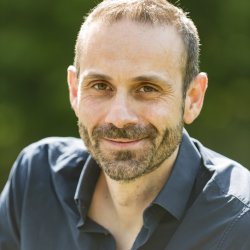
Professor Ferrante Neri
About
Biography
I am an all-round academic equally passionate about teaching and research. My teaching expertise is about Mathematical subjects for Computer Science while my research expertise lies at the intersection of Optimisation, Explainable AI, and Machine Learning. I am also happy to serve the community I work for, by undertaking managerial duties.
Before joining the faculty at Surrey I was at the University of Nottingham and previously I was at De Montfort University and at the University of Jyväskylä (Finland). I am a scholar in Optimisation and Computational Modelling. I work at the boundary between mathematical theory and practical engineering solutions. I’m the author of Linear Algebra for Computational Sciences and Engineering. I am also a very active editor for multiple journals including Information Sciences, Memetic Computing, and Integrated Computer-Aided Engineering.
My qualifications
Previous roles
Affiliations and memberships
ResearchResearch interests
My research expertise is in optimisation and machine learning. I am currently interested in algorithmic design for black-box optimisation problems informed by fitness landscape analysis. This topic lies at the intersection of optimisation, both exact and heuristic, and machine learning. I am also interested in applications of data science.
Historically, my main topic has been (and still is) the design and understanding of meta-heuristic algorithms. The main two subareas I contributed to are Memetic Computing and Differential Evolution. Since 2010 I am Chair/Vice-Chair of the IEEE Task Force on Memetic Computing.
Indicators of esteem
In the list of the top 2% scientists according to Stanford World Ranking of Scientists
Research interests
My research expertise is in optimisation and machine learning. I am currently interested in algorithmic design for black-box optimisation problems informed by fitness landscape analysis. This topic lies at the intersection of optimisation, both exact and heuristic, and machine learning. I am also interested in applications of data science.
Historically, my main topic has been (and still is) the design and understanding of meta-heuristic algorithms. The main two subareas I contributed to are Memetic Computing and Differential Evolution. Since 2010 I am Chair/Vice-Chair of the IEEE Task Force on Memetic Computing.
Indicators of esteem
In the list of the top 2% scientists according to Stanford World Ranking of Scientists
Supervision
Postgraduate research supervision
Current PhD students and starting month
Aisha E S E Saeid Aug 2022
Pengjin Wu Sep 2023
If you are looking for a PhD in a topic related to Artificial Intelligence, send me an email to have an informal chat about it. Please include in your email
- your CV
- a short description of the topic (maximum 2 pages) you would like to investigate
- a statement about how you are planning to fund your PhD studies, e.g., you are self-funded, have a scholarship, or are seeking for financial support
Be aware that PhD scholarships are available only at some points of the year through competitive processes (usually deadlines in January to start in October).
Postgraduate research supervision
Graduated PhD students
Hoang Lam Le, University of Nottingham. Thesis: “Novel Strategies to Accelerate Search Algorithms in Data Reduction", 2022
Shouyong Jiang, De Montfort University, "Dynamic Multi-Objective Optimisation", 2017
Michael Cochez, University of Jyväskylä, “Taming Big Knowledge Evolution”, 2016
Fabio Caraffini, De Montfort University, “Novel Memetic Structures for Continuous Optimisation”, 2014
Ilpo Poikolainen, University of Jyväskylä, “Simple Memetic Computing Structures for Global Optimization”, 2014
Annemari Soranto, University of Jyväskylä, “Interest-based topology management in unstructured peer-to-peer networks”, 2012
Ernesto Mininno, University of Jyväskylä, “Advanced Optimization Algorithms for Applications in Control Engineering”, 2011
