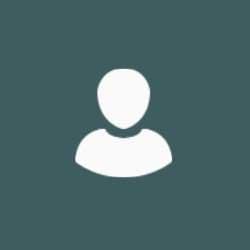
Giacomo Acciarini
About
My research project
Stochastic continuation for space trajectory design in uncertain environmentsAn essential part of any spacecraft mission is the design, planning, and operation of robust and fuel-efficient trajectories that can fulfill mission requirements while coping with the harsh reality of the space environment. These trajectories are traditionally engineered in a two-step approach. First, orbits are designed in a deterministic fashion, assuming that the dynamics and state of the spacecraft are known with infinite accuracy. Secondly, the robustness of these initial conditions is tested against model uncertainties and knowledge errors using brute-force Monte Carlo simulations that explore different realizations of the uncertainty set. This two-step approach is not only time-consuming but also contributes to slowing down the mission development process as the robustness of candidate orbits can only be assessed a-posteriori.
The goal of this project is to leverage advanced mathematical tools to directly calculate, up to a certain confidence level, regions of the phase space where the spacecraft is expected to orbit depending on the time scales and uncertainties of a user-defined problem. The numerical procedures will be general by nature and therefore applicable to a variety of spacecraft missions, including ESA’s Hera, aiming towards the binary asteroid 65803 Didymos, and the JAXA-lead MMX, destined for Phobos.
Supervisors
An essential part of any spacecraft mission is the design, planning, and operation of robust and fuel-efficient trajectories that can fulfill mission requirements while coping with the harsh reality of the space environment. These trajectories are traditionally engineered in a two-step approach. First, orbits are designed in a deterministic fashion, assuming that the dynamics and state of the spacecraft are known with infinite accuracy. Secondly, the robustness of these initial conditions is tested against model uncertainties and knowledge errors using brute-force Monte Carlo simulations that explore different realizations of the uncertainty set. This two-step approach is not only time-consuming but also contributes to slowing down the mission development process as the robustness of candidate orbits can only be assessed a-posteriori.
The goal of this project is to leverage advanced mathematical tools to directly calculate, up to a certain confidence level, regions of the phase space where the spacecraft is expected to orbit depending on the time scales and uncertainties of a user-defined problem. The numerical procedures will be general by nature and therefore applicable to a variety of spacecraft missions, including ESA’s Hera, aiming towards the binary asteroid 65803 Didymos, and the JAXA-lead MMX, destined for Phobos.
Publications
Accurately predicting eclipse events around irregular small bodies is crucial for spacecraft navigation, orbit determination, and spacecraft systems management. This paper introduces a novel approach leveraging neural implicit representations to model eclipse conditions efficiently and reliably. We propose neural network architectures that capture the complex silhouettes of asteroids and comets with high precision. Tested on four well-characterized bodies - Bennu, Itokawa, 67P/Churyumov-Gerasimenko, and Eros - our method achieves accuracy comparable to traditional ray-tracing techniques while offering orders of magnitude faster performance. Additionally, we develop an indirect learning framework that trains these models directly from sparse trajectory data using Neural Ordinary Differential Equations, removing the requirement to have prior knowledge of an accurate shape model. This approach allows for the continuous refinement of eclipse predictions, progressively reducing errors and improving accuracy as new trajectory data is incorporated.
This paper introduces DTACSNet, a Convolutional Neural Network (CNN) model specifically developed for efficient onboard atmospheric correction and cloud detection in optical Earth observation satellites. The model is developed with Sentinel-2 data. Through a comparative analysis with the operational Sen2Cor processor, DTACSNet demonstrates a significantly better performance in cloud scene classification (F2 score of 0.89 for DTACSNet compared to 0.51 for Sen2Cor v2.8) and a surface reflectance estimation with average absolute error below 2% in reflectance units. Moreover, we tested DTACSNet on hardware-constrained systems similar to recent deployed missions and show that DTACSNet is 11 times faster than Sen2Cor with a significantly lower memory consumption footprint. These preliminary results highlight the potential of DTACSNet to provide enhanced efficiency, autonomy, and responsiveness in onboard data processing for Earth observation satellite missions.
In 2023, the 12th edition of Global Trajectory Competition was organized around the problem referred to as "Sustainable Asteroid Mining". This paper reports the developments that led to the solution proposed by ESA's Advanced Concepts Team. Beyond the fact that the proposed approach failed to rank higher than fourth in the final competition leader-board, several innovative fundamental methodologies were developed which have a broader application. In particular, new methods based on machine learning as well as on manipulating the fundamental laws of astrodynamics were developed and able to fill with remarkable accuracy the gap between full low-thrust trajectories and their representation as impulsive Lambert transfers. A novel technique was devised to formulate the challenge of optimal subset selection from a repository of pre-existing optimal mining trajectories as an integer linear programming problem. Finally, the fundamental problem of searching for single optimal mining trajectories (mining and collecting all resources), albeit ignoring the possibility of having intra-ship collaboration and thus sub-optimal in the case of the GTOC12 problem, was efficiently solved by means of a novel search based on a look-ahead score and thus making sure to select asteroids that had chances to be re-visited later on.
