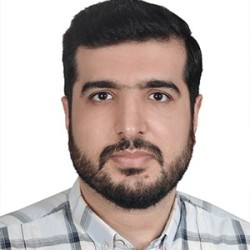
Dr Hamid Amiriara
Publications
In this paper, we address a physical layer security (PLS) framework for the integrated sensing and semantic communication (ISASC) system, where a multi-antenna dual-function semantic base station serves multiple single-antenna semantic communication users (SCUs) and monitors a malicious sensing target (MST), in the presence of a single-antenna eavesdropper (EVE), with both the MST and EVE aiming to wiretap information from the SCUs' signals. To enhance PLS, we employ joint artificial noise (AN) and dedicated sensing signal (DSS) in addition to wiretap coding. To evaluate the sensing accuracy, we derive the Cramer-Rao bound (CRB) as a function of the communication, sensing, and AN beamforming (BF) vectors. Subsequently, to assess the PLS level of the ISASC system, we determine a closed-form expression for the semantic secrecy rate (SSR). To achieve an optimal trade-off region between these two competing objectives, we formulate a multi-objective optimization problem for the joint design of the BF vectors. We apply semi-definite programming, Gaussian randomization method, and golden-section search techniques to address this problem. Simulation results demonstrate that the proposed scheme outperforms baseline schemes, achieving a superior trade-off between SSR and CRB.
This paper analyzes the performance of reconfigurable intelligent surface (RIS)-assisted device-to-device (D2D) communication systems, focusing on addressing co-channel interference, a prevalent issue due to the frequency reuse of sidelink in the underlay in-band D2D communications. In contrast to previous studies that either neglect interference or consider it only at the user, our research investigates a performance analysis in terms of outage probability (OP) for RIS-assisted D2D communication systems considering the presence of interference at both the user and the RIS. More specifically, we introduce a novel integral-form expression for an exact analysis of OP. Additionally, we present a new accurate approximation expression for OP, using the gamma distributions to approximate the fading of both desired and interference links, thereby yielding a closed-form expression. Nevertheless, both derived expressions, i.e., the exact integral-form and the approximate closed-form, contain special functions, such as Meijer’s G-function and the parabolic cylinder function, which complicate real-time OP analysis. To circumvent this, we employ a deep neural network (DNN) for real-time OP prediction, trained with data generated by the exact expression. Moreover, we present a tight upper bound that quantifies the impact of interference on achievable diversity order and coding gain. We validate the derived expressions through Monte Carlo simulations. Our analysis reveals that while interference does not affect the system’s diversity order, it significantly degrades the performance by reducing the coding gain. The results further demonstrate that increasing the number of RIS’s reflecting elements is an effective strategy to mitigate the adverse effects of the interference on the system performance.
This article addresses the joint power allocation and channel assignment (JPACA) problem in uplink non-orthogonal multiple access (NOMA) networks, an essential consideration for enhancing the performance of wireless communication systems. We introduce a novel methodology that integrates convex optimization (CO) and machine learning (ML) techniques to optimize resource allocation efficiently and effectively. Initially, we develop a CO-based algorithm that employs an alternating optimization strategy to iteratively solve for channel and power allocation, ensuring quality of service (QoS) while maximizing the system’s sum-rate. To overcome the inherent challenges of real-time application due to computational complexity, we further propose a ML-based approach that utilizes a stacking ensemble model combining convolutional neural network (CNN), feed-forward neural network (FNN), and random forest (RF). This model is trained on a dataset generated via the CO algorithm to predict optimal resource allocation in real-time scenarios. Simulation results demonstrate that our proposed methods not only reduce the computational load significantly but also maintain high system performance, closely approximating the results of more computationally intensive exhaustive search methods. The dual approach presented not only enhances computational efficiency but also aligns with the evolving demands of future wireless networks, marking a significant step towards intelligent and adaptive resource management in NOMA systems.
This paper presents exact formulas for the probability distribution function (PDF) and moment generating function (MGF) of the sum-product of statistically independent but not necessarily identically distributed (i.n.i.d.) Nakagami-m random variables (RVs) in terms of Meijer’s G-function. Additionally, exact series representations are also derived for the sum of double-Nakagami RVs, providing useful insights on the trade-off between accuracy and computational cost. Simple asymptotic analytical expressions are provided to gain further insight into the derived formula, and the achievable diversity order is obtained. The suggested statistical properties are proved to be a highly useful tool for modeling parallel cascaded Nakagami-m fading channels. The application of these new results is illustrated by deriving exact expressions and simple tight upper bounds for the outage probability (OP) and average symbol error rate (ASER) of several binary and multilevel modulation signals in intelligent reflecting surfaces (IRSs)-assisted communication systems operating over Nakagami-m fading channels. It is demonstrated that the new asymptotic expression is highly accurate and can be extended to encompass a wider range of scenarios. To validate the theoretical frameworks and formulations, Monte-Carlo simulation results are presented. Additionally, supplementary simulations are provided to compare the derived results with two common types of approximations available in the literature, namely the central limit theorem (CLT) and gamma distribution.
