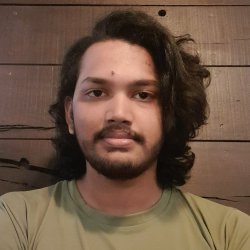
Hmrishav Bandyopadhyay
Academic and research departments
Centre for Vision, Speech and Signal Processing (CVSSP), Faculty of Engineering and Physical Sciences.About
My research project
VR sketch analysisFine-Grained VR Sketching Virtual Reality (VR) headsets and 3D printers rapidly make their way to consumer markets. With recent interest in virtual reality, VR sketching is gaining increasingly more popularity in industry and academia. My research is centered around identifying the potential of low-effort VR sketching to become a bridge to the practical adoption of 3D and VR-related technologies by average consumers and professional designers. In sketching research, the recent focus is on fine-grained tasks revolving around subtle intra-class differences. In particular, 2D sketches were proved to be efficient queries for 2D images fine-grained retrieval. Yet, in the context of the 3D shape retrieval from single or multiple 2D sketches the fine-grained performance was not demonstrated so far. 3D VR sketching in comparison allows to (1) alleviate the problem of a 2D projection ambiguity and (2) naturally evaluate and depict shapes & proportions.
Supervisors
Fine-Grained VR Sketching Virtual Reality (VR) headsets and 3D printers rapidly make their way to consumer markets. With recent interest in virtual reality, VR sketching is gaining increasingly more popularity in industry and academia. My research is centered around identifying the potential of low-effort VR sketching to become a bridge to the practical adoption of 3D and VR-related technologies by average consumers and professional designers. In sketching research, the recent focus is on fine-grained tasks revolving around subtle intra-class differences. In particular, 2D sketches were proved to be efficient queries for 2D images fine-grained retrieval. Yet, in the context of the 3D shape retrieval from single or multiple 2D sketches the fine-grained performance was not demonstrated so far. 3D VR sketching in comparison allows to (1) alleviate the problem of a 2D projection ambiguity and (2) naturally evaluate and depict shapes & proportions.
Publications
This paper, for the first time, marries large foundation models with human sketch understanding. We demonstrate what this brings – a paradigm shift in terms of generalised sketch representation learning (e.g., classification). This generalisation happens on two fronts: (i) generalisation across unknown categories (i.e., open-set), and (ii) generalisation traversing abstraction levels (i.e., good and bad sketches), both being timely challenges that remain unsolved in the sketch literature. Our design is intuitive and centred around transferring the already stellar generalisation ability of CLIP to benefit generalised learning for sketches. We first “condition” the vanilla CLIP model by learning sketchspecific prompts using a novel auxiliary head of raster to vector sketch conversion. This importantly makes CLIP “sketch-aware”. We then make CLIP acute to the inherently different sketch abstraction levels. This is achieved by learning a codebook of abstraction-specific prompt biases, a weighted combination of which facilitates the representation of sketches across abstraction levels – low abstract edge-maps, medium abstract sketches in TU-Berlin, and highly abstract doodles in QuickDraw. Our framework surpasses popular sketch representation learning algorithms in both zero-shot and few-shot setups and in novel settings across different abstraction boundaries.
We propose SketchINR, to advance the representation of vector sketches with implicit neural models. A variable length vector sketch is compressed into a latent space of fixed dimension that implicitly encodes the underlying shape as a function of time and strokes. The learned function predicts the $xy$ point coordinates in a sketch at each time and stroke. Despite its simplicity, SketchINR outperforms existing representations at multiple tasks: (i) Encoding an entire sketch dataset into a fixed size latent vector, SketchINR gives $60\times$ and $10\times$ data compression over raster and vector sketches, respectively. (ii) SketchINR's auto-decoder provides a much higher-fidelity representation than other learned vector sketch representations, and is uniquely able to scale to complex vector sketches such as FS-COCO. (iii) SketchINR supports parallelisation that can decode/render $\sim$$100\times$ faster than other learned vector representations such as SketchRNN. (iv) SketchINR, for the first time, emulates the human ability to reproduce a sketch with varying abstraction in terms of number and complexity of strokes. As a first look at implicit sketches, SketchINR's compact high-fidelity representation will support future work in modelling long and complex sketches.
In this paper, we democratise 3D content creation, enabling precise generation of 3D shapes from abstract sketches while overcoming limitations tied to drawing skills. We introduce a novel part-level modelling and alignment framework that facilitates abstraction modelling and cross-modal correspondence. Leveraging the same part-level decoder, our approach seamlessly extends to sketch modelling by establishing correspondence between CLIPasso edgemaps and projected 3D part regions, eliminating the need for a dataset pairing human sketches and 3D shapes. Additionally, our method introduces a seamless in-position editing process as a byproduct of cross-modal part-aligned modelling. Operating in a low-dimensional implicit space, our approach significantly reduces computational demands and processing time.
In this paper, we explore the unique modality of sketch for explainability, emphasising the profound impact of human strokes compared to conventional pixel-oriented studies. Beyond explanations of network behavior, we discern the genuine implications of explainability across diverse downstream sketch-related tasks. We propose a lightweight and portable explainability solution -- a seamless plugin that integrates effortlessly with any pre-trained model, eliminating the need for re-training. Demonstrating its adaptability, we present four applications: highly studied retrieval and generation, and completely novel assisted drawing and sketch adversarial attacks. The centrepiece to our solution is a stroke-level attribution map that takes different forms when linked with downstream tasks. By addressing the inherent non-differentiability of rasterisation, we enable explanations at both coarse stroke level (SLA) and partial stroke level (P-SLA), each with its advantages for specific downstream tasks.