Estimating the probability of collision between spacecraft is crucial for risk management and collision-avoidance strategies. Current methods often rely on Gaussian assumptions and simplifications, which can be inaccurate in highly nonlinear scenarios. This paper presents a general and efficient approach for computing collision probabilities without relying on such assumptions. Using high-order multivariate Taylor polynomials, we propagate statistical moments of initial uncertainties to the point of closest approach between the spacecraft. To compute the probability of collision, we derive a semi-analytical expression for the probability density function (PDF) of the closest approach distance, inferred from the propagated moments using orthogonal polynomials. Tested on various short-term and long-term encounters in low-Earth orbit, our method accurately handles nonlinear dynamics, non-Gaussian uncertainties, and irregular distributions. This versatile framework advances space situational awareness by providing precise collision probability estimates in complex dynamical environments. Moreover, our methodology applies to any dynamical system with uncertainty in its initial state and is therefore not restricted to collision probability estimation.
In the field of spaceflight mechanics and astrodynamics, determining eclipse regions is a frequent and critical challenge. This determination impacts various factors, including the acceleration induced by solar radiation pressure, the spacecraft power input, and its thermal state all of which must be accounted for in various phases of the mission design. This study leverages recent advances in neural image processing to develop fully differentiable models of eclipse regions for highly irregular celestial bodies. By utilizing test cases involving Solar System bodies previously visited by spacecraft, such as 433 Eros, 25143 Itokawa, 67P/Churyumov--Gerasimenko, and 101955 Bennu, we propose and study an implicit neural architecture defining the shape of the eclipse cone based on the Sun's direction. Employing periodic activation functions, we achieve high precision in modeling eclipse conditions. Furthermore, we discuss the potential applications of these differentiable models in spaceflight mechanics computations.
This paper presents a novel approach for propagating uncertainties in dynamical systems building on high-order Taylor expansions of the flow and moment-generating functions (MGFs). Unlike prior methods that focus on Gaussian distributions, our approach leverages the relationship between MGFs and distribution moments to extend high-order uncertainty propagation techniques to non-Gaussian scenarios. This significantly broadens the applicability of these methods to a wider range of problems and uncertainty types. High-order moment computations are performed one-off and symbolically, reducing the computational burden of the technique to the calculation of Taylor series coefficients around a nominal trajectory, achieved by efficiently integrating the system's variational equations. Furthermore, the use of the proposed approach in combination with event transition tensors, allows for accurate propagation of uncertainties at specific events, such as the landing surface of a celestial body, the crossing of a predefined Poincaré section, or the trigger of an arbitrary event during the propagation. Via numerical simulations we demonstrate the effectiveness of our method in various astrodynamics applications, including the unperturbed and perturbed two-body problem, and the circular restricted three-body problem, showing that it accurately propagates non-Gaussian uncertainties both at future times and at event manifolds.
The simplified general perturbations 4 (SGP4) orbital propagation model is one of the most widely used methods for rapidly and reliably predicting the positions and velocities of objects orbiting Earth. Over time, SGP models have undergone refinement to enhance their efficiency and accuracy. Nevertheless, they still do not match the precision offered by high-precision numerical propagators, which can predict the positions and velocities of space objects in low-Earth orbit with significantly smaller errors. In this study, we introduce a novel differentiable version of SGP4, named ∂SGP4. By porting the source code of SGP4 into a differentiable program based on PyTorch, we unlock a whole new class of techniques enabled by differentiable orbit propagation, including spacecraft orbit determination, state conversion, covariance similarity transformation, state transition matrix computation, and covariance propagation. Besides differentiability, our ∂SGP4 supports parallel propagation of a batch of two-line elements (TLEs) in a single execution and it can harness modern hardware accelerators like GPUs or XLA devices (e.g. TPUs) thanks to running on the PyTorch backend. Furthermore, the design of ∂SGP4 makes it possible to use it as a differentiable component in larger machine learning (ML) pipelines, where the propagator can be an element of a larger neural network that is trained or fine-tuned with data. Consequently, we propose a novel orbital propagation paradigm, ML-∂SGP4. In this paradigm, the orbital propagator is enhanced with neural networks attached to its input and output. Through gradient-based optimization, the parameters of this combined model can be iteratively refined to achieve precision surpassing that of SGP4. Fundamentally, the neural networks function as identity operators when the propagator adheres to its default behavior as defined by SGP4. However, owing to the differentiability ingrained within ∂SGP4, the model can be fine-tuned with ephemeris data to learn corrections to both inputs and outputs of SGP4. This augmentation enhances precision while maintaining the same computational speed of ∂SGP4 at inference time. This paradigm empowers satellite operators and researchers, equipping them with the ability to train the model using their specific ephemeris or high-precision numerical propagation data.
