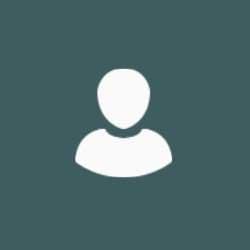
Dr Hermanus Draisma
About
Biography
I graduated from Leiden University in Leiden, The Netherlands in Biomedical Sciences in November 2005, on the topic of analysis of electrocardiographic and vectorcardiographic data (Dr. Cees Swenne). I then took on a PhD project at the Leiden/Amsterdam Centre for Drug Research in Leiden (funded by the Netherlands Bioinformatics Centre) with Profs. Thomas Hankemeier and Jan van der Greef in analysis of metabolomic data from twins and their families, which I completed in December 2010. I subsequently started working at the Netherlands Twin Register/Vrije Universiteit Amsterdam, Amsterdam, The Netherlands with Profs. Dorret Boomsma and Eco de Geus, mainly on various genetic and genome-wide association studies and heritability and familiality analyses. After a brief stint as a teacher Bioinformatics at the Leiden University of Applied Sciences, I joined the Leiden University Medical Center as a bioinformatic programmer, working on RNA-seq data analysis with Drs. Peter-Bram ’t Hoen and Szymon Kielbasa. In June 2017, I moved to Imperial College London, London, UK to work with Dr. Inga Prokopenko in her group within the Department of Medicine, section of Genomics of common disease (Prof. Philippe Froguel) on multivariate and longitudinal analysis of DNA methylation data, as part of a project funded by the Wellcome Trust. In April 2019, I moved together with Dr. Prokopenko to the University of Surrey to continue my work here.
News
In the media
ResearchResearch interests
I develop and apply statistical methods for epigenome- and genome-wide association analyses that leverage off the correlation among phenotypes to detect (epi)genotype-phenotype relationships. The epigenome comprises hereditary changes to the genetic code (DNA) present in human cells that do not affect the DNA sequence. Prominent examples of epigenetic changes are the addition to the DNA of particular chemical groups (methyl groups; thereby leading to so-called "methylation") and changes to the proteins (histones) associated with the DNA. The epigenetic profile of the DNA within a particular cell within a particular individual at a particular point in time affects whether the genes within that cell are turned 'on' or 'off'; the epigenome thereby acts as a kind of 'switch' to gene expression. As patterns of gene expression in turn may affect the values of detectable characteristics in humans (so-called "phenotypes"), one can imagine that indeed epigenetic profiles within the cells of an individual at a particular point in time influence that person’s phenotypic values.
One possible cause for correlations among different phenotypes at the epidemiological level is that variation in these phenotypes is caused by genetic variation in the same gene. Work from our group and others has shown that, in such cases, statistical methods for genotype-phenotype association that utilize the correlation among phenotypes ("multi-phenotype methods") will be more powerful in attempts to detect genotype-phenotype relationships than methods that consider each phenotype at a time. I investigate to which extent multi-phenotype methods for epigenotype-phenotype association have an analogous advantage over methods that consider each phenotype at a time.
In line with our group's continued interest in type 2 diabetes and in phenotypes related to this disease, my current focus in application of the multi-phenotype methods developed and implemented by our group is on glycaemic traits. These are phenotypes related to glucose metabolism, such as blood glucose and insulin measurements. They are of considerable relevance when studying the causes of diabetes, for instance because a number of genes driving variation in glycaemic traits seems to overlap with genes that are associated with the risk of type 2 diabetes. Moreover, in contrast to diabetes itself, one can study these glycaemic traits in all individuals from a particular population, thereby potentially enhancing the power to detect statistically significant associations.
Research interests
I develop and apply statistical methods for epigenome- and genome-wide association analyses that leverage off the correlation among phenotypes to detect (epi)genotype-phenotype relationships. The epigenome comprises hereditary changes to the genetic code (DNA) present in human cells that do not affect the DNA sequence. Prominent examples of epigenetic changes are the addition to the DNA of particular chemical groups (methyl groups; thereby leading to so-called "methylation") and changes to the proteins (histones) associated with the DNA. The epigenetic profile of the DNA within a particular cell within a particular individual at a particular point in time affects whether the genes within that cell are turned 'on' or 'off'; the epigenome thereby acts as a kind of 'switch' to gene expression. As patterns of gene expression in turn may affect the values of detectable characteristics in humans (so-called "phenotypes"), one can imagine that indeed epigenetic profiles within the cells of an individual at a particular point in time influence that person’s phenotypic values.
One possible cause for correlations among different phenotypes at the epidemiological level is that variation in these phenotypes is caused by genetic variation in the same gene. Work from our group and others has shown that, in such cases, statistical methods for genotype-phenotype association that utilize the correlation among phenotypes ("multi-phenotype methods") will be more powerful in attempts to detect genotype-phenotype relationships than methods that consider each phenotype at a time. I investigate to which extent multi-phenotype methods for epigenotype-phenotype association have an analogous advantage over methods that consider each phenotype at a time.
In line with our group's continued interest in type 2 diabetes and in phenotypes related to this disease, my current focus in application of the multi-phenotype methods developed and implemented by our group is on glycaemic traits. These are phenotypes related to glucose metabolism, such as blood glucose and insulin measurements. They are of considerable relevance when studying the causes of diabetes, for instance because a number of genes driving variation in glycaemic traits seems to overlap with genes that are associated with the risk of type 2 diabetes. Moreover, in contrast to diabetes itself, one can study these glycaemic traits in all individuals from a particular population, thereby potentially enhancing the power to detect statistically significant associations.