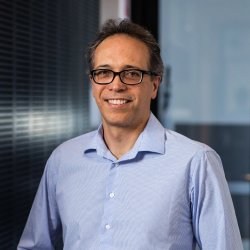
Professor Gustavo Carneiro
Academic and research departments
Centre for Vision, Speech and Signal Processing (CVSSP), School of Computer Science and Electronic Engineering.About
Biography
Gustavo Carneiro is a Professor of AI and Machine Learning at the University of Surrey, UK. Before that, from 2019 to 2022, he was a Professor at the School of Computer Science at the University of Adelaide, an ARC Future Fellow, and the Director of Medical Machine Learning at the Australian Institute of Machine Learning. He joined the University of Adelaide as a senior lecturer in 2011, has become an associate professor in 2015 and a professor in 2019. In 2014 and 2019, he joined the Technical University of Munich as a visiting professor and a Humboldt fellow. From 2008 to 2011 Dr. Carneiro was a Marie Curie IIF fellow and a visiting assistant professor at the Instituto Superior Tecnico (Lisbon, Portugal) within the Carnegie Mellon University-Portugal program (CMU-Portugal). From 2006 to 2008, Dr. Carneiro was a research scientist at Siemens Corporate Research in Princeton, USA. In 2005, he was a post-doctoral fellow at the University of British Columbia and at the University of California San Diego. Dr. Carneiro received his Ph.D. in computer science from the University of Toronto in 2004.
Areas of specialism
My qualifications
Previous roles
News
In the media
ResearchResearch interests
I have a wide-range of research interests. I am interested in applied research in medical image analysis and computer vision to theoretical research in machine learning.
Research projects
Current generic AI models for mammogram analysis provide biased results for patients and inflexible analysis for radiologists, reducing patients’ and radiologists’ trust in such models. In this project, we introduce an innovative design strategy for the development of new mammogram analysis AI models to increase their usability and trust by cooperating and personalising to radiologists and producing fair and accurate classification for all patient cohorts. In addition to measuring model accuracy for all patients, this proposal will introduce new assessment measures to evaluate the integration of the model into clinical practice in terms of radiologist’s performance improvement and workflow disruptiveness, and also to test the generalisation of the model to all patient sub-groups.
Delivering a workforce trained in the development of transformative technologies that will rapidly expand the Australian pharmaceutical, diagnostic and defence sector.
Development of new machine learning tools to help with the diagnosis of endometriosis from MRI and ultrasound
Development of new machine learning tools to allow the training of models using poorly curated training sets
Development of new medical image analysis methods for survival analysis and visual biomarker discovery
Development of new medical image analysis methods that can diagnose breast cancer from mammograms and MRI
One of the first methods in the field to use deep learning for the detection and segmentation of the left ventricle of the heart from ultrasound
Development of new deep learning methods for the quantification of tumour hypoxia from multi-modal microscopy images using weakly-supervised learning methods
Robotic Vision Australia is a national community of researchers and professionals, passionate about the potential for robotics, computer vision and AI to solve many of the world’s grand challenges. Robotic Vision Australia has been established to lead an agenda around Australia’s uptake of these innovative technologies and what we need to do to realise our potential as world leaders in this field.
This is a project that gathers researchers from the Instituto Superior Técnico, the Faculdade de Letras da Universidade de Lisboa, and the Museu Nacional do Azulejo with the purpose of designing a software to aid the study and the identification of Portuguese tile art.
Mainly due to the ease of reproduction and transportation, prints were used as the favoured means to make pictures and information available throughout the world. In this way, these art works quickly reached the hands of craftsman which used them as sources of inspiration, replicating them in different media, among which the tiles are particularly noteworthy. Trademark of Portuguese culture, the tiles have been produced continuously for five centuries, benefiting from the original prints in composition and theme, but using them freely; changing proportions, adding and removing figures, simplifying or enriching backgrounds, inverting figures, among other things.
The project aims to develop a tool that enables the cross-reference of information, matching prints and tiles, so as to identify the original sources of any given panel, as well as matching the tile panels and the figures portrayed in them. Another goal of the project is the creation of an annotated database, which will be available to help future research on Portuguese tile art.
Research interests
I have a wide-range of research interests. I am interested in applied research in medical image analysis and computer vision to theoretical research in machine learning.
Research projects
Current generic AI models for mammogram analysis provide biased results for patients and inflexible analysis for radiologists, reducing patients’ and radiologists’ trust in such models. In this project, we introduce an innovative design strategy for the development of new mammogram analysis AI models to increase their usability and trust by cooperating and personalising to radiologists and producing fair and accurate classification for all patient cohorts. In addition to measuring model accuracy for all patients, this proposal will introduce new assessment measures to evaluate the integration of the model into clinical practice in terms of radiologist’s performance improvement and workflow disruptiveness, and also to test the generalisation of the model to all patient sub-groups.
Delivering a workforce trained in the development of transformative technologies that will rapidly expand the Australian pharmaceutical, diagnostic and defence sector.
Development of new machine learning tools to help with the diagnosis of endometriosis from MRI and ultrasound
Development of new machine learning tools to allow the training of models using poorly curated training sets
Development of new medical image analysis methods for survival analysis and visual biomarker discovery
Development of new medical image analysis methods that can diagnose breast cancer from mammograms and MRI
One of the first methods in the field to use deep learning for the detection and segmentation of the left ventricle of the heart from ultrasound
Development of new deep learning methods for the quantification of tumour hypoxia from multi-modal microscopy images using weakly-supervised learning methods
Robotic Vision Australia is a national community of researchers and professionals, passionate about the potential for robotics, computer vision and AI to solve many of the world’s grand challenges. Robotic Vision Australia has been established to lead an agenda around Australia’s uptake of these innovative technologies and what we need to do to realise our potential as world leaders in this field.
This is a project that gathers researchers from the Instituto Superior Técnico, the Faculdade de Letras da Universidade de Lisboa, and the Museu Nacional do Azulejo with the purpose of designing a software to aid the study and the identification of Portuguese tile art.
Mainly due to the ease of reproduction and transportation, prints were used as the favoured means to make pictures and information available throughout the world. In this way, these art works quickly reached the hands of craftsman which used them as sources of inspiration, replicating them in different media, among which the tiles are particularly noteworthy. Trademark of Portuguese culture, the tiles have been produced continuously for five centuries, benefiting from the original prints in composition and theme, but using them freely; changing proportions, adding and removing figures, simplifying or enriching backgrounds, inverting figures, among other things.
The project aims to develop a tool that enables the cross-reference of information, matching prints and tiles, so as to identify the original sources of any given panel, as well as matching the tile panels and the figures portrayed in them. Another goal of the project is the creation of an annotated database, which will be available to help future research on Portuguese tile art.
Supervision
Postgraduate research supervision
I am currently supervising the following students and postdocs:
- Cuong Cao Nguyen (Research Associate at the University of Surrey, main supervisor)
- Tahir Hassan (Research Associate at the University of Surrey, main supervisor)
- Yuan Zhang (Ph.D. student at the University of Adelaide, main supervisor)
- Arpit Garg (Ph.D. student at the University of Adelaide, main supervisor)
- Dileepa Pitawela (Ph.D. student at the University of Adelaide, co-supervisor)
- Will Tapper (Ph.D. student at the University of Surrey, main supervisor)
- Zheng Zhang (Ph.D. student at the University of Surrey, main supervisor)
- David Butler (Ph.D. student at the University of Surrey, main supervisor)
- Nivedita Bijlani (Ph.D. student at the University of Surrey, co-supervisor)
- Nilesh Ramgolam (MPhil student at the University of Adelaide, co-supervisor)
- Wenjie Ai (Ph.D. student at the University of Surrey, main supervisor)
- Jamal Hashem (Ph.D. student at the University of Surrey, co-supervisor)
- Billy Vale (Ph.D. student at the University of Surrey, co-supervisor)
- Amirhossein Khakpour (Ph.D. student at the University of Surrey, main supervisor)
Postgraduate research supervision
I successfully supervised the following students and postdocs:
- Yuanhong Chen (Ph.D. main supervisor at the University of Adelaide, graduated in November 2024)
- Yanyan Wang (CSC Ph.D. visiting student at the University of Surrey, main supervisor in 2023-24)
- Yuyuan Liu (Ph.D. main supervisor at the University of Adelaide, graduated in October 2024 - received the Dean’s Commendation for Doctoral Thesis Excellence - Congrats)
- Chong Wang (Ph.D. main supervisor at the University of Adelaide, graduated in October 2024 - received the Dean’s Commendation for Doctoral Thesis Excellence - Congrats)
- Hu Wang (Research Associate at the University of Adelaide, main supervisor from 2021 to 2024)
- Adrian Galdran (Marie Curie Research Fellow at the Universitat Pompeu Fabra and University of Adelaide from 2022 to 2024)
- Cuong Cao Nguyen (Research Associate at the University of Adelaide, main supervisor from 2022 to 2024)
- Fengbei Liu (Ph.D. main supervisor at the University of Adelaide, graduated in January 2024 - received the Dean’s Commendation for Doctoral Thesis Excellence - Congrats Fengbei)
- Dung Anh Hoang (MPhil main supervisor at the University of Adelaide, graduated in July 2023)
- Renato Hermoza Aragones (Ph.D. main supervisor at the University of Adelaide, graduated in September 2022 - received the Dean’s Commendation for Doctoral Thesis Excellence - Congrats Renato)
- Yu Tian (Ph.D. main supervisor at the University of Adelaide, graduated in June 2022)
- Lauren Oakden-Rayner (Ph.D. co-supervisor at the University of Adelaide, graduated in March 2022 - received the Dean’s Commendation for Doctoral Thesis Excellence - Congrats Lauren)
- Cuong Cao Nguyen (Ph.D. main supervisor at the University of Adelaide, graduated in February 2022)
- Brandon Smart (Honour student main supervisor at the University of Adelaide, graduated in December 2021)
- Alireza Seyed Shakeri (Master student main supervisor at the University of Adelaide, finish project in December 2021)
- Hossein Askari (MPhil main supervisor at the University of Adelaide, main supervisor, graduated in October 2021)
- Artur Banach (Ph.D. co-supervisor student at Queensland University of Technology, graduated in October 2021)
- Yuan Zhang (Master student main supervisor at the University of Adelaide, graduated in July 2021)
- Wenping Du (Master student main supervisor at the University of Adelaide, graduated in July 2021)
- Gabriel Maicas (Research Associate main supervisor at the University of Adelaide from 2019 to 2021, main supervisor)
- Ragav Sachdeva (Honour student main supervisor at the University of Adelaide, graduated in December 2020)
- Yuanhong Chen (Honour student main supervisor at the University of Adelaide, graduated in December 2020)
- Hoang Son Le (Honour student main supervisor at the University of Adelaide, graduated in December 2020)
- Zachri Prinsloo (Honour student co-supervisor at the University of Adelaide, graduated in December 2020)
- Filipe Cordeiro (Prof. from UFRPE-Brazil, spent a sabbatical leave at the University of Adelaide from 2019 to 2020)
- Adrian Johnston (Ph.D. main supervisor at the University of Adelaide, graduated in September 2020)
- Fabio Faria (Prof. from UNIFESP-Brazil, spent a sabbatical leave at the University of Adelaide from 2019 to 2020)
- Michele Sasdelli (Research Associate main supervisor at the University of Adelaide from 2018 to 2020)
- Leonardo Zorron Cheng Tao Pu (Ph.D. co-supervisor at the University of Adelaide, graduated in July 2020 - received the Dean’s Commendation for Doctoral Thesis Excellence - Congrats Leo)
- Sam Karem (Master student main supervisor at the University of Adelaide, graduated in July 2020)
- Aniruddha Biswas (Master student main supervisor at the University of Adelaide, graduated in July 2020)
- Rafael Felix (Ph.D. main supervisor at the University of Adelaide, graduated in February 2020 - received the Dean’s Commendation for Doctoral Thesis Excellence - Congrats Rafael!)
- Toan Minh Tran (Ph.D. main supervisor at the University of Adelaide, graduated in January 2020)
- Fengbei Liu (Honours main supervisor at the University of Adelaide, graduated in December 2019)
- Yuyuan Liu (Honours main supervisor at the University of Adelaide, graduated in December 2019)
- Saskia Glaser (Master student main supervisor at the University of Adelaide, graduated in December 2019)
- Farbod Motlagh (Honours main supervisor at the University of Adelaide, graduated in July 2019)
- Jerome Williams (MPhil main supervisor at the University of Adelaide, graduated in August 2019)
- Yu Tian (Honours student main supervisor at the University of Adelaide - graduated in December 2018)
- Gabriel Maicas (Ph.D. main supervisor at the University of Adelaide, graduated in December 2018 - received the Dean’s Commendation for Doctoral Thesis Excellence - Congrats Gabriel!)
- Vijay Kumar (Post-doc main supervisor at the University of Adelaide from 2016 to 2018 - now at Xerox PARC)
- William Gale (Honours student main supervisor at the University of Adelaide - graduated in December 2017, working at Microsoft Research. William was awarded the National iAwards for Undergraduate Tertiary Students for his honours project - Congrats William!)
- Zhibin Liao (Ph.D. main supervisor at the University of Adelaide - graduated in September 2017 - After graduation, went to a postdoc at the University of British Columbia)
- Christian Lee (Masters student main supervisor at the University of Adelaide - graduated in August 2017)
- Andrew Lang (Honours student main supervisor at the University of Adelaide - graduated in November 2016) - check video of Andrew's project!
- Neeraj Dhungel (Ph.D. main supervisor at the University of Adelaide - graduated in October 2016 - Now a postdoc at the University of British Columbia)
- Zhi Lu (Visiting Ph.D student main supervisor in 2013 and Post-Doc at the University of Adelaide in 2014-2015. Now a post-doc at the University of South Australia)
- Tuan Anh Ngo (Ph.D. main supervisor at the University of Adelaide - graduated in August 2015 - received the Dean’s Commendation for Doctoral Thesis Excellence. Now a Professor at the Vietnam National University of Agriculture)
- Tom Schultz (Honours student main supervisor at the University of Adelaide - graduated in December 2014)
- Ren Ding (Honours student main supervisor at the University of Adelaide - graduated in July 2014)
- Qian Chen (Masters student main supervisor at the University of Adelaide - graduated in July 2014)
- Zhe Yin (Masters student main supervisor at the University of Adelaide - graduated in July 2014)
- Zhibin Liao (Honours main supervisor at the University of Adelaide - graduated in July/2013. Now a Ph.D. student)
- Xuance Wang (Honours co-supervisor at the University of Adelaide - graduated in December 2013)
- Danilo Dell'Agnello (Post Doc main supervisor at the University of Adelaide in 2012-13)
- Nuno Miguel Pinho da Silva (Post Doc co-supervisor at the Instituto Superior Tecnico, University of Lisbon in 2011-12).
- Joao Tiago Fonseca (M.Sc. co-supervisor at the Instituto Superior Tecnico, Technical University of Lisbon in 2011-12)
- Michael Wels (Siemens Ph.D. Student in 2008). Now at Siemens Healthcare.
- Fernando Amat (Siemens Intern main supervisor in 2007). Now at Janelia Farm Research Campus.
- Guillaume Heusch (Siemens Intern main supervisor in 2007-08).
Teaching
Courses taught at the University of Surrey, UK
- Artificial Intelligence - COM2028 (S2, 2024)
Courses taught at the University of Adelaide, Australia
- Grand Challenges in Computer Science (S2, 2022)
- Grand Challenges in Computer Science (S2, 2021)
- Grand Challenges in Computer Science (S2, 2020)
- Topics in Computer Science (S2, 2019)
- Grand Challenges in Computer Science (S2, 2019)
- Topics in Computer Science (S2, 2018)
- Grand Challenges in Computer Science (S2, 2018)
- Topics in Computer Science (S1, 2018)
- Puzzle-based Learning (S1, 2018)
- Artificial Intelligence (S1, 2018)
- Computer Graphics (S1, 2018) - Videos of Best Projects
- Grand Challenges (S2, 2018)
- Topics in Computer Science (Spring 2017)
- Computer Graphics (Fall 2017) - Videos of Best Projects
- Topics in Computer Science (Fall 2017)
- Advanced Topics in Computer Science (Fall 2017)
- Puzzle-Based Learning (Fall 2017)
- Introduction to Geometric Programming (Spring 2016)
- Topics in Computer Science (Spring 2016)
- Puzzle-Based Learning (Fall 2016)
- Topics in Computer Science (Fall 2016)
- Object Oriented Programming (Spring 2015)
- Topics in Computer Science (Spring 2015)
- Computer Graphics (Fall 2015) - Videos of Best Projects
- Puzzle-Based Learning (Fall 2015)
- Topics in Computer Science (Fall 2015)
- Puzzle-Based Learning (Fall 2014)
- Software Engineering in Industry (Fall 2014)
- Topics in Computer Science (Fall 2014)
- Puzzle-Based Learning (Spring 2013)
- Software Engineering Group Project 1B (Spring 2013)
- Master of Software Engineering Project (Spring 2013)
- Computer Graphics (Fall 2013) - Videos of Best Projects
- Puzzle-Based Learning (Fall 2013)
- Puzzle-Based Learning (Spring 2012)
- Computer Vision (Fall 2012)
- Computer Graphics (Fall 2012) - Videos of Best Projects
Courses I taught at the Instituto Superior Tecnico, Portugal
- Signals and Systems (Fall 2009)
- Robotics (Spring 2009)
- Modeling and Simulation (Spring 2009)
- Signal Processing (Fall 2008)
- Control (Fall 2008)
Courses I taught at the University of Toronto, Canada
- CSC 324 - Principles of Programming Languages (Fall 2004)
- CSC 446, Computer Methods for Partial Differential Equations (TA) (Winter 2002).
- CSC 418, Computer Graphics (TA) (1999-2003).
- CSC 458, Computer Networks (TA) (Winter 2000).
- CSC 258, Computer Organization (TA) (Summer 2000).
- CSC 260, An Introduction to Scientific, Symbolic, and Graphical Computation (TA) (Winter 2003).
- SCI 199, Computer and Images. (TA) (2000-2001)
Publications
Highlights
For an up-to-date list of my publications, please visit my Google Scholar page.
Six papers accepted to ECCV 2024
One paper accepted to CVPR 2024
Two papers published in Medical Image Analysis in 2024
One paper published in IEEE TMI in 2024
One paper accepted to NeurIPS 2023
Artificial intelligence (AI) readers of mammograms compare favourably to individual radiologists in detecting breast cancer. However, AI readers cannot perform at the level of multi-reader systems used by screening programs in countries such as Australia, Sweden, and the UK. Therefore, implementation demands human-AI collaboration. Here, we use a large, high-quality retrospective mammography dataset from Victoria, Australia to conduct detailed simulations of five potential AI-integrated screening pathways, and examine human-AI interaction effects to explore automation bias. Operating an AI reader as a second reader or as a high confidence filter improves current screening outcomes by 1.9-2.5% in sensitivity and up to 0.6% in specificity, achieving 4.6-10.9% reduction in assessments and 48-80.7% reduction in human reads. Automation bias degrades performance in multi-reader settings but improves it for single-readers. This study provides insight into feasible approaches for AI-integrated screening pathways and prospective studies necessary prior to clinical adoption.
With the development of Human-AI Collaboration in Classification (HAI-CC), integrating users and AI predictions becomes challenging due to the complex decision-making process. This process has three options: 1) AI autonomously classifies, 2) learning to complement, where AI collaborates with users, and 3) learning to defer, where AI defers to users. Despite their interconnected nature, these options have been studied in isolation rather than as components of a unified system. In this paper, we address this weakness with the novel HAI-CC methodology, called Learning to Complement and to Defer to Multiple Users (LECODU). LECODU not only combines learning to complement and learning to defer strategies, but it also incorporates an estimation of the optimal number of users to engage in the decision process. The training of LECODU maximises classification accuracy and minimises collaboration costs associated with user involvement. Comprehensive evaluations across real-world and synthesized datasets demonstrate LECODU's superior performance compared to state-of-the-art HAI-CC methods. Remarkably, even when relying on unreliable users with high rates of label noise, LECODU exhibits significant improvement over both human decision-makers alone and AI alone.
Background and Aim: Various deep learning models, based on convolutional neural network (CNN), have been shown to improve the detection of early esophageal neoplasia in Barrett's esophagus. Vision transformer (ViT), derived from natural language processing, has emerged as the new state-of-the-art for image recognition, outperforming predecessors such as CNN. This pilot study explores the use of ViT to classify the presence or absence of early esophageal neoplasia in endoscopic images of Barrett's esophagus. Methods: A BO dataset of 1918 images of Barrett's esophagus from 267 unique patients was used. The images were classified as dysplastic (D-BO) or non-dysplastic (ND-BO). A pretrained vision transformer model, ViTBase16, was used to develop our classifier models. Three ViT models were developed for comparison based on imaging modality: white-light imaging (WLI), narrow-band imaging (NBI), and combined modalities. Performance of each model was evaluated based on accuracy, sensitivity, specificity, confusion matrices, and receiver operating characteristic curves. Results: The ViT models demonstrated the following performance: WLI-ViT (Accuracy: 92%, Sensitivity: 82%, Specificity: 95%), NBI-ViT (Accuracy: 99%, Sensitivity: 97%, Specificity: 99%), and combined modalities-ViT (Accuracy: 93%, Sensitivity: 87%, Specificity: 95%). Combined modalities-ViT showed greater accuracy (94% vs 90%) and sensitivity (80% vs 70%) compared with WLI-ViT when classifying WLI images on a subgroup testing set. Conclusion: ViT exhibited high accuracy in classifying the presence or absence of EON in endoscopic images of Barrett's esophagus. ViT has the potential to be widely applicable to other endoscopic diagnoses of gastrointestinal diseases.
The efficacy of deep learning-based Computer-Aided Diagnosis (CAD) methods for skin diseases relies on analyzing multiple data modalities (i.e., clinical+dermoscopic images, and patient metadata) and addressing the challenges of multi-label classification. Current approaches tend to rely on limited multi-modal techniques and treat the multi-label problem as a multiple multi-class problem, overlooking issues related to imbalanced learning and multi-label correlation. This paper introduces the innovative Skin Lesion Classifier, utilizing a Multi-modal Multi-label TransFormer-based model (SkinM2Former). For multi-modal analysis, we introduce the Tri-Modal Cross-attention Transformer (TMCT) that fuses the three image and metadata modalities at various feature levels of a transformer encoder. For multi-label classification, we introduce a multi-head attention (MHA) module to learn multi-label correlations, complemented by an optimisation that handles multi-label and imbalanced learning problems. SkinM2Former achieves a mean average accuracy of 77.27% and a mean diagnostic accuracy of 77.85% on the public Derm7pt dataset, outperforming state-of-the-art (SOTA) methods.
Endometriosis, affecting about 10% of individuals assigned female at birth, is challenging to diagnose and manage. Diagnosis typically involves the identification of various signs of the disease using either laparoscopic surgery or the analysis of T1/T2 MRI images, with the latter being quicker and cheaper but less accurate. A key diagnostic sign of endometriosis is the obliteration of the Pouch of Douglas (POD). However, even experienced clinicians struggle with accurately classifying POD obliteration from MRI images, which complicates the training of reliable AI models. In this paper, we introduce the Human-AI Collaborative Multi-modal Multi-rater Learning (HAICOMM) methodology to address the challenge above. HAICOMM is the first method that explores three important aspects of this problem: 1) multi-rater learning to extract a cleaner label from the multiple "noisy" labels available per training sample; 2) multi-modal learning to leverage the presence of T1/T2 MRI images for training and testing; and 3) human-AI collaboration to build a system that leverages the predictions from clinicians and the AI model to provide more accurate classification than standalone clinicians and AI models. Presenting results on the multi-rater T1/T2 MRI endometriosis dataset that we collected to validate our methodology, the proposed HAICOMM model outperforms an ensemble of clinicians, noisy-label learning models, and multi-rater learning methods.
Acquiring fine-grained object detection annotations in unconstrained images is time-consuming, expensive, and prone to noise, especially in crowdsourcing scenarios. Most prior object detection methods assume accurate annotations; A few recent works have studied object detection with noisy crowdsourced annotations, with evaluation on distinct synthetic crowdsourced datasets of varying setups under artificial assumptions. To address these algorithmic limitations and evaluation inconsistency, we first propose a novel Bayesian Detector Combination (BDC) framework to more effectively train object detectors with noisy crowdsourced annotations, with the unique ability of automatically inferring the annotators' label qualities. Unlike previous approaches, BDC is model-agnostic, requires no prior knowledge of the annotators' skill level, and seamlessly integrates with existing object detection models. Due to the scarcity of real-world crowdsourced datasets, we introduce large synthetic datasets by simulating varying crowdsourcing scenarios. This allows consistent evaluation of different models at scale. Extensive experiments on both real and synthetic crowdsourced datasets show that BDC outperforms existing state-of-the-art methods, demonstrating its superiority in leveraging crowdsourced data for object detection. Our code and data are available at https://github.com/zhiqin1998/bdc.
Post-Training Quantization (PTQ) has received significant attention because it requires only a small set of calibration data to quantize a full-precision model, which is more practical in real-world applications in which full access to a large training set is not available. However, it often leads to overfitting on the small calibration dataset. Several methods have been proposed to address this issue, yet they still rely on only the calibration set for the quantization and they do not validate the quantized model due to the lack of a validation set. In this work, we propose a novel meta-learning based approach to enhance the performance of post-training quantization. Specifically, to mitigate the overfitting problem, instead of only training the quantized model using the original calibration set without any validation during the learning process as in previous PTQ works, in our approach, we both train and validate the quantized model using two different sets of images. In particular, we propose a meta-learning based approach to jointly optimize a transformation network and a quantized model through bi-level optimization. The transformation network modifies the original calibration data and the modified data will be used as the training set to learn the quantized model with the objective that the quantized model achieves a good performance on the original calibration data. Extensive experiments on the widely used ImageNet dataset with different neural network architectures demonstrate that our approach outperforms the state-of-the-art PTQ methods.
The costly and time-consuming annotation process to produce large training sets for modelling semantic LiDAR segmentation methods has motivated the development of semi-supervised learning (SSL) methods. However, such SSL approaches often concentrate on employing consistency learning only for individual LiDAR representations. This narrow focus results in limited perturbations that generally fail to enable effective consistency learning. Additionally, these SSL approaches employ contrastive learning based on the sampling from a limited set of positive and negative embedding samples. This paper introduces a novel semi-supervised LiDAR semantic segmentation framework called ItTakesTwo (IT2). IT2 is designed to ensure consistent predictions from peer LiDAR representations, thereby improving the perturbation effectiveness in consistency learning. Furthermore, our contrastive learning employs informative samples drawn from a distribution of positive and negative embeddings learned from the entire training set. Results on public benchmarks show that our approach achieves remarkable improvements over the previous state-of-the-art (SOTA) methods in the field. The code is available at: https://github.com/yyliu01/IT2.
This paper proposes a novel AI model that automatically assesses the quality of transvaginal ultrasound (TVUS) images, offering support to sonographers, especially those still learning, in acquiring high-quality scans for gynecological pathology diagnosis. Addressing the challenge of varying interpretations by different medical professionals, this model approaches the issue as a multi-annotator noisy label problem. Our novel machine learning architecture first aggregates quality assessments from multiple raters using a weighted ensemble algorithm to estimate consensus labels. The model then employs a multi-axis vision transformer to enhance the process of image quality evaluation. We evaluated the model on a new multi-annotator TVUS dataset, where our model successfully predicted image quality with an accuracy of 80%. This development represents an exciting first step in empowering sonographers to assess scan quality on the spot, reduce the need for repeated imaging, and improve the diagnosis of gynecological pathology.
Audio-visual segmentation (AVS) is an emerging task that aims to accurately segment sounding objects based on audio-visual cues. The success of AVS learning systems depends on the effectiveness of cross-modal interaction. Such a requirement can be naturally fulfilled by leveraging transformer-based segmentation architecture due to its inherent ability to capture long-range dependencies and flexibility in handling different modalities. However, the inherent training issues of transformer-based methods, such as the low efficacy of cross-attention and unstable bipartite matching, can be amplified in AVS, particularly when the learned audio query does not provide a clear semantic clue. In this paper, we address these two issues with the new Class-conditional Prompting Machine (CPM). CPM improves the bipartite matching with a learning strategy combining class-agnostic queries with class-conditional queries. The efficacy of cross-modal attention is upgraded with new learning objectives for the audio, visual and joint modalities. We conduct experiments on AVS benchmarks, demonstrating that our method achieves state-of-the-art (SOTA) segmentation accuracy.
With the development of Human-AI Collaboration in Classification (HAI-CC), integrating users and AI predictions becomes challenging due to the complex decision-making process. This process has three options: 1) AI autonomously classifies, 2) learning to complement, where AI collaborates with users, and 3) learning to defer, where AI defers to users. Despite their interconnected nature, these options have been studied in isolation rather than as components of a unified system. In this paper, we address this weakness with the novel HAI-CC methodology, called Learning to Complement and to Defer to Multiple Users (LECODU). LECODU not only combines learning to complement and learning to defer strategies, but it also incorporates an estimation of the optimal number of users to engage in the decision process. The training of LECODU maximises classification accuracy and minimises collaboration costs associated with user involvement. Comprehensive evaluations across real-world and synthesized datasets demonstrate LECODU’s superior performance compared to state-of-the-art HAI-CC methods. Remarkably, even when relying on unreliable users with high rates of label noise, LECODU exhibits significant improvement over both human decision-makers alone and AI alone (Supported by the Engineering and Physical Sciences Research Council (EPSRC) through grant EP/Y018036/1). Code is available at https://github.com/zhengzhang37/LECODU.git.
Post-Training Quantization (PTQ) has received significant attention because it requires only a small set of calibration data to quantize a full-precision model, which is more practical in real-world applications in which full access to a large training set is not available. However, it often leads to overfitting on the small calibration dataset. Several methods have been proposed to address this issue, yet they still rely on only the calibration set for the quantization and they do not validate the quantized model due to the lack of a validation set. In this work, we propose a novel meta-learning based approach to enhance the performance of post-training quantization. Specifically, to mitigate the overfitting problem, instead of only training the quantized model using the original calibration set without any validation during the learning process as in previous PTQ works, in our approach, we both train and validate the quantized model using two different sets of images. In particular, we propose a meta-learning based approach to jointly optimize a transformation network and a quantized model through bi-level optimization. The transformation network modifies the original calibration data and the modified data will be used as the training set to learn the quantized model with the objective that the quantized model achieves a good performance on the original calibration data. Extensive experiments on the widely used ImageNet dataset with different neural network architectures demonstrate that our approach outperforms the state-of-the-art PTQ methods.
Audio-visual segmentation (AVS) is an emerging task that aims to accurately segment sounding objects based on audio-visual cues. The success of AVS learning systems depends on the effectiveness of cross-modal interaction. Such a requirement can be naturally fulfilled by leveraging transformer-based segmentation architecture due to its inherent ability to capture long-range dependencies and flexibility in handling different modalities. However, the inherent training issues of transformer-based methods, such as the low efficacy of cross-attention and unstable bipartite matching, can be amplified in AVS, particularly when the learned audio query does not provide a clear semantic clue. In this paper, we address these two issues with the new Class-conditional Prompting Machine (CPM). CPM improves the bipartite matching with a learning strategy combining class-agnostic queries with class-conditional queries. The efficacy of cross-modal attention is upgraded with new learning objectives for the audio, visual and joint modalities. We conduct experiments on AVS benchmarks, demonstrating that our method achieves state-of-the-art (SOTA) segmentation accuracy (This project is supported by the Australian Research Council (ARC) through grant FT190100525.).
The costly and time-consuming annotation process to produce large training sets for modelling semantic LiDAR segmentation methods has motivated the development of semi-supervised learning (SSL) methods. However, such SSL approaches often concentrate on employing consistency learning only for individual LiDAR representations. This narrow focus results in limited perturbations that generally fail to enable effective consistency learning. Additionally, these SSL approaches employ contrastive learning based on the sampling from a limited set of positive and negative embedding samples. This paper introduces a novel semi-supervised LiDAR semantic segmentation framework called ItTakesTwo (IT2). IT2 is designed to ensure consistent predictions from peer LiDAR representations, thereby improving the perturbation effectiveness in consistency learning. Furthermore, our contrastive learning employs informative samples drawn from a distribution of positive and negative embeddings learned from the entire training set. Results on public benchmarks show that our approach achieves remarkable improvements over the previous state-of-the-art (SOTA) methods in the field. https://github.com/yyliu01/IT2.
Deep learning faces a formidable challenge when handling noisy labels, as models tend to overfit samples affected by label noise. This challenge is further compounded by the presence of instance-dependent noise (IDN), a realistic form of label noise arising from ambiguous sample information. To address IDN, Label Noise Learning (LNL) incorporates a sample selection stage to differentiate clean and noisy-label samples. This stage uses an arbitrary criterion and a pre-defined curriculum that initially selects most samples as noisy and gradually decreases this selection rate during training. Such curriculum is sub-optimal since it does not consider the actual label noise rate in the training set. This paper addresses this issue with a new noise-rate estimation method that is easily integrated with most state-of-the-art (SOTA) LNL methods to produce a more effective curriculum. Synthetic and real-world benchmarks’ results demonstrate that integrating our approach with SOTA LNL methods improves accuracy in most cases. (Code is available at https://github.com/arpit2412/NoiseRateLearning. Supported by the Engineering and Physical Sciences Research Council (EPSRC) through grant EP/Y018036/1 and the Australian Research Council (ARC) through grant FT190100525.)
An important stage of most state-of-the-art (SOTA) noisy-label learning methods consists of a sample selection procedure that classifies samples from the noisy-label training set into noisy-label or clean-label subsets. The process of sample selection typically consists of one of the two approaches: loss-based sampling, where high-loss samples are considered to have noisy labels, or feature-based sampling, where samples from the same class tend to cluster together in the feature space and noisy-label samples are identified as anomalies within those clusters. Empirically, loss-based sampling is robust to a wide range of noise rates, while feature-based sampling tends to work effectively in particular scenarios, e.g., the filtering of noisy instances via their eigenvectors (FINE) sampling exhibits greater robustness in scenarios with low noise rates, and the K nearest neighbour (KNN) sampling mitigates better high noise-rate problems. This paper introduces the Adaptive Nearest Neighbours and Eigenvector-based (ANNE) sample selection methodology, a novel approach that integrates loss-based sampling with the feature-based sampling methods FINE and Adaptive KNN to optimize performance across a wide range of noise rate scenarios. ANNE achieves this integration by first partitioning the training set into high-loss and low-loss sub-groups using loss-based sampling. Subsequently, within the low-loss subset, sample selection is performed using FINE, while the high-loss subset employs Adaptive KNN for effective sample selection. We integrate ANNE into the noisy-label learning state of the art (SOTA) method SSR+, and test it on CIFAR-10/-100 (with symmetric, asymmetric and instance-dependent noise), Webvision and ANIMAL-10, where our method shows better accuracy than the SOTA in most experiments, with a competitive training time. The code is available at https://github.com/filipe-research/anne.
Background and Aims: Currently, it takes an average of 6.4 years to obtain a diagnosis for endometriosis. To address this delay, the IMAGENDO project combines ultrasounds (eTVUS) and Magnetic Resonance Images (eMRI) using Artificial Intelligence (AI). Our preliminary data has shown that a multimodal AI approach objectively assesses imaging data from eTVUS and eMRIs and improves diagnostic accuracy for endometriosis. Our first example shows how automated detection of Pouch of Douglas (POD) obliteration can be augmented by a novel multimodal approach. Aim: To improve eMRI detection of POD obliteration, by leveraging detection results from unpaired eTVUS data. Method: Retrospective specialist private and public imaging datasets of the female pelvis were obtained. After pre-training a machine learning model using 8,984 MRIs from a public dataset, we fine-tuned the algorithm using 88 private eMRIs, to detect POD obliteration. We then introduced another 749 unpaired eTVUSs to further improve our diagnostic model. Results: To resolve confounding problems with our eMRI datasets due to artefacts, mislabelling, and misreporting, we used model checking, student auditing and expert radiology review. Our results illustrated effective multimodal analysis methods which improved the POD obliteration detection accuracy from eMRI datasets. This model improved the Area Under the Curve (AUC) from 65.0% to 90.6%. Conclusion: We have been able to improve the accuracy of diagnosing endometriosis from eMRIs using a novel POD obliteration detection method. Our method extracts knowledge from unpaired eTVUSs and applies it to eMRI datasets. The detection of POD obliteration is automated from eMRI data. Combining images for corresponding endometriosis signs using algorithms, is the first step in improving the imaging diagnosis of endometriosis, when compared to surgery, and allows extrapolation of results when either imaging modality is missing. This will enable women to obtain a faster diagnosis, where adequate scanning is available, prior to undertaking surgery.
Generalisation of deep neural networks becomes vulnerable when distribution shifts are encountered between train (source) and test (target) domain data. Few-shot domain adaptation mitigates this issue by adapting deep neural networks pre-trained on the source domain to the target domain using a randomly selected and annotated support set from the target domain. This paper argues that randomly selecting the support set can be further improved for effectively adapting the pre-trained source models to the target domain. Alternatively, we propose SelectNAdapt, an algorithm to curate the selection of the target domain samples, which are then annotated and included in the support set. In particular, for the K-shot adaptation problem, we first leverage self-supervision to learn features of the target domain data. Then, we propose a per-class clustering scheme of the learned target domain features and select K representative target samples using a distance-based scoring function. Finally, we bring our selection setup towards a practical ground by relying on pseudo-labels for clustering semantically similar target domain samples. Our experiments show promising results on three few-shot domain adaptation benchmarks for image recognition compared to related approaches and the standard random selection.
Endometriosis is a debilitating condition affecting 5% to 10% of the women worldwide, where early detection and treatment are the best tools to manage the condition. Early detection can be done via surgery, but multi-modal medical imaging is preferable given the simpler and faster process. However, imaging-based endometriosis diagnosis is challenging as 1) there are few capable clinicians; and 2) it is characterised by small lesions unconfined to a specific location. These two issues challenge the development of endometriosis classifiers as the training datasets tend to be small and contain difficult samples, which leads to overfitting. Hence, it is important to consider generalisation techniques to mitigate this problem, particularly self-supervised pre-training methods that have shown outstanding results in computer vision and natural language processing applications. The main goal of this paper is to study the effectiveness of modern self-supervised pre-training techniques to overcome the two issues mentioned above for the classification of endometriosis from multi-modal imaging data. We also introduce a new masking image modelling self-supervised pre-training method that works with 3D multi-modal medical imaging. Furthermore, to the best of our knowledge, this paper presents the first endometriosis classifier, fine-tuned from the pre-trained model above, which works with multi-modal (i.e., T1 and T2) magnetic resonance imaging (MRI) data. Our results show that self-supervised pre-training improves endometriosis classification by as much as 31%, when compared with classifiers trained from scratch.
We introduce a new and rigorously-formulated PAC-Bayes meta-learning algorithm that solves few-shot learning. Our proposed method extends the PAC-Bayes framework from a single-task setting to the meta-learning multiple-task setting to upper-bound the error evaluated on any, even unseen, tasks and samples. We also propose a generative-based approach to estimate the posterior of task-specific model parameters more expressively compared to the usual assumption based on a multivariate normal distribution with a diagonal covariance matrix. We show that the models trained with our proposed meta-learning algorithm are well-calibrated and accurate, with state-of-the-art calibration errors while still being competitive on classification results on few-shot classification (mini-ImageNet and tiered-ImageNet) and regression (multi-modal task-distribution regression) benchmarks.
A new approach to optical fiber sensing is proposed and demonstrated that allows for specific measurement even in the presence of strong noise from undesired environmental perturbations. A deep neural network model is trained to statistically learn the relation of the complex optical interference output from a multimode optical fiber (MMF) with respect to a measurand of interest while discriminating the noise. This technique negates the need to carefully shield against, or compensate for, undesired perturbations, as is often the case for traditional optical fiber sensors. This is achieved entirely in software without any fiber postprocessing fabrication steps or specific packaging required, such as fiber Bragg gratings or specialized coatings. The technique is highly generalizable, whereby the model can be trained to identify any measurand of interest within any noisy environment provided the measurand affects the optical path length of the MMF's guided modes. We demonstrate the approach using a sapphire crystal optical fiber for temperature sensing under strong noise induced by mechanical vibrations, showing the power of the technique not only to extract sensing information buried in strong noise but to also enable sensing using traditionally challenging exotic materials. (C) 2021 Chinese Laser Press
The deployment of automated deep-learning classifiers in clinical practice has the potential to streamline the diagnosis process and improve the diagnosis accuracy, but the acceptance of those classifiers relies on both their accuracy and interpretability. In general, accurate deep-learning classifiers provide little model interpretability, while interpretable models do not have competitive classification accuracy. In this paper, we introduce a new deep-learning diagnosis framework, called InterNRL, that is designed to be highly accurate and interpretable. InterNRL consists of a student-teacher framework, where the student model is an interpretable prototype-based classifier (ProtoPNet) and the teacher is an accurate global image classifier (GlobalNet). The two classifiers are mutually optimised with a novel reciprocal learning paradigm in which the student ProtoPNet learns from optimal pseudo labels produced by the teacher GlobalNet, while GlobalNet learns from ProtoPNet's classification performance and pseudo labels. This reciprocal learning paradigm enables InterNRL to be flexibly optimised under both fully- and semi-supervised learning scenarios, reaching state-of-the-art classification performance in both scenarios for the tasks of breast cancer and retinal disease diagnosis. Moreover, relying on weakly-labelled training images, InterNRL also achieves superior breast cancer localisation and brain tumour segmentation results than other competing methods.
Noisy labels are unavoidable yet troublesome in the ecosystem of deep learning because models can easily overfit them. There are many types of label noise, such as symmetric, asymmetric and instance-dependent noise (IDN), with IDN being the only type that depends on image information. Such dependence on image information makes IDN a critical type of label noise to study, given that labelling mistakes are caused in large part by insufficient or ambiguous information about the visual classes present in images. Aiming to provide an effective technique to address IDN, we present a new graphical modelling approach called InstanceGM, that combines discriminative and generative models. The main contributions of InstanceGM are: i) the use of the continuous Bernoulli distribution to train the generative model, offering significant training advantages, and ii) the exploration of a state-of-the-art noisy-label discriminative classifier to generate clean labels from instance-dependent noisy-label samples. InstanceGM is competitive with current noisy-label learning approaches, particularly in IDN benchmarks using synthetic and real-world datasets, where our method shows better accuracy than the competitors in most experiments 1 .