Nano and microsatellites have expanded the acquisition of satellite images with higher spatial, temporal, and spectral resolutions. Nevertheless, downlinking all this data to the ground for processing becomes challenging as the amount of remote sensing data rises. Custom onboard algorithms are designed to make real-time decisions and to prioritize and reduce the amount of data transmitted to the ground. However, these onboard algorithms frequently require cloud-free bottom-of-atmosphere surface reflectance (SR) estimations as inputs to operate. In this context, this article presents the data transformations and autocalibration for Sentinel-2 (S-2) network (DTACSNet), an onboard cloud detection and atmospheric correction processor based on lightweight convolutional neural networks. DTACSNet provides cloud and cloud shadow masks and SR estimates 10× faster than the operational S-2 L2A processor in dedicated space-tested hardware: 7 mins versus 1 h for a 10 980 × 10 980 scene. The DTACSNet cloud masking, based on a lightweight neural network, obtains the highest F2-score (0.81), followed by the state-of-the-art KappaMask (0.74), Fmask (0.72), and Sen2Cor v.2.8 (0.51) algorithms. In addition, validation results on independent datasets show that DTACSNet can efficiently replicate Sen2Cor SR estimates, reporting a competitive accuracy with differences below 2%.
We formalize a Keplerian Travelling Salesperson Problem (ktsp) as a special version of tsp, where the " cities " correspond to objects on Keplerian orbits. In this context, the salesperson is modeled as a " spacecraft " tasked with achieving rendezvous (matching position and velocity) with each orbital object while minimizing the required Δí µí±£ from the propulsion system, subject to operational constraints such as time-of-flight limits. Unlike the classical tsp, which has been extensively studied, the Keplerian version introduces unique complexities that are relevant to real-world scenarios. These complexities arise from the dynamic movement of orbital objects and the resulting changes in transfer costs between them. The Keplerian Vehicle Routing Problem (kvrp) and Keplerian low-thrust Travelling Salesperson Problem (ktsp-lt) are also introduced, extending this framework further to scenarios involving multiple spacecraft that must collaboratively visit designated targets as well as spacecraft equipped with a low-thrust propulsion system. The specific problems modelled are related to design tasks that have gained prominence in the past decade as advanced trajectory optimization challenges, particularly highlighted in the Global Trajectory Optimization Competition series. These problems have been instrumental in automating the design of multi-rendezvous trajectories in asteroid belt missions, addressing challenges such as mission target selection. They have also been applied to plan active debris removal missions, design future asteroid mining strategies as well as ivestigate on-orbit servicing concepts. In this work, we explore different encodings of these problems into well-known combinatorial optimization tasks, focusing on Integer Linear Programming (ilp) formulations. We provide and study a umber of initial instances of various complexity. A key aspect of our work is the detailed process of unrolling time, which is critical for translating dynamic problems like ktsp into discrete optimization tasks. We also propose a possible strategy to mitigate the complexity involved in the resulting time-indexed formulations of ILPs: Interval-based Dynamic Discretization Discovery. We conclude with a preliminary experimental evaluation, comparing the performance of various ilp solvers to that of the beam search approach, commonly used to incrementally build mission plans in these cases.
We perform uncertainty propagation to an event manifold for Guidance \& Control Networks (G\&CNETs), aiming to enhance the certification tools for neural networks in this field.This work utilizes three previously solved optimal control problems with varying levels of dynamics nonlinearity and event manifold complexity. The G\&CNETs are trained to represent the optimal control policies of a time-optimal interplanetary transfer, a mass-optimal landing on an asteroid and energy-optimal drone racing, respectively.For each of these problems, we describe analytically the terminal conditions to an event manifold with respect to initial state uncertainties. Crucially, this expansion does not depend on time but solely on the initial conditions of the system, thereby making it possible to study the robustness of the G\&CNET at any specific stage of a mission defined by the event manifold.Once this analytical expression is found, we provide confidence bounds by applying the Cauchy-Hadamard theorem and perform uncertainty propagation using moment generating functions.While Monte Carlo-based (MC) methods can yield the results we present, this work is driven by the recognition that MC simulations alone may be insufficient for future certification of neural networks in guidance and control applications.