Giovanni Iacca, University of Jyväskylä, “Memory-saving Optimization Algorithms for Systems with Limited Hardware”, 2011
Matthieu Weber, University of Jyväskylä, “Parallel Global Optimization, Structuring Populations in Differential Evolution”, 2010
Ville Tirronen with University of Jyväskylä, “Global Optimization using Memetic Differential Evolution with Applications to Low-Level Machine Vision”, 2008
Teaching
Happiness is to be understood – Georgi Polonsky – (We’ll Live Till Monday)
Current Teaching
Computational Intelligence (Lectures, Week 1-11, Semester 1)
Foundations of Computing II (Linear Algebra Section, Week 1-6 Semester 2)
Past Teaching
Undergraduate Modules
- 2020-2022 Mathematics for Computer Scientists 2, University of Nottingham, UK
- 2019-2022 Languages and Computation, University of Nottingham, UK
- 2018-2019 Abstract Algebra I and II, De Montfort University, UK
- 2015-2018 Linear Algebra and Discrete Mathematics, De Montfort University, UK
- 2014-2018 Foundations and Algebra, De Montfort University, UK
- 2012-2013 Introduction to Artificial Intelligence and Mobile Robotics, De Montfort University, UK
- 2012-2013 Connected Devices, De Montfort University, UK
Postgraduate Modules
- 2013-2015 Computational Intelligence Optimisation, De Montfort University, UK
- 2007-2012 Research Projects, University of Jyväskylä, Finland
- 2007 Evolutionary Computational Intelligence, University of Jyväskylä, Finland
International Research/Postgraduate Modules
- 2020 Automatic Design of Optimisation Algorithms in the Continuous Domain, NATCOR Course Heuristics & Approximation Algorithms, Nottingham, UK
- 2008 Medicine+Computer Science=Computational Medicine; Computational Intelligence for Multi-drug Therapies, Winter School on Mathematical and Computational Biology, University of Newcastle, Australia
- 2007 Recent Advances in Evolutionary Computing, 17th Jyväskylä Summer School, University of Jyväskylä, Finland
Sustainable development goals
My research interests are related to the following:
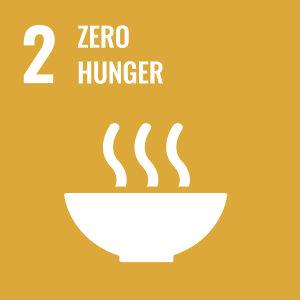
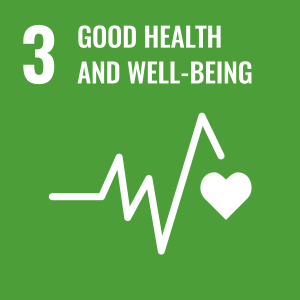
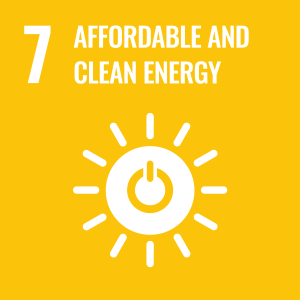
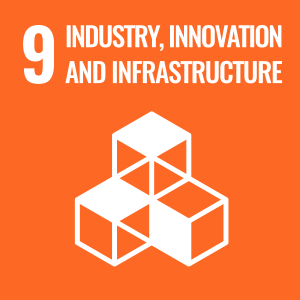
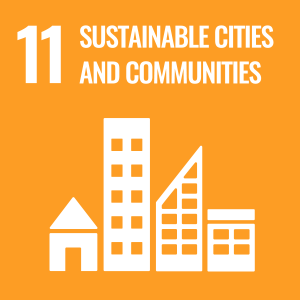
Publications
Evolutionary neural architecture search (ENAS) treats neural network design as an optimisation problem and addresses it via evolutionary computation. Despite being flexible and enabling automated design, ENAS typically suffers from high computational costs due to the need to train a network at each fitness evaluation. Surrogate-assisted ENAS methods mitigate the severity of this challenge by replacing the computationally expensive fitness function with an approximate computationally cheap fitness function for some fitness evaluations of the run. Currently, a major research challenge in the field is the smooth integration of such surrogate models (and, often, data collection mechanisms) within ENAS frameworks. This paper puts forth a simple yet effective way to address this challenge. During the initial stage of the optimisation, the proposed algorithm, score predictor-assisted ENAS (SPNAS), evolves a small population of candidate architectures using ground truth fitness, i.e., the testing error rate of the network following its training. The data collected in this stage are then used to train a multi-layer perceptron network that builds an alternative fitness function. Unlike algorithms in previous studies, this novel alternative fitness does not approximate the error rate but is designed to preserve its order relation over populations of candidate architectures. Thus, this approach naturally allows for a computationally cheap population ranking. Most of the evolution is then carried out with the surrogate (i.e., alternative) fitness on a large population without retraining the surrogate model or calculating the ground truth fitness. Experiments conducted on the EvoXBench platform show that on its seven search spaces, SPNAS achieves excellent results in terms of error rate despite the modest use of ground-truth fitness calls.
