community level in densely populated urban areas. A case study of three scenarios in Karachi, Pakistan is selected to test the model. Scenario 1 presents a decentralised approach where every node has the possibility to install batteries locally. Scenario 2 considers a more centralised approach where only 2 central battery nodes are connected to different residential nodes. Lastly, the scalability of the model is tested with Scenario 3 which considers a model with 4 times as many nodes. The optimisation model indicates the profitability of different centralised/decentralised approaches with the highest level of decentralisation showing both the lowest cost and highest profit made, demonstrating the trade-off between battery sizing and line costs for privatised local energy storage.
Multi-period Heat Exchanger Networks (HENs) are designed as heat recovery energy efficient systems over a set of operating conditions for process streams. The problem becomes more complex when detailed exchanger designs are accounted for in the network synthesis problem. Typically, in mixed-integer nonlinear programming (MINLP) multi-period HEN optimisation, the maximum area heat exchanger across all periods is considered. However, when considering detailed designs, often this exchanger is unsuitable for operation over all periods. In this study, a trust-region algorithm is proposed to incorporate detailed exchanger designs for multi-period operation. The exchanger design is modelled using surrogate models inside a network-level NLP model which is derived from the multi-period MINLP HENS model solution. The method is applied to a case study and the results show the effectiveness of the proposed algorithm.
The year 2020 has seen the emergence of a global pandemic as a result of the disease COVID-19. This report reviews knowledge of the transmission of COVID-19 indoors, examines the evidence for mitigating measures, and considers the implications for wintertime with a focus on ventilation.
Residential heating displays huge decarbonisation potential towards Net-Zero. The complexity of heating system and socio-economic system appeals for a systematic design to avoid exacerbating fuel poverty. This study develops a three-layer heat-for-all model which integrates building stocks analysis, distributed heating system optimisation, economic and environmental impacts simulation to tackle heating decarbonisation and fuel poverty simultaneously. This whole system model is a powerful decision support tool that can help conceive heating decarbonisation strategies for wider regions and countries. More than 400,000 scenarios are created, considering the effects of future policy schemes (No Grant, Business as Usual, Proposed), minimum emission reduction target, carbon intensity of grid, future natural gas, and electricity prices. Results show that optimised heating system decarbonisation plan heavily relies on future energy prices. In the case study, only air source heat pumps are chosen when electricity price is lower than 3 times gas price. Secondly, investment in heating system could stimulate the greenhouse gas emission of whole supply chain, hedging the emission reduction achieved in heating system. This further reveals that life cycle thinking is imperative in GHG emission mitigation. Thirdly, electricity decarbonisation plays a vital role in achieving whole system emission reduction. The grid carbon intensity reduction makes substantial contribution to the emission reduction of heating system and industry system. In tackling fuel poverty, it's worth noticing that the fuel poverty is aggravated with more grant support under certain scenarios, since current policy schemes focus on capital investment in heating system but overlook the increased energy bills. It appeals for a more comprehensive policy design considering all stakeholders. [Display omitted] •A three-layer whole system approach to tackle heating decarbonisation and fuel poverty.•Optimised heating system decarbonisation plan heavily relies on future energy prices.•Investment in heating system stimulates the greenhouse gas emissions of whole supply chain.•Electricity decarbonisation plays a pivotal role in whole system emission reduction.•Fuel poverty will be exacerbated without better policy design.
