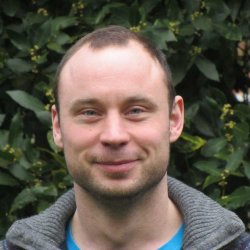
Ian Ackerley
Academic and research departments
Faculty of Engineering and Physical Sciences, Centre for Vision, Speech and Signal Processing (CVSSP).Publications
Positron Emission Tomography and Computed Tomography (PET-CT) is a vital imaging technique for accurate cancer diagnosis, staging, and treatment planning, offering complementary morphological and anatomical information. A 5-layer 3D convolutional deep learning texture model was employed to identify glycolytic regions in PET-CT data, achieving an average sensitivity and specificity of 96.1% and 99.4%, respectively, for binary classification targeting primary tumor patches. Using a dataset of PET-CT data from 486 esophageal patients, we analyzed network activations across each layer for characteristic activation patterns of four glycolytic uptake classes: primary tumor, bladder, liver, and myocardium. PCA analysis of the activations was performed to isolate uncorrelated features learned during training and reveal unique feature clusters in PCA space, demonstrating that glycolytic regions with high SUV values exhibit distinct textures learnable by deep learning architectures. This information was used to prune low activation probability nodes in the network, resulting in a more efficient deployable network with slightly improved classification performance. A comprehensive quantitative evaluation of redundant filters in the network, examining filter combinations that result in positive (tumor-present) and negative (tumor-absent) predictions across multiple patients will be presented, alongside preliminary results on the use of AI for automatic staging.