With global warming driving more extreme weather events, real-time monitoring and forecasting of severe convective weather are increasingly critical. This letter presents a multitask generative adversarial network (MT-GAN) to improve nowcasting (short-term prediction) of severe weather, specifically precipitation and lightning. MT-GAN forecasts with 6-min temporal resolution and up to 60-min lead times. Its dual-stream architecture handles radar reflectivity and lightning data, using an encoder-decoder framework with SimVP for temporal feature extraction. A two-level feature fusion enhances spatiotemporal predictions, while a Patch-D discriminator improves realism. Tested on a central China dataset, MT-GAN outperforms ConvLSTM and PredRNN++ in image quality and nowcasting accuracy, with a stronger emphasis on convective regions for better lightning initiation detection.
Cloud cover experiences rapid fluctuations, significantly impacting the irradiance reaching the ground and causing frequent variations in photovoltaic power output. Accurate detection of thin and fragmented clouds is crucial for reliable photovoltaic power generation forecasting. In this paper, we introduce a novel cloud detection method, termed Adaptive Laplacian Coordination Enhanced Cross-Feature U-Net (ALCU-Net). This method augments the traditional U-Net architecture with three innovative components: an Adaptive Feature Coordination (AFC) module, an Adaptive Laplacian Cross-Feature U-Net with a Multi-Grained Laplacian-Enhanced (MLE) feature module, and a Criss-Cross Feature Fused Detection (CCFE) module. The AFC module enhances spatial coherence and bridges semantic gaps across multi-channel images. The Adaptive Laplacian Cross-Feature U-Net integrates features from adjacent hierarchical levels, using the MLE module to refine cloud characteristics and edge details over time. The CCFE module, embedded in the U-Net decoder, leverages criss-cross features to improve detection accuracy. Experimental evaluations show that ALCU-Net consistently outperforms existing cloud detection methods, demonstrating superior accuracy in identifying both thick and thin clouds and in mapping fragmented cloud patches across various environments, including oceans, polar regions, and complex ocean-land mixtures.
Feature selection (FS) is recognized for its role in enhancing the performance of learning algorithms, especially for high-dimensional datasets. In recent times, FS has been framed as a multi-objective optimization problem, leading to the application of various multi-objective evolutionary algorithms (MOEAs) to address it. However, the solution space expands exponentially with the dataset's dimensionality. Simultaneously, the extensive search space often results in numerous local optimal solutions due to a large proportion of unrelated and redundant features [H. Adeli and H. S. Park, Fully automated design of super-high-rise building structures by a hybrid ai model on a massively parallel machine, AI Mag. 17 (1996) 87–93]. Consequently, existing MOEAs struggle with local optima stagnation, particularly in large-scale multi-objective FS problems (LSMOFSPs). Di®erent LSMOFSPs generally exhibit unique characteristics, yet most existing MOEAs rely on a single candidate solution generation strategy (CSGS), which may be less e±cient for diverse LSMOFSPs [H. S. Park and H. Adeli, Distributed neural dynamics algorithms for optimization of large steel structures, J. Struct. Eng. ASCE 123 (1997) 880–888; M. Aldwaik and H. Adeli, Advances in optimization of highrise building structures, Struct. Multidiscip. Optim. 50 (2014) 899–919; E. G. Gonzalez, J. R. Villar, Q. Tan, J. Sedano and C. Chira, An e±cient multi-robot path planning solution using a* and coevolutionary algorithms, Integr. Comput. Aided Eng. 30 (2022) 41–52]. Moreover, selecting an appropriate MOEA and determining its corresponding parameter values for a speci¯ed LSMOFSP is time-consuming. To address these challenges, a multi-objective self-adaptive particle swarm optimization (MOSaPSO) algorithm is proposed, combined with a rapid nondominated sorting approach. MOSaPSO employs a self-adaptive mechanism, along with ¯ve modi¯ed e±cient CSGSs, to generate new solutions. Experiments were conducted on ten datasets, and the results demonstrate that the number of features is e®ectively reduced by MOSaPSO while lowering the classi¯cation error rate. Furthermore, superior performance is observed in comparison to its counterparts on both the training and test sets, with advantages becoming increasingly evident as the dimensionality increases.
