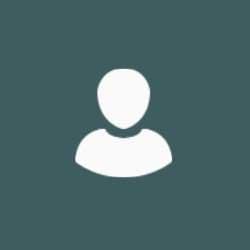
Dr Harry Holt
About
My research project
Trajectory design using state-dependent closed-loop control laws via reinforcement learningLow-thrust many-revolution trajectory design is becoming increasingly important with the development of high specific impulse, low-thrust engines. Closed-loop feedback-driven (CLFD) control laws allow the computation of sub-optimal trajectories with minimal computational cost. They treat the problem from a targeting perspective and, hence, value stability over optimality. In this research, a reinforcement learning framework is used to make the parameters of Lyapunov-based control laws state-dependent to increase their optimality whilst maintaining their stability and closed-loop nature. The major draw for reinforcement learning algorithms is their performance in unfamiliar environments and thus, we investigate how the performance of these control laws can be increased in high-fidelity environments where the dynamics are unknown to the controller and constantly evolving.
Low-thrust many-revolution trajectory design is becoming increasingly important with the development of high specific impulse, low-thrust engines. Closed-loop feedback-driven (CLFD) control laws allow the computation of sub-optimal trajectories with minimal computational cost. They treat the problem from a targeting perspective and, hence, value stability over optimality. In this research, a reinforcement learning framework is used to make the parameters of Lyapunov-based control laws state-dependent to increase their optimality whilst maintaining their stability and closed-loop nature. The major draw for reinforcement learning algorithms is their performance in unfamiliar environments and thus, we investigate how the performance of these control laws can be increased in high-fidelity environments where the dynamics are unknown to the controller and constantly evolving.