Inter-satellite links are gaining prominence due to their capacity to provide low latency, enhanced data rates, improved security, and reduced interference compared to satellite-ground-satellite connections. Notably, they are slated for implementation in the second generation of the European Space Agency's (ESA) Galileo constellation—Europe's proprietary global navigation satellite system. Furthermore, inter-satellite links have already been successfully deployed in the existing generation of Starlink satellites. At a given epoch, the arrangement of satellites defines a network of communication links. These links may not always be present, for instance when satellites are out of range, or they might exhibit failure with some probability due to factors like geography, weather conditions, hardware malfunctions, or positioning errors. Ensuring a consistent quality of service necessitates the establishment of a reliable network at all times. This entails maintaining a high probability of having a functional path between any pair of satellites or ground stations. Reliable constellations with inter-satellite communication are particularly important for quantum communication over intercontinental range, which is currently not possible via terrestrial connections. In such constellations, satellites should not only be connected but connected via short paths of at most í µí± hops, as otherwise quantum decoherence can cause problems. Measuring the reliability of networks is a classical #P-complete problem and, thus, extremely difficult. Reliability is traditionally studied with advanced Monte Carlo simulations, analytic approaches, or sampling methods such as line sampling and variance reduction schemes. Unfortunately, these strategies are computationally expensive and scale poorly. Weighted model counting, the problem of counting the weighted number of satisfying assignments of a propositional formula, is a promising alternative from the toolbox of probabilistic reasoning. Recent advances in incorporating structure-guided approaches, algebraic decision diagrams, and compilations into deterministic, decomposable negation normal forms provide a variety of open-source model counters that can handle industry-scale instances. We evaluate these tools on the problem of computing the reliability of a constellation with inter-satellite communication and, for the first time, provide theoretical sound estimates of the reliability of the imposed networks. Such estimates are the first and central step in developing an algorithmic pipeline to design robust inter-satellite topologies automatically. Our techniques generalize to the case in which we consider a network reliably if satellites are connected via short paths and, hence, we can provide similar estimates for quantum communication constellations.
As megaconstellations are launched and the space sector grows, space debris pollution is posing an increasing threat to operational spacecraft. Low Earth orbit is a junkyard of dead satellites, rocket bodies, shrapnels, and other debris that travel at very high speed in an uncontrolled manner. Collisions at orbital speeds can generate fragments and potentially trigger a cascade of more collisions endangering the whole population, a scenario known since the late 1970s as the Kessler syndrome. In this work we present Kessler: an open-source Python package for machine learning (ML) applied to collision avoidance. Kessler provides functionalities to import and export conjunction data messages (CDMs) in their standard format and predict the evolution of conjunction events based on explainable ML models. In Kessler we provide Bayesian recurrent neural networks that can be trained with existing collections of CDM data and then deployed in order to predict the contents of future CDMs in a given conjunction event, conditioned on all CDMs received up to now, with associated uncertainty estimates about all predictions. Furthermore Kessler includes a novel generative model of conjunction events and CDM sequences implemented using probabilistic programming, simulating the CDM generation process of the Combined Space Operations Center (CSpOC). The model allows Bayesian inference and also the generation of large datasets of realistic synthetic CDMs that we believe will be pivotal to enable further ML approaches given the sensitive nature and public unavailability of real CDM data.
Small bodies are ubiquitous in our Solar System, and they constitute a key element in understanding the origin of Earth and the emergence of life. Yet navigating a spacecraft around these bodies is very challenging, due to the difficulties of fully observing and characterizing these environments from the ground. These difficulties often translate into large uncertainties in the parameters that characterize these dynamical systems, ranging from uncertainties in the shape and mass distribution of the target bodies to those regarding the position and velocity of the spacecraft that navigates them. Small-body environments remain among the most perturbed and chaotic, making preliminary mission analysis particularly challenging. In particular, since the discovery of the first binary asteroids (Ida-Dactyl), binary systems have attracted much interest due to their considerable number (about 15% of the near-Earth orbit population) and their potential to reveal hints about the formation and evolution of our Solar System. Previous studies have modeled the dynamical environment of these systems using a perturbed version of the circular restricted three-body problem (CR3BP), where solar radiation pressure and irregularities of the gravity field of the body are accounted for. However, these analyses have predominantly focused on deterministic periodic orbits, which are then perturbed to examine sensitivity concerning initial conditions and/or parameter uncertainties. More recently, stochastic continuation approaches have been identified as a promising tool for integrating uncertainties into preliminary mission design for three-body systems. Unlike traditional iterative procedures, these techniques directly incorporate uncertainties, offering a more streamlined approach. By identifying natural regions of motion where spacecraft are statistically more likely to maintain periodic orbits, these methods offer a robust framework for bounded motion analysis. Expanding upon that, we extend the approach to small bodies, accounting for the irregularities in their gravity field. As a case study, we will apply these methodologies to the Hera spacecraft's mission to the Didymos & Dimorphos binary system, showing how these techniques can serve as a powerful preliminary mission design tool to identify safe regions of bounded motion around small bodies.