Prototypical part network (ProtoPNet) methods have been designed to achieve interpretable classification by associating predictions with a set of training prototypes, which we refer to as trivial prototypes because they are trained to lie far from the classification boundary in the feature space. Note that it is possible to make an analogy between ProtoPNet and support vector machine (SVM) given that the classification from both methods relies on computing similarity with a set of training points (i.e., trivial prototypes in ProtoPNet, and support vectors in SVM). However, while trivial prototypes are located far from the classification boundary, support vectors are located close to this boundary, and we argue that this discrepancy with the well-established SVM theory can result in ProtoPNet models with inferior classification accuracy. In this paper, we aim to improve the classification of ProtoPNet with a new method to learn support prototypes that lie near the classification boundary in the feature space, as suggested by the SVM theory. In addition, we target the improvement of classification results with a new model, named ST-ProtoPNet, which exploits our support prototypes and the trivial prototypes to provide more effective classification. Experimental results on CUB-200-2011, Stanford Cars, and Stan-ford Dogs datasets demonstrate that ST-ProtoPNet achieves state-of-the-art classification accuracy and interpretability results. We also show that the proposed support prototypes tend to be better localised in the object of interest rather than in the background region.
Deep learning methods have shown outstanding classification accuracy in medical imaging problems, which is largely attributed to the availability of large-scale datasets manually annotated with clean labels. However, given the high cost of such manual annotation, new medical imaging classification problems may need to rely on machine-generated noisy labels extracted from radiology reports. Indeed, many Chest X-Ray (CXR) classifiers have been modelled from datasets with noisy labels, but their training procedure is in general not robust to noisy-label samples, leading to sub-optimal models. Furthermore, CXR datasets are mostly multi-label, so current multi-class noisy-label learning methods cannot be easily adapted. In this paper, we propose a new method designed for noisy multi-label CXR learning, which detects and smoothly re-labels noisy samples from the dataset to be used in the training of common multi-label classifiers. The proposed method optimises a bag of multi-label descriptors (BoMD) to promote their similarity with the semantic descriptors produced by language models from multi-label image annotations. Our experiments on noisy multi-label training sets and clean testing sets show that our model has state-of-the-art accuracy and robustness in many CXR multi-label classification benchmarks, including a new benchmark that we propose to systematically assess noisy multi-label methods.
The missing modality issue is critical but non-trivial to be solved by multi-modal models. Current methods aiming to handle the missing modality problem in multi-modal tasks, either deal with missing modalities only during evaluation or train separate models to handle specific missing modality settings. In addition, these models are designed for specific tasks, so for example, classification models are not easily adapted to segmentation tasks and vice versa. In this paper, we propose the Shared-Specific Feature Modelling (ShaSpec) method that is considerably simpler and more effective than competing approaches that address the issues above. ShaSpec is designed to take advantage of all available input modalities during training and evaluation by learning shared and specific features to better represent the input data. This is achieved from a strategy that relies on auxiliary tasks based on distribution alignment and domain classification, in addition to a residual feature fusion procedure. Also, the design simplicity of ShaSpec enables its easy adaptation to multiple tasks, such as classification and segmentation. Experiments are conducted on both medical image segmentation and computer vision classification, with results indicating that ShaSpec outperforms competing methods by a large margin. For instance, on BraTS2018, ShaSpec improves the SOTA by more than 3% for enhancing tumour, 5% for tumour core and 3% for whole tumour.
Semantic segmentation models classify pixels into a set of known ("in-distribution") visual classes. When deployed in an open world, the reliability of these models depends on their ability to not only classify in-distribution pixels but also to detect out-of-distribution (OoD) pixels. Historically, the poor OoD detection performance of these models has motivated the design of methods based on model re-training using synthetic training images that include OoD visual objects. Although successful, these re-trained methods have two issues: 1) their in-distribution segmentation accuracy may drop during re-training, and 2) their OoD detection accuracy does not generalise well to new contexts outside the training set (e.g., from city to country context). In this paper, we mitigate these issues with: (i) a new residual pattern learning (RPL) module that assists the segmentation model to detect OoD pixels with minimal deterioration to inlier segmentation accuracy; and (ii) a novel context-robust contrastive learning (CoroCL) that enforces RPL to robustly detect OoD pixels in various contexts. Our approach improves by around 10% FPR and 7% AuPRC previous state-of-the-art in Fishyscapes, Segment-Me-If-You-Can, and RoadAnomaly datasets.
Generalized zero shot learning (GZSL) is defined by a training process containing a set of visual samples from seen classes and a set of semantic samples from seen and unseen classes, while the testing process consists of the classification of visual samples from seen and unseen classes. Current approaches are based on testing processes that focus on only one of the modalities (visual or semantic), even when the training uses both modalities (mostly for regularizing the training process). This under-utilization of modalities, particularly during testing, can hinder the classification accuracy of the method. In addition, we note a scarce attention to the development of learning methods that explicitly optimize a balanced performance of seen and unseen classes. Such issue is one of the reasons behind the vastly superior classification accuracy of seen classes in GZSL methods. In this paper, we mitigate these issues by proposing a new GZSL method based on multi-modal training and testing processes, where the optimization explicitly promotes a balanced classification accuracy between seen and unseen classes. Furthermore, we explore Bayesian inference for the visual and semantic classifiers, which is another novelty of our work in the GZSL framework. Experiments show that our method holds the state of the art (SOTA) results in terms of harmonic mean (H-mean) classification between seen and unseen classes and area under the seen and unseen curve (AUSUC) on several public GZSL benchmarks.
Deep learning architectures have achieved promising results in different areas (e.g., medicine, agriculture, and security). However, using those powerful techniques in many real applications becomes challenging due to the large labeled collections required during training. Several works have pursued solutions to overcome it by proposing strategies that can learn more for less, e.g., weakly and semi-supervised learning approaches. As these approaches do not usually address memorization and sensitivity to adversarial examples, this paper presents three deep metric learning approaches combined with Mixup for incomplete-supervision scenarios. We show that some state-of-the-art approaches in metric learning might not work well in such scenarios. Moreover, the proposed approaches outperform most of them in different datasets.
Uncertainty in Artificial Intelligence, 27-30 July 2021 We propose probabilistic task modelling -- a generative probabilistic model for collections of tasks used in meta-learning. The proposed model combines variational auto-encoding and latent Dirichlet allocation to model each task as a mixture of Gaussian distribution in an embedding space. Such modelling provides an explicit representation of a task through its task-theme mixture. We present an efficient approximation inference technique based on variational inference method for empirical Bayes parameter estimation. We perform empirical evaluations to validate the task uncertainty and task distance produced by the proposed method through correlation diagrams of the prediction accuracy on testing tasks. We also carry out experiments of task selection in meta-learning to demonstrate how the task relatedness inferred from the proposed model help to facilitate meta-learning algorithms.
In this paper, we introduce a novel methodology for characterising the performance of deep learning networks (ResNets and DenseNet) with respect to training convergence and generalisation as a function of mini-batch size and learning rate for image classification. This methodology is based on novel measurements derived from the eigenvalues of the approximate Fisher information matrix, which can be efficiently computed even for high capacity deep models. Our proposed measurements can help practitioners to monitor and control the training process (by actively tuning the mini-batch size and learning rate) to allow for good training convergence and generalisation. Furthermore, the proposed measurements also allow us to show that it is possible to optimise the training process with a new dynamic sampling training approach that continuously and automatically change the mini-batch size and learning rate during the training process. Finally, we show that the proposed dynamic sampling training approach has a faster training time and a competitive classification accuracy compared to the current state of the art.
Generalised zero-shot learning (GZSL) is a classification problem where the learning stage relies on a set of seen visual classes and the inference stage aims to identify both the seen visual classes and a new set of unseen visual classes. Critically, both the learning and inference stages can leverage a semantic representation that is available for the seen and unseen classes. Most state-of-the-art GZSL approaches rely on a mapping between latent visual and semantic spaces without considering if a particular sample belongs to the set of seen or unseen classes. In this paper, we propose a novel GZSL method that learns a joint latent representation that combines both visual and semantic information. This mitigates the need for learning a mapping between the two spaces. Our method also introduces a domain classification that estimates whether a sample belongs to a seen or an unseen class. Our classifier then combines a class discriminator with this domain classifier with the goal of reducing the natural bias that GZSL approaches have toward the seen classes. Experiments show that our method achieves state-of-the-art results in terms of harmonic mean, the area under the seen and unseen curve and unseen classification accuracy on public GZSL benchmark data sets. Our code will be available upon acceptance of this paper.
Cardiac magnetic resonance (CMR) is used extensively in the diagnosis and management of cardiovascular disease. Deep learning methods have proven to deliver segmentation results comparable to human experts in CMR imaging, but there have been no convincing results for the problem of end-to-end segmentation and diagnosis from CMR. This is in part due to a lack of sufficiently large datasets required to train robust diagnosis models. In this paper, we propose a learning method to train diagnosis models, where our approach is designed to work with relatively small datasets. In particular, the optimisation loss is based on multi-task learning that jointly trains for the tasks of segmentation and diagnosis classification. We hypothesize that segmentation has a regularizing effect on the learning of features relevant for diagnosis. Using the 100 training and 50 testing samples available from the Automated Cardiac Diagnosis Challenge (ACDC) dataset, which has a balanced distribution of 5 cardiac diagnoses, we observe a reduction of the classification error from 32% to 22%, and a faster convergence compared to a baseline without segmentation. To the best of our knowledge, this is the best diagnosis results from CMR using an end-to-end diagnosis and segmentation learning method.
We propose a new method for breast cancer screening from DCE-MRI based on a post-hoc approach that is trained using weakly annotated data (i.e., labels are available only at the image level without any lesion delineation). Our proposed post-hoc method automatically diagnosis the whole volume and, for positive cases, it localizes the malignant lesions that led to such diagnosis. Conversely, traditional approaches follow a pre-hoc approach that initially localises suspicious areas that are subsequently classified to establish the breast malignancy -- this approach is trained using strongly annotated data (i.e., it needs a delineation and classification of all lesions in an image). Another goal of this paper is to establish the advantages and disadvantages of both approaches when applied to breast screening from DCE-MRI. Relying on experiments on a breast DCE-MRI dataset that contains scans of 117 patients, our results show that the post-hoc method is more accurate for diagnosing the whole volume per patient, achieving an AUC of 0.91, while the pre-hoc method achieves an AUC of 0.81. However, the performance for localising the malignant lesions remains challenging for the post-hoc method due to the weakly labelled dataset employed during training.
We propose a method that substantially improves the efficiency of deep distance metric learning based on the optimization of the triplet loss function. One epoch of such training process based on a naive optimization of the triplet loss function has a run-time complexity O(N^3), where N is the number of training samples. Such optimization scales poorly, and the most common approach proposed to address this high complexity issue is based on sub-sampling the set of triplets needed for the training process. Another approach explored in the field relies on an ad-hoc linearization (in terms of N) of the triplet loss that introduces class centroids, which must be optimized using the whole training set for each mini-batch - this means that a naive implementation of this approach has run-time complexity O(N^2). This complexity issue is usually mitigated with poor, but computationally cheap, approximate centroid optimization methods. In this paper, we first propose a solid theory on the linearization of the triplet loss with the use of class centroids, where the main conclusion is that our new linear loss represents a tight upper-bound to the triplet loss. Furthermore, based on the theory above, we propose a training algorithm that no longer requires the centroid optimization step, which means that our approach is the first in the field with a guaranteed linear run-time complexity. We show that the training of deep distance metric learning methods using the proposed upper-bound is substantially faster than triplet-based methods, while producing competitive retrieval accuracy results on benchmark datasets (CUB-200-2011 and CAR196).
Current approaches to explaining the decisions of deep learning systems for medical tasks have focused on visualising the elements that have contributed to each decision. We argue that such approaches are not enough to "open the black box" of medical decision making systems because they are missing a key component that has been used as a standard communication tool between doctors for centuries: language. We propose a model-agnostic interpretability method that involves training a simple recurrent neural network model to produce descriptive sentences to clarify the decision of deep learning classifiers. We test our method on the task of detecting hip fractures from frontal pelvic x-rays. This process requires minimal additional labelling despite producing text containing elements that the original deep learning classification model was not specifically trained to detect. The experimental results show that: 1) the sentences produced by our method consistently contain the desired information, 2) the generated sentences are preferred by doctors compared to current tools that create saliency maps, and 3) the combination of visualisations and generated text is better than either alone.
The training of deep learning models generally requires a large amount of annotated data for effective convergence and generalisation. However, obtaining high-quality annotations is a laboursome and expensive process due to the need of expert radiologists for the labelling task. The study of semi-supervised learning in medical image analysis is then of crucial importance given that it is much less expensive to obtain unlabelled images than to acquire images labelled by expert radiologists. Essentially, semi-supervised methods leverage large sets of unlabelled data to enable better training convergence and generalisation than using only the small set of labelled images. In this paper, we propose Self-supervised Mean Teacher for Semi-supervised (S$^2$MTS$^2$) learning that combines self-supervised mean-teacher pre-training with semi-supervised fine-tuning. The main innovation of S$^2$MTS$^2$ is the self-supervised mean-teacher pre-training based on the joint contrastive learning, which uses an infinite number of pairs of positive query and key features to improve the mean-teacher representation. The model is then fine-tuned using the exponential moving average teacher framework trained with semi-supervised learning. We validate S$^2$MTS$^2$ on the multi-label classification problems from Chest X-ray14 and CheXpert, and the multi-class classification from ISIC2018, where we show that it outperforms the previous SOTA semi-supervised learning methods by a large margin.
The automatic detection of frames containing polyps from a colonoscopy video sequence is an important first step for a fully automated colonoscopy analysis tool. Typically, such detection system is built using a large annotated data set of frames with and without polyps, which is expensive to be obtained. In this paper, we introduce a new system that detects frames containing polyps as anomalies from a distribution of frames from exams that do not contain any polyps. The system is trained using a one-class training set consisting of colonoscopy frames without polyps -- such training set is considerably less expensive to obtain, compared to the 2-class data set mentioned above. During inference, the system is only able to reconstruct frames without polyps, and when it tries to reconstruct a frame with polyp, it automatically removes (i.e., photoshop) it from the frame -- the difference between the input and reconstructed frames is used to detect frames with polyps. We name our proposed model as anomaly detection generative adversarial network (ADGAN), comprising a dual GAN with two generators and two discriminators. We show that our proposed approach achieves the state-of-the-art result on this data set, compared with recently proposed anomaly detection systems.
2020 33rd SIBGRAPI Conference on Graphics, Patterns and Images (SIBGRAPI) Noisy Labels are commonly present in data sets automatically collected from the internet, mislabeled by non-specialist annotators, or even specialists in a challenging task, such as in the medical field. Although deep learning models have shown significant improvements in different domains, an open issue is their ability to memorize noisy labels during training, reducing their generalization potential. As deep learning models depend on correctly labeled data sets and label correctness is difficult to guarantee, it is crucial to consider the presence of noisy labels for deep learning training. Several approaches have been proposed in the literature to improve the training of deep learning models in the presence of noisy labels. This paper presents a survey on the main techniques in literature, in which we classify the algorithm in the following groups: robust losses, sample weighting, sample selection, meta-learning, and combined approaches. We also present the commonly used experimental setup, data sets, and results of the state-of-the-art models.
Deep learning models have demonstrated outstanding performance in several problems, but their training process tends to require immense amounts of computational and human resources for training and labeling, constraining the types of problems that can be tackled. Therefore, the design of effective training methods that require small labeled training sets is an important research direction that will allow a more effective use of resources.Among current approaches designed to address this issue, two are particularly interesting: data augmentation and active learning. Data augmentation achieves this goal by artificially generating new training points, while active learning relies on the selection of the "most informative" subset of unlabeled training samples to be labelled by an oracle. Although successful in practice, data augmentation can waste computational resources because it indiscriminately generates samples that are not guaranteed to be informative, and active learning selects a small subset of informative samples (from a large un-annotated set) that may be insufficient for the training process. In this paper, we propose a Bayesian generative active deep learning approach that combines active learning with data augmentation -- we provide theoretical and empirical evidence (MNIST, CIFAR-$\{10,100\}$, and SVHN) that our approach has more efficient training and better classification results than data augmentation and active learning.
Anomaly detection with weakly supervised video-level labels is typically formulated as a multiple instance learning (MIL) problem, in which we aim to identify snippets containing abnormal events, with each video represented as a bag of video snippets. Although current methods show effective detection performance, their recognition of the positive instances, i.e., rare abnormal snippets in the abnormal videos, is largely biased by the dominant negative instances, especially when the abnormal events are subtle anomalies that exhibit only small differences compared with normal events. This issue is exacerbated in many methods that ignore important video temporal dependencies. To address this issue, we introduce a novel and theoretically sound method, named Robust Temporal Feature Magnitude learning (RTFM), which trains a feature magnitude learning function to effectively recognise the positive instances, substantially improving the robustness of the MIL approach to the negative instances from abnormal videos. RTFM also adapts dilated convolutions and self-attention mechanisms to capture long- and short-range temporal dependencies to learn the feature magnitude more faithfully. Extensive experiments show that the RTFM-enabled MIL model (i) outperforms several state-of-the-art methods by a large margin on four benchmark data sets (ShanghaiTech, UCF-Crime, XD-Violence and UCSD-Peds) and (ii) achieves significantly improved subtle anomaly discriminability and sample efficiency. Code is available at https://github.com/tianyu0207/RTFM.
Unsupervised anomaly detection (UAD) methods are trained with normal (or healthy) images only, but during testing, they are able to classify normal and abnormal (or disease) images. UAD is an important medical image analysis (MIA) method to be applied in disease screening problems because the training sets available for those problems usually contain only normal images. However, the exclusive reliance on normal images may result in the learning of ineffective low-dimensional image representations that are not sensitive enough to detect and segment unseen abnormal lesions of varying size, appearance, and shape. Pre-training UAD methods with self-supervised learning, based on computer vision techniques, can mitigate this challenge, but they are sub-optimal because they do not explore domain knowledge for designing the pretext tasks, and their contrastive learning losses do not try to cluster the normal training images, which may result in a sparse distribution of normal images that is ineffective for anomaly detection. In this paper, we propose a new self-supervised pre-training method for MIA UAD applications, named Pseudo Multi-class Strong Augmentation via Contrastive Learning (PMSACL). PMSACL consists of a novel optimisation method that contrasts a normal image class from multiple pseudo classes of synthesised abnormal images, with each class enforced to form a dense cluster in the feature space. In the experiments, we show that our PMSACL pre-training improves the accuracy of SOTA UAD methods on many MIA benchmarks using colonoscopy, fundus screening and Covid-19 Chest X-ray datasets. The code is made publicly available via https://github.com/tianyu0207/PMSACL.
Overall survival (OS) time prediction is one of the most common estimates of the prognosis of gliomas and is used to design an appropriate treatment planning. State-of-the-art (SOTA) methods for OS time prediction follow a pre-hoc approach that require computing the segmentation map of the glioma tumor sub-regions (necrotic, edema tumor, enhancing tumor) for estimating OS time. However, the training of the segmentation methods require ground truth segmentation labels which are tedious and expensive to obtain. Given that most of the large-scale data sets available from hospitals are unlikely to contain such precise segmentation, those SOTA methods have limited applicability. In this paper, we introduce a new post-hoc method for OS time prediction that does not require segmentation map annotation for training. Our model uses medical image and patient demographics (represented by age) as inputs to estimate the OS time and to estimate a saliency map that localizes the tumor as a way to explain the OS time prediction in a post-hoc manner. It is worth emphasizing that although our model can localize tumors, it uses only the ground truth OS time as training signal, i.e., no segmentation labels are needed. We evaluate our post-hoc method on the Multimodal Brain Tumor Segmentation Challenge (BraTS) 2019 data set and show that it achieves competitive results compared to pre-hoc methods with the advantage of not requiring segmentation labels for training.
In multi-modal learning, some modalities are more influential than others, and their absence can have a significant impact on classification/segmentation accuracy. Hence, an important research question is if it is possible for trained multi-modal models to have high accuracy even when influential modalities are absent from the input data. In this paper, we propose a novel approach called Meta-learned Cross-modal Knowledge Distillation (MCKD) to address this research question. MCKD adaptively estimates the importance weight of each modality through a meta-learning process. These dynamically learned modality importance weights are used in a pairwise cross-modal knowledge distillation process to transfer the knowledge from the modalities with higher importance weight to the modalities with lower importance weight. This cross-modal knowledge distillation produces a highly accurate model even with the absence of influential modalities. Differently from previous methods in the field, our approach is designed to work in multiple tasks (e.g., segmentation and classification) with minimal adaptation. Experimental results on the Brain tumor Segmentation Dataset 2018 (BraTS2018) and the Audiovision-MNIST classification dataset demonstrate the superiority of MCKD over current state-of-the-art models. Particularly in BraTS2018, we achieve substantial improvements of 3.51\% for enhancing tumor, 2.19\% for tumor core, and 1.14\% for the whole tumor in terms of average segmentation Dice score.
Unsupervised anomaly detection (UAD) learns one-class classifiers exclusively with normal (i.e., healthy) images to detect any abnormal (i.e., unhealthy) samples that do not conform to the expected normal patterns. UAD has two main advantages over its fully supervised counterpart. Firstly, it is able to directly leverage large datasets available from health screening programs that contain mostly normal image samples, avoiding the costly manual labelling of abnormal samples and the subsequent issues involved in training with extremely class-imbalanced data. Further, UAD approaches can potentially detect and localise any type of lesions that deviate from the normal patterns. One significant challenge faced by UAD methods is how to learn effective low-dimensional image representations to detect and localise subtle abnormalities, generally consisting of small lesions. To address this challenge, we propose a novel self-supervised representation learning method, called Constrained Contrastive Distribution learning for anomaly detection (CCD), which learns fine-grained feature representations by simultaneously predicting the distribution of augmented data and image contexts using contrastive learning with pretext constraints. The learned representations can be leveraged to train more anomaly-sensitive detection models. Extensive experiment results show that our method outperforms current state-of-the-art UAD approaches on three different colonoscopy and fundus screening datasets. Our code is available at https://github.com/tianyu0207/CCD.
Knowledge distillation is an attractive approach for learning compact deep neural networks, which learns a lightweight student model by distilling knowledge from a complex teacher model. Attention-based knowledge distillation is a specific form of intermediate feature-based knowledge distillation that uses attention mechanisms to encourage the student to better mimic the teacher. However, most of the previous attention-based distillation approaches perform attention in the spatial domain, which primarily affects local regions in the input image. This may not be sufficient when we need to capture the broader context or global information necessary for effective knowledge transfer. In frequency domain, since each frequency is determined from all pixels of the image in spatial domain, it can contain global information about the image. Inspired by the benefits of the frequency domain, we propose a novel module that functions as an attention mechanism in the frequency domain. The module consists of a learnable global filter that can adjust the frequencies of student's features under the guidance of the teacher's features, which encourages the student's features to have patterns similar to the teacher's features. We then propose an enhanced knowledge review-based distillation model by leveraging the proposed frequency attention module. The extensive experiments with various teacher and student architectures on image classification and object detection benchmark datasets show that the proposed approach outperforms other knowledge distillation methods.
The training of medical image analysis systems using machine learning approaches follows a common script: collect and annotate a large dataset, train the classifier on the training set, and test it on a hold-out test set. This process bears no direct resemblance with radiologist training, which is based on solving a series of tasks of increasing difficulty, where each task involves the use of significantly smaller datasets than those used in machine learning. In this paper, we propose a novel training approach inspired by how radiologists are trained. In particular, we explore the use of meta-training that models a classifier based on a series of tasks. Tasks are selected using teacher-student curriculum learning, where each task consists of simple classification problems containing small training sets. We hypothesize that our proposed meta-training approach can be used to pre-train medical image analysis models. This hypothesis is tested on the automatic breast screening classification from DCE-MRI trained with weakly labeled datasets. The classification performance achieved by our approach is shown to be the best in the field for that application, compared to state of art baseline approaches: DenseNet, multiple instance learning and multi-task learning.
Real-time and robust automatic detection of polyps from colonoscopy videos are essential tasks to help improve the performance of doctors during this exam. The current focus of the field is on the development of accurate but inefficient detectors that will not enable a real-time application. We advocate that the field should instead focus on the development of simple and efficient detectors that an be combined with effective trackers to allow the implementation of real-time polyp detectors. In this paper, we propose a Kalman filtering tracker that can work together with powerful, but efficient detectors, enabling the implementation of real-time polyp detectors. In particular, we show that the combination of our Kalman filtering with the detector PP-YOLO shows state-of-the-art (SOTA) detection accuracy and real-time processing. More specifically, our approach has SOTA results on the CVC-ClinicDB dataset, with a recall of 0.740, precision of 0.869, $F_1$ score of 0.799, an average precision (AP) of 0.837, and can run in real time (i.e., 30 frames per second). We also evaluate our method on a subset of the Hyper-Kvasir annotated by our clinical collaborators, resulting in SOTA results, with a recall of 0.956, precision of 0.875, $F_1$ score of 0.914, AP of 0.952, and can run in real time.
MICAAI 2020 Intra-operative automatic semantic segmentation of knee joint structures can assist surgeons during knee arthroscopy in terms of situational awareness. However, due to poor imaging conditions (e.g., low texture, overexposure, etc.), automatic semantic segmentation is a challenging scenario, which justifies the scarce literature on this topic. In this paper, we propose a novel self-supervised monocular depth estimation to regularise the training of the semantic segmentation in knee arthroscopy. To further regularise the depth estimation, we propose the use of clean training images captured by the stereo arthroscope of routine objects (presenting none of the poor imaging conditions and with rich texture information) to pre-train the model. We fine-tune such model to produce both the semantic segmentation and self-supervised monocular depth using stereo arthroscopic images taken from inside the knee. Using a data set containing 3868 arthroscopic images captured during cadaveric knee arthroscopy with semantic segmentation annotations, 2000 stereo image pairs of cadaveric knee arthroscopy, and 2150 stereo image pairs of routine objects, we show that our semantic segmentation regularised by self-supervised depth estimation produces a more accurate segmentation than a state-of-the-art semantic segmentation approach modeled exclusively with semantic segmentation annotation.
We introduce Probabilistic Object Detection, the task of detecting objects in images and accurately quantifying the spatial and semantic uncertainties of the detections. Given the lack of methods capable of assessing such probabilistic object detections, we present the new Probability-based Detection Quality measure (PDQ).Unlike AP-based measures, PDQ has no arbitrary thresholds and rewards spatial and label quality, and foreground/background separation quality while explicitly penalising false positive and false negative detections. We contrast PDQ with existing mAP and moLRP measures by evaluating state-of-the-art detectors and a Bayesian object detector based on Monte Carlo Dropout. Our experiments indicate that conventional object detectors tend to be spatially overconfident and thus perform poorly on the task of probabilistic object detection. Our paper aims to encourage the development of new object detection approaches that provide detections with accurately estimated spatial and label uncertainties and are of critical importance for deployment on robots and embodied AI systems in the real world.
Methods to detect malignant lesions from screening mammograms are usually trained with fully annotated datasets, where images are labelled with the localisation and classification of cancerous lesions. However, real-world screening mammogram datasets commonly have a subset that is fully annotated and another subset that is weakly annotated with just the global classification (i.e., without lesion localisation). Given the large size of such datasets, researchers usually face a dilemma with the weakly annotated subset: to not use it or to fully annotate it. The first option will reduce detection accuracy because it does not use the whole dataset, and the second option is too expensive given that the annotation needs to be done by expert radiologists. In this paper, we propose a middle-ground solution for the dilemma, which is to formulate the training as a weakly- and semi-supervised learning problem that we refer to as malignant breast lesion detection with incomplete annotations. To address this problem, our new method comprises two stages, namely: (1) pre-training a multi-view mammogram classifier with weak supervision from the whole dataset, and (2) extending the trained classifier to become a multi-view detector that is trained with semi-supervised student–teacher learning, where the training set contains fully and weakly-annotated mammograms. We provide extensive detection results on two real-world screening mammogram datasets containing incomplete annotations and show that our proposed approach achieves state-of-the-art results in the detection of malignant breast lesions with incomplete annotations. •We explore a new experimental setting and method for the detection of malignant breast lesions using large-scale real-world screening mammogram datasets that have both weak and fully annotations.•We proposed a new two-stage training method to jointly process GRAD-CAM and pseudo-label predictions in a student–teacher and cross-view manner.•We also propose innovations to the student–teacher framework to avoid the misalignment between the student and teacher’s parameter space.•We provide extensive experiments on two real-world breast cancer screening mammogram datasets containing incomplete annotations.
Data augmentation is an essential part of the training process applied to deep learning models. The motivation is that a robust training process for deep learning models depends on large annotated datasets, which are expensive to be acquired, stored and processed. Therefore a reasonable alternative is to be able to automatically generate new annotated training samples using a process known as data augmentation. The dominant data augmentation approach in the field assumes that new training samples can be obtained via random geometric or appearance transformations applied to annotated training samples, but this is a strong assumption because it is unclear if this is a reliable generative model for producing new training samples. In this paper, we provide a novel Bayesian formulation to data augmentation, where new annotated training points are treated as missing variables and generated based on the distribution learned from the training set. For learning, we introduce a theoretically sound algorithm --- generalised Monte Carlo expectation maximisation, and demonstrate one possible implementation via an extension of the Generative Adversarial Network (GAN). Classification results on MNIST, CIFAR-10 and CIFAR-100 show the better performance of our proposed method compared to the current dominant data augmentation approach mentioned above --- the results also show that our approach produces better classification results than similar GAN models.
In monocular depth estimation, unsupervised domain adaptation has recently been explored to relax the dependence on large annotated image-based depth datasets. However, this comes at the cost of training multiple models or requiring complex training protocols. We formulate unsupervised domain adaptation for monocular depth estimation as a consistency-based semi-supervised learning problem by assuming access only to the source domain ground truth labels. To this end, we introduce a pairwise loss function that regularises predictions on the source domain while enforcing perturbation consistency across multiple augmented views of the unlabelled target samples. Importantly, our approach is simple and effective, requiring only training of a single model in contrast to the prior work. In our experiments, we rely on the standard depth estimation benchmarks KITTI and NYUv2 to demonstrate state-of-the-art results compared to related approaches. Furthermore, we analyse the simplicity and effectiveness of our approach in a series of ablation studies. The code is available at \url{https://github.com/AmirMaEl/SemiSupMDE}.
Unsupervised Deep Distance Metric Learning (UDML) aims to learn sample similarities in the embedding space from an unlabeled dataset. Traditional UDML methods usually use the triplet loss or pairwise loss which requires the mining of positive and negative samples w.r.t. anchor data points. This is, however, challenging in an unsupervised setting as the label information is not available. In this paper, we propose a new UDML method that overcomes that challenge. In particular, we propose to use a deep clustering loss to learn centroids, i.e., pseudo labels, that represent semantic classes. During learning, these centroids are also used to reconstruct the input samples. It hence ensures the representativeness of centroids - each centroid represents visually similar samples. Therefore, the centroids give information about positive (visually similar) and negative (visually dissimilar) samples. Based on pseudo labels, we propose a novel unsupervised metric loss which enforces the positive concentration and negative separation of samples in the embedding space. Experimental results on benchmarking datasets show that the proposed approach outperforms other UDML methods.
We study the problem of few-shot learning-based denoising where the training set contains just a handful of clean and noisy samples. A solution to mitigate the small training set issue is to pre-train a denoising model with small training sets containing pairs of clean and synthesized noisy signals, produced from empirical noise priors, and fine-tune on the available small training set. While such transfer learning seems effective, it may not generalize well because of the limited amount of training data. In this work, we propose a new meta-learning training approach for few-shot learning-based denoising problems. Our model is meta-trained using known synthetic noise models, and then fine-tuned with the small training set, with the real noise, as a few-shot learning task. Meta-learning from small training sets of synthetically generated data during meta-training enables us to not only generate an infinite number of training tasks, but also train a model to learn with small training sets -- both advantages have the potential to improve the generalisation of the denoising model. Our approach is empirically shown to produce more accurate denoising results than supervised learning and transfer learning in three denoising evaluations for images and 1-D signals. Interestingly, our study provides strong indications that meta-learning has the potential to become the main learning algorithm for denoising.
State-of-the-art (SOTA) anomaly segmentation approaches on complex urban driving scenes explore pixel-wise classification uncertainty learned from outlier exposure, or external reconstruction models. However, previous uncertainty approaches that directly associate high uncertainty to anomaly may sometimes lead to incorrect anomaly predictions, and external reconstruction models tend to be too inefficient for real-time self-driving embedded systems. In this paper, we propose a new anomaly segmentation method, named pixel-wise energy-biased abstention learning (PEBAL), that explores pixel-wise abstention learning (AL) with a model that learns an adaptive pixel-level anomaly class, and an energy-based model (EBM) that learns inlier pixel distribution. More specifically, PEBAL is based on a non-trivial joint training of EBM and AL, where EBM is trained to output high-energy for anomaly pixels (from outlier exposure) and AL is trained such that these high-energy pixels receive adaptive low penalty for being included to the anomaly class. We extensively evaluate PEBAL against the SOTA and show that it achieves the best performance across four benchmarks. Code is available at https://github.com/tianyu0207/PEBAL.
Effective semi-supervised learning (SSL) in medical image analysis (MIA) must address two challenges: 1) work effectively on both multi-class (e.g., lesion classification) and multi-label (e.g., multiple-disease diagnosis) problems, and 2) handle imbalanced learning (because of the high variance in disease prevalence). One strategy to explore in SSL MIA is based on the pseudo labelling strategy, but it has a few shortcomings. Pseudo-labelling has in general lower accuracy than consistency learning, it is not specifically designed for both multi-class and multi-label problems, and it can be challenged by imbalanced learning. In this paper, unlike traditional methods that select confident pseudo label by threshold, we propose a new SSL algorithm, called anti-curriculum pseudo-labelling (ACPL), which introduces novel techniques to select informative unlabelled samples, improving training balance and allowing the model to work for both multi-label and multi-class problems, and to estimate pseudo labels by an accurate ensemble of classifiers (improving pseudo label accuracy). We run extensive experiments to evaluate ACPL on two public medical image classification benchmarks: Chest X-Ray14 for thorax disease multi-label classification and ISIC2018 for skin lesion multi-class classification. Our method outperforms previous SOTA SSL methods on both datasets
Generalised zero-shot learning (GZSL) methods aim to classify previously seen and unseen visual classes by leveraging the semantic information of those classes. In the context of GZSL, semantic information is non-visual data such as a text description of both seen and unseen classes. Previous GZSL methods have utilised transformations between visual and semantic embedding spaces, as well as the learning of joint spaces that include both visual and semantic information. In either case, classification is then performed on a single learned space. We argue that each embedding space contains complementary information for the GZSL problem. By using just a visual, semantic or joint space some of this information will invariably be lost. In this paper, we demonstrate the advantages of our new GZSL method that combines the classification of visual, semantic and joint spaces. Most importantly, this ensembling allows for more information from the source domains to be seen during classification. An additional contribution of our work is the application of a calibration procedure for each classifier in the ensemble. This calibration mitigates the problem of model selection when combining the classifiers. Lastly, our proposed method achieves state-of-the-art results on the CUB, AWA1 and AWA2 benchmark data sets and provides competitive performance on the SUN data set.
In this paper, we propose and analyse a system that can automatically detect, localise and classify polyps from colonoscopy videos. The detection of frames with polyps is formulated as a few-shot anomaly classification problem, where the training set is highly imbalanced with the large majority of frames consisting of normal images and a small minority comprising frames with polyps. Colonoscopy videos may contain blurry images and frames displaying feces and water jet sprays to clean the colon -- such frames can mistakenly be detected as anomalies, so we have implemented a classifier to reject these two types of frames before polyp detection takes place. Next, given a frame containing a polyp, our method localises (with a bounding box around the polyp) and classifies it into five different classes. Furthermore, we study a method to improve the reliability and interpretability of the classification result using uncertainty estimation and classification calibration. Classification uncertainty and calibration not only help improve classification accuracy by rejecting low-confidence and high-uncertain results, but can be used by doctors to decide how to decide on the classification of a polyp. All the proposed detection, localisation and classification methods are tested using large data sets and compared with relevant baseline approaches.
The most competitive noisy label learning methods rely on an unsupervised classification of clean and noisy samples, where samples classified as noisy are re-labelled and "MixMatched" with the clean samples. These methods have two issues in large noise rate problems: 1) the noisy set is more likely to contain hard samples that are in-correctly re-labelled, and 2) the number of samples produced by MixMatch tends to be reduced because it is constrained by the small clean set size. In this paper, we introduce the learning algorithm PropMix to handle the issues above. PropMix filters out hard noisy samples, with the goal of increasing the likelihood of correctly re-labelling the easy noisy samples. Also, PropMix places clean and re-labelled easy noisy samples in a training set that is augmented with MixUp, removing the clean set size constraint and including a large proportion of correctly re-labelled easy noisy samples. We also include self-supervised pre-training to improve robustness to high noisy label scenarios. Our experiments show that PropMix has state-of-the-art (SOTA) results on CIFAR-10/-100(with symmetric, asymmetric and semantic label noise), Red Mini-ImageNet (from the Controlled Noisy Web Labels), Clothing1M and WebVision. In severe label noise bench-marks, our results are substantially better than other methods. The code is available athttps://github.com/filipe-research/PropMix.
We introduce a new and rigorously-formulated PAC-Bayes meta-learning algorithm that solves few-shot learning. Our proposed method extends the PAC-Bayes framework from a single task setting to the meta-learning multiple task setting to upper-bound the error evaluated on any, even unseen, tasks and samples. We also propose a generative-based approach to estimate the posterior of task-specific model parameters more expressively compared to the usual assumption based on a multivariate normal distribution with a diagonal covariance matrix. We show that the models trained with our proposed meta-learning algorithm are well calibrated and accurate, with state-of-the-art calibration and classification results on few-shot classification (mini-ImageNet and tiered-ImageNet) and regression (multi-modal task-distribution regression) benchmarks.
In generalized zero shot learning (GZSL), the set of classes are split into seen and unseen classes, where training relies on the semantic features of the seen and unseen classes and the visual representations of only the seen classes, while testing uses the visual representations of the seen and unseen classes. Current methods address GZSL by learning a transformation from the visual to the semantic space, exploring the assumption that the distribution of classes in the semantic and visual spaces is relatively similar. Such methods tend to transform unseen testing visual representations into one of the seen classes' semantic features instead of the semantic features of the correct unseen class, resulting in low accuracy GZSL classification. Recently, generative adversarial networks (GAN) have been explored to synthesize visual representations of the unseen classes from their semantic features - the synthesized representations of the seen and unseen classes are then used to train the GZSL classifier. This approach has been shown to boost GZSL classification accuracy, however, there is no guarantee that synthetic visual representations can generate back their semantic feature in a multi-modal cycle-consistent manner. This constraint can result in synthetic visual representations that do not represent well their semantic features. In this paper, we propose the use of such constraint based on a new regularization for the GAN training that forces the generated visual features to reconstruct their original semantic features. Once our model is trained with this multi-modal cycle-consistent semantic compatibility, we can then synthesize more representative visual representations for the seen and, more importantly, for the unseen classes. Our proposed approach shows the best GZSL classification results in the field in several publicly available datasets.
This paper addresses the semantic instance segmentation task in the open-set conditions, where input images can contain known and unknown object classes. The training process of existing semantic instance segmentation methods requires annotation masks for all object instances, which is expensive to acquire or even infeasible in some realistic scenarios, where the number of categories may increase boundlessly. In this paper, we present a novel open-set semantic instance segmentation approach capable of segmenting all known and unknown object classes in images, based on the output of an object detector trained on known object classes. We formulate the problem using a Bayesian framework, where the posterior distribution is approximated with a simulated annealing optimization equipped with an efficient image partition sampler. We show empirically that our method is competitive with state-of-the-art supervised methods on known classes, but also performs well on unknown classes when compared with unsupervised methods.
Monocular depth estimation has become one of the most studied applications in computer vision, where the most accurate approaches are based on fully supervised learning models. However, the acquisition of accurate and large ground truth data sets to model these fully supervised methods is a major challenge for the further development of the area. Self-supervised methods trained with monocular videos constitute one the most promising approaches to mitigate the challenge mentioned above due to the wide-spread availability of training data. Consequently, they have been intensively studied, where the main ideas explored consist of different types of model architectures, loss functions, and occlusion masks to address non-rigid motion. In this paper, we propose two new ideas to improve self-supervised monocular trained depth estimation: 1) self-attention, and 2) discrete disparity prediction. Compared with the usual localised convolution operation, self-attention can explore a more general contextual information that allows the inference of similar disparity values at non-contiguous regions of the image. Discrete disparity prediction has been shown by fully supervised methods to provide a more robust and sharper depth estimation than the more common continuous disparity prediction, besides enabling the estimation of depth uncertainty. We show that the extension of the state-of-the-art self-supervised monocular trained depth estimator Monodepth2 with these two ideas allows us to design a model that produces the best results in the field in KITTI 2015 and Make3D, closing the gap with respect self-supervised stereo training and fully supervised approaches.
Meta-learning is an effective method to handle imbalanced and noisy-label learning, but it depends on a validation set containing randomly selected, manually labelled and balanced distributed samples. The random selection and manual labelling and balancing of this validation set is not only sub-optimal for meta-learning, but it also scales poorly with the number of classes. Hence, recent meta-learning papers have proposed ad-hoc heuristics to automatically build and label this validation set, but these heuristics are still sub-optimal for meta-learning. In this paper, we analyse the meta-learning algorithm and propose new criteria to characterise the utility of the validation set, based on: 1) the informativeness of the validation set; 2) the class distribution balance of the set; and 3) the correctness of the labels of the set. Furthermore, we propose a new imbalanced noisy-label meta-learning (INOLML) algorithm that automatically builds a validation set by maximising its utility using the criteria above. Our method shows significant improvements over previous meta-learning approaches and sets the new state-of-the-art on several benchmarks.