An important problem in data science, feature selection (FS) consists of finding the optimal subset of features and eliminating irrelevant or redundant features. The FS task on high-dimensional data is challenging for the FS methods currently available in the literature. To overcome this limitation, we propose a novel feature selection method called External Attention-Based Feature Ranker for Large-Scale Feature Selection (EAR-FS) whose function is based on the logic of an attention mechanism and a hybrid metaheuristic. EAR-FS comprises three interdependent modules: 1) in the training module design, a multilayer perceptron network endowed with an attention module is trained to fit the dataset; 2) in feature ranking by attention, the trained attention module is used for attention updating and to rank features according to their importance; 3) in subset generation, a two-stage heuristic approach is applied to determine a small number of features that still guarantee high-accuracy performance. The experimental benchmark comprised 26 datasets of small, large and very large sizes, ranging from 15 to 12, 533 features. Experiments performed against the state-of-the-art algorithms of FS show that * Corresponding author our algorithm is efficient at selecting a small number of features from large datasets while guaranteeing excellent levels of classification accuracy. For instance , EAR-FS demonstrated its capability to reduce the features of the 11 Tumor dataset by 97% while maintaining a classifier accuracy of over 93%.
Spiking neural membrane systems (SN P systems) are a class of bio-inspired models inspired by the activities and connectivity of neurons. Extensive studies have been made on SN P systems with synchronisation-based communication, while further efforts are needed for the systems with rhythm-based communication. In this work, we design an asynchronous SN P system with resonant connections where all the enabled neurons in the same group connected by resonant connections should instantly produce spikes with the same rhythm. In the designed system, each of the 3 modules collaboratively work to implement one types of the 3 operations associated with the edge detection of digital images, and they collaborate each other through the resonant connections. An algorithm called EDSNP for edge detection is proposed to simulate the working of the designed asynchronous SN P system. A quantitative analysis of EDSNP and the related methods for edge detection is conducted to evaluate the performance of EDSNP. The performance of the EDSNP in processing the testing images are superior to the compared methods, based on the quantitative metrics of accuracy, error rate, mean square error, peak signal-to-noise ratio, and true positive rate. The results indicate the potential of the temporal firing and the proper neuronal connections in the SN P system to achieve good performance in edge detection.
Existing unsupervised domain adaptation (UDA) methods rely on aligning the features from the source and target domains explicitly or implicitly in a common space (i.e., the domain invariant space). Explicit distribution matching ignores the discriminability of learned features, while the implicit counterpart such as self-supervised learning suffers from pseudo-label noises. With distribution alignment, it is challenging to acquire a common space which maintains fully the discriminative structure of both domains. In this work, we propose a novel HomeomorphisM Alignment (HMA) approach characterized by aligning the source and target data in two separate spaces. Specifically, an invertible neural network based homeomorphism is constructed. Distribution matching is then used as a sewing up tool for connecting this homeomorphism mapping between the source and target feature spaces. Theoretically, we show that this mapping can preserve the data topological structure (e.g., the cluster/group structure). This property allows for more discriminative model adaptation by leveraging both the original and transformed features of source data in a supervised manner, and those of target domain in an unsupervised manner (e.g., prediction consistency). Extensive experiments demonstrate that our method can achieve the state-of-the-art results. Code is released at https://github.com/buerzlh/HMA.
Fitness landscape analysis (FLA) refers to a set of techniques to characterise optimisation problems. This paper presents an FLA of three types of genetic programming (GP) benchmarks: parity, symbolic regression, and artificial ant. We applied a modern graph-based FLA tool called Local Optima Networks and several classical FLA metrics (fitness distance correlation, neutrality, and ruggedness measures) to study the tree-based GP search spaces. Our analysis shows that the search spaces for all problems contain many local optima and are highly deceptive. The parity problems are highly rugged and neutral. Conversely, the problems of symbolic regression are less rugged and neutral. Finally, the artificial ant problem is highly rugged but less neutral. Our results indicate that a mutation in deep nodes makes finding the global optimum difficult.