In the field of spaceflight mechanics and astrodynamics, determining eclipse regions is a frequent and critical challenge. This determination impacts various factors , including the acceleration induced by solar radiation pressure, the spacecraft power input, and its thermal state all of which must be accounted for in various phases of the mission design. This study leverages recent advances in neural image processing to develop fully dif-ferentiable models of eclipse regions for highly irregular celestial bodies. By utilizing test cases involving Solar System bodies previously visited by spacecraft, such as 433 Eros, 25143 Itokawa, 67P/Churyumov–Gerasimenko, and 101955 Bennu, we propose and study an implicit neural architecture defining the shape of the eclipse cone based on the Sun's direction. Employing periodic activation functions, we achieve high precision in modeling eclipse conditions. Furthermore, we discuss the potential applications of these differentiable models in spaceflight mechanics computations.
The organizers of the third Space Optimization Competition (SpOC)1 give a brief overview of the competition. We lay out the timeline and discuss administrative decisions. As was common in previous editions, the competition consists of three problems. We present them swiftly and provide their scientific background and motivation.
Dyson spheres are hypothetical megastructures encircling stars in order to harvest most of their energy output. During the 11th edition of the GTOC challenge, participants were tasked with a complex trajectory planning related to the construction of a precursor Dyson structure, a heliocentric ring made of twelve stations. To this purpose, we developed several new approaches that synthesize techniques from machine learning, combinatorial optimization, planning and scheduling, and evolutionary optimization effectively integrated into a fully automated pipeline. These include a machine learned transfer time estimator, improving the established Edelbaum approximation and thus better informing a Lazy Race Tree Search to identify and collect asteroids with high arrival mass for the stations; a series of optimally-phased low-thrust transfers to all stations computed by indirect optimization techniques, exploiting the synodic periodicity of the system; and a modified Hungarian scheduling algorithm, which utilizes evolutionary techniques to arrange a mass-balanced arrival schedule out of all transfer possibilities. We describe the steps of our pipeline in detail with a special focus on how our approaches mutually benefit from each other. Lastly, we outline and analyze the final solution of our team, ACT&Friends, which ranked second at the GTOC 11 challenge. •We developed a machine learning model to correct for Edelbaum approximation.•We deploy a Lazy Race Tree Search to find 10 trajectories for the asteroid allocation subtask of GTOC 11.•We compute the matrix M for the task assignment problem solving all well-phased opportunities to all 12 stations.•We map the scheduling aspects of the GTOC 11 problem to the assignment problem.•We combine the Hungarian algorithm with evolutionary techniques to solve the corresponding scheduling problem.
Numerical continuation techniques are powerful tools that have been extensively used to identify particular solutions of nonlinear dynamical systems and enable trajectory design in chaotic astrodynamics problems such as the Circular Restricted Three-Body Problem. However , the applicability of equilibrium points and periodic orbits may be questionable in real-world applications where the uncertainties of the initial conditions of the spacecraft and dynamical parameters of the problem (e.g., mass ratio parameter) are taken into consideration. Usually, the robustness of a candidate trajectory is tested via a two-step approach, whereby trajectories are first designed in a deterministic scenario, and then Monte Carlo methods are a posteriori used to check their robustness. While this strategy is ubiquitous in preliminary mission design, it can however lead to time-consuming and potentially not robust solutions, meaning that the found tra-jectories are not designed to account for uncertainties. Instead, the robustness of the determin-istic optimal solutions is usually ensured. Due to uncertain parameters and initial conditions, the spacecraft might not follow the reference periodic orbit owing to growing uncertainties that cause the satellite to deviate from its nominal path. Hence, it is crucial to keep track of the probability of finding the spacecraft in a given region. Building on previous work, we extend numerical continuation to moments of the distribution (i.e., stochastic continuation) by directly continuing moments of the probability density function of the spacecraft state. Only assuming normality of the initial conditions, and leveraging moment-generating functions, Isserlis' theorem, and the algebra of truncated polynomials, we propagate the distribution of the spacecraft state at consecutive surface of section crossings while retaining a symbolic map of the final moments of the distribution that depend on the initial mean and covariance matrix only. While the technique is only valid for initial Gaussian distributions, it does not assume that the distribution maintains its normality throughout the integration. The symbolic Poincaré map can then be directly used to evaluate the final moments of an initial distribution , as a function of the initial mean and covariance. This can therefore be used to accelerate the evolving step in the stochastic continuation procedure. The goal of the work is to offer a differential algebra-based general framework to continue 3D periodic orbits in the presence of uncertain dynamical systems. The proposed approach is compared against traditional Monte Carlo simulations to validate the uncertainty propagation approach and demonstrate the advantages of the proposed in terms of uncertainty propagation computational burden and access to higher-dimensional problems.