To solve deep metric learning problems and producing feature embeddings, current methodologies will commonly use a triplet model to minimise the relative distance between samples from the same class and maximise the relative distance between samples from different classes. Though successful, the training convergence of this triplet model can be compromised by the fact that the vast majority of the training samples will produce gradients with magnitudes that are close to zero. This issue has motivated the development of methods that explore the global structure of the embedding and other methods that explore hard negative/positive mining. The effectiveness of such mining methods is often associated with intractable computational requirements. In this paper, we propose a novel deep metric learning method that combines the triplet model and the global structure of the embedding space. We rely on a smart mining procedure that produces effective training samples for a low computational cost. In addition, we propose an adaptive controller that automatically adjusts the smart mining hyper-parameters and speeds up the convergence of the training process. We show empirically that our proposed method allows for fast and more accurate training of triplet ConvNets than other competing mining methods. Additionally, we show that our method achieves new state-of-the-art embedding results for CUB-200-2011 and Cars196 datasets.
Unsupervised anomaly detection (UAD) aims to find anomalous images by optimising a detector using a training set that contains only normal images. UAD approaches can be based on reconstruction methods, self-supervised approaches, and Imagenet pre-trained models. Reconstruction methods, which detect anomalies from image reconstruction errors, are advantageous because they do not rely on the design of problem-specific pretext tasks needed by self-supervised approaches, and on the unreliable translation of models pre-trained from non-medical datasets. However, reconstruction methods may fail because they can have low reconstruction errors even for anomalous images. In this paper, we introduce a new reconstruction-based UAD approach that addresses this low-reconstruction error issue for anomalous images. Our UAD approach, the memory-augmented multi-level cross-attentional masked autoencoder (MemMC-MAE), is a transformer-based approach, consisting of a novel memory-augmented self-attention operator for the encoder and a new multi-level cross-attention operator for the decoder. MemMCMAE masks large parts of the input image during its reconstruction, reducing the risk that it will produce low reconstruction errors because anomalies are likely to be masked and cannot be reconstructed. However, when the anomaly is not masked, then the normal patterns stored in the encoder's memory combined with the decoder's multi-level cross attention will constrain the accurate reconstruction of the anomaly. We show that our method achieves SOTA anomaly detection and localisation on colonoscopy, pneumonia, and covid-19 chest x-ray datasets.
Deep neural network models are robust to a limited amount of label noise, but their ability to memorise noisy labels in high noise rate problems is still an open issue. The most competitive noisy-label learning algorithms rely on a 2-stage process comprising an unsupervised learning to classify training samples as clean or noisy, followed by a semi-supervised learning that minimises the empirical vicinal risk (EVR) using a labelled set formed by samples classified as clean, and an unlabelled set with samples classified as noisy. In this paper, we hypothesise that the generalisation of such 2-stage noisy-label learning methods depends on the precision of the unsupervised classifier and the size of the training set to minimise the EVR. We empirically validate these two hypotheses and propose the new 2-stage noisy-label training algorithm LongReMix. We test LongReMix on the noisy-label benchmarks CIFAR-10, CIFAR-100, WebVision, Clothing1M, and Food101-N. The results show that our LongReMix generalises better than competing approaches, particularly in high label noise problems. Furthermore, our approach achieves state-of-the-art performance in most datasets. The code is available at https://github.com/filipe-research/LongReMix.
Label noise is common in large real-world datasets, and its presence harms the training process of deep neural networks. Although several works have focused on the training strategies to address this problem, there are few studies that evaluate the impact of data augmentation as a design choice for training deep neural networks. In this work, we analyse the model robustness when using different data augmentations and their improvement on the training with the presence of noisy labels. We evaluate state-of-the-art and classical data augmentation strategies with different levels of synthetic noise for the datasets MNist, CIFAR-10, CIFAR-100, and the real-world dataset Clothing1M. We evaluate the methods using the accuracy metric. Results show that the appropriate selection of data augmentation can drastically improve the model robustness to label noise, increasing up to 177.84% of relative best test accuracy compared to the baseline with no augmentation, and an increase of up to 6% in absolute value with the state-of-the-art DivideMix training strategy.
Foundations and Trends in Robotics: Vol. 8: No. 1-2, pp 1-224 (2020) For robots to navigate and interact more richly with the world around them, they will likely require a deeper understanding of the world in which they operate. In robotics and related research fields, the study of understanding is often referred to as semantics, which dictates what does the world "mean" to a robot, and is strongly tied to the question of how to represent that meaning. With humans and robots increasingly operating in the same world, the prospects of human-robot interaction also bring semantics and ontology of natural language into the picture. Driven by need, as well as by enablers like increasing availability of training data and computational resources, semantics is a rapidly growing research area in robotics. The field has received significant attention in the research literature to date, but most reviews and surveys have focused on particular aspects of the topic: the technical research issues regarding its use in specific robotic topics like mapping or segmentation, or its relevance to one particular application domain like autonomous driving. A new treatment is therefore required, and is also timely because so much relevant research has occurred since many of the key surveys were published. This survey therefore provides an overarching snapshot of where semantics in robotics stands today. We establish a taxonomy for semantics research in or relevant to robotics, split into four broad categories of activity, in which semantics are extracted, used, or both. Within these broad categories we survey dozens of major topics including fundamentals from the computer vision field and key robotics research areas utilizing semantics, including mapping, navigation and interaction with the world. The survey also covers key practical considerations, including enablers like increased data availability and improved computational hardware, and major application areas where...
The deployment of automated systems to diagnose diseases from medical images is challenged by the requirement to localise the diagnosed diseases to justify or explain the classification decision. This requirement is hard to fulfil because most of the training sets available to develop these systems only contain global annotations, making the localisation of diseases a weakly supervised approach. The main methods designed for weakly supervised disease classification and localisation rely on saliency or attention maps that are not specifically trained for localisation, or on region proposals that can not be refined to produce accurate detections. In this paper, we introduce a new model that combines region proposal and saliency detection to overcome both limitations for weakly supervised disease classification and localisation. Using the ChestX-ray14 data set, we show that our proposed model establishes the new state-of-the-art for weakly-supervised disease diagnosis and localisation.
Minimally invasive surgery (MIS) has many documented advantages, but the surgeon's limited visual contact with the scene can be problematic. Hence, systems that can help surgeons navigate, such as a method that can produce a 3D semantic map, can compensate for the limitation above. In theory, we can borrow 3D semantic mapping techniques developed for robotics, but this requires finding solutions to the following challenges in MIS: 1) semantic segmentation, 2) depth estimation, and 3) pose estimation. In this paper, we propose the first 3D semantic mapping system from knee arthroscopy that solves the three challenges above. Using out-of-distribution non-human datasets, where pose could be labeled, we jointly train depth+pose estimators using selfsupervised and supervised losses. Using an in-distribution human knee dataset, we train a fully-supervised semantic segmentation system to label arthroscopic image pixels into femur, ACL, and meniscus. Taking testing images from human knees, we combine the results from these two systems to automatically create 3D semantic maps of the human knee. The result of this work opens the pathway to the generation of intraoperative 3D semantic mapping, registration with pre-operative data, and robotic-assisted arthroscopy
This paper compares well-established Convolutional Neural Networks (CNNs) to recently introduced Vision Transformers for the task of Diabetic Foot Ulcer Classification, in the context of the DFUC 2021 Grand-Challenge, in which this work attained the first position. Comprehensive experiments demonstrate that modern CNNs are still capable of outperforming Transformers in a low-data regime, likely owing to their ability for better exploiting spatial correlations. In addition, we empirically demonstrate that the recent Sharpness-Aware Minimization (SAM) optimization algorithm considerably improves the generalization capability of both kinds of models. Our results demonstrate that for this task, the combination of CNNs and the SAM optimization process results in superior performance than any other of the considered approaches.
Meta-training has been empirically demonstrated to be the most effective pre-training method for few-shot learning of medical image classifiers (i.e., classifiers modeled with small training sets). However, the effectiveness of meta-training relies on the availability of a reasonable number of hand-designed classification tasks, which are costly to obtain, and consequently rarely available. In this paper, we propose a new method to unsupervisedly design a large number of classification tasks to meta-train medical image classifiers. We evaluate our method on a breast dynamically contrast enhanced magnetic resonance imaging (DCE-MRI) data set that has been used to benchmark few-shot training methods of medical image classifiers. Our results show that the proposed unsupervised task design to meta-train medical image classifiers builds a pre-trained model that, after fine-tuning, produces better classification results than other unsupervised and supervised pre-training methods, and competitive results with respect to meta-training that relies on hand-designed classification tasks.
In this paper, we hypothesize that it is possible to localize image regions of preclinical tumors in a Chest X-ray (CXR) image by a weakly-supervised training of a survival prediction model using a dataset containing CXR images of healthy patients and their time-to-death label. These visual explanations can empower clinicians in early lung cancer detection and increase patient awareness of their susceptibility to the disease. To test this hypothesis, we train a censor-aware multi-class survival prediction deep learning classifier that is robust to imbalanced training, where classes represent quantized number of days for time-to-death prediction. Such multi-class model allows us to use post-hoc interpretability methods, such as Grad-CAM, to localize image regions of preclinical tumors. For the experiments, we propose a new benchmark based on the National Lung Cancer Screening Trial (NLST) dataset to test weakly-supervised preclinical tumor localization and survival prediction models, and results suggest that our proposed method shows state-of-the-art C-index survival prediction and weakly-supervised preclinical tumor localization results. To our knowledge, this constitutes a pioneer approach in the field that is able to produce visual explanations of preclinical events associated with survival prediction results. •First weakly-supervised survival prediction method to localize preclinical tumors.•State-of-the-art survival time prediction results on the NLST dataset.•Innovative explainable censor-aware multi-class survival prediction.•Innovative survival prediction robust to imbalanced time-to-death distribution.•New benchmark for explainable preclinical survival prediction using the NLST dataset.
Consistency learning using input image, feature, or network perturbations has shown remarkable results in semi-supervised semantic segmentation, but this approach can be seriously affected by inaccurate predictions of unlabelled training images. There are two consequences of these inaccurate predictions: 1) the training based on the "strict" cross-entropy (CE) loss can easily overfit prediction mistakes, leading to confirmation bias; and 2) the perturbations applied to these inaccurate predictions will use potentially erroneous predictions as training signals, degrading consistency learning. In this paper, we address the prediction accuracy problem of consistency learning methods with novel extensions of the mean-teacher (MT) model, which include a new auxiliary teacher, and the replacement of MT's mean square error (MSE) by a stricter confidence-weighted cross-entropy (Conf-CE) loss. The accurate prediction by this model allows us to use a challenging combination of network, input data and feature perturbations to improve the consistency learning generalisation, where the feature perturbations consist of a new adversarial perturbation. Results on public benchmarks show that our approach achieves remarkable improvements over the previous SOTA methods in the field. Our code is available at https://github.com/yyliu01/PS-MT.
MICCAI 2021 Highly imbalanced datasets are ubiquitous in medical image classification problems. In such problems, it is often the case that rare classes associated to less prevalent diseases are severely under-represented in labeled databases, typically resulting in poor performance of machine learning algorithms due to overfitting in the learning process. In this paper, we propose a novel mechanism for sampling training data based on the popular MixUp regularization technique, which we refer to as Balanced-MixUp. In short, Balanced-MixUp simultaneously performs regular (i.e., instance-based) and balanced (i.e., class-based) sampling of the training data. The resulting two sets of samples are then mixed-up to create a more balanced training distribution from which a neural network can effectively learn without incurring in heavily under-fitting the minority classes. We experiment with a highly imbalanced dataset of retinal images (55K samples, 5 classes) and a long-tail dataset of gastro-intestinal video frames (10K images, 23 classes), using two CNNs of varying representation capabilities. Experimental results demonstrate that applying Balanced-MixUp outperforms other conventional sampling schemes and loss functions specifically designed to deal with imbalanced data. Code is released at https://github.com/agaldran/balanced_mixup .
Recent advances in meta-learning has led to remarkable performances on several few-shot learning benchmarks. However, such success often ignores the similarity between training and testing tasks, resulting in a potential bias evaluation. We, therefore, propose a generative approach based on a variant of Latent Dirichlet Allocation to analyse task similarity to optimise and better understand the performance of meta-learning. We demonstrate that the proposed method can provide an insightful evaluation for meta-learning algorithms on two few-shot classification benchmarks that matches common intuition: the more similar the higher performance. Based on this similarity measure, we propose a task-selection strategy for meta-learning and show that it can produce more accurate classification results than methods that randomly select training tasks.
The efficacy of deep learning depends on large-scale data sets that have been carefully curated with reliable data acquisition and annotation processes. However, acquiring such large-scale data sets with precise annotations is very expensive and time-consuming, and the cheap alternatives often yield data sets that have noisy labels. The field has addressed this problem by focusing on training models under two types of label noise: 1) closed-set noise, where some training samples are incorrectly annotated to a training label other than their known true class; and 2) open-set noise, where the training set includes samples that possess a true class that is (strictly) not contained in the set of known training labels. In this work, we study a new variant of the noisy label problem that combines the open-set and closed-set noisy labels, and introduce a benchmark evaluation to assess the performance of training algorithms under this setup. We argue that such problem is more general and better reflects the noisy label scenarios in practice. Furthermore, we propose a novel algorithm, called EvidentialMix, that addresses this problem and compare its performance with the state-of-the-art methods for both closed-set and open-set noise on the proposed benchmark. Our results show that our method produces superior classification results and better feature representations than previous state-of-the-art methods. The code is available at https://github.com/ragavsachdeva/EvidentialMix.
Anomaly detection methods generally target the learning of a normal image distribution (i.e., inliers showing healthy cases) and during testing, samples relatively far from the learned distribution are classified as anomalies (i.e., outliers showing disease cases). These approaches tend to be sensitive to outliers that lie relatively close to inliers (e.g., a colonoscopy image with a small polyp). In this paper, we address the inappropriate sensitivity to outliers by also learning from inliers. We propose a new few-shot anomaly detection method based on an encoder trained to maximise the mutual information between feature embeddings and normal images, followed by a few-shot score inference network, trained with a large set of inliers and a substantially smaller set of outliers. We evaluate our proposed method on the clinical problem of detecting frames containing polyps from colonoscopy video sequences, where the training set has 13350 normal images (i.e., without polyps) and less than 100 abnormal images (i.e., with polyps). The results of our proposed model on this data set reveal a state-of-the-art detection result, while the performance based on different number of anomaly samples is relatively stable after approximately 40 abnormal training images.
There is a heated debate on how to interpret the decisions provided by deep learning models (DLM), where the main approaches rely on the visualization of salient regions to interpret the DLM classification process. However, these approaches generally fail to satisfy three conditions for the problem of lesion detection from medical images: 1) for images with lesions, all salient regions should represent lesions, 2) for images containing no lesions, no salient region should be produced,and 3) lesions are generally small with relatively smooth borders. We propose a new model-agnostic paradigm to interpret DLM classification decisions supported by a novel definition of saliency that incorporates the conditions above. Our model-agnostic 1-class saliency detector (MASD) is tested on weakly supervised breast lesion detection from DCE-MRI, achieving state-of-the-art detection accuracy when compared to current visualization methods.
Prototypical-part methods, e.g., ProtoPNet, enhance interpretability in image recognition by linking predictions to training prototypes, thereby offering intuitive insights into their decision-making. Existing methods, which rely on a point-based learning of prototypes, typically face two critical issues: 1) the learned prototypes have limited representation power and are not suitable to detect Out-of-Distribution (OoD) inputs, reducing their decision trustworthiness; and 2) the necessary projection of the learned prototypes back into the space of training images causes a drastic degradation in the predictive performance. Furthermore, current prototype learning adopts an aggressive approach that considers only the most active object parts during training, while overlooking sub-salient object regions which still hold crucial classification information. In this paper, we present a new generative paradigm to learn prototype distributions, termed as Mixture of Gaussian-distributed Prototypes (MGProto). The distribution of prototypes from MGProto enables both interpretable image classification and trustworthy recognition of OoD inputs. The optimisation of MGProto naturally projects the learned prototype distributions back into the training image space, thereby addressing the performance degradation caused by prototype projection. Additionally, we develop a novel and effective prototype mining strategy that considers not only the most active but also sub-salient object parts. To promote model compactness, we further propose to prune MGProto by removing prototypes with low importance priors. Experiments on CUB-200-2011, Stanford Cars, Stanford Dogs, and Oxford-IIIT Pets datasets show that MGProto achieves state-of-the-art image recognition and OoD detection performances, while providing encouraging interpretability results.
3D medical image segmentation methods have been successful, but their dependence on large amounts of voxel-level annotated data is a disadvantage that needs to be addressed given the high cost to obtain such annotation. Semi-supervised learning (SSL) solve this issue by training models with a large unlabelled and a small labelled dataset. The most successful SSL approaches are based on consistency learning that minimises the distance between model responses obtained from perturbed views of the unlabelled data. These perturbations usually keep the spatial input context between views fairly consistent, which may cause the model to learn segmentation patterns from the spatial input contexts instead of the segmented objects. In this paper, we introduce the Translation Consistent Co-training (TraCoCo) which is a consistency learning SSL method that perturbs the input data views by varying their spatial input context, allowing the model to learn segmentation patterns from visual objects. Furthermore, we propose the replacement of the commonly used mean squared error (MSE) semi-supervised loss by a new Cross-model confident Binary Cross entropy (CBC) loss, which improves training convergence and keeps the robustness to co-training pseudo-labelling mistakes. We also extend CutMix augmentation to 3D SSL to further improve generalisation. Our TraCoCo shows state-of-the-art results for the Left Atrium (LA) and Brain Tumor Segmentation (BRaTS19) datasets with different backbones. Our code is available at https://github.com/yyliu01/TraCoCo.
IEEE/CVF Winter Conference on Applications of Computer Vision (WACV), 2020, pp. 3090-3100 We introduce a new, rigorously-formulated Bayesian meta-learning algorithm that learns a probability distribution of model parameter prior for few-shot learning. The proposed algorithm employs a gradient-based variational inference to infer the posterior of model parameters to a new task. Our algorithm can be applied to any model architecture and can be implemented in various machine learning paradigms, including regression and classification. We show that the models trained with our proposed meta-learning algorithm are well calibrated and accurate, with state-of-the-art calibration and classification results on two few-shot classification benchmarks (Omniglot and Mini-ImageNet), and competitive results in a multi-modal task-distribution regression.
The diagnosis of the presence of metastatic lymph nodes from abdominal computed tomography (CT) scans is an essential task performed by radiologists to guide radiation and chemotherapy treatment. State-of-the-art deep learning classifiers trained for this task usually rely on a training set containing CT volumes and their respective image-level (i.e., global) annotation. However, the lack of annotations for the localisation of the regions of interest (ROIs) containing lymph nodes can limit classification accuracy due to the small size of the relevant ROIs in this problem. The use of lymph node ROIs together with global annotations in a multi-task training process has the potential to improve classification accuracy, but the high cost involved in obtaining the ROI annotation for the same samples that have global annotations is a roadblock for this alternative. We address this limitation by introducing a new training strategy from two data sets: one containing the global annotations, and another (publicly available) containing only the lymph node ROI localisation. We term our new strategy semi-supervised multi-domain multi-task training, where the goal is to improve the diagnosis accuracy on the globally annotated data set by incorporating the ROI annotations from a different domain. Using a private data set containing global annotations and a public data set containing lymph node ROI localisation, we show that our proposed training mechanism improves the area under the ROC curve for the classification task compared to several training method baselines.
Domain generalisation (DG) methods address the problem of domain shift, when there is a mismatch between the distributions of training and target domains. Data augmentation approaches have emerged as a promising alternative for DG. However, data augmentation alone is not sufficient to achieve lower generalisation errors. This project proposes a new method that combines data augmentation and domain distance minimisation to address the problems associated with data augmentation and provide a guarantee on the learning performance, under an existing framework. Empirically, our method outperforms baseline results on DG benchmarks.
We developed an automated deep learning system to detect hip fractures from frontal pelvic x-rays, an important and common radiological task. Our system was trained on a decade of clinical x-rays (~53,000 studies) and can be applied to clinical data, automatically excluding inappropriate and technically unsatisfactory studies. We demonstrate diagnostic performance equivalent to a human radiologist and an area under the ROC curve of 0.994. Translated to clinical practice, such a system has the potential to increase the efficiency of diagnosis, reduce the need for expensive additional testing, expand access to expert level medical image interpretation, and improve overall patient outcomes.
Automatic cell segmentation in microscopy images works well with the support of deep neural networks trained with full supervision. Collecting and annotating images, though, is not a sustainable solution for every new microscopy database and cell type. Instead, we assume that we can access a plethora of annotated image data sets from different domains (sources) and a limited number of annotated image data sets from the domain of interest (target), where each domain denotes not only different image appearance but also a different type of cell segmentation problem. We pose this problem as meta-learning where the goal is to learn a generic and adaptable few-shot learning model from the available source domain data sets and cell segmentation tasks. The model can be afterwards fine-tuned on the few annotated images of the target domain that contains different image appearance and different cell type. In our meta-learning training, we propose the combination of three objective functions to segment the cells, move the segmentation results away from the classification boundary using cross-domain tasks, and learn an invariant representation between tasks of the source domains. Our experiments on five public databases show promising results from 1- to 10-shot meta-learning using standard segmentation neural network architectures.
One-class classification (OCC) aims to learn an effective data description to enclose all normal training samples and detect anomalies based on the deviation from the data description. Current state-of-the-art OCC models learn a compact normality description by hyper-sphere minimisation, but they often suffer from overfitting the training data, especially when the training set is small or contaminated with anomalous samples. To address this issue, we introduce the interpolated Gaussian descriptor (IGD) method, a novel OCC model that learns a one-class Gaussian anomaly classifier trained with adversarially interpolated training samples. The Gaussian anomaly classifier differentiates the training samples based on their distance to the Gaussian centre and the standard deviation of these distances, offering the model a discriminability w.r.t. the given samples during training. The adversarial interpolation is enforced to consistently learn a smooth Gaussian descriptor, even when the training data is small or contaminated with anomalous samples. This enables our model to learn the data description based on the representative normal samples rather than fringe or anomalous samples, resulting in significantly improved normality description. In extensive experiments on diverse popular benchmarks, including MNIST, Fashion MNIST, CIFAR10, MVTec AD and two medical datasets, IGD achieves better detection accuracy than current state-of-the-art models. IGD also shows better robustness in problems with small or contaminated training sets. Code is available at https://github.com/tianyu0207/IGD.
Current deep image super-resolution (SR) approaches aim to restore high-resolution images from down-sampled images or by assuming degradation from simple Gaussian kernels and additive noises. However, these techniques only assume crude approximations of the real-world image degradation process, which should involve complex kernels and noise patterns that are difficult to model using simple assumptions. In this paper, we propose a more realistic process to synthesise low-resolution images for real-world image SR by introducing a new Kernel Adversarial Learning Super-resolution (KASR) framework. In the proposed framework, degradation kernels and noises are adaptively modelled rather than explicitly specified. Moreover, we also propose a high-frequency selective objective and an iterative supervision process to further boost the model SR reconstruction accuracy. Extensive experiments validate the effectiveness of the proposed framework on real-world datasets.
Endometriosis affects 1 in 9 women and those assigned female at birth. However, it takes 6.4 years to diagnose using the conventional standard of laparoscopy. Noninvasive imaging enables a timelier diagnosis, reducing diagnostic delay as well as the risk and expense of surgery. This review updates the exponentially increasing literature exploring the diagnostic value of endometriosis specialist transvaginal ultrasound (eTVUS), combinations of eTVUS and specialist magnetic resonance imaging, and artificial intelligence. Concentrating on literature that emerged after the publication of the IDEA consensus in 2016, we identified 6192 publications and reviewed 49 studies focused on diagnosing endometriosis using emerging imaging techniques. The diagnostic performance of eTVUS continues to improve but there are still limitations. eTVUS reliably detects ovarian endometriomas, shows high specificity for deep endometriosis and should be considered diagnostic. However, a negative scan cannot preclude endometriosis as eTVUS shows moderate sensitivity scores for deep endometriosis, with the sonographic evaluation of superficial endometriosis still in its infancy. The fast-growing area of artificial intelligence in endometriosis detection is still evolving, but shows great promise, particularly in the area of combined multimodal techniques. We finalize our commentary by exploring the implications of practice change for surgeons, sonographers, radiologists, and fertility specialists. Direct benefits for endometriosis patients include reduced diagnostic delay, better access to targeted therapeutics, higher quality operative procedures, and improved fertility treatment plans.
Monitoring the healing progress of diabetic foot ulcers is a challenging process. Accurate segmentation of foot ulcers can help podiatrists to quantitatively measure the size of wound regions to assist prediction of healing status. The main challenge in this field is the lack of publicly available manual delineation, which can be time consuming and laborious. Recently, methods based on deep learning have shown excellent results in automatic segmentation of medical images, however, they require large-scale datasets for training, and there is limited consensus on which methods perform the best. The 2022 Diabetic Foot Ulcers segmentation challenge was held in conjunction with the 2022 International Conference on Medical Image Computing and Computer Assisted Intervention, which sought to address these issues and stimulate progress in this research domain. A training set of 2000 images exhibiting diabetic foot ulcers was released with corresponding segmentation ground truth masks. Of the 72 (approved) requests from 47 countries, 26 teams used this data to develop fully automated systems to predict the true segmentation masks on a test set of 2000 images, with the corresponding ground truth segmentation masks kept private. Predictions from participating teams were scored and ranked according to their average Dice similarity coefficient of the ground truth masks and prediction masks. The winning team achieved a Dice of 0.7287 for diabetic foot ulcer segmentation. This challenge has now entered a live leaderboard stage where it serves as a challenging benchmark for diabetic foot ulcer segmentation.
Introduction Lymph node (LN) metastases are an important determinant of survival in patients with colon cancer, but remain difficult to accurately diagnose on preoperative imaging. This study aimed to develop and evaluate a deep learning model to predict LN status on preoperative staging CT. Methods In this ambispective diagnostic study, a deep learning model using a ResNet-50 framework was developed to predict LN status based on preoperative staging CT. Patients with a preoperative staging abdominopelvic CT who underwent surgical resection for colon cancer were enrolled. Data were retrospectively collected from February 2007 to October 2019 and randomly separated into training, validation, and testing cohort 1. To prospectively test the deep learning model, data for testing cohort 2 was collected from October 2019 to July 2021. Diagnostic performance measures were assessed by the AUROC. Results A total of 1,201 patients (median [range] age, 72 [28–98 years]; 653 [54.4%] male) fulfilled the eligibility criteria and were included in the training (n = 401), validation (n = 100), testing cohort 1 (n = 500) and testing cohort 2 (n = 200). The deep learning model achieved an AUROC of 0.619 (95% CI 0.507–0.731) in the validation cohort. In testing cohort 1 and testing cohort 2, the AUROC was 0.542 (95% CI 0.489–0.595) and 0.486 (95% CI 0.403–0.568), respectively. Conclusion A deep learning model based on a ResNet-50 framework does not predict LN status on preoperative staging CT in patients with colon cancer.
The problem of missing modalities is both critical and non-trivial to be handled in multi-modal models. It is common for multi-modal tasks that certain modalities contribute more compared to other modalities, and if those important modalities are missing, the model performance drops significantly. Such fact remains unexplored by current multi-modal approaches that recover the representation from missing modalities by feature reconstruction or blind feature aggregation from other modalities, instead of extracting useful information from the best performing modalities. In this paper, we propose a Learnable Cross-modal Knowledge Distillation (LCKD) model to adaptively identify important modalities and distil knowledge from them to help other modalities from the cross-modal perspective for solving the missing modality issue. Our approach introduces a teacher election procedure to select the most “qualified” teachers based on their single modality performance on certain tasks. Then, cross-modal knowledge distillation is performed between teacher and student modalities for each task to push the model parameters to a point that is beneficial for all tasks. Hence, even if the teacher modalities for certain tasks are missing during testing, the available student modalities can accomplish the task well enough based on the learned knowledge from their automatically elected teacher modalities. Experiments on the Brain Tumour Segmentation Dataset 2018 (BraTS2018) shows that LCKD outperforms other methods by a considerable margin, improving the state-of-the-art performance by 3.61% for enhancing tumour, 5.99% for tumour core, and 3.76% for whole tumour in terms of segmentation Dice score.
Delivering meaningful uncertainty estimates is essential for a successful deployment of machine learning models in the clinical practice. A central aspect of uncertainty quantification is the ability of a model to return predictions that are well-aligned with the actual probability of the model being correct, also known as model calibration. Although many methods have been proposed to improve calibration, no technique can match the simple, but expensive approach of training an ensemble of deep neural networks. In this paper we introduce a form of simplified ensembling that bypasses the costly training and inference of deep ensembles, yet it keeps its calibration capabilities. The idea is to replace the common linear classifier at the end of a network by a set of heads that are supervised with different loss functions to enforce diversity on their predictions. Specifically, each head is trained to minimize a weighted Cross-Entropy loss, but the weights are different among the different branches. We show that the resulting averaged predictions can achieve excellent calibration without sacrificing accuracy in two challenging datasets for histopathological and endoscopic image classification. Our experiments indicate that Multi-Head Multi-Loss classifiers are inherently well-calibrated, outperforming other recent calibration techniques and even challenging Deep Ensembles’ performance.
Molecular imaging is a key tool in the diagnosis and treatment of prostate cancer (PCa). Magnetic Resonance (MR) plays a major role in this respect with nuclear medicine imaging, particularly, Prostate-Specific Membrane Antigen-based, (PSMA-based) positron emission tomography with computed tomography (PET/CT) also playing a major role of rapidly increasing importance. Another key technology finding growing application across medicine and specifically in molecular imaging is the use of machine learning (ML) and artificial intelligence (AI). Several authoritative reviews are available of the role of MR-based molecular imaging with a sparsity of reviews of the role of PET/CT. This review will focus on the use of AI for molecular imaging for PCa. It will aim to achieve two goals: firstly, to give the reader an introduction to the AI technologies available, and secondly, to provide an overview of AI applied to PET/CT in PCa. The clinical applications include diagnosis, staging, target volume definition for treatment planning, outcome prediction and outcome monitoring. ML and AL techniques discussed include radiomics, convolutional neural networks (CNN), generative adversarial networks (GAN) and training methods: supervised, unsupervised and semi-supervised learning.
The early detection of glaucoma is essential in preventing visual impairment. Artificial intelligence (AI) can be used to analyze color fundus photographs (CFPs) in a cost-effective manner, making glaucoma screening more accessible. While AI models for glaucoma screening from CFPs have shown promising results in laboratory settings, their performance decreases significantly in real-world scenarios due to the presence of out-of-distribution and low-quality images. To address this issue, we propose the Artificial Intelligence for Robust Glaucoma Screening (AIROGS) challenge. This challenge includes a large dataset of around 113,000 images from about 60,000 patients and 500 different screening centers, and encourages the development of algorithms that are robust to ungradable and unexpected input data. We evaluated solutions from 14 teams in this paper and found that the best teams performed similarly to a set of 20 expert ophthalmologists and optometrists. The highest-scoring team achieved an area under the receiver operating characteristic curve of 0.99 (95% CI: 0.98-0.99) for detecting ungradable images on-the-fly. Additionally, many of the algorithms showed robust performance when tested on three other publicly available datasets. These results demonstrate the feasibility of robust AI-enabled glaucoma screening.
Bayesian Neural Networks (BNNs) offer probability distributions for model parameters, enabling uncertainty quantification in predictions. However, they often underperform compared to deterministic neural networks. Utilizing mutual learning can effectively enhance the performance of peer BNNs. In this paper, we propose a novel approach to improve BNNs performance through deep mutual learning. The proposed approaches aim to increase diversity in both network parameter distributions and feature distributions, promoting peer networks to acquire distinct features that capture different characteristics of the input, which enhances the effectiveness of mutual learning. Experimental results demonstrate significant improvements in the classification accuracy, negative log-likelihood, and expected calibration error when compared to traditional mutual learning for BNNs.
Applications for Machine Learning in Healthcare have rapidly increased in recent years. In particular, the analysis of images using machine learning and computer vision is one of the most important domains in the area. The idea that machines can outperform the human eye in recognizing subtle patterns is not new, but it is now gaining momentum with large financial investments and the arrival of many startups with a focus in this area. Several examples illustrate that machine learning has enabled us to detect more diffuse patterns that are difficult to detect by non-experts. This chapter provides a state-of-the-art review of machine learning and computer vision in medical image analysis. We start with a brief introduction to computer vision and an overview of deep learning architectures. We proceed to highlight relevant progress in clinical development and translation across various medical specialties of dermatology, pathology, ophthalmology, radiology, and cardiology, focusing on the domains of computer vision and machine learning. Furthermore, we introduce some of the challenges that the disciplines of computer vision and machine learning face within a traditional regulatory environment. This chapter highlights the developments of computer vision and machine learning in medicine by displaying a breadth of powerful examples that give the reader an understanding of the potential impact and challenges that computer vision and machine learning can play in the clinical environment.
Endometriosis affects 1 in 9 women, taking 6.4 years to diagnose using conventional laparoscopy. Non-invasive imaging enables timelier diagnosis, reducing diagnostic delay, risk and expense of surgery. This review updates literature exploring the diagnostic value of specialist endometriosis magnetic resonance imaging (eMRI), nuclear medicine (NM) and computed tomography (CT). Searching after the 2016 IDEA consensus, 6192 publications were identified, with 27 studies focused on imaging for endometriosis. eMRI was the subject of 14 papers, NM and CT, 11, and artificial intelligence (AI) utilizing eMRI, 2. eMRI papers describe diagnostic accuracy for endometriosis, methodologies, and innovations. Advantages of eMRI include its: ability to diagnose endometriosis in those unable to tolerate transvaginal endometriosis ultrasound (eTVUS); a panoramic pelvic view, easy translation to surgical fields; identification of hyperintense iron in endometriotic lesions; and ability to identify super-pelvic lesions. Sequence standardization means eMRI is less operator-dependent than eTVUS, but higher costs limit its role to a secondary diagnostic modality. eMRI for deep and ovarian endometriosis has sensitivities of 91-93.5% and specificities of 86-87.5% making it reliable for surgical mapping and diagnosis. Superficial lesions too small for detection in larger capture sequences, means a negative eMRI doesn’t exclude endometriosis. Combined with thin sequence capture and improved reader expertise, eMRI is poised for rapid adoption into clinical practice. NM labeling is diagnostically limited in absence of suitable unique marker for endometrial-like tissue. CT studies expose the reproductively aged to radiation. AI diagnostic tools, combining independent eMRI and eTVUS endometriosis markers, may result in powerful capability. Broader eMRI use, will optimize standards and protocols. Reporting systems correlating to surgical anatomy will facilitate interdisciplinary preoperative dialogues. eMRI endometriosis diagnosis should reduce repeat surgeries with mental and physical health benefits for patients. There is potential for early eMRI diagnoses to prevent chronic pain syndromes and protect fertility outcomes.
Introduction: Machine learning has previously been applied to text analysis. There is limited data regarding the acceptability or accuracy of such applications in medical education. This project examined medical student opinion regarding computer-based marking and evaluated the accuracy of deep learning (DL), a subtype of machine learning, in the scoring of medical short answer questions (SAQs). Methods: Fourth- and fifth-year medical students undertook an anonymised online examination. Prior to the examination, students completed a survey gauging their opinion on computer-based marking. Questions were marked by humans, and then a DL analysis was conducted using convolutional neural networks. In the DL analysis, following preprocessing, data were split into a training dataset (on which models were developed using 10-fold cross-validation) and a test dataset (on which performance analysis was conducted). Results: One hundred and eighty-one students completed the examination (participation rate 59.0%). While students expressed concern regarding the accuracy of computer-based marking, the majority of students agreed that computer marking would be more objective than human marking (67.0%) and reported they would not object to computer-based marking (55.5%). Regarding automated marking of SAQs, for 1-mark questions, there were consistently high classification accuracies (mean accuracy 0.98). For more complex 2-mark and 3-mark SAQs, in which multiclass classification was required, accuracy was lower (mean 0.65 and 0.59, respectively). Conclusions: Medical students may be supportive of computer-based marking due to its objectivity. DL has the potential to provide accurate marking of written questions, however further research into DL marking of medical examinations is required.
Knowledge distillation is an attractive approach for learning compact deep neural networks, which learns a lightweight student model by distilling knowledge from a complex teacher model. Attention-based knowledge distillation is a specific form of intermediate feature-based knowledge distillation that uses attention mechanisms to encourage the student to better mimic the teacher. However, most of the previous attention-based distillation approaches perform attention in the spatial domain, which primarily affects local regions in the input image. This may not be sufficient when we need to capture the broader context or global information necessary for effective knowledge transfer. In frequency domain, since each frequency is determined from all pixels of the image in spatial domain, it can contain global information about the image. Inspired by the benefits of the frequency domain, we propose a novel module that functions as an attention mechanism in the frequency domain. The module consists of a learnable global filter that can adjust the frequencies of student's features under the guidance of the teacher's features, which encourages the student's features to have patterns similar to the teacher's features. We then propose an enhanced knowledge review-based distillation model by leveraging the proposed frequency attention module. The extensive experiments with various teacher and student architectures on image classification and object detection benchmark datasets show that the proposed approach outperforms other knowledge distillation methods.
Medical image analysis models are not guaranteed to generalize beyond the image and task distributions used for training. This transfer-learning problem has been intensively investigated by the field, where several solutions have been proposed, such as pretraining using computer vision datasets, unsupervised pretraining using pseudo-labels produced by clustering techniques, self-supervised pretraining using contrastive learning with data augmentation, or pretraining based on image reconstruction. Despite fairly successful in practice, such transfer-learning approaches cannot offer the theoretical guarantees enabled by meta learning (ML) approaches which explicitly optimize an objective function that can improve the transferability of a learnt model to new image and task distributions. In this chapter, we present and discuss our recently proposed meta learning algorithms that can transfer learned models between different training and testing image and task distributions, where our main contribution lies in the way we design and sample classification and segmentation tasks to train medical image analysis models.
Noisy-labels are challenging for deep learning due to the high capacity of the deep models that can overfit noisy-label training samples. Arguably the most realistic and coincidentally challenging type of label noise is the instance-dependent noise (IDN), where the labelling errors are caused by the ambivalent information present in the images. The most successful label noise learning techniques to address IDN problems usually contain a noisy-label sample selection stage to separate clean and noisy-label samples during training. Such sample selection depends on a criterion, such as loss or gradient, and on a curriculum to define the proportion of training samples to be classified as clean at each training epoch. Even though the estimated noise rate from the training set appears to be a natural signal to be used in the definition of this curriculum, previous approaches generally rely on arbitrary thresholds or pre-defined selection functions to the best of our knowledge. This paper addresses this research gap by proposing a new noisy-label learning graphical model that can easily accommodate state-of-the-art (SOTA) noisy-label learning methods and provide them with a reliable noise rate estimate to be used in a new sample selection curriculum. We show empirically that our model integrated with many SOTA methods can improve their results in many IDN benchmarks, including synthetic and real-world datasets.
Precision medicine approaches rely on obtaining precise knowledge of the true state of health of an individual patient, which results from a combination of their genetic risks and environmental exposures. This approach is currently limited by the lack of effective and efficient non-invasive medical tests to define the full range of phenotypic variation associated with individual health. Such knowledge is critical for improved early intervention, for better treatment decisions, and for ameliorating the steadily worsening epidemic of chronic disease. We present proof-of-concept experiments to demonstrate how routinely acquired cross-sectional CT imaging may be used to predict patient longevity as a proxy for overall individual health and disease status using computer image analysis techniques. Despite the limitations of a modest dataset and the use of off-the-shelf machine learning methods, our results are comparable to previous ‘manual’ clinical methods for longevity prediction. This work demonstrates that radiomics techniques can be used to extract biomarkers relevant to one of the most widely used outcomes in epidemiological and clinical research – mortality, and that deep learning with convolutional neural networks can be usefully applied to radiomics research. Computer image analysis applied to routinely collected medical images offers substantial potential to enhance precision medicine initiatives.
One-class classification (OCC) aims to learn an effective data description to enclose all normal training samples and detect anomalies based on the deviation from the data description. Current state-of-the-art OCC models learn a compact normality description by hyper-sphere minimisation, but they often suffer from overfitting the training data, especially when the training set is small or contaminated with anomalous samples. To address this issue, we introduce the interpolated Gaussian descriptor (IGD) method, a novel OCC model that learns a one-class Gaussian anomaly classifier trained with adversarially interpolated training samples. The Gaussian anomaly classifier differentiates the training samples based on their distance to the Gaussian centre and the standard deviation of these distances, offering the model a discriminability w.r.t. the given samples during training The adversarial interpolation is enforced to consistently learn a smooth Gaussian descriptor, even when the training data is small or contaminated with anomalous samples. This enables our model to learn the data description based on the representative normal samples rather than fringe or anomalous samples, resulting in significantly improved normality description. In extensive experiments on diverse popular benchmarks, including MNIST, Fashion MNIST, CIFAR10, MVTec AD and two medical datasets, IGD achieves better detection accuracy than current state-of-the-art models. IGD also shows better robustness in problems with small or contaminated training sets.
Current approaches to explaining the decisions of deep learning systems for medical tasks have focused on visualising the elements that have contributed to each decision. We argue that such approaches are not enough to "open the black box" of medical decision making systems because they are missing a key component that has been used as a standard communication tool between doctors for centuries: language. We propose a model-agnostic interpretability method that involves training a simple recurrent neural network model to produce descriptive sentences to clarify the decision of deep learning classifiers. We test our method on the task of detecting hip fractures from frontal pelvic x-rays. This process requires minimal additional labelling despite producing text containing elements that the original deep learning classification model was not specifically trained to detect. The experimental results show that: 1) the sentences produced by our method consistently contain the desired information, 2) the generated sentences are preferred by the cohort of doctors tested compared to current tools that create saliency maps, and 3) the combination of visualisations and generated text is better than either alone.