This article proposes a procedure to perform an intelligent initialization for population-based algorithms. The proposed pre-processing procedure, namely Cluster-Based Population Initialization (CBPI) consists of three consecutive stages. At the first stage, the individuals belonging to a randomly sampled population undergo two subsequent local search algorithms, i.e. a simple local search that performs moves along the axes and Rosenbrock algorithm. At the second stage, the solutions processed by the two local searches undergo the K-means clustering algorithm and are grouped into sets on the basis of their euclidean distance. At the third stage the best individuals belonging to each cluster are saved into the initial population of a generic optimization algorithm. If the population has not been yet filled, the other individuals of the population are sampled within the clusters by using a fitness-based probabilistic criterion. This three stage procedure implicitly performs an initial screening of the problem features in order to roughly estimate the most interesting regions of the decision space. The proposed CBPI has been tested on multiple classical and modern Differential Evolution variants, on a wide array of test problems and dimensionality values as well as on a real-world problem. The proposed intelligent sampling appears to have a significant impact on the algorithmic functioning as it consistently enhances the performance of the algorithms with which it is integrated. (C) 2014 Elsevier Inc. All rights reserved.
This paper proposes and compares two approaches to defeat the noise due the measurement errors in control system design of electric drives. The former is based on a penalized fitness and two cooperative-competitive survivor selection schemes, the latter is based on a survivor selection scheme which makes use of the tolerance interval related to the noise distribution. These approaches use adaptive rules in parameter setting to execute both the explicit and the implicit averaging in order to obtain the noise defeating in the optimization process with a relatively low number of fitness evaluations. The results show that the two approaches differently bias the population diversity and that the first can outperform the second but requires a more accurate parameter setting.
This article deals with optimization problems to be solved in the absence of a full power computer device. The goal is to solve a complex optimization problem by using a control card related to portable devices, e.g. for the control of commercial robots. In order to handle this class of optimization problems, a novel Memetic Computing approach is presented. The proposed algorithm employs a Differential Evolution framework which instead of processing an actual population of candidate solutions, makes use of a statistical representation of the population which evolves over time. In addition, the framework uses a stochastic local search algorithm which attempts to enhance the performance of the elite. In this way, the memetic logic of performing the optimization by observing the decision space from complementary perspectives can be integrated within computational devices characterized by a limited memory. The proposed algorithm, namely Memetic compact Differential Evolution (McDE), has been tested and compared with other algorithms belonging to the same category for a real-world industrial application, i.e. the control system design of a cartesian robot for variable mass movements. For this real-world application, the proposed McDE displays high performance and has proven to considerably outperform other compact algorithms representing the current state-of-the-art in this sub-field of computational intelligence.
This paper proposes a memetic approach for solving complex optimization problems characterized by a noisy fitness function. The proposed approach aims at solving highly multivariate and multi-modal landscapes which are also affected by a pernicious noise. The proposed algorithm employs a Differential Evolution framework and combines within this three additional algorithmic components. A controlled randomization of scale factor and crossover rate are employed which should better handle uncertainties of the problem and generally enhance performance of the Differential Evolution. Two combined local search algorithms applied to the scale factor, during offspring generation, should enhance performance of the Differential Evolution framework in the case of multi-modal and high dimensional problems. An on-line statistical test aims at assuring that only strictly necessary samples are taken and that all pairwise selections are properly performed. The proposed algorithm has been tested on a various set of test problems and its behavior has been studied, dependent on the dimensionality and noise level. A comparative analysis with a standard Differential Evolution, a modern version of Differential Evolution employing randomization of the control parameters and four metaheuristics tailored to optimization in a noisy environment has been carried out. One of these metaheuristics is a classical algorithm for noisy optimization while the other three are modern Differential Evolution based algorithms for noisy optimization which well represent the state-of-the-art in the field. Numerical results show that the proposed memetic approach is an efficient and robust alternative for various and complex multivariate noisy problems and can be exported to real-world problems affected by a noise whose distribution can be approximated by a Gaussian distribution.