To achieve low probability of intercept (LPI) in radar networks for multiple target detection, it is necessary to find the optimal assignment of distributed radars to targets. The multi-radar to multi-target assignment (MRMTA) problem aims to find the best radar combination, but its brute-force (BF)-based approach over all possible sensor combinations has exponential complexity, making it challenging to implement in networks with a large number of radars or targets. This limits the implementation of the BF approach in networks that prioritize low latency and complexity. To address this challenge, we propose a supervised machine-learning (ML)-based solution for the MRMTA problem. Our proposed implementation scheme performs the training procedure offline, leading to a significant reduction in assignment complexity and processing latency. We conducted extensive numerical simulations to design an ML structure with high accuracy, convergence speed, and scalability. Simulation results demonstrate the efficiency and effectiveness of our proposed ML-based MRMTA solution, which achieves near-optimal LPI performance with considerably lower computation time than benchmark schemes. Our proposed solution has the potential to optimize the assignment of distributed radars to targets in LPI radar networks and improve the performance of complex networks with low latency and complexity requirements.
This article concentrates on the problem of associating an intelligent reflecting surface (IRS) to multiple users in a multiple-input single-output (MISO) downlink wireless communication network. The main objective of the paper is to maximize the sum-rate of all users by solving the joint optimization problem of the IRS-user association, IRS reflection, and BS beamforming, formulated as a non-convex mixed-integer optimization problem. The variable separation and relaxation are used to transform the problem into three convex sub-problems, which are alternatively solved through the convex optimization (CO) method. The major drawback of the proposed CO-based algorithm is high computational complexity. Thus, we make use of machine learning (ML) to tackle this problem. To this end, first, we convert the optimization problem into a regression problem. Then, we solve it with feed-forward neural networks (FNNs), trained by CO-based generated data. Simulation results show that the proposed ML-based algorithm has a performance equivalent to the CO-based algorithm, but with less computation complexity due to its offline training procedure.
The exact expressions and simple tight lower bounds for end-to-end average symbol error rate (ASER) and outage probability (OP) are derived in amplify-and-forward (AF) source-relay-destination cooperative link, provided that source-destination path is correlated with the source-relay path. Afterward, an optimum power allocation (PA) and relay location (RL) algorithm is presented. The effect of correlation factor and path-loss exponent (PLE) on the optimal nodes' power and location is investigated. The results show that optimizing relay location is more efficient than power allocation. Furthermore, a machine learning (ML) implementation of the proposed convex optimization-based algorithm is investigated to cop the computational burden. Specifically, the data set is obtained by using the proposed algorithm. Given the data set, the optimization algorithm can be translated into a regression problem, and feed-forward neural networks (FNNs) are then employed to solve this problem efficiently. The simulation results represented a compromise between accuracy and computation times for the ML-based joint PA-RL optimization.
The automatic identification of the modulation format of a detected signal is a major task of an intelligent receiver in both military and civilian applications. It is well known that the maximum likelihood (ML) classifier requires a priori knowledge of the incoming signal and channel (including amplitude, timing information, noise power, and the roll-off factor of the pulse-shaping filter). To relax this requirement, we introduce a novel estimator to estimate the parameters required by the ML classifier which is blind to the modulation scheme of the received signal, and this gives rise to a new blind modulation classifier for digital amplitude-phase modulated signals. While the proposed classifier is completely blind, the simulation results show that the performance of this classifier is very close to the optimal non-blind classifier.
Cooperative relay system (CRS) is an efficient wireless communication approach, with the advantage of creating diversity. In order to minimize the average symbol error rate (ASER) of a CRS with amplify-and-forward relaying protocol (AF-CRS) in the Nakagami-m fading channel, the optimum relay location and allocating optimal power is determined. To achieve this goal, the ASER for the AF-CRS in the Nakagami-m channel for different modulations is calculated. Then, three scenarios are considered. First, an algorithm, based on fixed point theory to determine the optimal location of the AF relay with a given power allocation for the source and relay, is proposed. Second, the problem of optimizing power allocation for different relay locations is solved. Finally, an algorithm for joint optimization of the power-location that leads to more efficient system operation, is proposed. In this paper, it is shown that the optimum power allocation and relay location is independent of the parameter of the Nakagami channel. Also, the effect of the path-loss exponent (PLE) on the optimal relay location in the CRS, which shows that the relay should be located closer to the source in dense channels (like near-Earth environments relative to free-space), is investigated. Finally, Simulations and numerical results, which confirm the theoretical achievements, are presented.
In this work, joint optimization of Power Allocation (PA) and Relay Location (RL) is investigated for Amplify-and-Forward (AF) cooperative relaying in order to minimize the Average Symbol Error Rate (ASER). First, a closed-form expression of the ASER is derived for different modulations in Rayleigh fading channel at a high signal-to-noise ratio regime based on moment generating function. Second, we optimize the RL for the AF cooperative relaying with any given power assignment at the source and the relay. Finally, we develop a closed-form explicit formula for joint RL and PA optimization which is a standard fix-point problem. Evaluation of the effect of path-loss exponent demonstrates that in a lossy channel, relay location closer to the source yields better performance. Finally, extensive numerical results and simulation comparisons confirm that the minimum ASER can be achieved via the joint PA-RL optimization.