Highly imbalanced datasets are ubiquitous in medical image classification problems. In such problems, it is often the case that rare classes associated to less prevalent diseases are severely under-represented in labeled databases, typically resulting in poor performance of machine learning algorithms due to overfitting in the learning process. In this paper, we propose a novel mechanism for sampling training data based on the popular MixUp regularization technique, which we refer to as Balanced-MixUp. In short, Balanced-MixUp simultaneously performs regular (i.e., instance-based) and balanced (i.e., class-based) sampling of the training data. The resulting two sets of samples are then mixed-up to create a more balanced training distribution from which a neural network can effectively learn without incurring in heavily under-fitting the minority classes. We experiment with a highly imbalanced dataset of retinal images (55K samples, 5 classes) and a long-tail dataset of gastro-intestinal video frames (10K images, 23 classes), using two CNNs of varying representation capabilities. Experimental results demonstrate that applying Balanced-MixUp outperforms other conventional sampling schemes and loss functions specifically designed to deal with Unbalanced data. Code is released at https://github.com/agaldran/balanced_mixup
In this paper we present a fully automated approach to the segmentation of pediatric brain tumors in multi-spectral 3-D magnetic resonance images. It is a top-down segmentation approach based on a Markov random field (MRF) model that combines probabilistic boosting trees (PBT) and lower-level segmentation via graph cuts. The PBT algorithm provides a strong discriminative observation model that classifies tumor appearance while a spatial prior takes into account the pair-wise homogeneity in terms of classification labels and multi-spectral voxel intensities. The discriminative model relies not only on observed local intensities but also on surrounding context for detecting candidate regions for pathology. A mathematically sound formulation for integrating the two approaches into a unified statistical framework is given. The proposed method is applied to the challenging task of detection and delineation of pediatric brain tumors. This segmentation task is characterized by a high non-uniformity of both the pathology and the surrounding non-pathologic brain tissue. A quantitative evaluation illustrates the robustness of the proposed method. Despite dealing with more complicated cases of pediatric brain tumors the results obtained are, mostly better than those reported for current state-of-the-art approaches to 3-D MR brain tumor segmentation in adult patients. The entire processing of one multi-spectral data set does not require any user interaction, and takes less time than previously proposed methods.
Introduction Despite global concerns about the safety and quality of health care, population-wide studies of hospital outcomes are uncommon. The SAFety, Effectiveness of care and Resource use among Australian Hospitals (SAFER Hospitals) study seeks to estimate the incidence of serious adverse events, mortality, unplanned rehospitalisations and direct costs following hospital encounters using nationwide data, and to assess the variation and trends in these outcomes. Methods and analysis SAFER Hospitals is a cohort study with retrospective and prospective components. The retrospective component uses data from 2012 to 2018 on all hospitalised patients age >= 18 years included in each State and Territories' Admitted Patient Collections. These routinely collected datasets record every hospital encounter from all public and most private hospitals using a standardised set of variables including patient demographics, primary and secondary diagnoses, procedures and patient status at discharge. The study outcomes are deaths, adverse events, readmissions and emergency care visits. Hospitalisation data will be linked to subsequent hospitalisations and each region's Emergency Department Data Collections and Death Registries to assess readmissions, emergency care encounters and deaths after discharge. Direct hospital costs associated with adverse outcomes will be estimated using data from the National Cost Data Collection. Variation in these outcomes among hospitals will be assessed adjusting for differences in hospitals' case-mix. The prospective component of the study will evaluate the temporal change in outcomes every 4 years from 2019 until 2030. Ethics and dissemination Human Research Ethics Committees of the respective Australian states and territories provided ethical approval to conduct this study. A waiver of informed consent was granted for the use of de-identified patient data. Study findings will be disseminated via presentations at conferences and publications in peer-reviewed journals.
Independent representations have recently attracted significant attention from the biological vision and cognitive science communities. It has been 1) argued that properties such as sparseness and independence play a major role in visual perception, and 2) shown that imposing such properties on visual representations originates receptive fields similar to those found in human vision. We present a study of the impact of feature independence in the performance of visual recognition architectures. The contributions of this study are of both theoretical and empirical natures, and support two main conclusions. The first is that the intrinsic complexity of the recognition problem (Bayes error) is higher for independent representations. The increase can be significant, close to 10% in the databases we considered. The second is that criteria commonly used in independent component analysis are not sufficient to eliminate all the dependencies that impact recognition. In fact, “independent components” can be less independent than previous representations, such as principal components or wavelet bases.
Convolutional Neural Networks (ConvNets) have successfully contributed to improve the accuracy of regression-based methods for computer vision tasks such as human pose estimation, landmark localization, and object detection. The network optimization has been usually performed with L2 loss and without considering the impact of outliers on the training process, where an outlier in this context is defined by a sample estimation that lies at an abnormal distance from the other training sample estimations in the objective space. In this work, we propose a regression model with ConvNets that achieves robustness to such outliers by minimizing Tukey's biweight function, an M-estimator robust to outliers, as the loss function for the ConvNet. In addition to the robust loss, we introduce a coarse-to-fine model, which processes input images of progressively higher resolutions for improving the accuracy of the regressed values. In our experiments, we demonstrate faster convergence and better generalization of our robust loss function for the tasks of human pose estimation and age estimation from face images. We also show that the combination of the robust loss function with the coarse-to-fine model produces comparable or better results than current state-of-the-art approaches in four publicly available human pose estimation datasets.
We study the impact of different loss functions on lesion segmentation from medical images. Although the Cross-Entropy (CE) loss is the most popular option when dealing with natural images, for biomedical image segmentation the soft Dice loss is often preferred due to its ability to handle imbalanced scenarios. On the other hand, the combination of both functions has also been successfully applied in this kind of tasks. A much less studied problem is the generalization ability of all these losses in the presence of Out-of-Distribution (OoD) data. This refers to samples appearing in test time that are drawn from a different distribution than training images. In our case, we train our models on images that always contain lesions, but in test time we also have lesion-free samples. We analyze the impact of the minimization of different loss functions on in-distribution performance, but also its ability to generalize to OoD data, via comprehensive experiments on polyp segmentation from endoscopic images and ulcer segmentation from diabetic feet images. Our findings are surprising: CE-Dice loss combinations that excel in segmenting in-distribution images have a poor performance when dealing with OoD data, which leads us to recommend the adoption of the CE loss for this kind of problems, due to its robustness and ability to generalize to OoD samples. Code associated to our experiments can be found at https://github.com/agaldran/lesion_losses_ood .
Deep neural networks currently deliver promising results for microscopy image cell segmentation, but they require large-scale labelled databases, which is a costly and time-consuming process. In this work, we relax the labelling requirement by combining self-supervised with semi-supervised learning. We propose the prediction of edge-based maps for self-supervising the training of the unlabelled images, which is combined with the supervised training of a small number of labelled images for learning the segmentation task. In our experiments, we evaluate on a few-shot microscopy image cell segmentation benchmark and show that only a small number of annotated images, e.g. 10% of the original training set, is enough for our approach to reach similar performance as with the fully annotated databases on 1- to 10-shots. Our code and trained models is made publicly available https://github.com/Yussef93/EdgeSSFewShotMicroscopy.
Nowadays the bag-of-visual-words is a very popular approach to perform the task of Visual Object Classification (VOC). Two key phases of VOC are the vocabulary building step, i.e. the construction of a `visual dictionary' including common codewords in the image corpus, and the assignment step, i.e. the encoding of the images by means of these codewords. Hard assignment of image descriptors to visual codewords is commonly used in both steps. However, as only a single visual word is assigned to a given feature descriptor, hard assignment may hamper the characterization of an image in terms of the distribution of visual words, which may lead to poor classification of the images. Conversely, soft assignment can improve classification results, by taking into account the relevance of the feature descriptor to more than one visual word. Fuzzy Set Theory (FST) is a natural way to accomplish soft assignment. In particular, fuzzy clustering can be well applied within the VOC framework. In this paper we investigate the effects of using the well-known Fuzzy C-means algorithm and its kernelized version to create the visual vocabulary and to perform image encoding. Preliminary results on the Pascal VOC data set show that fuzzy clustering can improve the encoding step of VOC. In particular, the use of KFCM provides better classification results than standard FCM and K-means.
The efficacy of deep learning depends on large-scale data sets that have been carefully curated with reliable data acquisition and annotation processes. However, acquiring such large-scale data sets with precise annotations is very expensive and time-consuming, and the cheap alternatives often yield data sets that have noisy labels. The field has addressed this problem by focusing on training models under two types of label noise: 1) closed-set noise, where some training samples are incorrectly annotated to a training label other than their known true class; and 2) open-set noise, where the training set includes samples that possess a true class that is (strictly) not contained in the set of known training labels. In this work, we study a new variant of the noisy label problem that combines the open-set and closed-set noisy labels, and introduce a benchmark evaluation to assess the performance of training algorithms under this setup. We argue that such problem is more general and better reflects the noisy label scenarios in practice. Furthermore, we propose a novel algorithm, called EvidentialMix, that addresses this problem and compare its performance with the state-of-the-art methods for both closed-set and open-set noise on the proposed benchmark. Our results show that our method produces superior classification results and better feature representations than previous state-of-the-art methods. The code is available at https:/github.com/ragavsachdeva/EvidentialMix.
Prototypical part network (ProtoPNet) methods have been designed to achieve interpretable classification by associating predictions with a set of training prototypes, which we refer to as trivial prototypes because they are trained to lie far from the classification boundary in the feature space. Note that it is possible to make an analogy between ProtoPNet and support vector machine (SVM) given that the classification from both methods relies on computing similarity with a set of training points (i.e., trivial prototypes in ProtoPNet, and support vectors in SVM). However, while trivial prototypes are located far from the classification boundary, support vectors are located close to this boundary, and we argue that this discrepancy with the well-established SVM theory can result in ProtoPNet models with inferior classification accuracy. In this paper, we aim to improve the classification of ProtoPNet with a new method to learn support prototypes that lie near the classification boundary in the feature space, as suggested by the SVM theory. In addition, we target the improvement of classification results with a new model, named ST-ProtoPNet, which exploits our support prototypes and the trivial prototypes to provide more effective classification. Experimental results on CUB-200-2011, Stanford Cars, and Stanford Dogs datasets demonstrate that ST-ProtoPNet achieves state-of-the-art classification accuracy and interpretability results. We also show that the proposed support prototypes tend to be better localised in the object of interest rather than in the background region.
Purpose Navigation in visually complex endoscopic environments requires an accurate and robust localisation system. This paper presents the single image deep learning based camera localisation method for orthopedic surgery. Methods The approach combines image information, deep learning techniques and bone-tracking data to estimate camera poses relative to the bone-markers. We have collected one arthroscopic video sequence for four knee flexion angles, per synthetic phantom knee model and a cadaveric knee-joint. Results Experimental results are shown for both a synthetic knee model and a cadaveric knee-joint with mean localisation errors of 9.66mm/0.85 degrees and 9.94mm/1.13 degrees achieved respectively. We have found no correlation between localisation errors achieved on synthetic and cadaveric images, and hence we predict that arthroscopic image artifacts play a minor role in camera pose estimation compared to constraints introduced by the presented setup. We have discovered that the images acquired for 90 degrees and 0 degrees knee flexion angles are respectively most and least informative for visual localisation. Conclusion The performed study shows deep learning performs well in visually challenging, feature-poor, knee arthroscopy environments, which suggests such techniques can bring further improvements to localisation in Minimally Invasive Surgery.
Label noise is common in large real-world datasets, and its presence harms the training process of deep neural networks. Although several works have focused on the training strategies to address this problem, there are few studies that evaluate the impact of data augmentation as a design choice for training deep neural networks. In this work, we analyse the model robustness when using different data augmentations and their improvement on the training with the presence of noisy labels. We evaluate state-of-the-art and classical data augmentation strategies with different levels of synthetic noise for the datasets MNist, CIFAR-10, CIFAR-100, and the real-world dataset Clothing1M. We evaluate the methods using the accuracy metric. Results show that the appropriate selection of data augmentation can drastically improve the model robustness to label noise, increasing up to 177.84% of relative best test accuracy compared to the baseline with no augmentation, and an increase of up to 6% in absolute value with the state-of-the-art DivideMix training strategy.
In microscopy image cell segmentation, it is common to train a deep neural network on source data, containing different types of microscopy images, and then fine-tune it using a support set comprising a few randomly selected and annotated training target images. In this paper, we argue that the random selection of unlabelled training target images to be annotated and included in the support set may not enable an effective fine-tuning process, so we propose a new approach to optimise this image selection process. Our approach involves a new scoring function to find informative unlabelled target images. In particular, we propose to measure the consistency in the model predictions on target images against specific data augmentations. However, we observe that the model trained with source datasets does not reliably evaluate consistency on target images. To alleviate this problem, we propose novel self-supervised pretext tasks to compute the scores of unlabelled target images. Finally, the top few images with the least consistency scores are added to the support set for oracle (i.e., expert) annotation and later used to fine-tune the model to the target images. In our evaluations that involve the segmentation of five different types of cell images, we demonstrate promising results on several target test sets compared to the random selection approach as well as other selection approaches, such as Shannon's entropy and Monte-Carlo dropout.
The learning with noisy labels has been addressed with both discriminative and generative models. Although discriminative models have dominated the field due to their simpler modeling and more efficient computational training processes, generative models offer a more effective means of disentangling clean and noisy labels and improving the estimation of the label transition matrix. However, generative approaches maximize the joint likelihood of noisy labels and data using a complex formulation that only indirectly optimizes the model of interest associating data and clean labels. Additionally, these approaches rely on generative models that are challenging to train and tend to use uninformative clean label priors. In this paper, we propose a new generative noisy-label learning approach that addresses these three issues. First, we propose a new model optimisation that directly associates data and clean labels. Second, the generative model is implicitly estimated using a discriminative model, eliminating the inefficient training of a generative model. Third, we propose a new informative label prior inspired by partial label learning as supervision signal for noisy label learning. Extensive experiments on several noisy-label benchmarks demonstrate that our generative model provides state-of-the-art results while maintaining a similar computational complexity as discriminative models.
Deep learning architectures have achieved promising results in different areas (e.g., medicine, agriculture, and security). However, using those powerful techniques in many real applications becomes challenging due to the large labeled collections required during training. Several works have pursued solutions to overcome it by proposing strategies that can learn more for less, e.g., weakly and semi-supervised learning approaches. As these approaches do not usually address memorization and sensitivity to adversarial examples, this paper presents three deep metric learning approaches combined with Mixup for incomplete-supervision scenarios. We show that some state-of-the-art approaches in metric learning might not work well in such scenarios. Moreover, the proposed approaches outperform most of them in different datasets.
Convolutional Neural Networks (CNN) have been being widely employed to solve the challenging remote sensing task of aerial scene classification. Nevertheless, it is not straightforward to find single CNN models that can solve all aerial scene classification tasks, allowing the development of a better alternative, which is to fuse CNN-based classifiers into an ensemble. However, an appropriate choice of the classifiers that will belong to the ensemble is a critical factor, as it is unfeasible to employ all the possible classifiers in the literature. Therefore, this work proposes a novel framework based on meta-heuristic optimization for creating optimized ensembles in the context of aerial scene classification. The experimental results were performed across nine meta-heuristic algorithms and three aerial scene literature datasets, being compared in terms of effectiveness (accuracy), efficiency (execution time), and behavioral performance in different scenarios. Our results suggest that the Univariate Marginal Distribution Algorithm shows more effective and efficient results than other commonly used meta-heuristic algorithms, such as Genetic Programming and Particle Swarm Optimization.
The segmentation of masses from mammogram is a challenging problem because of their variability in terms of shape, appearance and size, and the low signal-to-noise ratio of their appearance. We address this problem with structured output prediction models that use potential functions based on deep convolution neural network (CNN) and deep belief network (DBN). The two types of structured output prediction models that we study in this work are the conditional random field (CRF) and structured support vector machines (SSVM). The label inference for CRF is based on tree re-weighted belief propagation (TRW) and training is achieved with the truncated fitting algorithm; whilst for the SSVM model, inference is based upon graph cuts and training depends on a max-margin optimization. We compare the results produced by our proposed models using the publicly available mammogram datasets DDSM-BCRP and INbreast, where the main conclusion is that both models produce results of similar accuracy, but the CRF model shows faster training and inference. Finally, when compared to the current state of the art in both datasets, the proposed CRF and SSVM models show superior segmentation accuracy.
Polyps are among the earliest sign of Colorectal Cancer, with their detection and segmentation representing a key milestone for automatic colonoscopy analysis. This works describes our solution to the EndoCV 2021 challenge, within the sub-track of polyp segmentation. We build on our recently developed framework of pretrained double encoder-decoder networks, which has achieved state-of-the-art results for this task, but we enhance the training process to account for the high variability and heterogeneity of the data provided in this competition. Specifically, since the available data comes from six different centers, it contains highly variable resolutions and image appearances. Therefore, we introduce a center-sampling training procedure by which the origin of each image is taken into account for deciding which images should be sampled for training. We also increase the representation capability of the encoder in our architecture, in order to provide a more powerful encoding step that can better capture the more complex information present in the data. Experimental results are promising and validate our approach for the segmentation of polyps in a highly heterogeneous data scenarios. Adrian Galdran was funded by a Marie Skłodowska-Curie Global Fellowship (No 892297).
Background and Aims: Artificial Intelligence (AI) is rapidly evolving in gastrointestinal (GI) endoscopy. We undertook a systematic review and meta-analysis to assess the performance of AI at detecting early Barrett's neoplasia. Methods: We searched Medline, EMBASE and Cochrane Central Register of controlled trials database from inception to the 28th Jan 2022 to identify studies on the detection of early Barrett's neoplasia using AI. Study quality was assessed using Quality Assessment of Diagnostic Accuracy Studies - 2 (QUADAS-2). A random-effects model was used to calculate pooled sensitivity, specificity, and diagnostics odds ratio (DOR). Forest plots and a summary of the receiving operating characteristics (SROC) curves displayed the outcomes. Heterogeneity was determined by I-2, Tau(2) statistics and p-value. The funnel plots and Deek's test were used to assess publication bias. Results: Twelve studies comprising of 1,361 patients (utilizing 532,328 images on which the various AI models were trained) were used. The SROC was 0.94 (95% CI: 0.92-0.96). Pooled sensitivity, specificity and diagnostic odds ratio were 90.3% (95% CI: 87.1-92.7%), 84.4% (95% CI: 80.2-87.9%) and 48.1 (95% CI: 28.4-81.5), respectively. Subgroup analysis of AI models trained only on white light endoscopy was similar with pooled sensitivity and specificity of 91.2% (95% CI: 85.7-94.7%) and 85.1% (95% CI: 81.6%-88.1%), respectively. Conclusions: AI is highly accurate at detecting early Barrett's neoplasia and validated for patients with at least high-grade dysplasia and above. Further well-designed prospective randomized controlled studies of all histopathological subtypes of early Barrett's neoplasia are needed to confirm these findings further.
Tissue typology annotation in Whole Slide histological images is a complex and tedious, yet necessary task for the development of computational pathology models. We propose to address this problem by applying Open Set Recognition techniques to the task of jointly classifying tissue that belongs to a set of annotated classes, e.g. clinically relevant tissue categories, while rejecting in test time Open Set samples, i.e. images that belong to categories not present in the training set. To this end, we introduce a new approach for Open Set histopathological image recognition based on training a model to accurately identify image categories and simultaneously predict which data augmentation transform has been applied. In test time, we measure model confidence in predicting this transform, which we expect to be lower for images in the Open Set. We carry out comprehensive experiments in the context of colorectal cancer assessment from histological images, which provide evidence on the strengths of our approach to automatically identify samples from unknown categories. Code is released at https://github.com/agaldran/t3po.
Background: Artificial intelligence (AI) readers, derived from applying deep learning models to medical image analysis, hold great promise for improving population breast cancer screening. However, previous evaluations of AI readers for breast cancer screening have mostly been conducted using cancer-enriched cohorts and have lacked assessment of the potential use of AI readers alongside radiologists in multi-reader screening programs. Here, we present a new AI reader for detecting breast cancer from mammograms in a large-scale population screening setting, and a novel analysis of the potential for human-AI reader collaboration in a well-established, high-performing population screening program. We evaluated the performance of our AI reader and AI-integrated screening scenarios using a two-year, real-world, population dataset from Victoria, Australia, a screening program in which two radiologists independently assess each episode and disagreements are arbitrated by a third radiologist. Methods: We used a retrospective full-field digital mammography image and non-image dataset comprising 808,318 episodes, 577,576 clients and 3,404,326 images in the period 2013 to 2019. Screening episodes from 2016, 2017 and 2018 were sequential population cohorts containing 752,609 episodes, 565,087 clients and 3,169,322 images. The dataset was split by screening date into training, development, and testing sets. All episodes from 2017 and 2018 were allocated to the testing set (509,109 episodes; 3,651 screen-detected cancer episodes). Eight distinct AI models were trained on subsets of the training set (which included a validation set) and combined into our ensemble AI reader. Operating points were selected using the development set. We evaluated our AI reader on our testing set and on external datasets previously unseen by our models. Findings: The AI reader outperformed the mean individual radiologist on this large retrospective testing dataset with an area under the receiver operator characteristic curve of 0.92 (95% CI 0.91, 0.92). The AI reader generalised well across screening round, client demographics, device manufacturer and cancer type, and achieved state-of-the-art performance on external datasets compared to recently published AI readers. Our simulations of AI-integrated screening scenarios demonstrated that a reader-replacement human-AI collaborative system could achieve better sensitivity and specificity (82.6%, 96.1%) compared to the current two-reader consensus system (79.9%, 96.0%), with reduced human reading workload and cost. Our band-pass AI-integrated scenario also enabled both higher sensitivity and specificity (80.6%, 96.2%) with larger reductions in human reading workload and cost. Interpretation: This study demonstrated that human-AI collaboration in a population breast cancer screening program has potential to improve accuracy and lower radiologist workload and costs in real world screening programs. The next stage of validation is to undertake prospective studies that can also assess the effects of the AI systems on human performance and behaviour.
Meta-training has been empirically demonstrated to be the most effective pre-training method for few-shot learning of medical image classifiers (i.e., classifiers modeled with small training sets). However, the effectiveness of meta-training relies on the availability of a reasonable number of hand-designed classification tasks, which are costly to obtain, and consequently rarely available. In this paper, we propose a new method to unsupervisedly design a large number of classification tasks to meta-train medical image classifiers. We evaluate our method on a breast dynamically contrast enhanced magnetic resonance imaging (DCE-MRI) data set that has been used to benchmark few-shot training methods of medical image classifiers. Our results show that the proposed unsupervised task design to meta-train medical image classifiers builds a pre-trained model that, after fine-tuning, produces better classification results than other unsupervised and supervised pre-training methods, and competitive results with respect to meta-training that relies on hand-designed classification tasks.
This paper addresses Wireless Networks for Metropolitan Transports (WNMT), a class of moving or vehicle-to-infrastructure networks that may be used by public transportation systems to provide broadband access to their vehicles, stops, and passengers. We propose the WiMetroNet, a WNMT that is auto-configurable and scalable. It is based on a new Ad hoc routing protocol, the Wireless Metropolitan Routing Protocol (WMRP), which, coupled with data plane optimizations, was designed to be scalable to thousands of nodes.
Noisy Labels are commonly present in data sets automatically collected from the internet, mislabeled by non-specialist annotators, or even specialists in a challenging task, such as in the medical field. Although deep learning models have shown significant improvements in different domains, an open issue is their ability to memorize noisy labels during training, reducing their generalization potential. As deep learning models depend on correctly labeled data sets and label correctness is difficult to guarantee, it is crucial to consider the presence of noisy labels for deep learning training. Several approaches have been proposed in the literature to improve the training of deep learning models in the presence of noisy labels. This paper presents a survey on the main techniques in literature, in which we classify the algorithm in the following groups: robust losses, sample weighting, sample selection, meta-learning, and combined approaches. We also present the commonly used experimental setup, data sets, and results of the state-of-the-art models.
Anomaly detection methods generally target the learning of a normal image distribution (i.e., inliers showing healthy cases) and during testing, samples relatively far from the learned distribution are classified as anomalies (i.e., outliers showing disease cases). These approaches tend to be sensitive to outliers that lie relatively close to inliers (e.g., a colonoscopy image with a small polyp). In this paper, we address the inappropriate sensitivity to outliers by also learning from inliers. We propose a new few-shot anomaly detection method based on an encoder trained to maximise the mutual information between feature embeddings and normal images, followed by a few-shot score inference network, trained with a large set of inliers and a substantially smaller set of outliers. We evaluate our proposed method on the clinical problem of detecting frames containing polyps from colonoscopy video sequences, where the training set has 13350 normal images (i.e., without polyps) and less than 100 abnormal images (i.e., with polyps). The results of our proposed model on this data set reveal a state-of-the-art detection result, while the performance based on different number of anomaly samples is relatively stable after approximately 40 abnormal training images. Code is available at https://github.com/tianyu0207/FSAD-Net .
The extraction of optimal features, in a classification sense, is still quite challenging in the context of large-scale classification problems (such as visual recognition), involving a large number of classes and significant amounts of training data per class. We present an optimal, in the minimum Bayes error sense, algorithm for feature design that combines the most appealing properties of the two strategies that are currently dominant:feature extraction (FE) and feature selection (FS). The new algorithm proceeds by interleaving pairs of FS and FE steps, which amount to a sequential search for the most discriminant directions in a collection of two dimensional subspaces. It combines the fast convergence rate of FS with the ability of FE to uncover optimal features that are not part of the original basis functions, leading to solutions that are better than those achievable by either FE or FS alone, in a small number of iterations. Because the basic iteration has very low complexity, the new algorithm is scalable in the number of classes of the recognition problem, a property that is currently only available for feature extraction methods that are either sub-optimal or optimal under restrictive assumptions that do not hold for generic recognition. Experimental results show significant improvements over these methods, either through much greater robustness to local minima or by achieving significantly faster convergence.
This paper addresses the semantic instance segmentation task in the open-set conditions, where input images can contain known and unknown object classes. The training process of existing semantic instance segmentation methods requires annotation masks for all object instances, which is expensive to acquire or even infeasible in some realistic scenarios, where the number of categories may increase boundlessly. In this paper, we present a novel open-set semantic instance segmentation approach capable of segmenting all known and unknown object classes in images, based on the output of an object detector trained on known object classes. We formulate the problem using a Bayesian framework, where the posterior distribution is approximated with a simulated annealing optimization equipped with an efficient image partition sampler. We show empirically that our method is competitive with state-of-the-art supervised methods on known classes, but also performs well on unknown classes when compared with unsupervised methods.
Artistic image understanding is an interdisciplinary research field of increasing importance for the computer vision and the art history communities. For computer vision scientists, this problem offers challenges where new techniques can be developed; and for the art history community new automatic art analysis tools can be developed. On the positive side, artistic images are generally constrained by compositional rules and artistic themes. However, the low-level texture and color features exploited for photographic image analysis are not as effective because of inconsistent color and texture patterns describing the visual classes in artistic images. In this work, we present a new database of monochromatic artistic images containing 988 images with a global semantic annotation, a local compositional annotation, and a pose annotation of human subjects and animal types. In total, 75 visual classes are annotated, from which 27 are related to the theme of the art image, and 48 are visual classes that can be localized in the image with bounding boxes. Out of these 48 classes, 40 have pose annotation, with 37 denoting human subjects and 3 representing animal types. We also provide a complete evaluation of several algorithms recently proposed for image annotation and retrieval. We then present an algorithm achieving remarkable performance over the most successful algorithm hitherto proposed for this problem. Our main goal with this paper is to make this database, the evaluation process, and the benchmark results available for the computer vision community.
We propose a new training algorithm, ScanMix, that explores semantic clustering and semi-supervised learning (SSL) to allow superior robustness to severe label noise and competitive robustness to non-severe label noise problems, in comparison to the state of the art (SOTA) methods. ScanMix is based on the expectation maximisation framework, where the E-step estimates the latent variable to cluster the training images based on their appearance and classification results, and the M-step optimises the SSL classification and learns effective feature representations via semantic clustering. We present a theoretical result that shows the correctness and convergence of ScanMix, and an empirical result that shows that ScanMix has SOTA results on CIFAR-10/-100 (with symmetric, asymmetric and semantic label noise), Red Mini-ImageNet (from the Controlled Noisy Web Labels), Clothing1M and WebVision. In all benchmarks with severe label noise, our results are competitive to the current SOTA.
The deployment of automated systems to diagnose diseases from medical images is challenged by the requirement to localise the diagnosed diseases to justify or explain the classification decision. This requirement is hard to fulfil because most of the training sets available to develop these systems only contain global annotations, making the localisation of diseases a weakly supervised approach. The main methods designed for weakly supervised disease classification and localisation rely on saliency or attention maps that are not specifically trained for localisation, or on region proposals that can not be refined to produce accurate detections. In this paper, we introduce a new model that combines region proposal and saliency detection to overcome both limitations for weakly supervised disease classification and localisation. Using the ChestX-ray14 data set, we show that our proposed model establishes the new state-of-the-art for weakly-supervised disease diagnosis and localisation. We make our code available at https://github.com/renato145/RpSalWeaklyDet.
Current deep image super-resolution (SR) approaches attempt to restore high-resolution images from down-sampled images or by assuming degradation from simple Gaussian kernels and additive noises. However, such simple image processing techniques represent crude approximations of the real-world procedure of lowering image resolution. In this paper, we propose a more realistic process to lower image resolution by introducing a new Kernel Adversarial Learning Super-resolution (KASR) framework to deal with the real-world image SR problem. In the proposed framework, degradation kernels and noises are adaptively modeled rather than explicitly specified. Moreover, we also propose an iterative supervision process and high-frequency selective objective to further boost the model SR reconstruction accuracy. Extensive experiments validate the effectiveness of the proposed framework on real-world datasets.
Unsupervised anomaly detection (UAD) aims to find anomalous images by optimising a detector using a training set that contains only normal images. UAD approaches can be based on reconstruction methods, self-supervised approaches, and Imagenet pre-trained models. Reconstruction methods, which detect anomalies from image reconstruction errors, are advantageous because they do not rely on the design of problem-specific pretext tasks needed by self-supervised approaches, and on the unreliable translation of models pre-trained from non-medical datasets. However, reconstruction methods may fail because they can have low reconstruction errors even for anomalous images. In this paper, we introduce a new reconstruction-based UAD approach that addresses this low-reconstruction error issue for anomalous images. Our UAD approach, the memory-augmented multi-level cross-attentional masked autoencoder (MemMC-MAE), is a transformer-based approach, consisting of a novel memory-augmented self-attention operator for the encoder and a new multi-level cross-attention operator for the decoder. MemMC-MAE masks large parts of the input image during its reconstruction, reducing the risk that it will produce low reconstruction errors because anomalies are likely to be masked and cannot be reconstructed. However, when the anomaly is not masked, then the normal patterns stored in the encoder’s memory combined with the decoder’s multi-level cross-attention will constrain the accurate reconstruction of the anomaly. We show that our method achieves SOTA anomaly detection and localisation on colonoscopy, pneumonia, and covid-19 chest x-ray datasets.
The design of optimal feature sets for visual classification problems is still one of the most challenging topics in the area of computer vision. In this work, we propose a new algorithm that computes optimal features, in the minimum Bayes error sense, for visual recognition tasks. The algorithm now proposed combines the fast convergence rate of feature selection (FS) procedures with the ability of feature extraction (FE) methods to uncover optimal features that are not part of the original basis function set. This leads to solutions that are better than those achievable by either FE or FS alone, in a small number of iterations, making the algorithm scalable in the number of classes of the recognition problem. This property is currently only available for feature extraction methods that are either sub-optimal or optimal under restrictive assumptions that do not hold for generic imagery. Experimental results show significant improvements over these methods, either through much greater robustness to local minima or by achieving significantly faster convergence. (C) 2006 Elsevier B.V. All rights reserved.
Developing meta-learning algorithms that are un-biased toward a subset of training tasks often requires hand-designed criteria to weight tasks, potentially resulting in sub-optimal solutions. In this paper, we introduce a new principled and fully-automated task-weighting algorithm for meta-learning methods. By considering the weights of tasks within the same mini-batch as an action, and the meta-parameter of interest as the system state, we cast the task-weighting meta-learning problem to a trajectory optimisation and employ the iterative linear quadratic regulator to determine the optimal action or weights of tasks. We theoretically show that the proposed algorithm converges to an $\epsilon_{0}$-stationary point, and empirically demonstrate that the proposed approach out-performs common hand-engineering weighting methods in two few-shot learning benchmarks.
We introduce a new model for semantic annotation and retrieval from image databases. The new model is based on a probabilistic formulation that poses annotation and retrieval as classification problems, and produces solutions that are optimal in the minimum probability of error sense. It is also database centric, by establishing a one-to-one mapping between semantic classes and the groups of database images that share the associated semantic labels. In this work we show that, under the database centric probabilistic model, optimal annotation and retrieval can be implemented with algorithms that are conceptually simple, computationally efficient, and do not require prior semantic segmentation of training images. Due to its simplicity, the annotation and retrieval architecture is also amenable to sophisticated parameter tuning, a property that is exploited to investigate the role of feature selection in the design of optimal annotation and retrieval systems. Finally, we demonstrate the benefits of simply establishing a one-to-one mapping between keywords and the states of the semantic classification problem over the more complex, and currently popular, joint modeling of keyword and visual feature distributions. The database centric probabilistic retrieval model is compared to existing semantic labeling and retrieval methods, and shown to achieve higher accuracy than the previously best published results, at a fraction of their computational cost.
We present a new statistical pattern recognition approach for the problem of left ventricle endocardium tracking in ultrasound data. The problem is formulated as a sequential importance resampling algorithm such that the expected segmentation of the current time step is estimated based on the appearance, shape, and motion models that take into account all previous and current images and previous segmentation contours produced by the method. The new appearance and shape models decouple the affine and nonrigid segmentations of the left ventricle to reduce the running time complexity. The proposed motion model combines the systole and diastole motion patterns and an observation distribution built by a deep neural network. The functionality of our approach is evaluated using a dataset of diseased cases containing 16 sequences and another dataset of normal cases comprised of four sequences, where both sets present long axis views of the left ventricle. Using a training set comprised of diseased and healthy cases, we show that our approach produces more accurate results than current state-of-the-art endocardium tracking methods in two test sequences from healthy subjects. Using three test sequences containing different types of cardiopathies, we show that our method correlates well with interuser statistics produced by four cardiologists.
Effective semi-supervised learning (SSL) in medical image analysis (MIA) must address two challenges: 1) work effectively on both multi-class (e.g., lesion classification) and multi-label (e.g., multiple-disease diagnosis) problems, and 2) handle unbalanced learning (because of the high variance in disease prevalence). One strategy to explore in SSL MIA is based on the pseudo labelling strategy, but it has a few shortcomings. Pseudo-labelling has in general lower accuracy than consistency learning, it is not specifically design for both multi-class and multi-label problems, and it can be challenged by imbalanced learning. In this paper; unlike traditional methods that select confident pseudo label by threshold, we propose a new SSL algorithm, called anti-curriculum pseudo-labelling (ACPL), which introduces novel techniques to select informative unlabelled samples, improving training balance and allowing the model to work for both multi-label and multi-class problems, and to estimate pseudo labels by an accurate ensemble of classifiers (improving pseudo label accuracy). We run extensive experiments to evaluate ACPL on two public medical image classification benchmarks: Chest X-Ray14 for thorax disease multi-label classification and ISIC2018 for skin lesion multi-class classification. Our method outperforms previous SOTA SSL methods on both datasets(12).
Statistical pattern recognition models are one of the core research topics in the segmentation of the left ventricle of the heart from ultrasound data. The underlying statistical model usually relies on a complex model for the shape and appearance of the left ventricle whose parameters can be learned using a manually segmented data set. Unfortunately, this complex requires a large number of parameters that can be robustly learned only if the training set is sufficiently large. The difficulty in obtaining large training sets is currently a major roadblock for the further exploration of statistical models in medical image analysis. In this paper, we present a novel semi-supervised self-training model that reduces the need of large training sets for estimating the parameters of statistical models. This model is initially trained with a small set of manually segmented images, and for each new test sequence, the system re-estimates the model parameters incrementally without any further manual intervention. We show that state-of-the-art segmentation results can be achieved with training sets containing 50 annotated examples.
Minimally invasive surgery (MIS) has many documented advantages, but the surgeon's limited visual contact with the scene can be problematic. Hence, systems that can help surgeons navigate, such as a method that can produce a 3D semantic map, can compensate for the limitation above. In theory, we can borrow 3D semantic mapping techniques developed for robotics, but this requires finding solutions to the following challenges in MIS: 1) semantic segmentation, 2) depth estimation, and 3) pose estimation. In this paper, we propose the first 3D semantic mapping system from knee arthroscopy that solves the three challenges above. Using out-of-distribution non-human datasets, where pose could be labeled, we jointly train depth+pose estimators using self-supervised and supervised losses. Using an in-distribution human knee dataset, we train a fully-supervised semantic segmentation system to label arthroscopic image pixels into femur, ACL, and meniscus. Taking testing images from human knees, we combine the results from these two systems to automatically create 3D semantic maps of the human knee. The result of this work opens the pathway to the generation of intra-operative 3D semantic mapping, registration with pre-operative data, and robotic-assisted arthroscopy. Source code: https://github.com/YJonmo/EndoMapNet.
Tissue typology annotation in Whole Slide histological images is a complex and tedious, yet necessary task for the development of computational pathology models. We propose to address this problem by applying Open Set Recognition techniques to the task of jointly classifying tissue that belongs to a set of annotated classes, e.g. clinically relevant tissue categories, while rejecting in test time Open Set samples, i.e. images that belong to categories not present in the training set. To this end, we introduce a new approach for Open Set histopathological image recognition based on training a model to accurately identify image categories and simultaneously predict which data augmentation transform has been applied. In test time, we measure model confidence in predicting this transform, which we expect to be lower for images in the Open Set. We carry out comprehensive experiments in the context of colorectal cancer assessment from histological images, which provide evidence on the strengths of our approach to automatically identify samples from unknown categories. Code is released at https://github.com/agaldran/t3po .
The analysis of images taken from cultural heritage artifacts is an emerging area of research in the field of information retrieval. Current methodologies are focused on the analysis of digital images of paintings for the tasks of forgery detection and style recognition. In this paper, we introduce a graph-based method for the automatic annotation and retrieval of digital images of art prints. Such method can help art historians analyze printed art works using an annotated database of digital images of art prints. The main challenge lies in the fact that art prints generally have limited visual information. The results show that our approach produces better results in a weakly annotated database of art prints in terms of annotation and retrieval performance compared to state-of-the-art approaches based on bag of visual words.
Recent evolution of intelligent vehicles features the large scale emergence and introduction of multimode mobile terminals, with the capability to simultaneously or sequentially attach to conceptually different access networks. To cope with this, they require some abstracted framework for their management and control, especially in the case of intelligent selection between the various heterogeneous accesses. A solution to this issue, the Media Independent Handover Services, is being standardized as the IEEE 802.21. This draft standard has been studied and applied to the case of a B3G operator networks as a research topic in the EU-funded project Daidalos in order to enable seamless operation between heterogeneous technologies. This paper focuses on the seamless integration of broadcast technologies, in particular UMTS/MBMS networks, in the case when emergency information has to be delivered to a large number of mobile users. It describes an extension to the 802.21 framework which enables the dynamic selection and allocation of multicast resources and thus the efficient usage of the radio resources. The UNITS access is developed in the experimental software radio platform of EURECOM, and provides a direct inter-connection with an IPv6 Core Network, very close to the current LTE direction of the 3GPP. We describe here the additional Media Independent Handover primitives and how they are mapped to trigger the control of multicast (MBMS) resources in the UNITS network. This paper is introduced by the account of an on-the-road emergency situation, written as a showcase of the work performed in the EU FP6 research project DAIDALOS.
We present a novel method for the automatic deflection and segmentation of (sub-)cortical gray matter structures in 3-D magnetic resonance images of the human brain. Essentially, the method is a top-down segmentation approach based on the recently introduced concept Of Marginal Space Learning (MSL). We show that MSL naturally decomposes the parameter space of anatomy shapes along decreasing levels of geometrical abstraction into subspaces of increasing dimensionality by exploiting parameter invariance. At each level of: abstraction, i.e., in each subspace, we build strong discriminative models from annotated training data, and use these models to narrow the range of possible solutions until a final shape can be inferred. Contextual information is introduced into the system by representing candidate shape parameters with high-dimensional vectors of 3-D generalized Haar features and steer-able features derived from the observed volume intensities. Cur system allows us to detect and segment 8 (sub-)cortical gray matter Structures in T1-weighted 3-D MR brain scans from a variety of different scanners in on average 13.9 sec., which is faster than most of the approaches in the literature. In order to ensure comparability of the achieved results and to validate robustness, we evaluate our method on two publicly available gold standard databases consisting of several TA-weighted 3-D brain AIR scans from different; scanners and sites. The proposed method achieves an accuracy better than most state-of-the-art approaches using standardized distance and overlap metrics.
Endometriosis is a common chronic gynecological disorder that has many characteristics, including the pouch of Douglas (POD) obliteration, which can be diagnosed using Transvaginal gynecological ultrasound (TVUS) scans and magnetic resonance imaging (MRI). TVUS and MRI are complementary non-invasive endometriosis diagnosis imaging techniques, but patients are usually not scanned using both modalities and, it is generally more challenging to detect POD obliteration from MRI than TVUS. To mitigate this classification imbalance, we propose in this paper a knowledge distillation training algorithm to improve the POD obliteration detection from MRI by leveraging the detection results from unpaired TVUS data. More specifically, our algorithm pre-trains a teacher model to detect POD obliteration from TVUS data, and it also pre-trains a student model with 3D masked auto-encoder using a large amount of unlabelled pelvic 3D MRI volumes. Next, we distill the knowledge from the teacher TVUS POD obliteration detector to train the student MRI model by minimizing a regression loss that approximates the output of the student to the teacher using unpaired TVUS and MRI data. Experimental results on our endometriosis dataset containing TVUS and MRI data demonstrate the effectiveness of our method to improve the POD detection accuracy from MRI.
We propose a new methodology for creating 3-D models for computer graphics applications from 2-D concept sketches of human characters using minimal user interaction. This methodology will facilitate the fast production of high quality 3-D models by non-expert users involved in the development process of video games and movies. The workflow starts with an image containing the sketch of the human character from a single viewpoint, in which a 2-D body pose detector is run to infer the positions of the skeleton joints of the character. Then the 3-D body pose and camera motion are estimated from the 2-D body pose detected from above, where we take a recently proposed methodology that works with real humans and adapt it to work with concept sketches of human characters. The final step of our methodology consists of an optimization process based on a sampling importance re-sampling method that takes as input the estimated 3-D body pose and camera motion and builds a 3-D mesh of the body shape, which is then matched to the concept sketch image. Our main contributions are: 1) a novel adaptation of the 3-D from 2-D body pose estimation methods to work with sketches of humans that have non-standard body part proportions and constrained camera motion; and 2) a new optimization (that estimates a 3-D body mesh using an underlying low-dimensional linear model of human shape) guided by the quality of the matching between the 3-D mesh of the body shape and the concept sketch. We show qualitative results based on seven 3-D models inferred from 2-D concept sketches, and also quantitative results, where we take seven different 3-D meshes to generate concept sketches, and use our method to infer the 3-D model from these sketches, which allows us to measure the average Euclidean distance between the original and estimated 3-D models. Both qualitative and quantitative results show that our model has potential in the fast production of 3-D models from concept sketches.