Pattern Search is a family of optimisation algorithms that improve upon an initial solution by performing moves along the directions of a basis of vectors. In its original definition Pattern Search moves along the directions of each variable. Amongst its advantages, the algorithm does not require any knowledge of derivatives or analytical expression of the function to optimise. However, the performance of Pattern Search is heavily problem dependent since the search directions can be very effective on some problems and lead to poor performance on others. The present article proposes a novel enhancement of Pattern Search that explores the space by using problem-dependent search directions. Some points are sampled within the basin of attraction and the diagonalisation of the covariance matrix associated with the distribution of these points is performed. The proposed Covariance Pattern Search improves upon an initial point by varying it along the directions identified by the eigenvectors of the covariance matrix.
This paper studies the use of multiple scale factor values within distributed Differential Evolution structures employing the so-called exponential crossover. Four different scale factor schemes are proposed, tested, compared and analyzed. Two schemes simply employ multiple scale factor values and two also include an update logic during the evolution. The four schemes have been integrated for comparison within three recently proposed distributed Differential Evolution structures and tested on several various test problems. The results are then compared to those of a previous study where the so-called binomial crossover was employed. Numerical results show that, when associated to the exponential crossover, the employment of multiple scale factors is not systematically beneficial and in some cases even detrimental to the performance of the algorithm. The exponential crossover accentuates the exploitative character of the Differential Evolution, which cannot always be counterbalanced by the increase in the explorative aspect of the algorithm introduced by the employment of multiple scale factor values.
The performance of most metaheuristic algorithms depends on parameters whose settings essentially serve as a key function in determining the quality of the solution and the efficiency of the search. A trend that has emerged recently is to make the algorithm parameters automatically adapt to different problems during optimization, thereby liberating the user from the tedious and time-consuming task of manual setting. These fine-tuning techniques continue to be the object of ongoing research. Differential evolution (DE) is a simple yet powerful population-based metaheuristic. It has demonstrated good convergence, and its principles are easy to understand. DE is very sensitive to its parameter settings and mutation strategy; thus, this study aims to investigate these settings with the diverse versions of adaptive DE algorithms. This study has two main objectives: (1) to present an extension for the original taxonomy of evolutionary algorithms (EAs) parameter settings that has been overlooked by prior research and therefore minimize any confusion that might arise from the former taxonomy and (2) to investigate the various algorithmic design schemes that have been used in the different variants of adaptive DE and convey them in a new classification style. In other words, this study describes in depth the structural analysis and working principle that underlie the promising and recent work in this field, to analyze their advantages and disadvantages and to gain future insights that can further improve these algorithms. Finally, the interpretation of the literature and the comparative analysis of the algorithmic schemes offer several guidelines for designing and implementing adaptive DE algorithms. The proposed design framework provides readers with the main steps required to integrate any proposed meta-algorithm into parameter and/or strategy adaptation schemes.
Several variants of spiking neural P systems (SNPS) have been presented in the literature to perform arithmetic operations. However, each of these variants was designed only for one specific arithmetic operation. In this paper, a complete arithmetic calculator implemented by SNPS is proposed. An application of the proposed calculator to information fusion is also proposed. The information fusion is implemented by integrating the following three elements: (1) an addition and subtraction SNPS already reported in the literature; (2) a modified multiplication and division SNPS; (3) a novel storage SNPS, i.e. a method based on SNPS is introduced to calculate basic probability assignment of an event. This is the first attempt to apply arithmetic operation SNPS to fuse multiple information. The effectiveness of the presented general arithmetic SNPS calculator is verified by means of several examples.