The risk of collisions in Earth’s orbit is growing markedly. In January 2021, SpaceX and OneWeb released an operator-to-operator fact sheet that highlights the critical reliance on conjunction data messages (CDMs) and observations, demonstrating the need for a diverse sensing environment for orbital objects. Recently, the University of Oxford and the University of Surrey developed, in collaboration with Trillium Technologies and the European Space Operations Center, an opensource Python package for modeling the spacecraft collision avoidance process, called Kessler. Such tools can be used for importing/exporting CDMs in their standard format, modeling the current low-Earth orbit (LEO) population and its short-term propagation from a given catalog file, as well as modeling the evolution of conjunction events based on the current population and observation scenarios, hence emulating the CDMs generation process of the Combined Space Operations Center (CSpOC). The model also provides probabilistic programming and ML tools to predict future collision events and to perform Bayesian inference (i.e., optimal use of all available observations). In the framework of a United Kingdom Space Agency-funded project, we analyze and study the impact of megaconstellations and observation models in the collision avoidance process. First, we monitor and report how the estimated collision risk and other quantities at the time of closest approach (e.g. miss distance, uncertainties, etc.) vary, according to different observation models, which emulate different radar observation accuracy. Then, we analyze the impact of future megaconstellations on the number of warnings generated from the increase in the number of conjunctions leading to an increased burden on space operators. FCC licenses were used to identify credible megaconstellation sources to understand how a potential consistent increase in active satellites will impact LEO situational safety. We finally present how our simulations help understand the impact of these future megaconstellations on the current population, and how we can devise better ground observation strategies to quantify future observation needs and reduce the burden on operators.
This paper explores the application of stochastic continuation methods in the context of mission analysis for spacecraft trajectories around libration points in the planar circular restricted three-body problem. Traditional deterministic approaches have limitations in accounting for uncertainties, requiring a two-step process involving Monte Carlo techniques for assessing the robustness of the deterministic design. This might lead to suboptimal solutions and to a long and time-consuming design process. Stochastic continuation methods, which extend numerical continuation techniques to moments of probability density functions, offer a promising alternative. This paper aims to pioneer the application of stochastic continuation procedures in mission analysis, incorporating and acknowledging the stochastic nature of spacecraft missions from the early design phases. By extending existing frameworks to handle fixed points of stroboscopic or Poincaré mappings, the study focuses on robustifying and enhancing trajectory design by considering uncertainties in the determination of periodic orbits. The proposed approach has the potential to discover new solutions that may remain hidden in deterministic analyses, offering improved mission design outcomes. Specifically, this work concentrates on the planar circular restricted three-body problem, assuming uncertainties in both initial conditions and the mass ratio parameter. Stochastic continuation is employed to identify equilibrium points and periodic orbits in this uncertain dynamical system. The generalization of steady states and periodic orbits in uncertain environments is discussed, demonstrating the effectiveness of stochastic continuation in identifying safe operational regions in uncertain astrodynamics problems.
Abstract Thermospheric density is one of the main sources of uncertainty in the estimation of satellites' position and velocity in low‐Earth orbit. This has negative consequences in several space domains, including space traffic management, collision avoidance, re‐entry predictions, orbital lifetime analysis, and space object cataloging. In this paper, we investigate the prediction accuracy of empirical density models (e.g., NRLMSISE‐00 and JB‐08) against black‐box machine learning (ML) models trained on precise orbit determination‐derived thermospheric density data (from CHAMP, GOCE, GRACE, SWARM‐A/B satellites). We show that by using the same inputs, the ML models we designed are capable of consistently improving the predictions with respect to state‐of‐the‐art empirical models by reducing the mean absolute percentage error (MAPE) in the thermospheric density estimation from the range of 40%–60% to approximately 20%. As a result of this work, we introduce Karman: an open‐source Python software package developed during this study. Karman provides functionalities to ingest and preprocess thermospheric density, solar irradiance, and geomagnetic input data for ML readiness. Additionally, it facilitates developing and training ML models on the aforementioned data and benchmarking their performance at different altitudes, geographic locations, times, and solar activity conditions. Through this contribution, we offer the scientific community a comprehensive tool for comparing and enhancing thermospheric density models using ML techniques. Plain Language Summary Accurately modeling the density of the thermosphere is pivotal for spacecraft operations such as collision avoidance, re‐entry prediction, and orbital lifetime analysis. In this study, our aim is twofold. First, we want to study and compare the performance of data‐driven machine learning (ML) models in predicting thermospheric density data against standard empirical models used in the field, which are used as baseline. By training ML models using precise orbit determination‐derived satellite data, we show that they can achieve significant performance improvement compared to empirical models, with a reduction of 61% in the mean absolute percentage error. Second, we also provide the community with a shared software framework that supports the ingestion of solar irradiance, geomagnetic, and thermospheric density data, as well as a training and benchmarking framework to develop ML models. This framework allows researchers and operators to both train their ML models and to compare them at different periods of the solar cycle, geomagnetic storm conditions, geographical locations, and times. Key Points Machine learning (ML) models can significantly outperform existing physics‐based thermospheric neutral density models on exactly the same inputs ML models can improve over NRLMSISE‐00 and JB‐08 empirical density models by 61% and 39% respectively in the mean absolute percentage error The software allows the creation of ML‐ready data for training and benchmarking new models, supporting solar irradiance and geomagnetic data
Accurate estimation of thermospheric density is critical for precise modeling of satellite drag forces in low Earth orbit (LEO). Improving this estimation is crucial to tasks such as state estimation, collision avoidance, and re-entry calculations. The largest source of uncertainty in determining thermospheric density is modeling the effects of space weather driven by solar and geomagnetic activity. Current operational models rely on ground-based proxy indices which imperfectly correlate with the complexity of solar outputs and geomagnetic responses. In this work, we directly incorporate NASA's Solar Dynamics Observatory (SDO) extreme ultraviolet (EUV) spectral images into a neural thermospheric density model to determine whether the predictive performance of the model is increased by using space-based EUV imagery data instead of, or in addition to, the ground-based proxy indices. We demonstrate that EUV imagery can enable predictions with much higher temporal resolution and replace ground-based proxies while significantly increasing performance relative to current operational models. Our method paves the way for assimilating EUV image data into operational thermospheric density forecasting models for use in LEO satellite navigation processes.