We introduce a new methodology for the problem of artistic image analysis, which among other tasks, involves the automatic identification of visual classes present in an art work. In this paper, we advocate the idea that artistic image analysis must explore a graph that captures the network of artistic influences by computing the similarities in terms of appearance and manual annotation. One of the novelties of our methodology is the proposed formulation that is a principled way of combining these two similarities in a single graph. Using this graph, we show that an efficient random walk algorithm based on an inverted label propagation formulation produces more accurate annotation and retrieval results compared with the following baseline algorithms: bag of visual words, label propagation, matrix completion, and structural learning. We also show that the proposed approach leads to a more efficient inference and training procedures. This experiment is run on a database containing 988 artistic images (with 49 visual classification problems divided into a multiclass problem with 27 classes and 48 binary problems), where we show the inference and training running times, and quantitative comparisons with respect to several retrieval and annotation performance measures.
This paper focuses on one of the most fascinating and successful, but challenging generative models in the literature: the Generative Adversarial Networks (GAN). Recently, GAN has attracted much attention by the scientific community and the entertainment industry due to its effectiveness in generating complex and high-dimension data, which makes it a superior model for producing new samples, compared with other types of generative models. The traditional GAN (referred to as the Vanilla GAN) is composed of two neural networks, a generator and a discriminator, which are modeled using a minimax optimization. The generator creates samples to fool the discriminator that in turn tries to distinguish between the original and created samples. This optimization aims to train a model that can generate samples from the training set distribution. In addition to defining and explaining the Vanilla GAN and its main variations (e.g., DCGAN, WGAN, and SAGAN), this paper will present several applications that make GAN an extremely exciting method for the entertainment industry (e.g., style-transfer and image-to-image translation). Finally, the following measures to assess the quality of generated images are presented: Inception Search (IS), and Frechet Inception Distance (FID).
Generalised zero-shot learning (GZSL) methods aim to classify previously seen and unseen visual classes by leveraging the semantic information of those classes. In the context of GZSL, semantic information is non-visual data such as a text description of the seen and unseen classes. Previous GZSL methods have explored transformations between visual and semantic spaces, as well as the learning of a latent joint visual and semantic space. In these methods, even though learning has explored a combination of spaces (i.e., visual, semantic or joint latent space), inference tended to focus on using just one of the spaces. By hypothesising that inference must explore all three spaces, we propose a new GZSL method based on a multi-modal classification over visual, semantic and joint latent spaces. Another issue affecting current GZSL methods is the intrinsic bias toward the classification of seen classes - a problem that is usually mitigated by a domain classifier which modulates seen and unseen classification. Our proposed approach replaces the modulated classification by a computationally simpler multi-domain classification based on averaging the multi-modal calibrated classifiers from the seen and unseen domains. Experiments on GZSL benchmarks show that our proposed GZSL approach achieves competitive results compared with the state-of-the-art.
We present a detection model that is capable of accelerating the inference time of lesion detection from breast dynamically contrast-enhanced magnetic resonance images (DCE-MRI) at state-of-the-art accuracy. In contrast to previous methods based on computationally expensive exhaustive search strategies, our method reduces the inference time with a search approach that gradually focuses on lesions by progressively transforming a bounding volume until the lesion is detected. Such detection model is trained with reinforcement learning and is modeled by a deep Q-network (DQN) that iteratively outputs the next transformation to the current bounding volume. We evaluate our proposed approach in a breast MRI data set containing the T1-weighted and the first DCE-MRI subtraction volume from 117 patients and a total of 142 lesions. Results show that our proposed reinforcement learning based detection model reaches a true positive rate (TPR) of 0.8 at around three false positive detections and a speedup of at least 1.78 times compared to baselines methods.
Intelligent learning environments based on interactions within the digital world are increasingly popular as they provide mechanisms for interactive and adaptive learning, but learners find it difficult to transfer this to real world tasks. We present the initial development stages of CRISTAL, an intelligent simulator targeted at trainee radiologists which enhances the learning experience by enabling the virtual environment to adapt according to their real world experiences. Our system design has been influenced by feedback from trainees, and allows them to practice their reporting skills by writing freeform reports in natural language. This has the potential to be expanded to other areas such as short-form journalism and legal document drafting.
Ultrasound guidance is not in widespread use in prostate cancer radiotherapy workflows. This can be partially attributed to the need for image interpretation by a trained operator during ultrasound image acquisition. In this work, a one-class regressor, based on DenseNet and Gaussian processes, was implemented to automatically assess the quality of transperineal ultrasound images of the male pelvic region. The implemented deep learning approach was tested on 300 transperineal ultrasound images and it achieved a scoring accuracy of 94%, a specificity of 95% and a sensitivity of 92% with respect to the majority vote of 3 experts, which was comparable with the results of these experts. This is the first step toward a fully automatic workflow, which could potentially remove the need for ultrasound image interpretation and make real-time volumetric organ tracking in the radio- therapy environment using ultrasound more appealing. (C) 2019 World Federation for Ultrasound in Medicine & Biology. Published by Elsevier Inc. All rights reserved.
Many state-of-the-art noisy-label learning methods rely on learning mechanisms that estimate the samples' clean labels during training and discard their original noisy labels. However, this approach prevents the learning of the relationship between images, noisy labels and clean labels, which has been shown to be useful when dealing with instance-dependent label noise problems. Furthermore, methods that do aim to learn this relationship require cleanly annotated subsets of data, as well as distillation or multi-faceted models for training. In this paper, we propose a new training algorithm that relies on a simple model to learn the relationship between clean and noisy labels without the need for a cleanly labelled subset of data. Our algorithm follows a 3-stage process, namely: 1) self-supervised pre-training followed by an early-stopping training of the classifier to confidently predict clean labels for a subset of the training set; 2) use the clean set from stage (1) to bootstrap the relationship between images, noisy labels and clean labels, which we exploit for effective relabelling of the remaining training set using semi-supervised learning; and 3) supervised training of the classifier with all relabelled samples from stage (2). By learning this relationship, we achieve state-of-the-art performance in asymmetric and instance-dependent label noise problems.
In this paper we describe an algorithm for accurately segmenting the individual cytoplasm and nuclei from a clump of overlapping cervical cells. Current methods cannot undertake such a complete segmentation due to the challenges involved in delineating cells with severe overlap and poor contrast. Our approach initially performs a scene segmentation to highlight both free-lying cells, cell clumps and their nuclei. Then cell segmentation is performed using a joint level set optimization on all detected nuclei and cytoplasm pairs. This optimisation is constrained by the length and area of each cell, a prior on cell shape, the amount of cell overlap and the expected gray values within the overlapping regions. We present quantitative nuclei detection and cell segmentation results on a database of synthetically overlapped cell images constructed from real images of free-lying cervical cells. We also perform a qualitative assessment of complete fields of view containing multiple cells and cell clumps.
Purpose: Accurate clinical diagnosis of lymph node metastases is of paramount importance in the treatment of patients with abdominopelvic malignancy. This review assesses the diagnostic performance of deep learning algorithms and radiomics models for lymph node metastases in abdominopelvic malignancies. Methodology: Embase (PubMed, MEDLINE), Science Direct and IEEE Xplore databases were searched to identify eligible studies published between January 2009 and March 2019. Studies that reported on the accuracy of deep learning algorithms or radiomics models for abdominopelvic malignancy by CT or MRI were selected. Study characteristics and diagnostic measures were extracted. Estimates were pooled using random-effects meta analysis. Evaluation of risk of bias was performed using the QUADAS-2 tool. Results: In total, 498 potentially eligible studies were identified, of which 21 were included and 17 offered enough information for a quantitative analysis. Studies were heterogeneous and substantial risk of bias was found in 18 studies. Almost all studies employed radiomics models (n = 20). The single published deep-learning model out-performed radiomics models with a higher AUROC (0.912 vs 0.895), but both radiomics and deep learning models outperformed the radiologist's interpretation in isolation (0.774). Pooled results for radiomics nomograms amongst tumour subtypes demonstrated the highest AUC 0.895 (95 %CI, 0.810-0.980) for urological malignancy, and the lowest AUC 0.798 (95 %CI, 0.744-0.852) for colorectal malignancy. Conclusion: Radiomics models improve the diagnostic accuracy of lymph node staging for abdominopelvic malignancies in comparison with radiologist's assessment. Deep learning models may further improve on this, but data remain limited.
We present new achievements on the use of deep convolutional neural networks (CNN) in the problem of pedestrian detection (PD). In this paper, we aim to address the following questions: (i) Given non-deep state-of-the-art pedestrian detectors (e.g. ACF, LDCF), is it possible to improve their top performances?; (ii) is it possible to apply a pre-trained deep model to these detectors to boost their performances in the PD context? In this paper, we address the aforementioned questions by cascading CNN models (pre-trained on Imagenet) with state-of-the-art non-deep pedestrian detectors. Furthermore, we also show that this strategy is extensible to different segmentation maps (e.g. RGB, gradient, LUV) computed from the same pedestrian bounding box (i.e. the proposal). We demonstrate that the proposed approach is able to boost the detection performance of state-of-the-art non-deep pedestrian detectors. We apply the proposed methodology to address the pedestrian detection problem on the publicly available datasets INRIA and Caltech. (C) 2016 Elsevier Ltd. All rights reserved.
Multi-modal learning focuses on training models by equally combining multiple input data modalities during the prediction process. However, this equal combination can be detrimental to the prediction accuracy because different modalities are usually accompanied by varying levels of uncertainty. Using such uncertainty to combine modalities has been studied by a couple of approaches, but with limited success because these approaches are either designed to deal with specific classification or segmentation problems and cannot be easily translated into other tasks, or suffer from numerical instabilities. In this paper, we propose a new Uncertainty-awareMulti-modal Learner that estimates uncertainty by measuring feature density via Cross-modal Random Network Prediction (CRNP). CRNP is designed to require little adaptation to translate between different prediction tasks, while having a stable training process. From a technical point of view, CRNP is the first approach to explore random network prediction to estimate uncertainty and to combine multi-modal data. Experiments on two 3D multi-modal medical image segmentation tasks and three 2D multi-modal computer vision classification tasks show the effectiveness, adaptability and robustness of CRNP. Also, we provide an extensive discussion on different fusion functions and visualization to validate the proposed model.
It is challenging to map the spatial distribution of natural and planted forests based on satellite images because of the high correlation among them. This investigation aims to increase accuracies in classifications of natural forests and eucalyptus plantations by combining remote sensing data from multiple sources. We defined four vegetation classes: natural forest (NF), planted eucalyptus forest (PF), agriculture (A) and pasture (P), and sampled 410,251 pixels from 100 polygons of each class. Classification experiments were performed by using a random forest algorithm with images from Landsat-8, Sentinel-1, and SRTM. We considered four texture features (energy, contrast, correlation, and entropy) and NDVI. We used F1-score, overall accuracy and total disagreement metrics, to assess the classification performance, and Jeffries–Matusita (JM) distance to measure the spectral separability. Overall accuracy for Landsat-8 bands alone was 88.29%. A combination of Landsat-8 with Sentinel-1 bands resulted in a 3% overall accuracy increase and this band combination also improved the F1-score of NF, PF, P and A in 2.22%, 2.9%, 3.71%, and 8.01%, respectively. The total disagreement decreased from 11.71% to 8.71%. The increase in the statistical separability corroborates such improvement and is mainly observed between NF-PF (11.98%) and A-P (45.12%). We conclude that combining optical and radar remote sensing data increased the classification accuracy of natural and planted forests and may serve as a basis for large-scale semi-automatic mapping of forest resources.
Abstract With the advent of increased diversity and scale of molecular data, there has been a growing appreciation for the applications of machine learning and statistical methodologies to gain new biological insights. An important step in achieving this aim is the Relation Extraction process which specifies if an interaction exists between two or more biological entities in a published study. Here, we employed natural-language processing (CBOW) and deep Recurrent Neural Network (bi-directional LSTM) to predict relations between biological entities that describe protein subcellular localisation in plants. We applied our system to 1700 published Arabidopsis protein subcellular studies from the SUBA manually curated dataset. The system was able to extract relevant text and the classifier predicted interactions between protein name, subcellular localisation and experimental methodology. It obtained a final precision, recall rate, accuracy and F1 scores of 0.951, 0.828, 0.893 and 0.884 respectively. The classifier was subsequently tested on a similar problem in crop species (CropPAL) and demonstrated a comparable accuracy measure (0.897). Consequently, our approach can be used to extract protein functional features from unstructured text in the literature with high accuracy. The developed system will improve dissemination or protein functional data to the scientific community and unlock the potential of big data text analytics for generating new hypotheses from diverse datasets. Competing Interest Statement The authors have declared no competing interest.
Semantic segmentation models classify pixels into a set of known (``in-distribution'') visual classes. When deployed in an open world, the reliability of these models depends on their ability not only to classify in-distribution pixels but also to detect out-of-distribution (OoD) pixels. Historically, the poor OoD detection performance of these models has motivated the design of methods based on model re-training using synthetic training images that include OoD visual objects. Although successful, these re-trained methods have two issues: 1) their in-distribution segmentation accuracy may drop during re-training, and 2) their OoD detection accuracy does not generalise well to new contexts (e.g., country surroundings) outside the training set (e.g., city surroundings). In this paper, we mitigate these issues with: (i) a new residual pattern learning (RPL) module that assists the segmentation model to detect OoD pixels without affecting the inlier segmentation performance; and (ii) a novel context-robust contrastive learning (CoroCL) that enforces RPL to robustly detect OoD pixels among various contexts. Our approach improves by around 10\% FPR and 7\% AuPRC the previous state-of-the-art in Fishyscapes, Segment-Me-If-You-Can, and RoadAnomaly datasets. Our code is available at: https://github.com/yyliu01/RPL.
The automatic detection of frames containing polyps from a colonoscopy video sequence is an important first step for a fully automated colonoscopy analysis tool. Typically, such detection system is built using a large annotated data set of frames with and without polyps, which is expensive to be obtained. In this paper, we introduce a new system that detects frames containing polyps as anomalies from a distribution of frames from exams that do not contain any polyps. The system is trained using a one-class training set consisting of colonoscopy frames without polyps - such training set is considerably less expensive to obtain, compared to the 2-class data set mentioned above. During inference, the system is only able to reconstruct frames without polyps, and when it tries to reconstruct a frame with polyp, it automatically removes (i.e., photoshop) it from the frame - the difference between the input and reconstructed frames is used to detect frames with polyps. We name our proposed model as anomaly detection generative adversarial network (ADGAN), comprising a dual GAN with two generators and two discriminators. To test our framework, we use a new colonoscopy data set with 14317 images, split as a training set with 13350 images without polyps, and a testing set with 290 abnormal images containing polyps and 677 normal images without polyps. We show that our proposed approach achieves the state-of-the-art result on this data set, compared with recently proposed anomaly detection systems.
In the networking research and development field, one recurring problem faced is the duplication of effort to write first simulation and then implementation code. We posit an alternative development process that takes advantage of the built in network emulation features of Network Simulator 3 (ns-3) and allows developers to share most code between simulation and implementation of a protocol. Tests show that ns-3 can handle a data plane processing large packets, but has difficulties with small packets. When using ns-3 for implementing the control plane of a protocol, we found that ns-3 can even outperform a dedicated implementation. (C) 2011 Elsevier B. V. All rights reserved.
Next Generation Networks (NGN) will be based upon the "all IP" paradigm. The IP protocol will glue multiple technologies, for both access and core networks, in a common and scalable framework that will provide seamless communication mobility not only across those technologies, but also across different network operators. In this article we describe a framework for QoS support in such NGNs, where multi-interface terminals are given end-to-end QoS guarantees regardless of their point of attachment. The framework supports media independent handovers, triggered either by the user or by the network, to optimize network resources' distribution. The framework integrates layer two and layer three handovers by exploiting minimal additions to existing IETF and IEEE standards.
Standard two-view epipolar geometry assumes that images are taken using pinhole cameras. Real cameras, however, approximate ideal pinhole cameras using lenses and apertures. This leads to radial distortion effects in images that are not characterisable by the standard epipolar geometry model. The existence of radial distortion severely impacts the efficacy of point correspondence validation based on the epipolar constraint. Many previous works deal with radial distortion by augment- ing the epipolar geometry model (with additional parameters such as distortion coefficients and centre of distortion) to enable the modelling of radial distortion effects. Indirectly, this assumes that an accurate model of the radial distortion is known. In this paper, we take a different approach: we view radial distortion as a violation to the basic epipolar geometry equation. Instead of striving to model radial distortion, we adjust the epipolar geometry to account for the distortion effects. This adjustment is performed via moving least squares (MLS) surface approxi- mation, which we extend to allow for projective estimation. We also combine M-estimators with MLS to allow robust matching of interest points under severe radial distortion. Compared to previous works, our method is much simpler and involves just solving linear subproblems. It also exhibits a higher tolerance in cases where the exact model of radial distortion is unknown.
Recently, we have observed the traditional feature representations are being rapidly replaced by the deep learning representations, which produce significantly more accurate classification results when used together with the linear classifiers. However, it is widely known that non-linear classifiers can generally provide more accurate classification but at a higher computational cost involved in their training and testing procedures. In this paper, we propose a new efficient and accurate non-linear hierarchical classification method that uses the aforementioned deep learning representations. In essence, our classifier is based on a binary tree, where each node is represented by a linear classifier trained using a loss function that minimizes the classification error in a non-greedy way, in addition to postponing hard classification problems to further down the tree. In comparison with linear classifiers, our training process increases only marginally the training and testing time complexities, while showing competitive classification accuracy results. In addition, our method is shown to generalize better than shallow non-linear classifiers. Empirical validation shows that the proposed classifier produces more accurate classification results when compared to several linear and non-linear classifiers on Pascal VOC07 database.
Background Proximal femoral fractures are an important clinical and public health issue associated with substantial morbidity and early mortality. Artificial intelligence might offer improved diagnostic accuracy for these fractures, but typical approaches to testing of artificial intelligence models can underestimate the risks of artificial intelligence-based diagnostic systems. Methods We present a preclinical evaluation of a deep learning model intended to detect proximal femoral fractures in frontal x-ray films in emergency department patients, trained on films from the Royal Adelaide Hospital (Adelaide, SA, Australia). This evaluation included a reader study comparing the performance of the model against five radiologists (three musculoskeletal specialists and two general radiologists) on a dataset of 200 fracture cases and 200 non-fractures (also from the Royal Adelaide Hospital), an external validation study using a dataset obtained from Stanford University Medical Center, CA, USA, and an algorithmic audit to detect any unusual or unexpected model behaviour. Findings In the reader study, the area under the receiver operating characteristic curve (AUC) for the performance of the deep learning model was 0.994 (95% CI 0.988-0.999) compared with an AUC of 0.969 (0.960-0.978) for the five radiologists. This strong model performance was maintained on external validation, with an AUC of 0.980 (0.931-1.000). However, the preclinical evaluation identified barriers to safe deployment, including a substantial shift in the model operating point on external validation and an increased error rate on cases with abnormal bones (eg, Paget's disease). Interpretation The model outperfonned the radiologists tested and maintained performance on external validation, but showed several unexpected limitations during further testing. Thorough preclinical evaluation of artificial intelligence models, including algorithmic auditing, can reveal unexpected and potentially harmful behaviour even in high-performance artificial intelligence systems, which can inform future clinical testing and deployment decisions.
We propose a novel method for the automatic detection and measurement of fetal anatomical structures in ultrasound images. This problem offers a myriad of challenges, including: difficulty of modeling the appearance variations of the visual object of interest, robustness to speckle noise and signal dropout, and large search space of the detection procedure. Previous solutions typically rely on the explicit encoding of prior knowledge and formulation of the problem its it perceptual grouping task solved through clustering or variational approaches. These methods are constrained by the validity of the underlying assumptions and usually are not enough to capture the complex appearances of fetal anatomies. We propose a novel system for fast automatic detection and measurement of fetal anatomics that directly exploits a large database of expert annotated fetal anatomical structures in ultrasound iniages. Our method learns automatically to distinguish between the appearance of the object of interest and background by training a constrained probabilistic boosting tree classifier. This system is able to produce the automatic segmentation of several fetal anatomies using the same basic detection algorithm. We show results on fully automatic measurement of biparietal diameter (BPD), head circumference (HC), abdominal circumference (AC), Femur length (FL), humerus length (HL), and crown rump length (CRL). Notice that our approach is the first in the literature to deal with the HL and CRI, measurements. Extensive experiments (with clinical validation) show that our system is, on average, close to the accuracy of experts in terms of segmentation and obstetric measurements. Finally, this system runs under half second on it standard dual-core PC computer.
Local image features have been designed to be informative and repeatable under rigid transformations and illumination deformations. Even though current state-of-the-art local image features present a high degree of repeatability, their local appearance alone usually does not bring enough discriminative power to support a reliable matching, resulting in a relatively high number of mismatches in the correspondence set formed during the data association procedure. As a result, geometric filters, commonly based on global spatial configuration, have been used to reduce this number of mismatches. However, this approach presents a trade off between the effectiveness to reject mismatches and the robustness to non-rigid deformations. In this paper, we propose two geometric filters, based on semilocal spatial configuration of local features, that are designed to be robust to non-rigid deformations and to rigid transformations, without compromising its efficacy to reject mismatches. We compare our methods to the Hough transform, which is an efficient and effective mismatch rejection step based on global spatial configuration of features. In these comparisons, our methods are shown to be more effective in the task of rejecting mismatches for rigid transformations and non-rigid deformations at comparable time complexity figures. Finally, we demonstrate how to integrate these methods in a probabilistic recognition system such that the final verification step uses not only the similarity between features, but also their semi-local configuration.
Noisy labels are unavoidable yet troublesome in the ecosystem of deep learning because models can easily overfit them. There are many types of label noise, such as symmetric, asymmetric and instance-dependent noise (IDN), with IDN being the only type that depends on image information. Such dependence on image information makes IDN a critical type of label noise to study, given that labelling mistakes are caused in large part by insufficient or ambiguous information about the visual classes present in images. Aiming to provide an effective technique to address IDN, we present a new graphical modelling approach called InstanceGM, that combines discriminative and generative models. The main contributions of InstanceGM are: i) the use of the continuous Bernoulli distribution to train the generative model, offering significant training advantages, and ii) the exploration of a state-of-the-art noisy-label discriminative classifier to generate clean labels from instance-dependent noisy-label samples. InstanceGM is competitive with current noisy-label learning approaches, particularly in IDN benchmarks using synthetic and real-world datasets, where our method shows better accuracy than the competitors in most experiments.
In this paper, we present a novel method for the segmentation of breast masses from mammograms exploring structured and deep learning. Specifically, using structured support vector machine (SSVM), we formulate a model that combines different types of potential functions, including one that classifies image regions using deep learning. Our main goal with this work is to show the accuracy and efficiency improvements that these relatively new techniques can provide for the segmentation of breast masses from mammograms. We also propose an easily reproducible quantitative analysis to assess the performance of breast mass segmentation methodologies based on widely accepted accuracy and running time measurements on public datasets, which will facilitate further comparisons for this segmentation problem. In particular, we use two publicly available datasets (DDSM-BCRP and INbreast) and propose the computation of the running time taken for the methodology to produce a mass segmentation given an input image and the use of the Dice index to quantitatively measure the segmentation accuracy. For both databases, we show that our proposed methodology produces competitive results in terms of accuracy and running time.
The efficacy of cancer treatments (e.g., radiotherapy, chemotherapy, etc.) has been observed to critically depend on the proportion of hypoxic regions (i.e., a region deprived of adequate oxygen supply) in tumor tissue, so it is important to estimate this proportion from histological samples. Medical imaging data can be used to classify tumor tissue regions into necrotic or vital and then the vital tissue into normoxia (i.e., a region receiving a normal level of oxygen), chronic or acute hypoxia. Currently, this classification is a lengthy manual process performed using (immuno-)fluorescence (IF) and hematoxylin and eosin (HE) stained images of a histological specimen, which requires an expertise that is not widespread in clinical practice. In this paper, we propose a fully automated way to detect and classify tumor tissue regions into necrosis, normoxia, chronic hypoxia and acute hypoxia using IF and HE images from the same histological specimen. Instead of relying on any single classification methodology, we propose a principled combination of the following current state-of-the-art classifiers in the field: Adaboost, support vector machine, random forest and convolutional neural networks. Results show that on average we can successfully detect and classify more than 87% of the tumor tissue regions correctly. This automated system for estimating the proportion of chronic and acute hypoxia could provide clinicians with valuable information on assessing the efficacy of cancer treatments.
Current polyp detection methods from colonoscopy videos use exclusively normal (i.e., healthy) training images, which i) ignore the importance of temporal information in consecutive video frames, and ii) lack knowledge about the polyps. Consequently, they often have high detection errors, especially on challenging polyp cases (e.g., small, flat, or partially visible polyps). In this work, we formulate polyp detection as a weakly-supervised anomaly detection task that uses video-level labelled training data to detect frame-level polyps. In particular, we propose a novel convolutional transformer-based multiple instance learning method designed to identify abnormal frames (i.e., frames with polyps) from anomalous videos (i.e., videos containing at least one frame with polyp). In our method, local and global temporal dependencies are seamlessly captured while we simultaneously optimise video and snippet-level anomaly scores. A contrastive snippet mining method is also proposed to enable an effective modelling of the challenging polyp cases. The resulting method achieves a detection accuracy that is substantially better than current state-of-the-art approaches on a new large-scale colonoscopy video dataset introduced in this work.
We propose a new combination of deep belief networks and sparse manifold learning strategies for the 2D segmentation of non-rigid visual objects. With this novel combination, we aim to reduce the training and inference complexities while maintaining the accuracy of machine learning-based non-rigid segmentation methodologies. Typical non-rigid object segmentation methodologies divide the problem into a rigid detection followed by a non-rigid segmentation, where the low dimensionality of the rigid detection allows for a robust training (i.e., a training that does not require a vast amount of annotated images to estimate robust appearance and shape models) and a fast search process during inference. Therefore, it is desirable that the dimensionality of this rigid transformation space is as small as possible in order to enhance the advantages brought by the aforementioned division of the problem. In this paper, we propose the use of sparse manifolds to reduce the dimensionality of the rigid detection space. Furthermore, we propose the use of deep belief networks to allow for a training process that can produce robust appearance models without the need of large annotated training sets. We test our approach in the segmentation of the left ventricle of the heart from ultrasound images and lips from frontal face images. Our experiments show that the use of sparse manifolds and deep belief networks for the rigid detection stage leads to segmentation results that are as accurate as the current state of the art, but with lower search complexity and training processes that require a small amount of annotated training data.
The use of statistical pattern recognition models to segment the left ventricle of the heart in ultrasound images has gained substantial attention over the last few years. The main obstacle for the wider exploration of this methodology lies in the need for large annotated training sets, which are used for the estimation of the statistical model parameters. In this paper, we present a new on-line co-training methodology that reduces the need for large training sets for such parameter estimation. Our approach learns the initial parameters of two different models using a small manually annotated training set. Then, given each frame of a test sequence, the methodology not only produces the segmentation of the current frame, but it also uses the results of both classifiers to re-train each other incrementally. This on-line aspect of our approach has the advantages of producing segmentation results and re-training the classifiers on the fly as frames of a test sequence are presented, but it introduces a harder learning setting compared to the usual off-line co-training, where the algorithm has access to the whole set of un-annotated training samples from the beginning. Moreover, we introduce the use of the following new types of classifiers in the co-training framework: deep belief network and multiple model probabilistic data association. We show that our method leads to a fully automatic left ventricle segmentation system that achieves state-of-the-art accuracy on a public database with training sets containing at least twenty annotated images.
Objectives: Pouch of Douglas (POD) obliteration is a severe consequence of inflammation in the pelvis, often seen in patients with endometriosis. The sliding sign is a dynamic transvaginal ultrasound (TVS) test that can diagnose POD obliteration. We aimed to develop a deep learning (DL) model to automatically classify the state of the POD using recorded videos depicting the sliding sign test. Methods: Two expert sonologists performed, interpreted, and recorded videos of consecutive patients from September 2018 to April 2020. The sliding sign was classified as positive (i.e. normal) or negative (i.e. abnormal; POD obliteration). A DL model based on a temporal residual network was prospectively trained with a dataset of TVS videos. The model was tested on an independent test set and its diagnostic accuracy including area under the receiver operating characteristic curve (AUC), accuracy, sensitivity, specificity, and positive and negative predictive value (PPV/NPV) was compared to the reference standard sonologist classification (positive or negative sliding sign). Results: In a dataset consisting of 749 videos, a positive sliding sign was depicted in 646 (86.2%) videos, whereas 103 (13.8%) videos depicted a negative sliding sign. The dataset was split into training (414 videos), validation (139), and testing (196) maintaining similar positive/negative proportions. When applied to the test dataset using a threshold of 0.9, the model achieved: AUC 96.5% (95% CI: 90.8-100.0%), an accuracy of 88.8% (95% CI: 83.5-92.8%), sensitivity of 88.6% (95% CI: 83.0-92.9%), specificity of 90.0% (95% CI: 68.3-98.8%), a PPV of 98.7% (95% CI: 95.4-99.7%), and an NPV of 47.7% (95% CI: 36.8-58.2%). Conclusions: We have developed an accurate DL model for the prediction of the TVS-based sliding sign classification. Lay summary Endometriosis is a disease that affects females. It can cause very severe scarring inside the body, especially in the pelvis called the pouch of Douglas (POD). An ultrasound test called the 'sliding sign' can diagnose POD scarring. In our study, we provided input to a computer on how to interpret the sliding sign and determine whether there was POD scarring or not. This is a type of artificial intelligence called deep learning (DL). For this purpose, two expert ultrasound specialists recorded 749 videos of the sliding sign. Most of them (646) were normal and 103 showed POD scarring. In order for the computer to interpret, both normal and abnormal videos were required. After providing the necessary inputs to the computer, the DL model was very accurate (almost nine out of every ten videos was correctly determined by the DL model). In conclusion, we have developed an artificial intelligence that can interpret ultrasound videos of the sliding sign that show POD scarring that is almost as accurate as the ultrasound specialists. We believe this could help increase the knowledge on POD scarring in people with endometriosis.
Learning with noisy-labels has become an important research topic in computer vision where state-of-the-art (SOTA) methods explore: 1) prediction disagreement with co-teaching strategy that updates two models when they disagree on the prediction of training samples; and 2) sample selection to divide the training set into clean and noisy sets based on small training loss. However, the quick convergence of co-teaching models to select the same clean subsets combined with relatively fast overfitting of noisy labels may induce the wrong selection of noisy label samples as clean, leading to an inevitable confirmation bias that damages accuracy. In this paper, we introduce our noisy-label learning approach, called Asymmetric Co-teaching (AsyCo), which introduces novel prediction disagreement that produces more consistent divergent results of the co-teaching models, and a new sample selection approach that does not require small-loss assumption to enable a better robustness to confirmation bias than previous methods. More specifically, the new prediction disagreement is achieved with the use of different training strategies, where one model is trained with multi-class learning and the other with multi-label learning. Also, the new sample selection is based on multi-view consensus, which uses the label views from training labels and model predictions to divide the training set into clean and noisy for training the multi-class model and to re-label the training samples with multiple top-ranked labels for training the multi-label model. Extensive experiments on synthetic and real-world noisy-label datasets show that AsyCo improves over current SOTA methods.
In microscopy image cell segmentation, it is common to train a deep neural network on source data, containing different types of microscopy images, and then fine-tune it using a support set comprising a few randomly selected and annotated training target images. In this paper, we argue that the random selection of unlabelled training target images to be annotated and included in the support set may not enable an effective fine-tuning process, so we propose a new approach to optimise this image selection process. Our approach involves a new scoring function to find informative unlabelled target images. In particular, we propose to measure the consistency in the model predictions on target images against specific data augmentations. However, we observe that the model trained with source datasets does not reliably evaluate consistency on target images. To alleviate this problem, we propose novel self-supervised pretext tasks to compute the scores of unlabelled target images. Finally, the top few images with the least consistency scores are added to the support set for oracle (i.e., expert) annotation and later used to fine-tune the model to the target images. In our evaluations that involve the segmentation of five different types of cell images, we demonstrate promising results on several target test sets compared to the random selection approach as well as other selection approaches, such as Shannon's entropy and Monte-Carlo dropout.
There is a heated debate on how to interpret the decisions provided by deep learning models (DLM), where the main approaches rely on the visualization of salient regions to interpret the DLM classification process. However, these approaches generally fail to satisfy three conditions for the problem of lesion detection from medical images: 1) for images with lesions, all salient regions should represent lesions, 2) for images containing no lesions, no salient region should be produced, and 3) lesions are generally small with relatively smooth borders. We propose a new model-agnostic paradigm to interpret DLM classification decisions supported by a novel definition of saliency that incorporates the conditions above. Our model-agnostic 1-class saliency detector (MASD) is tested on weakly supervised breast lesion detection from DCE-MRI, achieving state-of-the-art detection accuracy when compared to current visualization methods.
We study the impact of different loss functions on lesion segmentation from medical images. Although the Cross-Entropy (CE) loss is the most popular option when dealing with natural images, for biomedical image segmentation the soft Dice loss is often preferred due to its ability to handle imbalanced scenarios. On the other hand, the combination of both functions has also been successfully applied in these types of tasks. A much less studied problem is the generalization ability of all these losses in the presence of Out-of-Distribution (OoD) data. This refers to samples appearing in test time that are drawn from a different distribution than training images. In our case, we train our models on images that always contain lesions, but in test time we also have lesion-free samples. We analyze the impact of the minimization of different loss functions on in-distribution performance, but also its ability to generalize to OoD data, via comprehensive experiments on polyp segmentation from endoscopic images and ulcer segmentation from diabetic feet images. Our findings are surprising: CE-Dice loss combinations that excel in segmenting in-distribution images have a poor performance when dealing with OoD data, which leads us to recommend the adoption of the CE loss for these types of problems, due to its robustness and ability to generalize to OoD samples. Code associated to our experiments can be found at https://github.com/agaldran/lesion_losses_ood.
One of the long-standing tasks in computer vision is to use a single 2-D view of an object in order to produce its 3-D shape. Recovering the lost dimension in this process has been the goal of classic shape-from-X methods, but often the assumptions made in those works are quite limiting to be useful for general 3-D objects. This problem has been recently addressed with deep learning methods containing a 2-D (convolution) encoder followed by a 3-D (deconvolution) decoder. These methods have been reasonably successful, but memory and run time constraints impose a strong limitation in terms of the resolution of the reconstructed 3-D shapes. In particular, state-of-the-art methods are able to reconstruct 3-D shapes represented by volumes of at most 323 voxels using state-of-the-art desktop computers. In this work, we present a scalable 2-D single view to 3-D volume reconstruction deep learning method, where the 3-D (deconvolution) decoder is replaced by a simple inverse discrete cosine transform (IDCT) decoder. Our simpler architecture has an order of magnitude faster inference when reconstructing 3-D volumes compared to the convolution-deconvolutional model, an exponentially smaller memory complexity while training and testing, and a sub-linear run-time training complexity with respect to the output volume size. We show on benchmark datasets that our method can produce high-resolution reconstructions with state of the art accuracy.
The performance of deep learning (DL) models is highly dependent on the quality and size of the training data, whose annotations are often expensive and hard to obtain. This work proposes a new strategy to train DL models by Learning Optimal samples Weights (LOW), making better use of the available data. LOW determines how much each sample in a batch should contribute to the training process, by automatically estimating its weight in the loss function. This effectively forces the model to focus on more relevant samples. Consequently, the models exhibit a faster convergence and better generalization, specially on imbalanced data sets where class distribution is long-tailed. LOW can be easily integrated to train any DL model and can be combined with any loss function, while adding marginal computational burden to the training process. Additionally, the analysis of how sample weights change during training provides insights on what the model is learning and which samples or classes are more challenging. Results on popular computer vision benchmarks and on medical data sets show that DL models trained with LOW perform better than with other state-of-the-art strategies. (c) 2020 Elsevier Ltd. All rights reserved.
Emerging access networks will use heterogeneous wireless technologies such as 802.11, 802.16 or UMTS, to offer users the best access to the Internet. Layer 2 access networks will consist of wireless bridges (access points) that isolate, concatenated, or in mesh provide access to mobile nodes. The transport of real time traffic over these networks may demand new QoS signalling, used to reserve resources. Besides the reservation, the new signalling needs to address the dynamics of the wireless links, the mobility of the terminals, and the multicast traffic. In this paper a new protocol is proposed aimed at solving this problem-the QoS Abstraction Layer (QoSAL). Existing only at the control plane, the QoSAL is located above the layer 2 and hides from layer 3 the details of each technology with respect to the QoS and to the network topology. The QoSAL has been designed, simulated, and tested. The results obtained demonstrate its usefulness in 4G networks.
The need for better adaptation of networks to transported flows has led to research on new approaches such as content aware networks and network aware applications. In parallel, recent developments of multimedia and content oriented services and applications such as IPTV, video streaming, video on demand, and Internet TV reinforced interest in multicast technologies. IP multicast has not been widely deployed due to interdomain and QoS support problems; therefore, alternative solutions have been investigated. This article proposes a management driven hybrid multicast solution that is multi-domain and media oriented, and combines overlay multicast, IP multicast, and P2P. The architecture is developed in a content aware network and network aware application environment, based on light network virtualization. The multicast trees can be seen as parallel virtual content aware networks, spanning a single or multiple IP domains, customized to the type of content to be transported while fulfilling the quality of service requirements of the service provider.
Time and order are considered crucial informatin in the art domain, and subject of many research efforts by historians. In this paper, we present a framework for estimating the ordering and date information of paintings and drawings. We formulate this problem as the embedding into a one dimension manifold, which aims to place paintings far or close to each other according to a measure of similarity. Out formulation can be seen as a manifold learning algorithm, albeit properly adapted to deal with existing questions in the art community. To solve this problem, we propose an approach based in Laplacian Eigenmaps and a convex optimization formulation. Both methods are able to incorporate art experties as priors to the estimation, in the form of constraints. Types of information include exact or approximate dating and partial orderings. We explore the use of soft penalty terms to allow for constraint violation to account for the fact that priors knowledge may contain small errors. Out problem is tested within the scope of the PrintART project, which aims to assist art historians in tracing Portuguese Tile art "Azulejos" back to the engravings that inspired them. Furthermore, we describe other possible applications where time information (and hence, this method) could be of use in art history, fake detection or curatorial treatment.
We introduce a new methodology that combines deep learning and level set for the automated segmentation of the left ventricle of the heart from cardiac cine magnetic resonance (MR) data. This combination is relevant for segmentation problems, where the visual object of interest presents large shape and appearance variations, but the annotated training set is small, which is the case for various medical image analysis applications, including the one considered in this paper. In particular, level set methods are based on shape and appearance terms that use small training sets, but present limitations for modelling the visual object variations. Deep learning methods can model such variations using relatively small amounts of annotated training, but they often need to be regularised to produce good generalisation. Therefore, the combination of these methods brings together the advantages of both approaches, producing a methodology that needs small training sets and produces accurate segmentation results. We test our methodology on the MICCAI 2009 left ventricle segmentation challenge database (containing 15 sequences for training, 15 for validation and 15 for testing), where our approach achieves the most accurate results in the semi-automated problem and state-of-the-art results for the fully automated challenge. Crown Copyright (C) 2016 Published by Elsevier B.V. All rights reserved.
The global COVID-19 pandemic has resulted in huge pressures on healthcare systems, with lung imaging, from chest radiographs (CXR) to computed tomography (CT) and ultrasound (US) of the thorax, playing an important role in the diagnosis and management of patients with coronavirus infection. The AI community reacted rapidly to the threat of the coronavirus pandemic by contributing numerous initiatives of developing AI technologies for interpreting lung images across the different modalities. We performed a thorough review of all relevant publications in 2020 [1] and identified numerous trends and insights that may help in accelerating the translation of AI technology in clinical practice in pandemic times. This workshop is devoted to the lessons learned from this accelerated process and in paving the way for further AI adoption. In particular, the objective is to bring together radiologists and AI experts to review the scientific progress in the development of AI technologies for medical imaging to address the COVID-19 pandemic and share observations regarding the data relevance, the data availability and the translational aspects of AI research and development. We aim at understanding if and what needs to be done differently in developing technologies of AI for lung images of COVID-19 patients, given the pressure of an unprecedented pandemic - which processes are working, which should be further adapted, and which approaches should be abandoned.
The advent of learning with noisy labels (LNL), multi-rater learning, and human-AI collaboration has revolutionised the development of robust classifiers, enabling them to address the challenges posed by different types of data imperfections and complex decision processes commonly encountered in real-world applications. While each of these methodologies has individually made significant strides in addressing their unique challenges, the development of techniques that can simultaneously tackle these three problems remains underexplored. This paper addresses this research gap by integrating noisy-label learning, multi-rater learning, and human-AI collaboration with new benchmarks and the innovative Learning to Complement with Multiple Humans (LECOMH) approach. LECOMH optimises the level of human collaboration during testing, aiming to optimise classification accuracy while minimising collaboration costs that vary from 0 to M, where M is the maximum number of human collaborators. We quantitatively compare LECOMH with leading human-AI collaboration methods using our proposed benchmarks. LECOMH consistently outperforms the competition, with accuracy improving as collaboration costs increase. Notably, LECOMH is the only method enhancing human labeller performance across all benchmarks.
Real-world large-scale medical image analysis (MIA) datasets have three challenges: 1) they contain noisy-labelled samples that affect training convergence and generalisation, 2) they usually have an imbalanced distribution of samples per class, and 3) they normally comprise a multi-label problem, where samples can have multiple diagnoses. Current approaches are commonly trained to solve a subset of those problems, but we are unaware of methods that address the three problems simultaneously. In this paper, we propose a new training module called Non-Volatile Unbiased Memory (NVUM), which non-volatility stores running average of model logits for a new regularization loss on noisy multi-label problem. We further unbias the classification prediction in NVUM update for imbalanced learning problem. We run extensive experiments to evaluate NVUM on new benchmarks proposed by this paper, where training is performed on noisy multi-label imbalanced chest X-ray (CXR) training sets, formed by Chest-Xray14 and CheXpert, and the testing is performed on the clean multi-label CXR datasets OpenI and PadChest. Our method outperforms previous state-of-the-art CXR classifiers and previous methods that can deal with noisy labels on all evaluations. Our code is available at https://github.com/FBLADL/NVUM.