Optimization Spiking Neural P System (OSNPS) is the first membrane computing model to directly derive an approximate solution of combinatorial problems with a specific reference to the 0/1 knapsack problem. OSNPS is composed of a family of parallel Spiking Neural P Systems (SNPS) that generate candidate solutions of the binary combinatorial problem and a Guider algorithm that adjusts the spiking probabilities of the neurons of the P systems. Although OSNPS is a pioneering structure in membrane computing optimization, its performance is competitive with that of modern and sophisticated metaheuristics for the knapsack problem only in low dimensional cases. In order to overcome the limitations of OSNPS, this paper proposes a novel Dynamic Guider algorithm which employs an adaptive learning and a diversity-based adaptation to control its moving operators. The resulting novel membrane computing model for optimization is here named Adaptive Optimization Spiking Neural P System (AOSNPS). Numerical result shows that the proposed approach is effective to solve the 0/1 knapsack problems and outperforms multiple various algorithms proposed in the literature to solve the same class of problems even for a large number of items (high dimensionality). Furthermore, case studies show that a AOSNPS is effective in fault sections estimation of power systems in different types of fault cases: including a single fault, multiple faults and multiple faults with incomplete and uncertain information in the IEEE 39 bus system and IEEE 118 bus system.
Back propagation (BP) is widely used for parameter search of fully-connected layers in many neural networks. Although BP has the potential of quickly converging to a solution, due to its gradient-based nature, it tends to fall into a local optimum. Metaheuristics such as evolutionary computation (EC) techniques, as gradient-free methods, may have excellent global search capability due to their stochastic nature. However, these techniques tend to perform worse than BP in terms of convergence speed. In this paper, a hybrid gradient descent search algorithm (HGDSA) is proposed for training the parameters in fully-connected neural networks. HGDSA initially searches the space extensively by means of an ensemble of gradient descent strategies in the early stage and then uses BP as an exploitative local search operator. Moreover, a self-adaptive method which selects strategies and updates the learning rates of strategies has been designed and embedded in the global search operators to prevent stagnation in local optima. To verify the effectiveness of HGDSA, experiments were performed on eleven classification datasets. Experimental results demonstrate that the proposed HGDSA possesses both powerful global and local search abilities. Furthermore, the proposed approach appears to be promising also on high-dimensional datasets.
Feature selection (FS) is an important research topic in machine learning. Usually, FS is modelled as a bi-objective optimization problem whose objectives are: 1) classification accuracy; 2) number of features. One of the main issues in real-world applications is missing data. Databases with missing data are likely to be unreliable. Thus, FS performed on a data set missing some data is also unreliable. In order to directly control this issue plaguing the field, we propose in this study a novel modelling of FS: we include reliability as the third objective of the problem. In order to address the modified problem, we propose the application of the non-dominated sorting genetic algorithm-III (NSGA-III). We selected six incomplete data sets from the University of California Irvine (UCI) machine learning repository. We used the mean imputation method to deal with the missing data. In the experiments, k-nearest neighbors (K-NN) is used as the classifier to evaluate the feature subsets. Experimental results show that the proposed three-objective model coupled with NSGA-III efficiently addresses the FS problem for the six data sets included in this study.
With the development of deep learning, the design of an appropriate network structure becomes fundamental. In recent years, the successful practice of Neural Architecture Search (NAS) has indicated that an automated design of the network structure can efficiently replace the design performed by human experts. Most NAS algorithms make the assumption that the overall structure of the network is linear and focus solely on accuracy to assess the performance of candidate networks. This paper introduces a novel NAS algorithm based on a multi-objective modeling of the network design problem to design accurate Convolutional Neural Networks (CNNs) with a small structure. The proposed algorithm makes use of a graph-based representation of the solutions which enables a high flexibility in the automatic design. Furthermore, the proposed algorithm includes novel ad-hoc crossover and mutation operators. We also propose a mechanism to accelerate the evaluation of the candidate solutions. Experimental results demonstrate that the proposed NAS approach can design accurate neural networks with limited size.
Pattern Search is a family of gradient-free direct search methods for numerical optimisation problems. The characterising feature of pattern search methods is the use of multiple directions spanning the problem domain to sample new candidate solutions. These directions compose a matrix of potential search moves, that is the pattern. Although some fundamental studies theoretically indicate that various directions can be used, the selection of the search directions remains an unaddressed problem. The present article proposes a procedure for selecting the directions that guarantee high convergence/high performance of pattern search. The proposed procedure consists of a fitness landscape analysis to characterise the geometry of the problem by sampling points and selecting those whose objective function values are below a threshold. The eigenvectors of the covariance matrix of this distribution are then used as search directions for the pattern search. Numerical results show that the proposed method systematically outperforms its standard counterpart and is competitive with modern complex direct search and metaheuristic methods.