Recent events, such as the loss of 38 satellites by SpaceX due to a geomagnetic storm have highlighted the importance of having more accurate estimation and prediction of thermospheric density. Solar and geomagnetic activities wield significant influence over the behavior of the thermospheric density, exerting an important impact on spacecraft motion in low-Earth orbit (LEO). The impending Solar Cycle 25's peak arrives at a time when the number of operational satellites in LEO is surging, driven by the proliferation of mega-constellations. This escalating satellite presence, spanning sectors from defense to commercial applications, increases the intricacy of the operational environment. The accuracy of thermospheric neutral density models, which underpin crucial safety-oriented tasks like satellite collision avoidance and space traffic management, is therefore pivotal. While the importance of solar events on thermospheric density is apparent, currently, the influence of the Sun in thermospheric density models is only included in the form of solar proxies (such as F10.7). This can be underwhelming, leading to mispredictions of thermospheric density values. A shared framework that supports the ingestion of inputs from various sources to devise thermospheric density models, and where thermospheric density models can be compared, is currently lacking. Furthermore, the recent advancements in machine learning (ML) offer a unique opportunity to construct thermospheric density models that use these models to describe the relationship between the Sun and the Earth's thermosphere. For this reason, this study introduces an open-source software package, called Karman, to help solve this problem. Essential for this, are three steps: first, the preparation and ingestion of input data from several sources in an ML-readiness fashion. Then, the construction of ML models that can be trained on these datasets. Finally, the creation of a benchmarking platform to compare ML models against state-of-the-art empirical models, evaluating their performances under varying conditions, such as geomagnetic storm strength, altitude, and solar irradiance levels.The utility of this framework is demonstrated through various experiments, showcasing its effectiveness in both benchmarking density models and discerning factors driving thermospheric density variations. The study compares the performance of traditional empirical models (NRLMSISE-00 and JB-08) with machine learning models trained on identical inputs. The results reveal a consistent 20-40\% improvement in accuracy, highlighting the potential of machine learning techniques.One particularly significant area addressed by this research involves the incorporation of additional inputs to refine density estimations. Current approaches rely on solar proxies for estimating the Sun's impact on the thermosphere. However, it is suggested that direct Extreme Ultraviolet (EUV) irradiance data could enhance accuracy. The framework outlined in this paper enables the integration of such inputs, facilitating the validation of hypotheses and supporting the evolution of thermospheric density models.In conclusion, this study presents a comprehensive framework for advancing thermospheric density modeling in the context of LEO satellites. Through the development of neural network models, an extensive dataset, and a benchmarking platform, the paper contributes significantly to the improvement of satellite trajectory predictions. As the space environment becomes increasingly intricate, tools such as the presented framework are crucial for maintaining the safety and effectiveness of satellite operations in LEO.
Low-thrust trajectories play a crucial role in optimizing scientific output and cost efficiency in asteroid belt missions. Unlike high-thrust transfers, low-thrust trajectories require solving complex optimal control problems. This complexity grows exponentially with the number of asteroids visited due to orbital mechanics intricacies. In the literature, methods for approximating low-thrust transfers without full optimization have been proposed, including analytical and machine learning techniques. In this work, we propose new analytical approximations and compare their accuracy and performance to machine learning methods. While analytical approximations leverage orbit theory to estimate trajectory costs, machine learning employs a more black-box approach , utilizing neural networks to predict optimal transfers based on various attributes. We build a dataset of about 3 million transfers, found by solving the time and fuel optimal control problems , for different time of flights, which we also release open-source. Comparison between the two methods on this database reveals the superiority of machine learning, especially for longer transfers. Despite challenges such as multi revolution transfers, both approaches maintain accuracy within a few percent in the final mass errors, on a database of trajectories involving numerous asteroids. This work contributes to the efficient exploration of mission opportunities in the asteroid belt, providing insights into the strengths and limitations of different approximation strategies.