Optimisation and simulation models for the design and operation of grid-connected distributed energy systems (DES) often exclude the inherent nonlinearities related to power flow and generation and storage units, to maintain an accuracy-complexity balance. Such models may provide sub-optimal or even infeasible designs and dispatch schedules. In DES, optimal power flow (OPF) is often misrepresented and treated as a standalone problem. OPF consists of highly nonlinear and nonconvex constraints related to the underlying alternating current (AC) distribution network. This aspect of the optimisation problem has often been overlooked by researchers in the process systems and optimisation area. In this review we address the disparity between OPF and DES models, highlighting the importance of including elements of OPF in DES design and operational models to ensure that the design and operation of microgrids meet the requirements of the underlying electrical grid. By analysing foundational models for both DES and OPF, we identify detailed technical power flow constraints that have been typically represented using oversimplified linear approximations in DES models. We also identify a subset of models, labelled DES-OPF, which include these detailed constraints and use innovative optimisation approaches to solve them. Results of these studies suggest that achieving feasible solutions with high-fidelity models is more important than achieving globally optimal solutions using less-detailed DES models. Recommendations for future work include the need for more comparisons between high-fidelity models and models with linear approximations, and the use of simulation tools to validate high-fidelity DES-OPF models. The review is aimed at a multidisciplinary audience of researchers and stakeholders who are interested in modelling DES to support the development of more robust and accurate optimisation models for the future.
Increasing deployment of renewable energy resources for power generation has been playing a pivotal role in reducing carbon emissions associated with electrical power systems. Distributed Energy Systems (DES) enable the integration of small-scale renewable energy resources and storage technologies within low-voltage (LV) distribution networks, which supply power to residential and commercial consumers. Care must be taken when designing these systems, as they could potentially impair the operation and infrastructure of existing power networks. While nonlinear balanced optimal power flow formulations have historically been incorporated into oversimplified Mixed-Integer Linear Programming (MILP) DES design models, these do not accurately model the distribution networks to which most DES are connected. Low-voltage radial distribution networks are most closely represented by nonconvex multi-phase formulations, which are computationally complex and difficult to solve. The exclusion of these constraints within DES design models could, however, lead to infeasible designs, i.e., designs which are incompatible with the existing network and its operations. This study proposes a novel optimisation algorithm, capable of solving the large-scale and combined problem of designing DES with multiphase optimal power flow. A large-scale Nonlinear Programming (NLP) model with full power flow constraints and reformulated complementarity constraints for DES operation is used to find a feasible upper bound, if the lower bound proposed by the MILP for DES design is infeasible. The algorithm is tested using a residential case study based on a section of the IEEE EU LV network. Results for this case study show that the proposed algorithm finds a feasible DES design and operational schedule by installing three times the battery capacity initially recommended by the MILP. The MILP design remains infeasible with respect to the multiphase power flow constraints. This framework could be used to support the increase of local renewable energy generation and consumption, and the subsequent reduction of carbon intensity in existing power networks.
The design and operation of distributed energy systems (DES) have often been modelled as linear optimisation problems. Although DES are increasingly connected to existing alternating current (AC) distribution networks, state-of-the-art DES modelling frameworks use oversimplified approximations which either exclude network constraints or overlook the inherent three-phase unbalance present in distribution networks. This can lead to poor designs which amplify network operational issues and result in greater costs to both the network and consumers/producers. This study presents a new modelling framework for DES design, which incorporates unbalanced optimal power flow within DES models for the first time. Furthermore, Robust Optimisation is included in this detailed modelling framework to ensure design feasibility under worst-case scenarios. Results show that previous frameworks tend to either overestimate or underestimate objectives when compared with the DES model combined with unbalanced power flow. Robust scenarios demonstrate that the new combined model is capable of closing the gap between objectives when compared with a linear DES-only model, albeit with different designs that do not violate grid constraints during baseline operation. These results suggest that this detailed framework can be utilised for DES design and network planning, as it produces more robust designs which can potentially help avert operational issues.
Gastric emptying (GE) is the process of food being processed by the stomach and delivered to the small intestine where nutrients such as lipids are absorbed into the blood circulation. The combination of an easy and inexpensive method to measure GE such as the CO2 breath test using the stable isotope [ 13 C]octanoic acid with semi-mechanistic modelling could foster a wider application in nutritional studies to further understand the metabolic response to food. Here, we discuss the use of the [ 13 C]octanoic acid breath test to label the solid phase of a meal, and the factors that influence GE to support mechanistic studies. Furthermore, we give an overview of existing mathematical models for the interpretation of the breath test data and how much nutritional studies could benefit from a physiological based pharmacokinetic model approach.