Domain generalisation represents the challenging problem of using multiple training domains to learn a model that can generalise to previously unseen target domains. Recent papers have proposed using data augmentation to produce realistic adversarial examples to simulate domain shift. Under current domain adaptation/generalisation theory, it is unclear whether training with data augmentation alone is sufficient to improve domain generalisation results. We propose an extension of the current domain generalisation theoretical framework and a new method that combines data augmentation and domain distance minimisation to reduce the upper bound on domain generalisation error. Empirically, our algorithm produces competitive results when compared with the state-of-the-art methods in the domain generalisation benchmark PACS. We have also performed an ablation study of the technique on a real-world chest x-ray dataset, consisting of a subset of CheXpert, Chest14, and PadChest datasets. The result shows that the proposed method works best when the augmented domains are realistic, but it can perform robustly even when domain augmentation fails to produce realistic samples.
This paper proposes a novel combination of manifold learning with deep belief networks for the detection and segmentation of left ventricle (LV) in 2D - ultrasound (US) images. The main goal is to reduce both training and inference complexities while maintaining the segmentation accuracy of machine learning based methods for non-rigid segmentation methodologies. The manifold learning approach used can be viewed as an atlas-based segmentation. It partitions the data into several patches. Each patch proposes a segmentation of the LV that somehow must be fused. This is accomplished by a deep belief network (DBN) multi-classifier that assigns a weight for each patch LV segmentation. The approach is thus threefold: (i) it does not rely on a single segmentation, (ii) it provides a great reduction in the rigid detection phase that is performed at lower dimensional space comparing with the initial contour space, and (iii) DBN's allows for a training process that can produce robust appearance models without the need of large annotated training sets.
This work presents an algorithm based on weak supervision to automatically localize an arthroscope on 3D ultrasound (US). The ultimate goal of this application is to combine 3D US with the 2D arthroscope view during knee arthroscopy, to provide the surgeon with a comprehensive view of the surgical site. The implemented algorithm consisted of a weakly supervised neural network, which was trained on 2D US images of different phantoms mimicking the imaging conditions during knee arthroscopy. Image-based classification was performed and the resulting class activation maps were used to localize the arthroscope. The localization performance was evaluated visually by three expert reviewers and by the calculation of objective metrics. Finally, the algorithm was also tested on a human cadaver knee. The algorithm achieved an average classification accuracy of 88.6% on phantom data and 83.3% on cadaver data. The localization of the arthroscope based on the class activation maps was correct in 92-100% of all true positive classifications for both phantom and cadaver data. These results are relevant because they show feasibility of automatic arthroscope localization in 3D US volumes, which is paramount to combining multiple image modalities that are available during knee arthroscopies.
State-of-the-art (SOTA) deep learning mammogram classifiers, trained with weakly-labelled images, often rely on global models that produce predictions with limited interpretability, which is a key barrier to their successful translation into clinical practice. On the other hand, prototype-based models improve interpretability by associating predictions with training image prototypes, but they are less accurate than global models and their prototypes tend to have poor diversity. We address these two issues with the proposal of BRAIxPro-toPNet++, which adds interpretability to a global model by ensembling it with a prototype-based model. BRAIxProtoPNet++ distills the knowledge of the global model when training the prototype-based model with the goal of increasing the classification accuracy of the ensemble. Moreover, we propose an approach to increase prototype diversity by guaranteeing that all prototypes are associated with different training images. Experiments on weakly-labelled private and public datasets show that BRAIxProtoPNet++ has higher classification accuracy than SOTA global and prototype-based models. Using lesion localisation to assess model interpretability, we show BRAIxProtoPNet++ is more effective than other prototype-based models and post-hoc explanation of global models. Finally, we show that the diversity of the prototypes learned by BRAIxProtoPNet++ is superior to SOTA prototype-based approaches.
The paper presents an end-to-end quality of service (QoS) architecture suitable for IP communications scenarios that include UMTS access networks. The rationale for the architecture is justified and its main features are described, notably the QoS management functions on the terminal equipment, the mapping between IP and UMTS QoS parameters and the negotiation of these parameters.
Artistic image understanding is an interdisciplinary research field of increasing importance for the computer vision and art history communities. One of the goals of this field is the implementation of a system that can automatically retrieve and annotate artistic images. The best approach in the field explores the artistic influence among different artistic images using graph-based learning methodologies that take into consideration appearance and label similarities, but the current state-of-the-art results indicate that there seems to be lots of room for improvements in terms of retrieval and annotation accuracy. In order to improve those results, we introduce novel human figure composition features that can compute the similarity between artistic images based on the location and number (i.e., composition) of human figures. Our main motivation for developing such features lies in the importance that composition (particularly the composition of human figures) has in the analysis of artistic images when defining the visual classes present in those images. We show that the introduction of such features in the current dominant methodology of the field improves significantly the state-of-the-art retrieval and annotation accuracies on the PRINTART database, which is a public database exclusively composed of artistic images.
We present a new supervised learning model designed for the automatic segmentation of the left ventricle (LV) of the heart in ultrasound images. We address the following problems inherent to supervised learning models: 1) the need of a large set of training images; 2) robustness to imaging conditions not present in the training data; and 3) complex search process. The innovations of our approach reside in a formulation that decouples the rigid and nonrigid detections, deep learning methods that model the appearance of the LV, and efficient derivative-based search algorithms. The functionality of our approach is evaluated using a data set of diseased cases containing 400 annotated images (from 12 sequences) and another data set of normal cases comprising 80 annotated images (from two sequences), where both sets present long axis views of the LV. Using several error measures to compute the degree of similarity between the manual and automatic segmentations, we show that our method not only has high sensitivity and specificity but also presents variations with respect to a gold standard (computed from the manual annotations of two experts) within interuser variability on a subset of the diseased cases. We also compare the segmentations produced by our approach and by two state-of-the-art LV segmentation models on the data set of normal cases, and the results show that our approach produces segmentations that are comparable to these two approaches using only 20 training images and increasing the training set to 400 images causes our approach to be generally more accurate. Finally, we show that efficient search methods reduce up to tenfold the complexity of the method while still producing competitive segmentations. In the future, we plan to include a dynamical model to improve the performance of the algorithm, to use semisupervised learning methods to reduce even more the dependence on rich and large training sets, and to design a shape model less dependent on the training set.
Deep learning methods have shown outstanding classification accuracy in medical imaging problems, which is largely attributed to the availability of large-scale datasets manually annotated with clean labels. However, given the high cost of such manual annotation, new medical imaging classification problems may need to rely on machine-generated noisy labels extracted from radiology reports. Indeed, many Chest X-ray (CXR) classifiers have already been modelled from datasets with noisy labels, but their training procedure is in general not robust to noisy-label samples, leading to sub-optimal models. Furthermore, CXR datasets are mostly multi-label, so current noisy-label learning methods designed for multi-class problems cannot be easily adapted. In this paper, we propose a new method designed for the noisy multi-label CXR learning, which detects and smoothly re-labels samples from the dataset, which is then used to train common multi-label classifiers. The proposed method optimises a bag of multi-label descriptors (BoMD) to promote their similarity with the semantic descriptors produced by BERT models from the multi-label image annotation. Our experiments on diverse noisy multi-label training sets and clean testing sets show that our model has state-of-the-art accuracy and robustness in many CXR multi-label classification benchmarks.
Generalisation of deep neural networks becomes vulnerable when distribution shifts are encountered between train (source) and test (target) domain data. Few-shot domain adaptation mitigates this issue by adapting deep neural networks pre-trained on the source domain to the target domain using a randomly selected and annotated support set from the target domain. This paper argues that randomly selecting the support set can be further improved for effectively adapting the pre-trained source models to the target domain. Alternatively, we propose SelectNAdapt, an algorithm to curate the selection of the target domain samples, which are then annotated and included in the support set. In particular, for the K-shot adaptation problem, we first leverage self-supervision to learn features of the target domain data. Then, we propose a per-class clustering scheme of the learned target domain features and select K representative target samples using a distance-based scoring function. Finally, we bring our selection setup towards a practical ground by relying on pseudo-labels for clustering semantically similar target domain samples. Our experiments show promising results on three few-shot domain adaptation benchmarks for image recognition compared to related approaches and the standard random selection.
We present the Region of Interest Autoencoder (ROIAE), a combined supervised and reconstruction model for the automatic visual detection of objects. More specifically, we augment the detection loss function with a reconstruction loss that targets only foreground examples. This allows us to exploit more effectively the information available in the sparsely populated foreground training data used in common detection problems. Using this training strategy we improve the accuracy of deep learning detection models. We carry out experiments on the Caltech-USA pedestrian detection dataset and demonstrate improvements over two supervised baselines. Our first experiment extends Fast R-CNN and achieves a 4% relative improvement in test accuracy over its purely supervised baseline. Our second experiment extends Region Proposal Networks, achieving a 14% relative improvement in test accuracy.
2022 44th Annual International Conference of the IEEE Engineering in Medicine & Biology Society (EMBC), 2022, pp. 3510-3513 Many applications in image-guided surgery and therapy require fast and reliable non-linear, multi-modal image registration. Recently proposed unsupervised deep learning-based registration methods have demonstrated superior performance compared to iterative methods in just a fraction of the time. Most of the learning-based methods have focused on mono-modal image registration. The extension to multi-modal registration depends on the use of an appropriate similarity function, such as the mutual information (MI). We propose guiding the training of a deep learning-based registration method with MI estimation between an image-pair in an end-to-end trainable network. Our results show that a small, 2-layer network produces competitive results in both mono- and multi-modal registration, with sub-second run-times. Comparisons to both iterative and deep learning-based methods show that our MI-based method produces topologically and qualitatively superior results with an extremely low rate of non-diffeomorphic transformations. Real-time clinical application will benefit from a better visual matching of anatomical structures and less registration failures/outliers.
Interconnection of different access network technologies is an important research topic in mobile telecommunications systems. In this paper, we propose an ns-3 architecture for simulating the interconnection of wireless local area network (WLAN) and Universal Mobile Telecommunications System (UMTS). This architecture is based on the architecture proposed by the Third Generation Partnership Project, being the use of virtual interfaces as its main innovation. In order to demonstrate the value of the proposed simulation framework, we implemented the UMTS and WLAN interconnection considering three joint radio resource management strategies for distributing arriving calls. From the simulations results, we can conclude that the proposed simulation architecture is suitable to test and evaluate performance aspects related to the interconnection and joint management of UMTS and WLAN technologies. Copyright (c) 2012 John Wiley & Sons, Ltd.
•We introduce a novel automated CAD system with minimal user intervention that can detect, segment and classify breast masses from mammograms. We explore deep learning and structured output models for the design and development of the proposed CAD system. More specifically for the detection, we propose a cascade of deep learning methods to select hypotheses that are refined based on Bayesian optimization. For the segmentation, we propose the use of deep structured output learning that is subsequently refined by a level set method. Finally, for the classification, we propose a deep learning classifier that is pre-trained with a regression to hand-crafted feature values and fine-tuned based on the annotations of the breast mass classification dataset. Our proposed CAD system produces the current state-of-the-art detection, segmentation and classification results for the INbreast dataset. [Display omitted] We present an integrated methodology for detecting, segmenting and classifying breast masses from mammograms with minimal user intervention. This is a long standing problem due to low signal-to-noise ratio in the visualisation of breast masses, combined with their large variability in terms of shape, size, appearance and location. We break the problem down into three stages: mass detection, mass segmentation, and mass classification. For the detection, we propose a cascade of deep learning methods to select hypotheses that are refined based on Bayesian optimisation. For the segmentation, we propose the use of deep structured output learning that is subsequently refined by a level set method. Finally, for the classification, we propose the use of a deep learning classifier, which is pre-trained with a regression to hand-crafted feature values and fine-tuned based on the annotations of the breast mass classification dataset. We test our proposed system on the publicly available INbreast dataset and compare the results with the current state-of-the-art methodologies. This evaluation shows that our system detects 90% of masses at 1 false positive per image, has a segmentation accuracy of around 0.85 (Dice index) on the correctly detected masses, and overall classifies masses as malignant or benign with sensitivity (Se) of 0.98 and specificity (Sp) of 0.7.
This paper concerns the robust estimation of non-rigid deformations from feature correspondences. We advance the surprising view that for many realistic physical deformations, the error of the mismatches (outliers) usually dwarfs the effects of the curvature of the manifold on which the correct matches (inliers) lie, to the extent that one can tightly enclose the manifold within the error bounds of a low-dimensional hyperplane for accurate outlier rejection. This justifies a simple RANSAC-driven deformable registration technique that is at least as accurate as other methods based on the optimisation of fully deformable models. We support our ideas with comprehensive experiments on synthetic and real data typical of the deformations examined in the literature.
We show two important findings on the use of deep convolutional neural networks (CNN) in medical image analysis. First, we show that CNN models that are pre-trained using computer vision databases (e.g., Imagenet) are useful in medical image applications, despite the significant differences in image appearance. Second, we show that multiview classification is possible without the pre-registration of the input images. Rather, we use the high-level features produced by the CNNs trained in each view separately. Focusing on the classification of mammograms using craniocaudal (CC) and mediolateral oblique (MLO) views and their respective mass and micro-calcification segmentations of the same breast, we initially train a separate CNN model for each view and each segmentation map using an Imagenet pre-trained model. Then, using the features learned from each segmentation map and unregistered views, we train a final CNN classifier that estimates the patient's risk of developing breast cancer using the Breast Imaging-Reporting and Data System (BI-RADS) score. We test our methodology in two publicly available datasets (InBreast and DDSM), containing hundreds of cases, and show that it produces a volume under ROC surface of over 0.9 and an area under ROC curve (for a 2-class problem - benign and malignant) of over 0.9. In general, our approach shows state-of-the-art classification results and demonstrates a new comprehensive way of addressing this challenging classification problem.
Accurate bowel segmentation is essential for diagnosis and treatment of bowel cancers. Unfortunately, segmenting the entire bowel in CT images is quite challenging due to unclear boundary, large shape, size, and appearance variations, as well as diverse filling status within the bowel. In this paper, we present a novel two-stage framework, named BowelNet, to handle the challenging task of bowel segmentation in CT images, with two stages of 1) jointly localizing all types of the bowel, and 2) finely segmenting each type of the bowel. Specifically, in the first stage, we learn a unified localization network from both partially- and fully-labeled CT images to robustly detect all types of the bowel. To better capture unclear bowel boundary and learn complex bowel shapes, in the second stage, we propose to jointly learn semantic information (i.e., bowel segmentation mask) and geometric representations (i.e., bowel boundary and bowel skeleton) for fine bowel segmentation in a multi-task learning scheme. Moreover, we further propose to learn a meta segmentation network via pseudo labels to improve segmentation accuracy. By evaluating on a large abdominal CT dataset, our proposed BowelNet method can achieve Dice scores of 0.764, 0.848, 0.835, 0.774, and 0.824 in segmenting the duodenum, jejunum-ileum, colon, sigmoid, and rectum, respectively. These results demonstrate the effectiveness of our proposed BowelNet framework in segmenting the entire bowel from CT images.
We introduce a new type of local feature based on the phase and amplitude responses of complex-valued steerable filters. The design of this local feature is motivated by a desire to obtain feature vectors which are semi-invariant under common image deformations, yet distinctive enough to provide useful identity information. A recent proposal for such local features involves combining differential invariants to particular image deformations, such as rotation. Our approach differs in that we consider a wider class of image deformations, including the addition of noise, along with both global and local brightness variations. We use steerable filters to make the feature robust to rotation. And we exploit the fact that phase data is often locally stable with respect to scale changes, noise, and common brightness changes. We provide empirical results comparing our local feature with one based on differential invariants. The results show that our phase-based local feature leads to better performance when dealing with common illumination changes and 2-D rotation, while giving comparable effects in terms of scale changes.
We introduce a new fully automated breast mass segmentation method from dynamic contrast-enhanced magnetic resonance imaging (DCE-MRI). The method is based on globally optimal inference in a continuous space (GOCS) using a shape prior computed from a semantic segmentation produced by a deep learning (DL) model. We propose this approach because the limited amount of annotated training samples does not allow the implementation of a robust DL model that could produce accurate segmentation results on its own. Furthermore, GOCS does not need precise initialisation compared to locally optimal methods on a continuous space (e.g., Mumford-Shah based level set methods); also, GOCS has smaller memory complexity compared to globally optimal inference on a discrete space (e.g., graph cuts). Experimental results show that the proposed method produces the current state-of-the-art mass segmentation (from DCE-MRI) results, achieving a mean Dice coefficient of 0.77 for the test set.
There has been a resurgence of interest in one of the most fundamental aspects of computer vision, which is related to the existence of a feedback mechanism in the inference of a visual classification process. Indeed, this mechanism was present in the first computer vision methodologies, but technical and theoretical issues imposed major roadblocks that forced researchers to seek alternative approaches based on pure feed-forward inference. These open loop approaches process the input image sequentially with increasingly more complex analysis steps, and any mistake made by intermediate steps impair all subsequent analysis tasks. On the other hand, closed-loop approaches involving feed- forward and feedback mechanisms can fix mistakes made during such intermediate stages. In this paper, we present a new closed- loop inference for computer vision problems based on an iterative analysis using deep belief networks (DBN). Specifically, an image is processed using a feed-forward mechanism that will produce a classification result, which is then used to sample an image from the current belief state of the DBN. Then the difference between the input image and the sampled image is fed back to the DBN for re- classification, and this process iterates until convergence. We show that our closed-loop vision inference improves the classification results compared to pure feed-forward mechanisms on the MNIST handwritten digit dataset and the Multiple Object Categories containing shapes of horses, dragonflies, llamas and rhinos.
AI-assisted colonoscopy has received lots of attention in the last decade. Several randomised clinical trials in the previous two years showed exciting results of the improving detection rate of polyps. However, current commercial AI-assisted colonoscopy systems focus on providing visual assistance for detecting polyps during colonoscopy. There is a lack of understanding of the needs of gastroenterologists and the usability issues of these systems. This paper aims to introduce the recent development and deployment of commercial AI-assisted colonoscopy systems to the HCI community, identify gaps between the expectation of the clinicians and the capabilities of the commercial systems, and highlight some unique challenges in Australia.
The detection and classification of anatomies from medical images has traditionally been developed in a two-stage process, where the first stage detects the regions of interest (ROIs), while the second stage classifies the detected ROIs. Recent developments from the computer vision community allowed the unification of these two stages into a single detection and classification model that is trained in an end to end fashion. This allows for a simpler and faster training and inference procedures because only one model (instead of the two models needed for the two-stage approach) is required. In this paper, we adapt a recently proposed onestage detection and classification approach for the new 5class polyp classification problem. We show that this onestage approach is not only competitive in terms of detection and classification accuracy with respect to the two-stage approach, but it is also substantially faster for training and testing. We also show that the one-stage approach produces competitive detection results compared to the state of the art results on the MICCAI 2015 polyp detection challenge.
A significant weakness of most current deep Convolutional Neural Networks is the need to train them using vast amounts of manually labelled data. In this work we propose a unsupervised framework to learn a deep convolutional neural network for single view depth prediction, without requiring a pre-training stage or annotated ground-truth depths. We achieve this by training the network in a manner analogous to an autoencoder. At training time we consider a pair of images, source and target, with small, known camera motion between the two such as a stereo pair. We train the convolutional encoder for the task of predicting the depth map for the source image. To do so, we explicitly generate an inverse warp of the target image using the predicted depth and known inter-view displacement, to reconstruct the source image; the photometric error in the reconstruction is the reconstruction loss for the encoder. The acquisition of this training data is considerably simpler than for equivalent systems, requiring no manual annotation, nor calibration of depth sensor to camera. We show that our network trained on less than half of the KITTI dataset gives comparable performance to that of the state-of-the-art supervised methods for single view depth estimation.
Capturing large amounts of accurate and diverse 3D data for training is often time consuming and expensive, either requiring many hours of artist time to model each object, or to scan from real world objects using depth sensors or structure from motion techniques. To address this problem, we present a method for reconstructing 3D textured point clouds from single input images without any 3D ground truth training data. We recast the problem of 3D point cloud estimation as that of performing two separate processes, a novel view synthesis and a depth/shape estimation from the novel view images. To train our models we leverage the recent advances in deep generative modelling and self-supervised learning. We show that our method outperforms recent supervised methods, and achieves state of the art results when compared with another recently proposed unsupervised method. Furthermore, we show that our method is capable of recovering textural information which is often missing from many previous approaches that rely on supervision.
Knee arthroscopy is a complex minimally invasive surgery that can cause unintended injuries to femoral cartilage or postoperative complications, or both. Autonomous robotic systems using real-time volumetric ultrasound (US) imaging guidance hold potential for reducing significantly these issues and for improving patient outcomes. To enable the robotic system to navigate autonomously in the knee joint, the imaging system should provide the robot with a real-time comprehensive map of the surgical site. To this end, the first step is automatic image quality assessment, to ensure that the boundaries of the relevant knee structures are defined well enough to be detected, outlined, and then tracked. In this article, a recently developed one-class classifier deep learning algorithm was used to discriminate among the US images acquired in a simulated surgical scenario on which the femoral cartilage either could or could not be outlined. A total of 38 656 2-D US images were extracted from 151 3-D US volumes, collected from six volunteers, and were labeled as "1" or as "0" when an expert was or was not able to outline the cartilage on the image, respectively. The algorithm was evaluated using the expert labels as ground truth with a fivefold cross validation, where each fold was trained and tested on average with 15 640 and 6246 labeled images, respectively. The algorithm reached a mean accuracy of 78.4% ± 5.0, mean specificity of 72.5% ± 9.4, mean sensitivity of 82.8% ± 5.8, and mean area under the curve of 85% ± 4.4. In addition, interobserver and intraobserver tests involving two experts were performed on an image subset of 1536 2-D US images. Percent agreement values of 0.89 and 0.93 were achieved between two experts (i.e., interobserver) and by each expert (i.e., intraobserver), respectively. These results show the feasibility of the first essential step in the development of automatic US image acquisition and interpretation systems for autonomous robotic knee arthroscopy.
A large number of different lesions and pathologies can affect the human digestive system, resulting in life-threatening situations. Early detection plays a relevant role in the successful treatment and the increase of current survival rates to, e.g., colorectal cancer. The standard procedure enabling detection, endoscopic video analysis, generates large quantities of visual data that need to be carefully analyzed by an specialist. Due to the wide range of color, shape, and general visual appearance of pathologies, as well as highly varying image quality, such process is greatly dependent on the human operator experience and skill. In this work, we detail our solution to the task of multi-category classification of images from the gastrointestinal (GI) human tract within the 2020 Endotect Challenge. Our approach is based on a Convolutional Neural Network minimizing a hierarchical error function that takes into account not only the finding category, but also its location within the GI tract (lower/upper tract), and the type of finding (pathological finding/therapeutic intervention/anatomical landmark/mucosal views' quality). We also describe in this paper our solution for the challenge task of polyp segmentation in colonoscopies, which was addressed with a pretrained double encoder-decoder network. Our internal cross-validation results show an average performance of 91.25 Mathews Correlation Coefficient (MCC) and 91.82 Micro-F1 score for the classification task, and a 92.30 F1 score for the polyp segmentation task. The organization provided feedback on the performance in a hidden test set for both tasks, which resulted in 85.61 MCC and 86.96 F1 score for classification, and 91.97 F1 score for polyp segmentation. At the time of writing no public ranking for this challenge had been released.
The increased diversity and scale of published biological data has to led to a growing appreciation for the applications of machine learning and statistical methodologies to gain new insights. Key to achieving this aim is solving the Relationship Extraction problem which specifies the semantic interaction between two or more biological entities in a published study. Here, we employed two deep neural network natural language processing (NLP) methods, namely: the continuous bag of words (CBOW), and the bi-directional long short-term memory (bi-LSTM). These methods were employed to predict relations between entities that describe protein subcellular localisation in plants. We applied our system to 1700 published Arabidopsis protein subcellular studies from the SUBA manually curated dataset. The system combines pre-processing of full-text articles in a machine-readable format with relevant sentence extraction for downstream NLP analysis. Using the SUBA corpus, the neural network classifier predicted interactions between protein name, subcellular localisation and experimental methodology with an average precision, recall rate, accuracy and F1 scores of 95.1%, 82.8%, 89.3% and 88.4% respectively (n = 30). Comparable scoring metrics were obtained using the CropPAL database as an independent testing dataset that stores protein subcellular localisation in crop species, demonstrating wide applicability of prediction model. We provide a framework for extracting protein functional features from unstructured text in the literature with high accuracy, improving data dissemination and unlocking the potential of big data text analytics for generating new hypotheses.
We propose a method that substantially improves the efficiency of deep distance metric learning based on the optimization of the triplet loss function. One epoch of such training process based on a na¨ıve optimization of the triplet loss function has a run-time complexity O(N^3), where N is the number of training samples. Such optimization scales poorly, and the most common approach proposed to address this high complexity issue is based on sub-sampling the set of triplets needed for the training process. Another approach explored in the field relies on an ad-hoc linearization (in terms of N) of the triplet loss that introduces class centroids, which must be optimized using the whole training set for each mini-batch - this means that a na¨ıve implementation of this approach has run-time complexity O(N^2). This complexity issue is usually mitigated with poor, but computationally cheap, approximate centroid optimization methods. In this paper, we first propose a solid theory on the linearization of the triplet loss with the use of class centroids, where the main conclusion is that our new linear loss represents a tight upper-bound to the triplet loss. Furthermore, based on the theory above, we propose a training algorithm that no longer requires the centroid optimization step, which means that our approach is the first in the field with a guaranteed linear run-time complexity. We show that the training of deep distance metric learning methods using the proposed upper-bound is substantially faster than triplet-based methods, while producing competitive retrieval accuracy results on benchmark datasets (CUB-200-2011 and CAR196).
Minimally invasive surgery (MIS) is among the preferred procedures for treating a number of ailments as patients benefit from fast recovery and reduced blood loss. The trade-off is that surgeons lose direct visual contact with the surgical site and have limited intra-operative imaging techniques for real-time feedback. Computer vision methods as well as segmentation and tracking of the tissues and tools in the video frames, are increasingly being adopted to MIS to alleviate such limitations. So far, most of the advances in MIS have been focused on laparoscopic applications, with scarce literature on knee arthroscopy. Here for the first time, we propose a new method for the automatic segmentation of multiple tissue structures for knee arthroscopy. The training data of 3868 images were collected from 4 cadaver experiments, 5 knees, and manually contoured by two clinicians into four classes: Femur, Anterior Cruciate Ligament (ACL), Tibia, and Meniscus. Our approach adapts the U-net and the U-net++ architectures for this segmentation task. Using the cross-validation experiment, the mean Dice similarity coefficients for Femur, Tibia, ACL, and Meniscus are 0.78, 0.50, 0.41, 0.43 using the U-net and 0.79, 0.50, 0.51, 0.48 using the U-net++. While the reported segmentation method is of great applicability in terms of contextual awareness for the surgical team, it can also be used for medical robotic applications such as SLAM and depth mapping.
Ultrasound (US) imaging is a complex imaging modality, where the tissues are typically characterised by an inhomogeneous image intensity and by a variable image definition at the boundaries that depends on the direction of the incident sound wave. For this reason, conventional image segmentation approaches where the regions of interest are represented by exact masks are inherently inefficient for US images. To solve this issue, we present the first application of a Bayesian convolutional neural network (CNN) based on Monte Carlo dropout on US imaging. This approach is particularly relevant for quantitative applications since differently from traditional CNNs, it enables to infer for each image pixel not only the probability of being part of the target but also the algorithm confidence (i.e. uncertainty) in assigning that probability. In this work, this technique has been applied on US images of the femoral cartilage in the framework of a new application, where high-refresh-rate volumetric US is used for guidance in minimally invasive robotic surgery for the knee. Two options were explored, where the Bayesian CNN was trained with the femoral cartilage contoured either on US, or on magnetic resonance imaging (MRI) and then projected onto the corresponding US volume. To evaluate the segmentation performance, we propose a novel approach where a probabilistic ground-truth annotation was generated combining the femoral cartilage contours from registered US and MRI volumes. Both cases produced a significantly better segmentation performance when compared against traditional CNNs, achieving a dice score coefficient increase of about 6% and 8%, respectively.
We describe an automated methodology for the analysis of unregistered cranio-caudal (CC) and medio-lateral oblique (MLO) mammography views in order to estimate the patient's risk of developing breast cancer. The main innovation behind this methodology lies in the use of deep learning models for the problem of jointly classifying unregistered mammogram views and respective segmentation maps of breast lesions (i.e., masses and micro-calcifications). This is a holistic methodology that can classify a whole mammographic exam, containing the CC and MLO views and the segmentation maps, as opposed to the classification of individual lesions, which is the dominant approach in the field. We also demonstrate that the proposed system is capable of using the segmentation maps generated by automated mass and micro-calcification detection systems, and still producing accurate results. The semi-automated approach (using manually defined mass and micro-calcification segmentation maps) is tested on two publicly available data sets (INbreast and DDSM), and results show that the volume under ROC surface (VUS) for a 3-class problem (normal tissue, benign, and malignant) is over 0.9, the area under ROC curve (AUC) for the 2-class "benign versus malignant" problem is over 0.9, and for the 2-class breast screening problem (malignancy versus normal/benign) is also over 0.9. For the fully automated approach, the VUS results on INbreast is over 0.7, and the AUC for the 2-class "benign versus malignant" problem is over 0.78, and the AUC for the 2-class breast screening is 0.86.
Navigation in endoscopic environments requires an accurate and robust localisation system. A key challenge in such environments is the paucity of visual features that hinders accurate tracking. This article examines the performance of three image enhancement techniques for tracking under such feature-poor conditions including Contrast Limited Adaptive Histogram Specification (CLAHS), Fast Local Laplacian Filtering (LLAP) and a new combination of the two coined Local Laplacian of Specified Histograms (LLSH). Two cadaveric knee arthroscopic datasets and an underwater seabed inspection dataset are used for the analysis, where results are interpreted by defining visual saliency as the number of correctly matched key-point (SIFT and SURF) features. Experimental results show a significant improvement in contrast quality and feature matching performance when image enhancement techniques are used. Results also demonstrate the LLSHs ability to vastly improve SURF tracking performance indicating more than 87% of successfully matched frames. A comparative analysis provides some important insights useful in the design of vision-based navigation for autonomous agents in feature-poor environments.
Intra-operative automatic semantic segmentation of knee joint structures can assist surgeons during knee arthroscopy in terms of situational awareness. However, due to poor imaging conditions (e.g., low texture, overexposure, etc.), automatic semantic segmentation is a challenging scenario, which justifies the scarce literature on this topic. In this paper, we propose a novel self-supervised monocular depth estimation to regularise the training of the semantic segmentation in knee arthroscopy. To further regularise the depth estimation, we propose the use of clean training images captured by the stereo arthroscope of routine objects (presenting none of the poor imaging conditions and with rich texture information) to pre-train the model. We fine-tune such model to produce both the semantic segmentation and self-supervised monocular depth using stereo arthroscopic images taken from inside the knee. Using a data set containing 3868 arthroscopic images captured during cadaveric knee arthroscopy with semantic segmentation annotations, 2000 stereo image pairs of cadaveric knee arthroscopy, and 2150 stereo image pairs of routine objects, we show that our semantic segmentation regularised by self-supervised depth estimation produces a more accurate segmentation than a state-of-the-art semantic segmentation approach modeled exclusively with semantic segmentation annotation.
Polyps represent an early sign of the development of Colorectal Cancer. The standard procedure for their detection consists of colonoscopic examination of the gastrointestinal tract. However, the wide range of polyp shapes and visual appearances, as well as the reduced quality of this image modality, turn their automatic identification and segmentation with computational tools into a challenging computer vision task. In this work, we present a new strategy for the delineation of gastrointestinal polyps from endoscopic images based on a direct extension of common encoder-decoder networks for semantic segmentation. In our approach, two pretrained encoder-decoder networks are sequentially stacked: the second network takes as input the concatenation of the original frame and the initial prediction generated by the first network, which acts as an attention mechanism enabling the second network to focus on interesting areas within the image, thereby improving the quality of its predictions. Quantitative evaluation carried out on several polyp segmentation databases shows that double encoder-decoder networks clearly outperform their single encoder-decoder counterparts in all cases. In addition, our best double encoder-decoder combination attains excellent segmentation accuracy and reaches state-of-the-art performance results in all the considered datasets, with a remarkable boost of accuracy on images extracted from datasets not used for training.
The classification of breast masses from mammograms into benign or malignant has been commonly addressed with machine learning classifiers that use as input a large set of hand-crafted features, usually based on general geometrical and texture information. In this paper, we propose a novel deep learning method that automatically learns features based directly on the optmisation of breast mass classification from mammograms, where we target an improved classification performance compared to the approach described above. The novelty of our approach lies in the two-step training process that involves a pre-training based on the learning of a regressor that estimates the values of a large set of hand-crafted features, followed by a fine-tuning stage that learns the breast mass classifier. Using the publicly available INbreast dataset, we show that the proposed method produces better classification results, compared with the machine learning model using hand-crafted features and with deep learning method trained directly for the classification stage without the pre-training stage. We also show that the proposed method produces the current state-of-the-art breast mass classification results for the INbreast dataset. Finally, we integrate the proposed classifier into a fully automated breast mass detection and segmentation, which shows promising results.
There are two challenges associated with the interpretability of deep learning models in medical image analysis applications that need to be addressed: confidence calibration and classification uncertainty. Confidence calibration associates the classification probability with the likelihood that it is actually correct - hence, a sample that is classified with confidence X% has a chance of X% of being correctly classified. Classification uncertainty estimates the noise present in the classification process, where such noise estimate can be used to assess the reliability of a particular classification result. Both confidence calibration and classification uncertainty are considered to be helpful in the interpretation of a classification result produced by a deep learning model, but it is unclear how much they affect classification accuracy and calibration, and how they interact. In this paper, we study the roles of confidence calibration (via post-process temperature scaling) and classification uncertainty (computed either from classification entropy or the predicted variance produced by Bayesian methods) in deep learning models. Results suggest that calibration and uncertainty improve classification interpretation and accuracy. This motivates us to propose a new Bayesian deep learning method that relies both on calibration and uncertainty to improve classification accuracy and model interpretability. Experiments are conducted on a recently proposed five-class polyp classification problem, using a data set containing 940 high-quality images of colorectal polyps, and results indicate that our proposed method holds the state-of-the-art results in terms of confidence calibration and classification accuracy. (C) 2020 Elsevier B.V. All rights reserved.
Automatic cell segmentation in microscopy images works well with the support of deep neural networks trained with full supervision. Collecting and annotating images, though, is not a sustainable solution for every new microscopy database and cell type. Instead, we assume that we can access a plethora of annotated image data sets from different domains (sources) and a limited number of annotated image data sets from the domain of interest (target), where each domain denotes not only different image appearance but also a different type of cell segmentation problem. We pose this problem as meta-learning where the goal is to learn a generic and adaptable few-shot learning model from the available source domain data sets and cell segmentation tasks. The model can be afterwards fine-tuned on the few annotated images of the target domain that contains different image appearance and different cell type. In our meta-learning training, we propose the combination of three objective functions to segment the cells, move the segmentation results away from the classification boundary using cross-domain tasks, and learn an invariant representation between tasks of the source domains. Our experiments on five public databases show promising results from 1- to 10-shot meta-learning using standard segmentation neural network architectures.
The problem of non-rigid object segmentation is formulated in a two-stage approach in Machine Learning based methodologies. In the first stage, the automatic initialization problem is solved by the estimation of a rigid shape of the object. In the second stage, the non-rigid segmentation is performed. The rational behind this strategy, is that the rigid detection can be performed at lower dimensional space than the original contour space. In this paper, we explore this idea and propose the use of manifolds to reduce even more the dimensionality of the rigid transformation space (first stage) of current state-of-the-art top-down segmentation methodologies. Also, we propose the use of deep belief networks to allow for a training process capable to produce robust appearance models. Experiments in lips segmentation from frontal face images are conducted to testify the performance of the proposed algorithm.
In this paper, we introduce and evaluate the systems submitted to the first Overlapping Cervical Cytology Image Segmentation Challenge, held in conjunction with the IEEE International Symposium on Biomedical Imaging 2014. This challengewas organized to encourage the development and benchmarking of techniques capable of segmenting individual cells from overlapping cellular clumps in cervical cytology images, which is a prerequisite for the development of the next generation of computer-aided diagnosis systems for cervical cancer. In particular, these automated systems must detect and accurately segment both the nucleus and cytoplasm of each cell, even when they are clumped together and, hence, partially occluded. However, this is an unsolved problem due to the poor contrast of cytoplasm boundaries, the large variation in size and shape of cells, and the presence of debris and the large degree of cellular overlap. The challenge initially utilized a database of 16 high-resolution (x40 magnification) images of complex cellular fields of view, in which the isolated real cells were used to construct a database of 945 cervical cytology images synthesized with a varying number of cells and degree of overlap, in order to provide full access of the segmentation ground truth. These synthetic images were used to provide a reliable and comprehensive framework for quantitative evaluation on this segmentation problem. Results from the submitted methods demonstrate that all the methods are effective in the segmentation of clumps containing at most three cells, with overlap coefficients up to 0.3. This highlights the intrinsic difficulty of this challenge and provides motivation for significant future improvement.
The design and use of statistical pattern recognition models can be regarded as one of the core research topics in the segmentation of the left ventricle of the heart from ultrasound data. These models trade a strong prior model of the shape and appearance of the left ventricle for a statistical model whose parameters can be learned using a manually segmented data set (this set is commonly known as the training set). The trouble is that such statistical model is usually quite complex, requiring a large number of parameters that can be robustly learned only if the training set is sufficiently large. The difficulty in obtaining large training sets is currently a major roadblock for the further exploration of statistical models in medical image analysis problems, such as the automatic left ventricle segmentation. In this paper, we present a novel semi-supervised self-training model that reduces the need of large training sets for estimating the parameters of statistical models. This model is initially trained with a small set of manually segmented images, and for each new test sequence, the system reestimates the model parameters incrementally without any further manual intervention. We show that state-of-the-art segmentation results can be achieved with training sets containing 50 annotated examples for the problem of left ventricle segmentation from ultrasound data.
Medical Image Computing and Computer-Assisted Intervention 2023 (MICCAI 2023) The problem of missing modalities is both critical and non-trivial to be handled in multi-modal models. It is common for multi-modal tasks that certain modalities contribute more compared to other modalities, and if those important modalities are missing, the model performance drops significantly. Such fact remains unexplored by current multi-modal approaches that recover the representation from missing modalities by feature reconstruction or blind feature aggregation from other modalities, instead of extracting useful information from the best performing modalities. In this paper, we propose a Learnable Cross-modal Knowledge Distillation (LCKD) model to adaptively identify important modalities and distil knowledge from them to help other modalities from the cross-modal perspective for solving the missing modality issue. Our approach introduces a teacher election procedure to select the most ``qualified'' teachers based on their single modality performance on certain tasks. Then, cross-modal knowledge distillation is performed between teacher and student modalities for each task to push the model parameters to a point that is beneficial for all tasks. Hence, even if the teacher modalities for certain tasks are missing during testing, the available student modalities can accomplish the task well enough based on the learned knowledge from their automatically elected teacher modalities. Experiments on the Brain Tumour Segmentation Dataset 2018 (BraTS2018) shows that LCKD outperforms other methods by a considerable margin, improving the state-of-the-art performance by 3.61% for enhancing tumour, 5.99% for tumour core, and 3.76% for whole tumour in terms of segmentation Dice score.
In this paper, we introduce a novel methodology for characterizing the performance of deep learning networks (ResNets and DenseNet) with respect to training convergence and generalization as a function of mini-batch size and learning rate for image classification. This methodology is based on novel measurements derived from the eigenvalues of the approximate Fisher information matrix, which can be efficiently computed even for high capacity deep models. Our proposed measurements can help practitioners to monitor and control the training process (by actively tuning the mini-batch size and learning rate) to allow for good training convergence and generalization. Furthermore, the proposed measurements also allow us to show that it is possible to optimize the training process with a new dynamic sampling training approach that continuously and automatically change the mini-batch size and learning rate during the training process. Finally, we show that the proposed dynamic sampling training approach has a faster training time and a competitive classification accuracy compared to the current state of the art.
Knee arthroscopy is a minimally invasive surgery used in the treatment of intra-articular knee pathology which may cause unintended damage to femoral cartilage. An ultrasound (US)-guided autonomous robotic platform for knee arthroscopy can be envisioned to minimise these risks and possibly to improve surgical outcomes. The first necessary tool for reliable guidance during robotic surgeries was an automatic segmentation algorithm to outline the regions at risk. In this work, we studied the feasibility of using a state-of-the-art deep neural network (UNet) to automatically segment femoral cartilage imaged with dynamic volumetric US (at the refresh rate of 1 Hz), under simulated surgical conditions. Six volunteers were scanned which resulted in the extraction of 18278 2-D US images from 35 dynamic 3-D US scans, and these were manually labelled. The UNet was evaluated using a five-fold cross-validation with an average of 15531 training and 3124 testing labelled images per fold. An intra-observer study was performed to assess intra-observer variability due to inherent US physical properties. To account for this variability, a novel metric concept named Dice coefficient with boundary uncertainty (DSCUB) was proposed and used to test the algorithm. The algorithm performed comparably to an experienced orthopaedic surgeon, with DSCUB of 0.87. The proposed UNet has the potential to localise femoral cartilage in robotic knee arthroscopy with clinical accuracy.
We introduce a new method to automatically annotate and retrieve images using a vocabulary of image semantics. The novel contributions include a discriminant formulation of the problem, a multiple instance learning solution that enables the estimation of concept probability distributions without prior image segmentation, and a hierarchical description of the density of each image class that enables very efficient training. Compared to current methods of image annotation and retrieval, the one now proposed has significantly smaller time complexity and better recognition performance. Specifically, its recognition complexity is O(CxR), where C is the number of classes (or image annotations) and R is the number of image regions, while the best results in the literature have complexity O(TxR), where T is the number of training images. Since the number of classes grows substantially slower than that of training images, the proposed method scales better during training, and processes test images faster. This is illustrated through comparisons in terms of complexity, time, and recognition performance with current state-of-the-art methods.