In this study, we investigate trajectories involving multiple impulses within the framework of a generic spacecraft dynamics. Revisiting the age-old query of "How many impulses?", we present novel manipulations heavily leveraging on the properties of the state transition matrix.Surprisingly, we are able to rediscover classical results leading to the introduction of a primer vector, albeit not making use of Pontryagin Maximum Principle as in the original developments by Lawden. Furthermore, our mathematical framework exhibits great flexibility and enables the introduction of what we term a "surrogate primer vector" extending a well known concept widely used in mission design. This enhancement allows to derive new simple optimality conditions that provide insights into the possibility to add and/or move multiple impulsive manoeuvres and improve the overall mass budget. This proves especially valuable in scenarios where a baseline trajectory arc is, for example, limited to a single impulse—an instance where traditional primer vector developments become singular and hinder conclusive outcomes.In demonstrating the practical application of the surrogate primer vector, we examine a specific case involving the four-body dynamics of a spacecraft within an Earth-Moon-Sun system. The system is characterized by the high-precision and differentiable VSOP2013 and ELP2000 ephemerides models. The focal point of our investigation is a reference trajectory representing a return from Mars, utilizing the weak stability boundary (WSB) of the Sun-Earth-Moon system. The trajectory incorporates two consecutive lunar flybys to insert the spacecraft into a lunar distant retrograde orbit (DRO). Conventionally, this trajectory necessitates a single maneuver at the DRO injection point.Prior to implementing the surrogate primer vector, a local optimization of the trajectory is performed. Upon application of the surrogate primer vector, we successfully identify potential maneuver injection points, strategically reducing the overall mission cost. The introduction of these additional maneuvers, followed by local optimization, validates that the revised trajectory indeed incurs a lower cost compared to the original configuration.
This study addresses optimal impulsive trajectory design within the Circular Restricted Three-Body Problem (CR3BP), presenting a global optimization-based approach to identify minimum ∆V transfers between periodic orbits , including heteroclinic connections. By combining a Monotonic Basin Hopping (MBH) algorithm with a sequential quadratic solver in a parallel optimization framework, a wide range of minimum ∆V transfers are efficiently found. To validate this approach, known connections from the literature are reproduced. Consequently, three-dimensional periodic orbits are explored and a systematic search for minimum propellant trajectories is conducted within a selected interval of Jacobi constants and a maximum time of flight. Analysis of the results reveals the presence of very low ∆V solutions and showcases the algo-rithm's effectiveness across various mission scenarios .
We introduce a novel neural architecture termed thermoNET, designed to represent thermospheric density in satellite orbital propagation using a reduced amount of differentiable computations. Due to the appearance of a neural network on the right-hand side of the equations of motion, the resulting satellite dynamics is governed by a NeuralODE, a neural Ordinary Differential Equation, characterized by its fully differentiable nature, allowing the derivation of variational equations (hence of the state transition matrix) and facilitating its use in connection to advanced numerical techniques such as Taylor-based numerical propagation and differential algebraic techniques. Efficient training of the network parameters occurs through two distinct approaches.In the first approach, the network undergoes training independently of spacecraft dynamics, engaging in a pure regression task against ground truth models, including JB-08 and NRLMSISE-00. In the second paradigm, network parameters are learned based on observed dynamics, adapting through ODE sensitivities. In both cases, the outcome is a flexible, compact model of the thermosphere density greatly enhancing numerical propagation efficiency while maintaining accuracy in the orbital predictions.
The Fokker-Planck equation is a partial differential equation that describes how the probability density function of an object state varies, when subject to deterministic and random forces. The solution to this equation is crucial in many space applications, such as space debris trajectory tracking and prediction, guidance navigation and control under uncertainties, space situational awareness, and mission analysis and planning. However, no general closed-form solutions are known and several methods exist to tackle its solution. In this work, we use a known technique to transform this equation into a set of linear ordinary differential equations in the context of orbital dynamics. In particular, we show the advantages of the applied methodology, which allows to decouple the time and state-dependent components and to retain the entire shape of the probability density function through time, in the presence of both deterministic and stochastic dynamics. With this approach, the probability density function values at future times and for different initial conditions can be computed without added costs, provided that some time-independent integrals are solved offline. We showcase the efficacy and use of this method on some orbital dynamics example, by also leveraging the use of automatic differentiation for efficiently computing the involved derivatives.