Ultrasound imaging is one of the image modalities that can be used for radiation dose guidance during radiotherapy workflows of prostate cancer patients. To allow for image acquisition during the treatment, the ultrasound probe needs to be positioned on the body of the patient before the radiation delivery starts using e.g. a mechanical arm. This is an essential step, as the operator cannot be present in the room when the radiation beam is turned on. Changes in anatomical structures or small motions of the patient during the dose delivery can compromise ultrasound image quality, due to e.g. loss of acoustic coupling or sudden appearance of shadowing artifacts. Currently, an operator is still needed to identify this quality loss. We introduce a prototype deep learning algorithm that can automatically assign a quality score to 2D US images of the male pelvic region based on their usability during an ultrasound guided radiotherapy workflow. It has been shown that the performance of this algorithm is comparable with a medical accredited sonographer and two radiation oncologists.
Computerized algorithms and solutions in processing and diagnosis mammography X-ray, cardiovascular CT/MRI scans, and microscopy image play an important role in disease detection and computer-aided decision-making. Machine learning techniques have powered many aspects in medical investigations and clinical practice. Recently, deep learning is emerging a leading machine learning tool in computer vision and begins attracting considerable attentions in medical imaging. In this chapter, we provide a snapshot of this fast growing field specifically for mammography, cardiovascular, and microscopy image analysis. We briefly explain the popular deep neural networks and summarize current deep learning achievements in various tasks such as detection, segmentation, and classification in these heterogeneous imaging modalities. In addition, we discuss the challenges and the potential future trends for ongoing work.
We Ngo, Tuan Anh a Carneiro, Gustavo segmentation methodology that combines the structured output inference from deep belief networks and the delineation from level set Level set method to produce accurate segmentation of anatomies from medical images. Deep belief networks can be used in the implementation of accurate segmentation models if large annotated training sets are available, but the limited availability of such large datasets in medical image analysis problems motivates the development of methods that can circumvent this demand. In this chapter, we propose the use of level set methods containing several shape and appearance terms, where one of the terms consists of the result from the deep belief network. This combination reduces the demand for large annotated training sets from the deep belief network and at the same time increases the capacity of the level set method to model more effectively the shape and appearance of the visual object of interest. We test our methodology on the Medical Image Computing and Computer Assisted Intervention (MICCAI) 2009 left ventricle segmentation challenge dataset and on Japanese Society of Radiological Technology (JSRT) lung segmentation dataset, where our approach achieves the most accurate results of the field using the semi-automated methodology and state-of-the-art results for the fully automated challenge.
We introduce a new deep convolutional neural network (ConvNet) module that promotes competition amongst a set of convolutional filters of multiple sizes. This new module is inspired by the inception module, where we replace the original collaborative pooling stage (consisting of a concatenation of the multiple size filter outputs) by a competitive pooling represented by a maxout activation unit. This extension has the following two objectives: 1) the selection of the maximum response amongst the multiple size filters prevents filter co-adaptation and allows the formation of multiple sub-networks within the same model, which has been shown to facilitate the training of complex learning problems; and 2) the maxout unit reduces the dimensionality of the outputs from the multiple size filters. We show that the use of our proposed module in typical deep ConvNets produces classification results that are competitive with the state-of-the-art results on the following benchmark datasets: MNIST, CIFAR-10, CIFAR-100, SVHN, and ImageNet ILSVRC 2012. (C) 2017 Elsevier Ltd. All rights reserved.
The design of feature spaces for local image descriptors is an important research subject in computer vision due to its applicability in several problems, such as visual classification and image matching. In order to be useful, these descriptors have to present a good trade off between discriminating power and robustness to typical image deformations. The feature spaces of the most useful local descriptors have been manually designed based on the goal above, but this design often limits the use of these descriptors for some specific matching and visual classification problems. Alternatively, there has been a growing interest in producing feature spaces by an automatic combination of manually designed feature spaces, or by an automatic selection of feature spaces and spatial pooling methods, or by the use of distance metric learning methods. While most of these approaches are usually applied to specific matching or classification problems, where test classes are the same as training classes, a few works aim at the general feature transform problem where the training classes are different from the test classes. The hope in the latter works is the automatic design of a universal feature space for local descriptor matching, which is the topic of our work. In this paper, we propose a new incremental method for learning automatically feature spaces for local descriptors. The method is based on an ensemble of non-linear feature extractors trained in relatively small and random classification problems with supervised distance metric learning techniques. Results on two widely used public databases show that our technique produces competitive results in the field.
The problem of automatic tracking and segmentation of the left ventricle (LV) of the heart from ultrasound images can be formulated with an algorithm that computes the expected segmentation value in the current time step given all previous and current observations using a filtering distribution. This filtering distribution depends on the observation and transition models, and since it is hard to compute the expected value using the whole parameter space of segmentations, one has to resort to Monte Carlo sampling techniques to compute the expected segmentation parameters. Generally, it is straightforward to compute probability values using the filtering distribution, but it is hard to sample from it, which indicates the need to use a proposal distribution to provide an easier sampling method. In order to be useful, this proposal distribution must be carefully designed to represent a reasonable approximation for the filtering distribution. In this paper, we introduce a new LV tracking and segmentation algorithm based on the method described above, where our contributions are focused on a new transition and observation models, and a new proposal distribution. Our tracking and segmentation algorithm achieves better overall results on a previously tested dataset used as a benchmark by the current state-of-the-art tracking algorithms of the left ventricle of the heart from ultrasound images.
Unsupervised anomaly detection (UAD) learns one-class classifiers exclusively with normal (i.e., healthy) images to detect any abnormal (i.e., unhealthy) samples that do not conform to the expected normal patterns. UAD has two main advantages over its fully supervised counterpart. Firstly, it is able to directly leverage large datasets available from health screening programs that contain mostly normal image samples, avoiding the costly manual labelling of abnormal samples and the subsequent issues involved in training with extremely class-imbalanced data. Further, UAD approaches can potentially detect and localise any type of lesions that deviate from the normal patterns. One significant challenge faced by UAD methods is how to learn effective low-dimensional image representations to detect and localise subtle abnormalities, generally consisting of small lesions. To address this challenge, we propose a novel self-supervised representation learning method, called Constrained Contrastive Distribution learning for anomaly detection (CCD), which learns fine-grained feature representations by simultaneously predicting the distribution of augmented data and image contexts using contrastive learning with pretext constraints. The learned representations can be leveraged to train more anomaly-sensitive detection models. Extensive experiment results show that our method outperforms current state-of-the-art UAD approaches on three different colonoscopy and fundus screening datasets. Our code is available at https://github. com tianyu0207/CCD.
Many state-of-the-art noisy-label learning methods rely on learning mechanisms that estimate the samples' clean labels during training and discard their original noisy labels. However, this approach prevents the learning of the relationship between images, noisy labels and clean labels, which has been shown to be useful when dealing with instance-dependent label noise problems. Furthermore, methods that do aim to learn this relationship require cleanly annotated subsets of data, as well as distillation or multi-faceted models for training. In this paper, we propose a new training algorithm that relies on a simple model to learn the relationship between clean and noisy labels without the need for a cleanly labelled subset of data. Our algorithm follows a 3-stage process, namely: 1) self-supervised pre-training followed by an early-stopping training of the classifier to confidently predict clean labels for a subset of the training set; 2) use the clean set from stage (1) to bootstrap the relationship between images, noisy labels and clean labels, which we exploit for effective relabelling of the remaining training set using semi-supervised learning; and 3) supervised training of the classifier with all relabelled samples from stage (2). By learning this relationship, we achieve state-of-the-art performance in asymmetric and instance-dependent label noise problems.
Classification is one of the most studied tasks in data mining and machine learning areas and many works in the literature have been presented to solve classification problems for multiple fields of knowledge such as medicine, biology, security, and remote sensing. Since there is no single classifier that achieves the best results for all kinds of applications, a good alternative is to adopt classifier fusion strategies. A key point in the success of classifier fusion approaches is the combination of diversity and accuracy among classifiers belonging to an ensemble. With a large amount of classification models available in the literature, one challenge is the choice of the most suitable classifiers to compose the final classification system, which generates the need of classifier selection strategies. We address this point by proposing a framework for classifier selection and fusion based on a four-step protocol called CIF-E (Classifiers, Initialization, Fitness function, and Evolutionary algorithm). We implement and evaluate 24 varied ensemble approaches following the proposed CIF-E protocol and we are able to find the most accurate approach. A comparative analysis has also been performed among the best approaches and many other baselines from the literature. The experiments show that the proposed evolutionary approach based on Univariate Marginal Distribution Algorithm (UMDA) can outperform the state-of-the-art literature approaches in many well-known UCI datasets.
We propose a new fully automated non-rigid segmentation approach based on the distance regularized level set method that is initialized and constrained by the results of a structured inference using deep belief networks. This recently proposed level-set formulation achieves reasonably accurate results in several segmentation problems, and has the advantage of eliminating periodic re-initializations during the optimization process, and as a result it avoids numerical errors. Nevertheless, when applied to challenging problems, such as the left ventricle segmentation from short axis cine magnetic ressonance (MR) images, the accuracy obtained by this distance regularized level set is lower than the state of the art. The main reasons behind this lower accuracy are the dependence on good initial guess for the level set optimization and on reliable appearance models. We address these two issues with an innovative structured inference using deep belief networks that produces reliable initial guess and appearance model. The effectiveness of our method is demonstrated on the MICCAI 2009 left ventricle segmentation challenge, where we show that our approach achieves one of the most competitive results (in terms of segmentation accuracy) in the field.
Delivering meaningful uncertainty estimates is essential for a successful deployment of machine learning models in the clinical practice. A central aspect of uncertainty quantification is the ability of a model to return predictions that are well-aligned with the actual probability of the model being correct, also known as model calibration. Although many methods have been proposed to improve calibration, no technique can match the simple, but expensive approach of training an ensemble of deep neural networks. In this paper we introduce a form of simplified ensembling that bypasses the costly training and inference of deep ensembles, yet it keeps its calibration capabilities. The idea is to replace the common linear classifier at the end of a network by a set of heads that are supervised with different loss functions to enforce diversity on their predictions. Specifically, each head is trained to minimize a weighted Cross-Entropy loss, but the weights are different among the different branches. We show that the resulting averaged predictions can achieve excellent calibration without sacrificing accuracy in two challenging datasets for histopathological and endoscopic image classification. Our experiments indicate that Multi-Head Multi-Loss classifiers are inherently well-calibrated, outperforming other recent calibration techniques and even challenging Deep Ensembles' performance. Code to reproduce our experiments can be found at \url{https://github.com/agaldran/mhml_calibration} .
Deep feedforward neural networks with piecewise linear activations are currently producing the state-of-the-art results in several public datasets (e.g., CIFAR-10, CIFAR-100, MNIST, and SVHN). The combination of deep learning models and piecewise linear activation functions allows for the estimation of exponentially complex functions with the use of a large number of subnetworks specialized in the classification of similar input examples. During the training process, these subnetworks avoid overfitting with an implicit regularization scheme based on the fact that they must share their parameters with other subnetworks. Using this framework, we have made an empirical observation that can improve even more the performance of such models. We notice that these models assume a balanced initial distribution of data points with respect to the domain of the piecewise linear activation function. If that assumption is violated, then the piecewise linear activation units can degenerate into purely linear activation units, which can result in a significant reduction of their capacity to learn complex functions. Furthermore, as the number of model layers increases, this unbalanced initial distribution makes the model ill-conditioned. Therefore, we propose the introduction of batch normalisation units into deep feedforward neural networks with piecewise linear activations, which drives a more balanced use of these activation units, where each region of the activation function is trained with a relatively large proportion of training samples. Also, this batch normalisation promotes the pre-conditioning of very deep learning models. We show that by introducing maxout and batch normalisation units to the network in network model results in a model that produces classification results that are better than or comparable to the current state of the art in CIFAR-10, CIFAR-100, MNIST, and SVHN datasets.
The training of deep learning models generally requires a large amount of annotated data for effective convergence and generalisation. However, obtaining high-quality annotations is a laboursome and expensive process due to the need of expert radiologists for the labelling task. The study of semi-supervised learning in medical image analysis is then of crucial importance given that it is much less expensive to obtain unlabelled images than to acquire images labelled by expert radiologists. Essentially, semi-supervised methods leverage large sets of unlabelled data to enable better training convergence and generalisation than using only the small set of labelled images. In this paper, we propose Selfsupervised Mean Teacher for Semi-supervised (S-2 MTS2) learning that combines self-supervised mean-teacher pre-training with semi-supervised fine-tuning. The main innovation of S-2 MTS2 is the self-supervised meanteacher pre-training based on the joint contrastive learning, which uses an infinite number of pairs of positive query and key features to improve the mean-teacher representation. The model is then fine-tuned using the exponential moving average teacher framework trained with semisupervised learning. We validate (SMTS2)-M-2 on the multi-label classification problems from Chest X-ray14 and CheXpert, and the multi-class classification from ISIC2018, where we show that it outperforms the previous SOTA semi-supervised learning methods by a large margin. Our code will be available upon paper acceptance.
Noisy labels are unavoidable yet troublesome in the ecosystem of deep learning because models can easily overfit them. There are many types of label noise, such as symmetric, asymmetric and instance-dependent noise (IDN), with IDN being the only type that depends on image information. Such dependence on image information makes IDN a critical type of label noise to study, given that labelling mistakes are caused in large part by insufficient or ambiguous information about the visual classes present in images. Aiming to provide an effective technique to address IDN, we present a new graphical modelling approach called InstanceGM, that combines discriminative and generative models. The main contributions of InstanceGM are: i) the use of the continuous Bernoulli distribution to train the generative model, offering significant training advantages, and ii) the exploration of a state-of-the-art noisy-label discriminative classifier to generate clean labels from instance-dependent noisy-label samples. InstanceGM is competitive with current noisy-label learning approaches, particularly in IDN benchmarks using synthetic and real-world datasets, where our method shows better accuracy than the competitors in most experiments.
The segmentation of the left ventricle (LV) still constitutes an active research topic in medical image processing field. The problem is usually tackled using pattern recognition methodologies. The main difficulty with pattern recognition methods is its dependence of a large manually annotated training sets for a robust learning strategy. However, in medical imaging, it is difficult to obtain such large annotated data. In this paper, we propose an on-line semi-supervised algorithm capable of reducing the need of large training sets. The main difference regarding semi-supervised techniques is that, the proposed framework provides both an on-line retraining and segmentation, instead of on-line retraining and off-line segmentation. Our proposal is applied to a fully automatic LV segmentation with substantially reduced training sets while maintaining good segmentation accuracy.
Real-world large-scale medical image analysis (MIA) datasets have three challenges: 1) they contain noisy-labelled samples that affect training convergence and generalisation, 2) they usually have an imbalanced distribution of samples per class, and 3) they normally comprise a multi-label problem, where samples can have multiple diagnoses. Current approaches are commonly trained to solve a subset of those problems, but we are unaware of methods that address the three problems simultaneously. In this paper, we propose a new training module called Non-Volatile Unbiased Memory (NVUM), which non-volatility stores running average of model logits for a new regularization loss on noisy multi-label problem. We further unbias the classification prediction in NVUM update for imbalanced learning problem. We run extensive experiments to evaluate NVUM on new benchmarks proposed by this paper, where training is performed on noisy multi-label imbalanced chest X-ray (CXR) training sets, formed by Chest-Xray14 and CheXpert, and the testing is performed on the clean multi-label CXR datasets OpenI and PadChest. Our method outperforms previous state-of-the-art CXR classifiers and previous methods that can deal with noisy labels on all evaluations.
This paper introduces a new semi-automated methodology combining a level set method with a top-down segmentation produced by a deep belief network for the problem of left ventricle segmentation from cardiac magnetic resonance images (MRI). Our approach combines the level set advantages that uses several a priori facts about the object to be segmented (e.g., smooth contour, strong edges, etc.) with the knowledge automatically learned from a manually annotated database (e.g., shape and appearance of the object to be segmented). The use of deep belief networks is justified because of its ability to learn robust models with few annotated images and its flexibility that allowed us to adapt it to a top-down segmentation problem. We demonstrate that our method produces competitive results using the database of the MICCAI grand challenge on left ventricle segmentation from cardiac MRI images, where our methodology produces results on par with the best in the field in each one of the measures used in that challenge (perpendicular distance, Dice metric, and percentage of good detections). Therefore, we conclude that our proposed methodology is one of the most competitive approaches in the field.
When analysing screening mammograms, radiologists can naturally process information across two ipsilateral views of each breast, namely the cranio-caudal (CC) and mediolateral-oblique (MLO) views. These multiple related images provide complementary diagnostic information and can improve the radiologist's classification accuracy. Unfortunately, most existing deep learning systems, trained with globally-labelled images, lack the ability to jointly analyse and integrate global and local information from these multiple views. By ignoring the potentially valuable information present in multiple images of a screening episode, one limits the potential accuracy of these systems. Here, we propose a new multi-view global-local analysis method that mimics the radiologist's reading procedure, based on a global consistency learning and local co-occurrence learning of ipsilateral views in mammograms. Extensive experiments show that our model outperforms competing methods, in terms of classification accuracy and generalisation, on a large-scale private dataset and two publicly available datasets, where models are exclusively trained and tested with global labels.
Mammography, Screening, Convolutional Neural Network (CNN) Published under a CC BY 4.0 license. See also the commentary by Cadrin-Chênevert in this issue.
In generalized zero shot learning (GZSL), the set of classes are split into seen and unseen classes, where training relies on the semantic features of the seen and unseen classes and the visual representations of only the seen classes, while testing uses the visual representations of the seen and unseen classes. Current methods address GZSL by learning a transformation from the visual to the semantic space, exploring the assumption that the distribution of classes in the semantic and visual spaces is relatively similar. Such methods tend to transform unseen testing visual representations into one of the seen classes' semantic features instead of the semantic features of the correct unseen class, resulting in low accuracy GZSL classification. Recently, generative adversarial networks (GAN) have been explored to synthesize visual representations of the unseen classes from their semantic features - the synthesized representations of the seen and unseen classes are then used to train the GZSL classifier. This approach has been shown to boost GZSL classification accuracy, but there is one important missing constraint: there is no guarantee that synthetic visual representations can generate back their semantic feature in a multi-modal cycle-consistent manner. This missing constraint can result in synthetic visual representations that do not represent well their semantic features, which means that the use of this constraint can improve GAN-based approaches. In this paper, we propose the use of such constraint based on a new regularization for the GAN training that forces the generated visual features to reconstruct their original semantic features. Once our model is trained with this multi-modal cycle-consistent semantic compatibility, we can then synthesize more representative visual representations for the seen and, more importantly, for the unseen classes. Our proposed approach shows the best GZSL classification results in the field in several publicly available datasets.
We propose a new method for breast cancer screening from DCE-MRI based on a post-hoc approach that is trained using weakly annotated data (i.e., labels are available only at the image level without any lesion delineation). Our proposed post-hoc method automatically diagnosis the whole volume and, for positive cases, it localizes the malignant lesions that led to such diagnosis. Conversely, traditional approaches follow a pre-hoc approach that initially localises suspicious areas that are subsequently classified to establish the breast malignancy - this approach is trained using strongly annotated data (i.e., it needs a delineation and classification of all lesions in an image). We also aim to establish the advantages and disadvantages of both approaches when applied to breast screening from DCE-MRI. Relying on experiments on a breast DCE-MRI dataset that contains scans of 117 patients, our results show that the post-hoc method is more accurate for diagnosing the whole volume per patient, achieving an AUC of 0.91, while the pre-hoc method achieves an AUC of 0.81. However, the performance for localising the malignant lesions remains challenging for the post-hoc method due to the weakly labelled dataset employed during training. (C) 2019 Elsevier B.V. All rights reserved.
Despite the complicated structure of modern deep neural network architectures, they are still optimized with algorithms based on Stochastic Gradient Descent (SGD). However, the reason behind the effectiveness of SGD is not well understood, making its study an active research area. In this paper, we formulate deep neural network optimization as a dynamical system and show that the rigorous theory developed to study chaotic systems can be useful to understand SGD and its variants. In particular, we first observe that the inverse of the instability timescale of SGD optimization, represented by the largest Lyapunov exponent, corresponds to the most negative eigenvalue of the Hessian of the loss. This observation enables the introduction of an efficient method to estimate the largest eigenvalue of the Hessian. Then, we empirically show that for a large range of learning rates, SGD traverses the loss landscape across regions with largest eigenvalue of the Hessian similar to the inverse of the learning rate. This explains why effective learning rates can be found to be within a large range of values and shows that SGD implicitly uses the largest eigenvalue of the Hessian while traversing the loss landscape. This sheds some light on the effectiveness of SGD over more sophisticated second-order methods. We also propose a quasi-Newton method that dynamically estimates an optimal learning rate for the optimization of deep learning models. We demonstrate that our observations and methods are robust across different architectures and loss functions on CIFAR-10 dataset.
Painted tile panels (Azulejos) are one of the most representative Portuguese forms of art. Most of these panels are inspired on, and sometimes are literal copies of, famous paintings, or prints of those paintings. In order to study the Azulejos, art historians need to trace these roots. To do that they manually search art image databases, looking for images similar to the representation on the tile panel. This is an overwhelming task that should be automated as much as possible. Among several cues, the pose of humans and the general composition of people in a scene is quite discriminative. We build an image descriptor, combining the kinematic chain of each character, and contextual information about their composition, in the scene. Given a query image, our system computes its similarity profile over the database. Using nearest neighbors in the space of the descriptors, the proposed system retrieves the prints that most likely inspired the tiles' work.
Deep neural networks currently deliver promising results for microscopy image cell segmentation, but they require large-scale labelled databases, which is a costly and time-consuming process. In this work, we relax the labelling requirement by combining self-supervised with semi-supervised learning. We propose the prediction of edge-based maps for self-supervising the training of the unlabelled images, which is combined with the supervised training of a small number of labelled images for learning the segmentation task. In our experiments, we evaluate on a few-shot microscopy image cell segmentation benchmark and show that only a small number of annotated images, e.g. 10% of the original training set, is enough for our approach to reach similar performance as with the fully annotated databases on 1- to 10-shots. Our code and trained models is made publicly available
Endometriosis is a common chronic gynecological disorder that has many characteristics, including the pouch of Douglas (POD) obliteration, which can be diagnosed using Transvaginal gynecological ultrasound (TVUS) scans and magnetic resonance imaging (MRI). TVUS and MRI are complementary non-invasive endometriosis diagnosis imaging techniques, but patients are usually not scanned using both modalities and, it is generally more challenging to detect POD obliteration from MRI than TVUS. To mitigate this classification imbalance, we propose in this paper a knowledge distillation training algorithm to improve the POD obliteration detection from MRI by leveraging the detection results from unpaired TVUS data. More specifically, our algorithm pre-trains a teacher model to detect POD obliteration from TVUS data, and it also pre-trains a student model with 3D masked autoencoder using a large amount of unlabelled pelvic 3D MRI volumes. Next, we distill the knowledge from the teacher TVUS POD obliteration detector to train the student MRI model by minimizing a regression loss that approximates the output of the student to the teacher using unpaired TVUS and MRI data. Experimental results on our endometriosis dataset containing TVUS and MRI data demonstrate the effectiveness of our method to improve the POD detection accuracy from MRI.
Background and Aims: Endoscopy guidelines recommend adhering to policies such as resect and discard only if the optical biopsy is accurate. However, accuracy in predicting histology can vary greatly. Computer-aided diagnosis (CAD) for characterization of colorectal lesions may help with this issue. In this study, CAD software developed at the University of Adelaide (Australia) that includes serrated polyp differentiation was validated with Japanese images on narrow-band imaging (NBI) and blue-laser imaging (BLI). Methods: CAD software developed using machine learning and densely connected convolutional neural net-works was modeled with NBI colorectal lesion images (Olympus 190 series - Australia) and validated for NBI (Olympus 290 series) and BLI (Fujifilm 700 series) with Japanese datasets. All images were correlated with histology according to the modified Sano classification. The CAD software was trained with Australian NBI images and tested with separate sets of images from Australia (NBI) and Japan (NBI and BLI). Results: An Australian dataset of 1235 polyp images was used as training, testing, and internal validation sets. A Japanese dataset of 20 polyp images on NBI and 49 polyp images on BLI was used as external validation sets. The CAD software had a mean area under the curve (AUC) of 94.3% for the internal set and 84.5% and 90.3% for the external sets (NBI and BLI, respectively). Conclusions: The CAD achieved AUCs comparable with experts and similar results with NBI and BLI. Accurate CAD prediction was achievable, even when the predicted endoscopy imaging technology was not part of the training set.
Cardiac magnetic resonance (CMR) is used extensively in the diagnosis and management of cardiovascular disease. Deep learning methods have proven to deliver segmentation results comparable to human experts in CMR imaging, but there have been no convincing results for the problem of end-to-end segmentation and diagnosis from CMR. This is in part due to a lack of sufficiently large datasets required to train robust diagnosis models. In this paper, we propose a learning method to train diagnosis models, where our approach is designed to work with relatively small datasets. In particular, the optimization loss is based on multi-task learning that jointly trains for the tasks of segmentation and diagnosis classification. We hypothesize that segmentation has a regularizing effect on the learning of features relevant for diagnosis. Using the 100 training and 50 testing samples available from the Automated Cardiac Diagnosis Challenge (ACDC) dataset, which has a balanced distribution of 5 cardiac diagnoses, we observe a reduction of the classification error from 32% to 22%, and a faster convergence compared to a baseline without segmentation. To the best of our knowledge, this is the best diagnosis results from CMR using an end-to-end diagnosis and segmentation learning method.
The training of medical image analysis systems using machine learning approaches follows a common script: collect and annotate a large dataset, train the classifier on the training set, and test it on a hold-out test set. This process bears no direct resemblance with radiologist training, which is based on solving a series of tasks of increasing difficulty, where each task involves the use of significantly smaller datasets than those used in machine learning. In this paper, we propose a novel training approach inspired by how radiologists are trained. In particular, we explore the use of meta-training that models a classifier based on a series of tasks. Tasks are selected using teacher-student curriculum learning, where each task consists of simple classification problems containing small training sets. We hypothesize that our proposed meta-training approach can be used to pre-train medical image analysis models. This hypothesis is tested on the automatic breast screening classification from DCE-MRI trained with weakly labeled datasets. The classification performance achieved by our approach is shown to be the best in the field for that application, compared to state of art baseline approaches: DenseNet, multiple instance learning and multi-task learning.
Artificial intelligence (AI) is increasingly being used in medical imaging analysis. We aimed to evaluate the diagnostic accuracy of AI models used for detection of lymph node metastasis on pre-operative staging imaging for colorectal cancer. A systematic review was conducted according to PRISMA guidelines using a literature search of PubMed (MEDLINE), EMBASE, IEEE Xplore and the Cochrane Library for studies published from January 2010 to October 2020. Studies reporting on the accuracy of radiomics models and/or deep learning for the detection of lymph node metastasis in colorectal cancer by CT/MRI were included. Conference abstracts and studies reporting accuracy of image segmentation rather than nodal classification were excluded. The quality of the studies was assessed using a modified questionnaire of the QUADAS-2 criteria. Characteristics and diagnostic measures from each study were extracted. Pooling of area under the receiver operating characteristic curve (AUROC) was calculated in a meta-analysis. Seventeen eligible studies were identified for inclusion in the systematic review, of which 12 used radiomics models and five used deep learning models. High risk of bias was found in two studies and there was significant heterogeneity among radiomics papers (73.0%). In rectal cancer, there was a per-patient AUROC of 0.808 (0.739-0.876) and 0.917 (0.882-0.952) for radiomics and deep learning models, respectively. Both models performed better than the radiologists who had an AUROC of 0.688 (0.603 to 0.772). Similarly in colorectal cancer, radiomics models with a per-patient AUROC of 0.727 (0.633-0.821) outperformed the radiologist who had an AUROC of 0.676 (0.627-0.725). AI models have the potential to predict lymph node metastasis more accurately in rectal and colorectal cancer, however, radiomics studies are heterogeneous and deep learning studies are scarce. PROSPERO CRD42020218004 .
Overall survival (OS) time prediction is one of the most common estimates of the prognosis of gliomas and is used to design an appropriate treatment planning. State-of-the-art (SOTA) methods for OS time prediction follow a pre-hoc approach that require computing the segmentation map of the glioma tumor sub-regions (necrotic, edema tumor, enhancing tumor) for estimating OS time. However, the training of the segmentation methods require ground truth segmentation labels which are tedious and expensive to obtain. Given that most of the large-scale data sets available from hospitals are unlikely to contain such precise segmentation, those SOTA methods have limited applicability. In this paper, we introduce a new post-hoc method for OS time prediction that does not require segmentation map annotation for training. Our model uses medical image and patient demographics (represented by age) as inputs to estimate the OS time and to estimate a saliency map that localizes the tumor as a way to explain the OS time prediction in a post-hoc manner. It is worth emphasizing that although our model can localize tumors, it uses only the ground truth OS time as training signal, i.e., no segmentation labels are needed. We evaluate our post-hoc method on the Multimodal Brain Tumor Segmentation Challenge (BraTS) 2019 data set and show that it achieves competitive results compared to prehoc methods with the advantage of not requiring segmentation labels for training. We make our code available at https://github.com/renato145/posthocOS.
Multi-modal learning focuses on training models by equally combining multiple input data modalities during the prediction process. However, this equal combination can be detrimental to the prediction accuracy because different modalities are usually accompanied by varying levels of uncertainty. Using such uncertainty to combine modalities has been studied by a couple of approaches, but with limited success because these approaches are either designed to deal with specific classification or segmentation problems and cannot be easily translated into other tasks, or suffer from numerical instabilities. In this paper, we propose a new Uncertainty-aware Multi-modal Learner that estimates uncertainty by measuring feature density via Cross-modal Random Network Prediction (CRNP). CRNP is designed to require little adaptation to translate between different prediction tasks, while having a stable training process. From a technical point of view, CRNP is the first approach to explore random network prediction to estimate uncertainty and to combine multi-modal data. Experiments on two 3D multi-modal medical image segmentation tasks and three 2D multi-modal computer vision classification tasks show the effectiveness, adaptability and robustness of CRNP. Also, we provide an extensive discussion on different fusion functions and visualization to validate the proposed model.
The solution for the top-down segmentation of non-rigid visual objects using machine learning techniques is generally regarded as too complex to be solved in its full generality given the large dimensionality of the search space of the explicit representation of the segmentation contour. In order to reduce this complexity, the problem is usually divided into two stages: rigid detection and non-rigid segmentation. The rationale is based on the fact that the rigid detection can be run in a lower dimensionality space (i.e., less complex and faster) than the original contour space, and its result is then used to constrain the non-rigid segmentation. In this paper, we propose the use of sparse manifolds to reduce the dimensionality of the rigid detection search space of current state-of-the-art top-down segmentation methodologies. The main goals targeted by this smaller dimensionality search space are the decrease of the search running time complexity and the reduction of the training complexity of the rigid detector. These goals are attainable given that both the search and training complexities are function of the dimensionality of the rigid search space. We test our approach in the segmentation of the left ventricle from ultrasound images and lips from frontal face images. Compared to the performance of state-of-the-art non-rigid segmentation system, our experiments show that the use of sparse manifolds for the rigid detection leads to the two goals mentioned above.
Malignant tumors that contain a high proportion of regions deprived of adequate oxygen supply (hypoxia) in areas supplied by a microvessel (i.e., a microcirculatory supply unit - MCSU) have been shown to present resistance to common cancer treatments. Given the importance of the estimation of this proportion for improving the clinical prognosis of such treatments, a manual annotation has been proposed, which uses two image modalities of the same histological specimen and produces the number and proportion of MCSUs classified as normoxia (normal oxygenation level), chronic hypoxia (limited diffusion), and acute hypoxia (transient disruptions in perfusion), but this manual annotation requires an expertise that is generally not available in clinical settings. Therefore, in this paper, we propose a new methodology that automates this annotation. The major challenge is that the training set comprises weakly labeled samples that only contains the number of MCSU types per sample, which means that we do not have the underlying structure of MCSU locations and classifications. Hence, we formulate this problem as a latent structured output learning that minimizes a high order loss function based on the number of MCSU types, where the underlying MCSU structure is flexible in terms of number of nodes and connections. Using a database of 89 pairs of weakly annotated images (from eight tumors), we show that our methodology produces highly correlated number and proportion of MCSU types compared to the manual annotations.
The automatic segmentation of the left ventricle of the heart in ultrasound images has been a core research topic in medical image analysis. Most of the solutions are based on low-level segmentation methods, which uses a prior model of the appearance of the left ventricle, but imaging conditions violating the assumptions present in the prior can damage their performance. Recently, pattern recognition methods have become more robust to imaging conditions by automatically building an appearance model from training images, but they present a few challenges, such as: the need of a large set of training images, robustness to imaging conditions not present in the training data, and complex search process. In this paper we handle the second problem using the recently proposed deep neural network and the third problem with efficient searching algorithms. Quantitative comparisons show that the accuracy of our approach is higher than state-of-the-art methods. The results also show that efficient search strategies reduce ten times the run-time complexity.
The study of the visual art of printmaking is fundamental for art history. Printmaking methods have been used for centuries to replicate visual art works, which have influenced generations of artists. Particularly in this work, we are interested in the influence of prints on artistic tile panel painters, who have produced an impressive body of work in Portugal. The study of such panels has gained interest by art historians, who try to understand the influence of prints on tile panels artists in order to understand the evolution of this type of visual arts. Several databases of digitized art images have been used for such end, but the use of these databases relies on manual image annotations, an effective internal organization, and an ability of the art historian to visually recognize relevant prints. We propose an automation of these tasks using statistical pattern recognition techniques that takes into account not only the manual annotations available, but also the visual characteristics of the images. Specifically, we introduce a new network link-analysis method for the automatic annotation and retrieval of digital images of prints. Using a database of 307 annotated images of prints, we show that the annotation and retrieval results produced by our approach are better than the results of state-of-the-art content-based image retrieval methods.
The early detection of glaucoma is essential in preventing visual impairment. Artificial intelligence (AI) can be used to analyze color fundus photographs (CFPs) in a cost-effective manner, making glaucoma screening more accessible. While AI models for glaucoma screening from CFPs have shown promising results in laboratory settings, their performance decreases significantly in real-world scenarios due to the presence of out-of-distribution and low-quality images. To address this issue, we propose the Artificial Intelligence for Robust Glaucoma Screening (AIROGS) challenge. This challenge includes a large dataset of around 113,000 images from about 60,000 patients and 500 different screening centers, and encourages the development of algorithms that are robust to ungradable and unexpected input data. We evaluated solutions from 14 teams in this paper, and found that the best teams performed similarly to a set of 20 expert ophthalmologists and optometrists. The highest-scoring team achieved an area under the receiver operating characteristic curve of 0.99 (95% CI: 0.98-0.99) for detecting ungradable images on-the-fly. Additionally, many of the algorithms showed robust performance when tested on three other publicly available datasets. These results demonstrate the feasibility of robust AI-enabled glaucoma screening.
This paper compares well-established Convolutional Neural Networks (CNNs) to recently introduced Vision Transformers for the task of Diabetic Foot Ulcer Classification, in the context of the DFUC 2021 Grand-Challenge, in which this work attained the first position. Comprehensive experiments demonstrate that modern CNNs are still capable of outperforming Transformers in a low-data regime, likely owing to their ability for better exploiting spatial correlations. In addition, we empirically demonstrate that the recent Sharpness-Aware Minimization (SAM) optimization algorithm improves considerably the generalization capability of both kinds of models. Our results demonstrate that for this task, the combination of CNNs and the SAM optimization process results in superior performance than any other of the considered approaches.
This book constitutes the refereed proceedings of two workshops held at the 19th International Conference on Medical Image Computing and Computer-Assisted Intervention, MICCAI 2016, in Athens, Greece, in October 2016: the First Workshop on Large-Scale Annotation of Biomedical Data and Expert Label Synthesis, LABELS 2016, and the Second International Workshop on Deep Learning in Medical Image Analysis, DLMIA 2016. The 28 revised regular papers presented in this book were carefully reviewed and selected from a total of 52 submissions. The 7 papers selected for LABELS deal with topics from the following fields: crowd-sourcing methods; active learning; transfer learning; semi-supervised learning; and modeling of label uncertainty. The 21 papers selected for DLMIA span a wide range of topics such as image description; medical imaging-based diagnosis; medical signal-based diagnosis; medical image reconstruction and model selection using deep learning techniques; meta-heuristic techniques for fine-tuning parameter in deep learning-based architectures; and applications based on deep learning techniques.
When analysing screening mammograms, radiologists can naturally process information across two ipsilateral views of each breast, namely the cranio-caudal (CC) and mediolateral-oblique (MLO) views. These multiple related images provide complementary diagnostic information and can improve the radiologist's classification accuracy. Unfortunately, most existing deep learning systems, trained with globally-labelled images, lack the ability to jointly analyse and integrate global and local information from these multiple views. By ignoring the potentially valuable information present in multiple images of a screening episode, one limits the potential accuracy of these systems. Here, we propose a new multi-view global-local analysis method that mimics the radiologist's reading procedure, based on a global consistency learning and local co-occurrence learning of ipsilateral views in mammograms. Extensive experiments show that our model outperforms competing methods, in terms of classification accuracy and generalisation, on a large-scale private dataset and two publicly available datasets, where models are exclusively trained and tested with global labels.
In this paper, we propose a multi-view deep residual neural network (mResNet) for the fully automated classification of mammograms as either malignant or normal/benign. Specifically, our mResNet approach consists of an ensemble of deep residual networks (ResNet), which have six input images, including the unregistered craniocaudal (CC) and mediolateral oblique (MLO) mammogram views as well as the automatically produced binary segmentation maps of the masses and micro-calcifications in each view. We then form the mResNet by concatenating the outputs of each ResNet at the second to last layer, followed by a final, fully connected, layer. The resulting mResNet is trained in an end-to-end fashion to produce a case-based mammogram classifier that has the potential to be used in breast screening programs. We empirically show on the publicly available INbreast dataset, that the proposed mResNet classifies mammograms into malignant or normal/benign with an AUC of 0.8.
To solve deep metric learning problems and producing feature embeddings, current methodologies will commonly use a triplet model to minimise the relative distance between samples from the same class and maximise the relative distance between samples from different classes. Though successful, the training convergence of this triplet model can be compromised by the fact that the vast majority of the training samples will produce gradients with magnitudes that are close to zero. This issue has motivated the development of methods that explore the global structure of the embedding and other methods that explore hard negative/positive mining. The effectiveness of such mining methods is often associated with intractable computational requirements. In this paper, we propose a novel deep metric learning method that combines the triplet model and the global structure of the embedding space. We rely on a smart mining procedure that produces effective training samples for a low computational cost. In addition, we propose an adaptive controller that automatically adjusts the smart mining hyper-parameters and speeds up the convergence of the training process. We show empirically that our proposed method allows for fast and more accurate training of triplet ConvNets than other competing mining methods. Additionally, we show that our method achieves new state-of-the-art embedding results for CUB-200-2011 and Cars196 datasets.
Audio-visual segmentation (AVS) is a complex task that involves accurately segmenting the corresponding sounding object based on audio-visual queries. Successful audio-visual learning requires two essential components: 1) an unbiased dataset with high-quality pixel-level multi-class labels, and 2) a model capable of effectively linking audio information with its corresponding visual object. However, these two requirements are only partially addressed by current methods, with training sets containing biased audio-visual data, and models that generalise poorly beyond this biased training set. In this work, we propose a new strategy to build cost-effective and relatively unbiased audio-visual semantic segmentation benchmarks. Our strategy, called Visual Post-production (VPO), explores the observation that it is not necessary to have explicit audio-visual pairs extracted from single video sources to build such benchmarks. We also refine the previously proposed AVSBench to transform it into the audio-visual semantic segmentation benchmark AVSBench-Single+. Furthermore, this paper introduces a new pixel-wise audio-visual contrastive learning method to enable a better generalisation of the model beyond the training set. We verify the validity of the VPO strategy by showing that state-of-the-art (SOTA) models trained with datasets built by matching audio and visual data from different sources or with datasets containing audio and visual data from the same video source produce almost the same accuracy. Then, using the proposed VPO benchmarks and AVSBench-Single+, we show that our method produces more accurate audio-visual semantic segmentation than SOTA models. Code and dataset will be available.
In this paper, we propose new prognostic methods that predict 5-year mortality in elderly individuals using chest computed tomography (CT). The methods consist of a classifier that performs this prediction using a set of features extracted from the CT image and segmentation maps of multiple anatomic structures. We explore two approaches: 1) a unified framework based on two state-of-the-art deep learning models extended to 3-D inputs, where features and classifier are automatically learned in a single optimisation process; and 2) a multi-stage framework based on the design and selection and extraction of hand-crafted radiomics features, followed by the classifier learning process. Experimental results, based on a dataset of 48 annotated chest CTs, show that the deep learning models produces a mean 5-year mortality prediction AUC in [68.8%,69.8%] and accuracy in [64.5%,66.5%], while radiomics produces a mean AUC of 64.6% and accuracy of 64.6%. The successful development of the proposed models has the potential to make a profound impact in preventive and personalised healthcare.
Survival time prediction from medical images is important for treatment planning, where accurate estimations can improve healthcare quality. One issue affecting the training of survival models is censored data. Most of the current survival prediction approaches are based on Cox models that can deal with censored data, but their application scope is limited because they output a hazard function instead of a survival time. On the other hand, methods that predict survival time usually ignore censored data, resulting in an under-utilization of the training set. In this work, we propose a new training method that predicts survival time using all censored and uncensored data. We propose to treat censored data as samples with a lower-bound time to death and estimate pseudo labels to semi-supervise a censor-aware survival time regressor. We evaluate our method on pathology and x-ray images from the TCGA-GM and NLST datasets. Our results establish the state-of-the-art survival prediction accuracy on both datasets.
We propose a probabilistic, hierarchical, and discriminant (PHD) framework for fast and accurate detection of deformable anatomic structures from medical images. The PHD framework has three characteristics. First, it integrates distinctive primitives of the anatomic structures at global, segmental, and landmark levels in a probabilistic manner. Second, since the configuration of the anatomic structures lies in a high-dimensional parameter space, it seeks the best configuration via a hierarchical evaluation of the detection probability that quickly prunes the search space. Finally, to separate the primitive from the background, it adopts a discriminative boosting learning implementation. We apply the PHD framework for accurately detecting various deformable anatomic structures from M- mode and Doppler echocardiograms in about a second.
In this paper, we propose a new method for the segmentation of breast masses from mammograms using a conditional random field (CRF) model that combines several types of potential functions, including one that classifies image regions using deep learning. The inference method used in this model is the tree re-weighted (TRW) belief propagation, which allows a learning mechanism that directly minimizes the mass segmentation error and an inference approach that produces an optimal result under the approximations of the TRW formulation. We show that the use of these inference and learning mechanisms and the deep learning potential functions provides gains in terms of accuracy and efficiency in comparison with the current state of the art using the publicly available datasets INbreast and DDSM-BCRP.
Learning from noisy labels (LNL) plays a crucial role in deep learning. The most promising LNL methods rely on identifying clean-label samples from a dataset with noisy annotations. Such an identification is challenging because the conventional LNL problem, which assumes a single noisy label per instance, is non-identifiable, i.e., clean labels cannot be estimated theoretically without additional heuristics. In this paper, we aim to formally investigate this identifiability issue using multinomial mixture models to determine the constraints that make the problem identifiable. Specifically, we discover that the LNL problem becomes identifiable if there are at least $2C - 1$ noisy labels per instance, where $C$ is the number of classes. To meet this requirement without relying on additional $2C - 2$ manual annotations per instance, we propose a method that automatically generates additional noisy labels by estimating the noisy label distribution based on nearest neighbours. These additional noisy labels enable us to apply the Expectation-Maximisation algorithm to estimate the posterior probabilities of clean labels, which are then used to train the model of interest. We empirically demonstrate that our proposed method is capable of estimating clean labels without any heuristics in several label noise benchmarks, including synthetic, web-controlled, and real-world label noises. Furthermore, our method performs competitively with many state-of-the-art methods.
Objective. We compared the performance between sonographers and automated fetal biometry measurements (Auto OB) with respect to the following measurements: biparietal diameter (BPD), head circumference (HC), abdominal circumference (AC) and femur length (FL). Methods. The first set of experiments involved assessing the performance of Auto OB relative to the five sonographers, using 240 images for each user. Each sonographer made measurements in 80 images per anatomy. The second set of experiments compared the performance of Auto OB with respect to the data generated by the five sonographers for inter-observer variability (i.e., sonographers and clinicians) using a set of 10 images per anatomy. Results. Auto OB correlated well with manual measurements for BPD, HC, AC and FL (r > 0.98, p < 0.001 for all measurements). The errors produced by Auto OB for BPD is 1.46% (σ = 1.74%), where σ denotes standard deviation), for HC is 1.25% (σ = 1.34%), for AC is 3% (σ = 6.16%) and for FL is 3.52% (σ = 3.72%). In general, these errors represent deviations of less than 3 days for fetuses younger than 30 weeks, and less than 7 days for fetuses between 30 and 40 weeks of age. Conclusion. The measurements produced by Auto OB are comparable to the measurements done by sonographers.
We introduce Probabilistic Object Detection, the task of detecting objects in images and accurately quantifying the spatial and semantic uncertainties of the detections. Given the lack of methods capable of assessing such probabilistic object detections, we present the new Probability-based Detection Quality measure (PDQ). Unlike AP-based measures, PDQ has no arbitrary thresholds and rewards spatial and label quality, and foreground/background separation quality while explicitly penalising false positive and false negative detections. We contrast PDQ with existing mAP and moLRP measures by evaluating state-of-the-art detectors and a Bayesian object detector based on Monte Carlo Dropout. Our experiments indicate that conventional object detectors tend to be spatially overconfident and thus perform poorly on the task of probabilistic object detection. Our paper aims to encourage the development of new object detection approaches that provide detections with accurately estimated spatial and label uncertainties and are of critical importance for deployment on robots and embodied AI systems in the real world.
Noisy labels present a significant challenge in deep learning because models are prone to overfitting. This problem has driven the development of sophisticated techniques to address the issue, with one critical component being the selection of clean and noisy label samples. Selecting noisy label samples is commonly based on the small-loss hypothesis or on feature-based sampling, but we present empirical evidence that shows that both strategies struggle to differentiate between noisy label and hard samples, resulting in relatively large proportions of samples falsely selected as clean. To address this limitation, we propose a novel peer-agreement based sample selection (PASS). An automated thresholding technique is then applied to the agreement score to select clean and noisy label samples. PASS is designed to be easily integrated into existing noisy label robust frameworks, and it involves training a set of classifiers in a round-robin fashion, with peer models used for sample selection. In the experiments, we integrate our PASS with several state-of-the-art (SOTA) models, including InstanceGM, DivideMix, SSR, FaMUS, AugDesc, and C2D, and evaluate their effectiveness on several noisy label benchmark datasets, such as CIFAR-100, CIFAR-N, Animal-10N, Red Mini-Imagenet, Clothing1M, Mini-Webvision, and Imagenet. Our results demonstrate that our new sample selection approach improves the existing SOTA results of algorithms.
•The femoral condyle cartilage is one of the structure most at risk during knee arthroscopy.•The first methodology to track in real-time the femoral condyle cartilage in ultrasound images.•Effective combination of a neural network architecture for medical image segmentation and the siamese framework for visual tracking.•Tracking performance comparable to two experienced surgeons.•Outperforming state-of-the-art segmentation models and trackers in the tracking of the femoral cartilage. [Display omitted] The tracking of the knee femoral condyle cartilage during ultrasound-guided minimally invasive procedures is important to avoid damaging this structure during such interventions. In this study, we propose a new deep learning method to track, accurately and efficiently, the femoral condyle cartilage in ultrasound sequences, which were acquired under several clinical conditions, mimicking realistic surgical setups. Our solution, that we name Siam-U-Net, requires minimal user initialization and combines a deep learning segmentation method with a siamese framework for tracking the cartilage in temporal and spatio-temporal sequences of 2D ultrasound images. Through extensive performance validation given by the Dice Similarity Coefficient, we demonstrate that our algorithm is able to track the femoral condyle cartilage with an accuracy which is comparable to experienced surgeons. It is additionally shown that the proposed method outperforms state-of-the-art segmentation models and trackers in the localization of the cartilage. We claim that the proposed solution has the potential for ultrasound guidance in minimally invasive knee procedures.
We introduce two new structured output models that use a latent graph, which is flexible in terms of the number of nodes and structure, where the training process minimises a high-order loss function using a weakly annotated training set. These models are developed in the context of microscopy imaging of malignant tumours, where the estimation of the number and proportion of classes of microcirculatory supply units (MCSU) is important in the assessment of the efficacy of common cancer treatments (an MCSU is a region of the tumour tissue supplied by a microvessel). The proposed methodologies take as input multimodal microscopy images of a tumour, and estimate the number and proportion of MCSU classes. This estimation is facilitated by the use of an underlying latent graph (not present in the manual annotations), where each MCSU is represented by a node in this graph, labelled with the MCSU class and image location. The training process uses the manual weak annotations available, consisting of the number of MCSU classes per training image, where the training objective is the minimisation of a high-order loss function based on the norm of the error between the manual and estimated annotations. One of the models proposed is based on a new flexible latent structure support vector machine (FLSSVM) and the other is based on a deep convolutional neural network (DCNN) model. Using a dataset of 89 weakly annotated pairs of multimodal images from eight tumours, we show that the quantitative results from DCNN are superior, but the qualitative results from FLSSVM are better and both display high correlation values regarding the number and proportion of MCSU classes compared to the manual annotations.
Longitudinal imaging forms an essential component in the management and follow-up of many medical conditions. The presence of lesion changes on serial imaging can have significant impact on clinical decision making, highlighting the important role for automated change detection. Lesion changes can represent anomalies in serial imaging, which implies a limited availability of annotations and a wide variety of possible changes that need to be considered. Hence, we introduce a new unsupervised anomaly detection and localisation method trained exclusively with serial images that do not contain any lesion changes. Our training automatically synthesises lesion changes in serial images, introducing detection and localisation pseudo-labels that are used to self-supervise the training of our model. Given the rarity of these lesion changes in the synthesised images, we train the model with the imbalance robust focal Tversky loss. When compared to supervised models trained on different datasets, our method shows competitive performance in the detection and localisation of new demyelinating lesions on longitudinal magnetic resonance imaging in multiple sclerosis patients. Code for the models will be made available at https://github.com/toson87/MSChangeDetection.
Through the years, several CAD systems have been developed to help radiologists in the hard task of detecting signs of cancer in mammograms. In these CAD systems, mass segmentation plays a central role in the decision process. In the literature, mass segmentation has been typically evaluated in a intra-sensor scenario, where the methodology is designed and evaluated in similar data. However, in practice, acquisition systems and PACS from multiple vendors abound and current works fails to take into account the differences in mammogram data in the performance evaluation. In this work it is argued that a comprehensive assessment of the mass segmentation methods requires the design and evaluation in datasets with different properties. To provide a more realistic evaluation, this work proposes: a) improvements to a state of the art method based on tailored features and a graph model; b) a head-to-head comparison of the improved model with recently proposed methodologies based in deep learning and structured prediction on four reference databases, performing a cross-sensor evaluation. The results obtained support the assertion that the evaluation methods from the literature are optimistically biased when evaluated on data gathered from exactly the same sensor and/or acquisition protocol.
We introduce a new, rigorously-formulated Bayesian meta-learning algorithm that learns a probability distribution of model parameter prior for few-shot learning. The proposed algorithm employs a gradient-based variational inference to infer the posterior of model parameters for a new task. Our algorithm can be applied to any model architecture and can be implemented in various machine learning paradigms, including regression and classification. We show that the models trained with our proposed meta-learning algorithm are well calibrated and accurate, with state-of-the-art calibration and classification results on three few-shot classification benchmarks (Om- niglot, mini-ImageNet and tiered-ImageNet), and competitive results in a multi-modal task-distribution regression.
State-of-the-art (SOTA) anomaly segmentation approaches on complex urban driving scenes explore pixel-wise classification uncertainty learned from outlier exposure, or external reconstruction models. However, previous uncertainty approaches that directly associate high uncertainty to anomaly may sometimes lead to incorrect anomaly predictions, and external reconstruction models tend to be too inefficient for real-time self-driving embedded systems. In this paper, we propose a new anomaly segmentation method, named pixel-wise energy-biased abstention learning (PEBAL), that explores pixel-wise abstention learning (AL) with a model that learns an adaptive pixel-level anomaly class, and an energy-based model (EBM) that learns inlier pixel distribution. More specifically, PEBAL is based on a non-trivial joint training of EBM and AL, where EBM is trained to output high-energy for anomaly pixels (from outlier exposure) and AL is trained such that these high-energy pixels receive adaptive low penalty for being included to the anomaly class. We extensively evaluate PEBAL against the SOTA and show that it achieves the best performance across four benchmarks. Code is available at https://github.com/tianyu0207/PEBAL.
The use of an ensemble of feature spaces trained with distance metric learning methods has been empirically shown to be useful for the task of automatically designing local image descriptors. In this paper, we present a quantitative analysis which shows that in general, nonlinear distance metric learning methods provide better results than linear methods for automatically designing local image descriptors. In addition, we show that the learned feature spaces present better results than state of- the-art hand designed features in benchmark quantitative comparisons. We discuss the results and suggest relevant problems for further investigation.
Current polyp detection methods from colonoscopy videos use exclusively normal (i.e., healthy) training images, which i) ignore the importance of temporal information in consecutive video frames, and ii) lack knowledge about the polyps. Consequently, they often have high detection errors, especially on challenging polyp cases (e.g., small, flat, or partially visible polyps). In this work, we formulate polyp detection as a weakly-supervised anomaly detection task that uses video-level labelled training data to detect frame-level polyps. In particular, we propose a novel convolutional transformer-based multiple instance learning method designed to identify abnormal frames (i.e., frames with polyps) from anomalous videos (i.e., videos containing at least one frame with polyp). In our method, local and global temporal dependencies are seamlessly captured while we simultaneously optimise video and snippet-level anomaly scores. A contrastive snippet mining method is also proposed to enable an effective modelling of the challenging polyp cases. The resulting method achieves a detection accuracy that is substantially better than current state-of-the-art approaches on a new large-scale colonoscopy video dataset introduced in this work.
In this paper, we present an improved algorithm for the segmentation of cytoplasm and nuclei from clumps of overlapping cervical cells. This problem is notoriously difficult because of the degree of overlap among cells, the poor contrast of cell cytoplasm and the presence of mucus, blood, and inflammatory cells. Our methodology addresses these issues by utilizing a joint optimization of multiple level set functions, where each function represents a cell within a clump, that have both unary (intracell) and pairwise (intercell) constraints. The unary constraints are based on contour length, edge strength, and cell shape, while the pairwise constraint is computed based on the area of the overlapping regions. In this way, our methodology enables the analysis of nuclei and cytoplasm from both free-lying and overlapping cells. We provide a systematic evaluation of our methodology using a database of over 900 images generated by synthetically overlapping images of free-lying cervical cells, where the number of cells within a clump is varied from 2 to 10 and the overlap coefficient between pairs of cells from 0.1 to 0.5. This quantitative assessment demonstrates that our methodology can successfully segment clumps of up to 10 cells, provided the overlap between pairs of cells is
Recently, there has been an increasing interest in the investigation of statistical pattern recognition models for the fully automatic segmentation of the left ventricle (LV) of the heart from ultrasound data. The main vulnerability of these models resides in the need of large manually annotated training sets for the parameter estimation procedure. The issue is that these training sets need to be annotated by clinicians, which makes this training set acquisition process quite expensive. Therefore, reducing the dependence on large training sets is important for a more extensive exploration of statistical models in the LV segmentation problem. In this paper, we present a novel incremental on-line semi-supervised learning model that reduces the need of large training sets for estimating the parameters of statistical models. Compared to other semi-supervised techniques, our method yields an on-line incremental re-training and segmentation instead of the off-line incremental re-training and segmentation more commonly found in the literature. Another innovation of our approach is that we use a statistical model based on deep learning architectures, which are easily adapted to this on-line incremental learning framework. We show that our fully automatic LV segmentation method achieves state-of-the-art accuracy with training sets containing less than twenty annotated images.
Generalised zero-shot learning (GZSL) is defined by a training process containing a set of visual samples from seen classes and a set of semantic samples from seen and unseen classes, while the testing process consists of the classification of visual samples from the seen and the unseen classes. Current approaches are based on inference processes that rely on the result of a single modality classifier (visual, semantic, or latent joint space) that balances the classification between the seen and unseen classes using gating mechanisms. There are a couple of problems with such approaches: 1) multi-modal classifiers are known to generally be more accurate than single modality classifiers, and 2) gating mechanisms rely on a complex one-class training of an external domain classifier that modulates the seen and unseen classifiers. In this paper, we mitigate these issues by proposing a novel GZSL method – augmentation network that tackles multi-modal and multi-domain inference for generalised zero-shot learning (AN-GZSL). The multi-modal inference combines visual and semantic classification and automatically balances the seen and unseen classification using temperature calibration, without requiring any gating mechanisms or external domain classifiers. Experiments show that our method produces the new state-of-the-art GZSL results for fine-grained benchmark data sets CUB and FLO and for the large-scale data set ImageNet. We also obtain competitive results for coarse-grained data sets SUN and AWA. We show an ablation study that justifies each stage of the proposed AN-GZSL.
We present a novel methodology for the automated detection of breast lesions from dynamic contrast-enhanced magnetic resonance volumes (DCE-MRI). Our method, based on deep reinforcement learning, significantly reduces the inference time for lesion detection compared to an exhaustive search, while retaining state-of-art accuracy. This speed-up is achieved via an attention mechanism that progressively focuses the search for a lesion (or lesions) on the appropriate region(s) of the input volume. The attention mechanism is implemented by training an artificial agent to learn a search policy, which is then exploited during inference. Specifically, we extend the deep Q-network approach, previously demonstrated on simpler problems such as anatomical landmark detection, in order to detect lesions that have a significant variation in shape, appearance, location and size. We demonstrate our results on a dataset containing 117 DCE-MRI volumes, validating run-time and accuracy of lesion detection.
Real-time and robust automatic detection of polyps from colonoscopy videos are essential tasks to help improve the performance of doctors during this exam. The current focus of the field is on the development of accurate but inefficient detectors that will not enable a real-time application. We advocate that the field should instead focus on the development of simple and efficient detectors that can be combined with effective trackers to allow the implementation of real-time polyp detectors. In this paper, we propose a Kalman filtering tracker that can work together with powerful, but efficient detectors, enabling the implementation of real-time polyp detectors. In particular, we show that the combination of our Kalman filtering with the detector PP-YOLO shows state-of-the-art (SOTA) detection accuracy and real-time processing. More specifically, our approach has SOTA results on the CVC-ClinicDB dataset, with a recall of 0.740, precision of 0.869, F-1 score of 0.799, an average precision (AP) of 0.837, and can run in real time (i.e., 30 frames per second). We also evaluate our method on a subset of the Hyper-Kvasir annotated by our clinical collaborators, resulting in SOTA results, with a recall of 0.956, precision of 0.875, F-1 score of 0.914, AP of 0.952, and can run in real time.
Consistency learning using input image, feature, or network perturbations has shown remarkable results in semi-supervised semantic segmentation, but this approach can be seriously affected by inaccurate predictions of unlabelled training images. There are two consequences of these inaccurate predictions: 1) the training based on the "strict" cross-entropy (CE) loss can easily overfit prediction mistakes, leading to confirmation bias; and 2) the perturbations applied to these inaccurate predictions will use potentially erroneous predictions as training signals, degrading consistency learning. In this paper; we address the prediction accuracy problem of consistency learning methods with novel extensions of the mean-teacher (MT) model, which include a new auxiliary teacher; and the replacement of MT's mean square error (MSE) by a stricter confidence-weighted cross-entropy (Conf-CE) loss. The accurate prediction by this model allows us to use a challenging combination of network, input data and feature perturbations to improve the consistency learning generalisation, where the feature perturbations consist of a new adversarial perturbation. Results on public benchmarks show that our approach achieves remarkable improvements over the previous SOTA methods in the field.(1) Our code is available at https : //github.com/ yyliu 01 / PS-MT.
The use of 3-D ultrasound data has several advantages over 2-D ultrasound for fetal biometric measurements, such as considerable decrease in the examination time, possibility of post-exam data processing by experts and the ability to produce 2-D views of the fetal anatomies in orientations that cannot be seen in common 2-D ultrasound exams. However, the search for standardized planes and the precise localization of fetal anatomies in ultrasound volumes are hard and time consuming processes even for expert physicians and sonographers. The relative low resolution in ultrasound volumes, small size of fetus anatomies and inter-volume position, orientation and size variability make this localization problem even more challenging. In order to make the plane search and fetal anatomy localization problems completely automatic, we introduce a novel principled probabilistic model that combines discriminative and generative classifiers with contextual information and sequential sampling. We implement a system based on this model, where the user queries consist of semantic keywords that represent anatomical structures of interest. After queried, the system automatically displays standardized planes and produces biometric measurements of the fetal anatomies. Experimental results on a held-out test set show that the automatic measurements are within the inter-user variability of expert users. It resolves for position, orientation and size of three different anatomies in less than 10 seconds in a dual-core computer running at 1.7 GHz.
Methods to detect malignant lesions from screening mammograms are usually trained with fully annotated datasets, where images are labelled with the localisation and classification of cancerous lesions. However, real-world screening mammogram datasets commonly have a subset that is fully annotated and another subset that is weakly annotated with just the global classification (i.e., without lesion localisation). Given the large size of such datasets, researchers usually face a dilemma with the weakly annotated subset: to not use it or to fully annotate it. The first option will reduce detection accuracy because it does not use the whole dataset, and the second option is too expensive given that the annotation needs to be done by expert radiologists. In this paper, we propose a middle-ground solution for the dilemma, which is to formulate the training as a weakly- and semi-supervised learning problem that we refer to as malignant breast lesion detection with incomplete annotations. To address this problem, our new method comprises two stages, namely: 1) pre-training a multi-view mammogram classifier with weak supervision from the whole dataset, and 2) extending the trained classifier to become a multi-view detector that is trained with semi-supervised student-teacher learning, where the training set contains fully and weakly-annotated mammograms. We provide extensive detection results on two real-world screening mammogram datasets containing incomplete annotations, and show that our proposed approach achieves state-of-the-art results in the detection of malignant breast lesions with incomplete annotations.
Pattern recognition methods have become a powerful tool for segmentation in the sense that they are capable of automatically building a segmentation model from training images. However, they present several difficulties, such as requirement of a large set of training data, robustness to imaging conditions not present in the training set, and complexity of the search process. In this paper we tackle the second problem by using a deep belief network learning architecture, and the third problem by resorting to efficient searching algorithms. As an example, we illustrate the performance of the algorithm in lip segmentation and tracking in video sequences. Quantitative comparison using different strategies for the search process are presented. We also compare our approach to a state-of-the-art segmentation and tracking algorithm. The comparison show that our algorithm produces competitive segmentation results and that efficient search strategies reduce ten times the run-complexity.
In this paper, we propose an ensemble classification approach to the Pedestrian Detection (PD) problem, resorting to distinct input channels and Convolutional Neural Networks (CNN). This methodology comprises two stages: (i) the proposals extraction, and (ii) the ensemble classification. In order to obtain the proposals, we apply several detectors specifically developed for the PD task. Afterwards, these proposals are converted into different input channels (e.g. gradient magnitude, LUV or RGB), and classified by each CNN. Finally, several ensemble methods are used to combine the output probabilities of each CNN model. By correctly selecting the best combination strategy, we achieve improvements, comparatively to the single CNN models predictions.
Anomaly detection with weakly supervised video-level labels is typically formulated as a multiple instance learning (MIL) problem, in which we aim to identify snippets containing abnormal events, with each video represented as a bag of video snippets. Although current methods show effective detection performance, their recognition of the positive instances, i.e., rare abnormal snippets in the abnormal videos, is largely biased by the dominant negative instances, especially when the abnormal events are subtle anomalies that exhibit only small differences compared with normal events. This issue is exacerbated in many methods that ignore important video temporal dependencies. To address this issue, we introduce a novel and theoretically sound method, named Robust Temporal Feature Magnitude learning (RTFM), which trains a feature magnitude learning function to effectively recognise the positive instances, substantially improving the robustness of the MIL approach to the negative instances from abnormal videos. RTFM also adapts dilated convolutions and self-attention mechanisms to capture long- and short-range temporal dependencies to learn the feature magnitude more faithfully. Extensive experiments show that the RTFM-enabled MIL model (i) outperforms several state-of-the-art methods by a large margin on four benchmark data sets (ShanghaiTech, UCF-Crime, XD-Violence and UCSD-Peds) and (ii) achieves significantly improved subtle anomaly discriminability and sample efficiency.
This work introduces a new pattern recognition model for segmenting and tracking lip contours in video sequences. We formulate the problem as a general nonrigid object tracking method, where the computation of the expected segmentation is based on a filtering distribution. This is a difficult task because one has to compute the expected value using the whole parameter space of segmentation. As a result, we compute the expected segmentation using sequential Monte Carlo sampling methods, where the filtering distribution is approximated with a proposal distribution to be used for sampling. The key contribution of this paper is the formulation of this proposal distribution using a new observation model based on deep belief networks and a new transition model. The efficacy of the model is demonstrated in publicly available databases of video sequences of people talking and singing. Our method produces results comparable to state-of-the-art models, but showing potential to be more robust to imaging conditions.
Generalised zero-shot learning (GZSL) is a classification problem where the learning stage relies on a set of seen visual classes and the inference stage aims to identify both the seen visual classes and a new set of unseen visual classes. Critically, both the learning and inference stages can leverage a semantic representation that is available for the seen and unseen classes. Most state-of-the-art GZSL approaches rely on a mapping between latent visual and semantic spaces without considering if a particular sample belongs to the set of seen or unseen classes. In this paper, we propose a novel GZSL method that learns a joint latent representation that combines both visual and semantic information. This mitigates the need for learning a mapping between the two spaces. Our method also introduces a domain classification that estimates whether a sample belongs to a seen or an unseen class. Our classifier then combines a class discriminator with this domain classifier with the goal of reducing the natural bias that GZSL approaches have toward the seen classes. Experiments show that our method achieves state-of-the-art results in terms of harmonic mean, the area under the seen and unseen curve and unseen classification accuracy on public GZSL benchmark data sets. Our code will be available upon acceptance of this paper.
In this paper, we explore the use of deep convolution and deep belief networks as potential functions in structured prediction models for the segmentation of breast masses from mammograms. In particular, the structured prediction models are estimated with loss minimization parameter learning algorithms, representing: a) conditional random field (CRF), and b) structured support vector machine (SSVM). For the CRF model, we use the inference algorithm based on tree re-weighted belief propagation with truncated fitting training, and for the SSVM model the inference is based on graph cuts with maximum margin training. We show empirically the importance of deep learning methods in producing state-of-the-art results for both structured prediction models. In addition, we show that our methods produce results that can be considered the best results to date on DDSM-BCRP and INbreast databases. Finally, we show that the CRF model is significantly faster than SSVM, both in terms of inference and training time, which suggests an advantage of CRF models when combined with deep learning potential functions.
Machine learning models for medical image analysis often suffer from poor performance on important subsets of a population that are not identified during training or testing. For example, overall performance of a cancer detection model may be high, but the model may still consistently miss a rare but aggressive cancer subtype. We refer to this problem as hidden stratification , and observe that it results from incompletely describing the meaningful variation in a dataset. While hidden stratification can substantially reduce the clinical efficacy of machine learning models, its effects remain difficult to measure. In this work, we assess the utility of several possible techniques for measuring hidden stratification effects, and characterize these effects both via synthetic experiments on the CIFAR-100 benchmark dataset and on multiple real-world medical imaging datasets. Using these measurement techniques, we find evidence that hidden stratification can occur in unidentified imaging subsets with low prevalence, low label quality, subtle distinguishing features, or spurious correlates, and that it can result in relative performance differences of over 20% on clinically important subsets. Finally, we discuss the clinical implications of our findings, and suggest that evaluation of hidden stratification should be a critical component of any machine learning deployment in medical imaging.
We introduce a new method that characterizes quantitatively local image descriptors in terms of their distinctiveness and robustness to geometric transformations and brightness deformations. The quantitative characterization of these properties is important for recognition systems based on local descriptors because it allows for the implementation of a classifier that selects descriptors based on their distinctiveness and robustness properties. This classification results in: (a) recognition time reduction due to a smaller number of descriptors present in the test image and in the database of model descriptors; (b) improvement of the recognition accuracy since only the most reliable descriptors for the recognition task are kept in the model and test images; and (c) better scalability given the smaller number of descriptors per model. Moreover, the quantitative characterization of distinctiveness and robustness of local descriptors provides a more accurate formulation of the recognition process, which has the potential to improve the recognition accuracy. We show how to train a multi-layer perceptron that quickly classifies robust and distinctive local image descriptors. A regressor is also trained to provide quantitative models for each descriptor. Experimental results show that the use of these trained models not only improves the performance of our recognition system, but it also reduces significantly the computation time for the recognition process. (C) 2008 Elsevier B.V. All rights reserved.
Survival time prediction from medical images is important for treatment planning, where accurate estimations can improve healthcare quality. One issue affecting the training of survival models is censored data. Most of the current survival prediction approaches are based on Cox models that can deal with censored data, but their application scope is limited because they output a hazard function instead of a survival time. On the other hand, methods that predict survival time usually ignore censored data, resulting in an under-utilization of the training set. In this work, we propose a new training method that predicts survival time using all censored and uncensored data. We propose to treat censored data as samples with a lower-bound time to death and estimate pseudo labels to semi-supervise a censor-aware survival time regressor. We evaluate our method on pathology and x-ray images from the TCGA-GM and NLST datasets. Our results establish the state-of-the-art survival prediction accuracy on both datasets.
Future public transportation systems will provide broadband access to passengers, carrying legacy terminals with 802.11 connectivity. Passengers will be able to communicate with the Internet and with each other, while connected to 802.11 Access Points deployed in vehicles and bus stops/metro stations, and without requiring special mobility or routing protocols to run in their terminals. Existing solutions, such as 802.11s and OLSR, are not efficient and do not scale to large networks, thereby requiring the network to be segmented in many small areas, causing the terminals to change IP address when moving between areas. This paper presents WiMetroNet, a large mesh network of mobile routers (Rbridges) operating at layer 2.5 over heterogeneous wireless technologies. This architecture contains an efficient user plane that optimizes the transport of DHCP and ARP traffic, and provides a transparent terminal mobility solution using techniques that minimize the routing overhead for large networks. We offer two techniques to reduce routing overhead associated with terminal mobility. One approach is based on TTL-limited flooding of a routing message and on the concept of forwarding packets only to the vicinity of the last known location of the terminal, and then forward the packets to a new location of the terminal. The other technique lets the network remain unaware for a very long time that the terminal has moved; only when packets arrive at the old PoA does the PoA send back a "binding update" message to the correspondent node, to correct the route for future packets for the same terminal. Simulation and analytical results are presented, and the routing protocol is shown to scale to large networks with good user plane results, namely packet delivery rate, delay, and handover interruption time. (C) 2011 Elsevier B.V. All rights reserved.
Mass detection from mammograms plays a crucial role as a pre- processing stage for mass segmentation and classification. The detection of masses from mammograms is considered to be a challenging problem due to their large variation in shape, size, boundary and texture and also because of their low signal to noise ratio compared to the surrounding breast tissue. In this paper, we present a novel approach for detecting masses in mammograms using a cascade of deep learning and random forest classifiers. The first stage classifier consists of a multi-scale deep belief network that selects suspicious regions to be further processed by a two-level cascade of deep convolutional neural networks. The regions that survive this deep learning analysis are then processed by a two-level cascade of random forest classifiers that use morphological and texture features extracted from regions selected along the cascade. Finally, regions that survive the cascade of random forest classifiers are combined using connected component analysis to produce state-of-the-art results. We also show that the proposed cascade of deep learning and random forest classifiers are effective in the reduction of false positive regions, while maintaining a high true positive detection rate. We tested our mass detection system on two publicly available datasets: DDSM-BCRP and INbreast. The final mass detection produced by our approach achieves the best results on these publicly available datasets with a true positive rate of 0.96 ± 0.03 at 1.2 false positive per image on INbreast and true positive rate of 0.75 at 4.8 false positive per image on DDSM-BCRP.
In recently published clinical trial results, hypoxia-modified therapies have shown to provide more positive outcomes to cancer patients, compared with standard cancer treatments. The development and validation of these hypoxia-modified therapies depend on an effective way of measuring tumor hypoxia, but a standardized measurement is currently unavailable in clinical practice. Different types of manual measurements have been proposed in clinical research, but in this paper we focus on a recently published approach that quantifies the number and proportion of hypoxic regions using high resolution (immuno-)fluorescence (IF) and hematoxylin and eosin (HE) stained images of a histological specimen of a tumor. We introduce new machine learning-based methodologies to automate this measurement, where the main challenge is the fact that the clinical annotations available for training the proposed methodologies consist of the total number of normoxic, chronically hypoxic, and acutely hypoxic regions without any indication of their location in the image. Therefore, this represents a weakly-supervised structured output classification problem, where training is based on a high-order loss function formed by the norm of the difference between the manual and estimated annotations mentioned above. We propose four methodologies to solve this problem: 1) a naive method that uses a majority classifier applied on the nodes of a fixed grid placed over the input images; 2) a baseline method based on a structured output learning formulation that relies on a fixed grid placed over the input images; 3) an extension to this baseline based on a latent structured output learning formulation that uses a graph that is flexible in terms of the amount and positions of nodes; and 4) a pixel-wise labeling based on a fully-convolutional neural network. Using a data set of 89 weakly annotated pairs of IF and HE images from eight tumors, we show that the quantitative results of methods (3) and (4) above are equally competitive and superior to the naive (1) and baseline (2) methods. All proposed methodologies show high correlation values with respect to the clinical annotations.
•A new noisy-label learning algorithm, called ScanMix•ScanMix combines semantic clustering and semi-supervised learning•ScanMix is remarkably robust to severe label noise rates•ScanMix provides competitive performance in a wide range of noisy-label learning problems•A new theoretical result that shows the correctness and convergence of ScanMix [Display omitted] We propose a new training algorithm, ScanMix, that explores semantic clustering and semi-supervised learning (SSL) to allow superior robustness to severe label noise and competitive robustness to non-severe label noise problems, in comparison to the state of the art (SOTA) methods. ScanMix is based on the expectation maximisation framework, where the E-step estimates the latent variable to cluster the training images based on their appearance and classification results, and the M-step optimises the SSL classification and learns effective feature representations via semantic clustering. We present a theoretical result that shows the correctness and convergence of ScanMix, and an empirical result that shows that ScanMix has SOTA results on CIFAR-10/-100 (with symmetric, asymmetric and semantic label noise), Red Mini-ImageNet (from the Controlled Noisy Web Labels), Clothing1M and WebVision. In all benchmarks with severe label noise, our results are competitive to the current SOTA.
Monocular depth estimation has become one of the most studied applications in computer vision, where the most accurate approaches are based on fully supervised learning models. However, the acquisition of accurate and large ground truth data sets to model these fully supervised methods is a major challenge for the further development of the area. Self-supervised methods trained with monocular videos constitute one the most promising approaches to mitigate the challenge mentioned above due to the wide-spread availability of training data. Consequently, they have been intensively studied, where the main ideas explored consist of different types of model architectures, loss functions, and occlusion masks to address non-rigid motion. In this paper, we propose two new ideas to improve self-supervised monocular trained depth estimation: 1) self-attention, and 2) discrete disparity prediction. Compared with the usual localised convolution operation, self-attention can explore a more general contextual information that allows the inference of similar disparity values at non-contiguous regions of the image. Discrete disparity prediction has been shown by fully supervised methods to provide a more robust and sharper depth estimation than the more common continuous disparity prediction, besides enabling the estimation of depth uncertainty. We show that the extension of the state-of-the-art self-supervised monocular trained depth estimator Monodepth2 with these two ideas allows us to design a model that produces the best results in the field in KITTI 2015 and Make3D, closing the gap with respect self-supervised stereo training and fully supervised approaches.
There has recently been significant interest in top-down image segmentation methods, which incorporate the recognition of visual concepts as an intermediate step of segmentation. This work addresses the problem of top-down segmentation with weak supervision. Under this framework, learning does not require a set of manually segmented examples for each concept of interest, but simply a weakly labeled training set. This is a training set where images are annotated with a set of keywords describing their contents, but visual concepts are not explicitly segmented and no correspondence is specified between keywords and image regions. We demonstrate, both analytically and empirically, that weakly supervised segmentation is feasible when certain conditions hold. We also propose a simple weakly supervised segmentation algorithm that extends state-of-theart bottom-up segmentation methods in the direction of perceptually meaningful segmentation1.
The diagnosis of the presence of metastatic lymph nodes from abdominal computed tomography (CT) scans is an essential task performed by radiologists to guide radiation and chemotherapy treatment. State-of-the-art deep learning classifiers trained for this task usually rely on a training set containing CT volumes and their respective image-level (i.e., global) annotation. However, the lack of annotations for the localisation of the regions of interest (ROIs) containing lymph nodes can limit classification accuracy due to the small size of the relevant ROIs in this problem. The use of lymph node ROIs together with global annotations in a multi-task training process has the potential to improve classification accuracy, but the high cost involved in obtaining the ROI annotation for the same samples that have global annotations is a roadblock for this alternative. We address this limitation by introducing a new training strategy from two data sets: one containing the global annotations, and another (publicly available) containing only the lymph node ROI localisation. We term our new strategy semi-supervised multi-domain multi-task training, where the goal is to improve the diagnosis accuracy on the globally annotated data set by incorporating the ROI annotations from a different domain. Using a private data set containing global annotations and a public data set containing lymph node ROI localisation, we show that our proposed training mechanism improves the area under the ROC curve for the classification task compared to several training method baselines.
Automatic delineation and robust measurement of fetal anat-omical structures in 2D ultrasound images is a challenging task due to the complexity of the object appearance, noise, shadows, and quantity of information to be processed. Previous solutions rely on explicit encoding of prior knowledge and formulate the problem as a perceptual grouping task solved through clustering or variational approaches. These methods are known to be limited by the validity of the underlying assumptions and cannot capture complex structure appearances. We propose a novel system for fast automatic obstetric measurements by directly exploiting a large database of expert annotated fetal anatomical structures in ultrasound images. Our method learns to distinguish between the appearance of the object of interest and background by training a discriminative constrained probabilistic boosting tree classifier. This system is able to handle previously unsolved problems in this domain, such as the effective segmentation of fetal abdomens. We show results on fully automatic measurement of head circumference, biparietal diameter, abdominal circumference and femur length. Unparalleled extensive experiments show that our system is, on average, close to the accuracy of experts in terms of segmentation and obstetric measurements. Finally, this system runs under half second on a standard dual-core PC computer.
In this paper, we propose a new methodology for segmenting non-rigid visual objects, where the search procedure is conducted directly on a sparse low-dimensional manifold, guided by the classification results computed from a deep belief network. Our main contribution is the fact that we do not rely on the typical sub-division of segmentation tasks into rigid detection and non-rigid delineation. Instead, the non-rigid segmentation is performed directly, where points in the sparse low-dimensional can be mapped to an explicit contour representation in image space. Our proposal shows significantly smaller search and training complexities given that the dimensionality of the manifold is much smaller than the dimensionality of the search spaces for rigid detection and non-rigid delineation aforementioned, and that we no longer require a two-stage segmentation process. We focus on the problem of left ventricle endocardial segmentation from ultrasound images, and lip segmentation from frontal facial images using the extended Cohn-Kanade (CK+) database. Our experiments show that the use of sparse low dimensional manifolds reduces the search and training complexities of current segmentation approaches without a significant impact on the segmentation accuracy shown by state-of-the-art approaches.
•We propose a new two-stage noisy-label learning algorithm, called LongReMix.•The first stage finds a highly precise, but potentially small, set of clean samples.•The second stage is designed to be robust to small sets of clean samples.•LongReMix reaches SOTA performance on the main noisy-label learning benchmarks. State-of-the-art noisy-label learning algorithms rely on an unsupervised learning to classify training samples as clean or noisy, followed by a semi-supervised learning (SSL) that minimises the empirical vicinal risk using a labelled set formed by samples classified as clean, and an unlabelled set with samples classified as noisy. The classification accuracy of such noisy-label learning methods depends on the precision of the unsupervised classification of clean and noisy samples, and the robustness of SSL to small clean sets. We address these points with a new noisy-label training algorithm, called LongReMix, which improves the precision of the unsupervised classification of clean and noisy samples and the robustness of SSL to small clean sets with a two-stage learning process. The stage one of LongReMix finds a small but precise high-confidence clean set, and stage two augments this high-confidence clean set with new clean samples and oversamples the clean data to increase the robustness of SSL to small clean sets. We test LongReMix on CIFAR-10 and CIFAR-100 with introduced synthetic noisy labels, and the real-world noisy-label benchmarks CNWL (Red Mini-ImageNet), WebVision, Clothing1M, and Food101-N. The results show that our LongReMix produces significantly better classification accuracy than competing approaches, particularly in high noise rate problems. Furthermore, our approach achieves state-of-the-art performance in most datasets. The code is available at https://github.com/filipe-research/LongReMix.
Computer-aided diagnosis of digital chest X-ray (CXR) images critically depends on the automated segmentation of the lungs, which is a challenging problem due to the presence of strong edges at the rib cage and clavicle, the lack of a consistent lung shape among different individuals, and the appearance of the lung apex. From recently published results in this area, hybrid methodologies based on a combination of different techniques (e.g., pixel classification and deformable models) are producing the most accurate lung segmentation results. In this paper, we propose a new methodology for lung segmentation in CXR using a hybrid method based on a combination of distance regularized level set and deep structured inference. This combination brings together the advantages of deep learning methods (robust training with few annotated samples and top-down segmentation with structured inference and learning) and level set methods (use of shape and appearance priors and efficient optimization techniques). Using the publicly available Japanese Society of Radiological Technology (JSRT) dataset, we show that our approach produces the most accurate lung segmentation results in the field. In particular, depending on the initialization used, our methodology produces an average accuracy on JSTR that varies from 94.8% to 98.5%.
The segmentation of anatomical structures is a crucial first stage of most medical imaging analysis procedures. A primary example is the segmentation of the left ventricle (LV), from cardiac imagery. Accuracy in the segmentation often requires a considerable amount of expert intervention and guidance which are expensive. Thus, automating the segmentation is welcome, but difficult because of the LV shape variability within and across individuals. To cope with this difficulty, the algorithm should have the skills to interpret the shape of the anatomical structure (i.e. LV shape) using distinct kinds of information, (i.e. different views of the same feature space). These different views will ascribe to the algorithm a more general capability that surely allows for the robustness in the segmentation accuracy. In this paper, we propose an on-line co-training algorithm using a bottom-up and top-down classifiers (each one having a different view of the data) to perform the segmentation of the LV. In particular, we consider a setting in which the LV shape can be partitioned into two distinct views and use a co-training as a way to boost each of the classifiers, thus providing a principled way to use both views together. We testify the usefulness of the approach on a public data base illustrating that the approach compares favorably with other recent proposed methodologies.
This paper proposes a novel approach for the non-rigid segmentation of deformable objects in image sequences, which is based on one-shot segmentation that unifies rigid detection and non-rigid segmentation using elastic regularization. The domain of application is the segmentation of a visual object that temporally undergoes a rigid transformation (e.g., affine transformation) and a non-rigid transformation (i.e., contour deformation). The majority of segmentation approaches to solve this problem are generally based on two steps that run in sequence: a rigid detection, followed by a non-rigid segmentation. In this paper, we propose a new approach, where both the rigid and non-rigid segmentation are performed in a single shot using a sparse low-dimensional manifold that represents the visual object deformations. Given the multi-modality of these deformations, the manifold partitions the training data into several patches, where each patch provides a segmentation proposal during the inference process. These multiple segmentation proposals are merged using the classification results produced by deep belief networks (DBN) that compute the confidence on each segmentation proposal. Thus, an ensemble of DBN classifiers is used for estimating the final segmentation. Compared to current methods proposed in the field, our proposed approach is advantageous in four aspects: (i) it is a unified framework to produce rigid and non-rigid segmentations; (ii) it uses an ensemble classification process, which can help the segmentation robustness; (iii) it provides a significant reduction in terms of the number of dimensions of the rigid and non-rigid segmentations search spaces, compared to current approaches that divide these two problems; and (iv) this lower dimensionality of the search space can also reduce the need for large annotated training sets to be used for estimating the DBN models. Experiments on the problem of left ventricle endocardial segmentation from ultrasound images, and lip segmentation from frontal facial images using the extended Cohn-Kanade (CK+) database, demonstrate the potential of the methodology through qualitative and quantitative evaluations, and the ability to reduce the search and training complexities without a significant impact on the segmentation accuracy.