Mathematics, despite being the foundation of computer science, is nowadays often considered a totally separate subject. The fact that many jobs in computer science do not explicitly require any specific mathematical knowledge posed questions about the importance of mathematics within computer science undergraduate curricula. In many educational systems, a prior high school knowledge of mathematics is often not a mandatory requirement to be enrolled into a degree of computer science. On the other hand, several studies report that mathematics is important to computer scientists since it provides essential analytical and critical skills and since many professional and research tasks in computer science require an in-depth understanding of mathematical concepts. From this assumption, this article proposes an analysis of the cohort of computer science’ students, with a specific reference to British Universities, and identifies some challenges that lecturers of mathematical subjects normally face. On the basis of this analysis this article proposes two teaching techniques to promote effective learning. The proposed techniques aim at addressing the diversity of cohorts in terms of mathematical background and skepticism from part of the cohort of students to consider mathematics as an essential element of their education. Numerical results indicate the validity and effectiveness of the proposed teaching techniques.
Neural architecture search has attracted great attention in the research community and has been successfully applied in the industry recently. Differentiable architecture search (DARTS) is an efficient architecture search method. However, the networks searched by DARTS are often unstable due to the large gap in the architecture depth between the search phase and the verification phase. In addition, due to unfair exclusive competition between different candidate operations, DARTS is prone to skip connection aggregation, which may cause performance collapse. In this article, we propose progressive partial channel connections based on channel attention for differentiable architecture search (PA-DARTS) to solve the above problems. In the early stage of searching, we only select a few key channels for convolution using channel attention and reserve all candidate operations. As the search progresses, we gradually increase the number of channels and eliminate unpromising candidate operations to ensure that the search phase and verification phase are all carried out on 20 cells. Due to the existence of the partial channel connections based on channel attention, we can eliminate the unfair competition between operations and increase the stability of PA-DARTS. Experimental results showed that PA-DARTS could achieve 97.59% and 83.61% classification accuracy on CIFAR-10 and CIFAR-100, respectively. On ImageNet, our algorithm achieved 75.3% classification accuracy.
Generative adversarial networks (GANs) are a powerful generative technique but frequently face challenges with training stability. Network architecture plays a significant role in determining the final output of GANs, but designing a fine architecture demands extensive domain expertise. This paper aims to address this issue by searching for high-performance generator’s architectures through neural architecture search (NAS). The proposed approach, called evolutionary weight sharing generative adversarial networks (EWSGAN), is based on weight sharing and comprises two steps. First, a supernet of the generator is trained using weight sharing. Second, a multi-objective evolutionary algorithm (MOEA) is employed to identify optimal subnets from the supernet. These subnets inherit weights directly from the supernet for fitness assessment. Two strategies are used to stabilise the training of the generator supernet: a fair single-path sampling strategy and a discarding strategy. Experimental results indicate that the architecture searched by our method achieved a new state-of-the-art among NAS-GAN methods with a Fréchet inception distance (FID) of 9.09 and an inception score (IS) of 8.99 on the CIFAR-10 dataset. It also demonstrates competitive performance on the STL-10 dataset, achieving FID of 21.89 and IS of 10.51.
Simultaneous Localization and Mapping (SLAM) is a fundamental element of intelligent mobile robotics. An efficient and reliable point cloud feature extraction plays an important role in SLAM technology. This paper studies the feature extraction method of the point cloud in the process of constructing a high-precision map by the 3D lidar sensor, and proposes a method based on the point cloud. A valuable contribution of the proposed method is that the extracted point cloud features can be observed repeatedly. Through comparative experiments, it is verified that the number of extracted features based on point cloud segmentation is significantly reduced, the calculation time is significantly reduced, and it is more conducive to the subsequent point cloud feature registration. Therefore, the method proposed in this paper provides important enlightenment for improving the accuracy and efficiency of constructing a high-precision map of an intelligent mobile robot by 3D lidar.
In this paper, a hybrid feature selection algorithm based on a multi-objective algorithm with ReliefF (MOFS-RFGA) is proposed. Combining the advantages of filter and wrapper methods, the two types of algorithms are hybridized to improve the capability when solving feature selection problems. First, the ReliefF algorithm is used to score the features according to their importance to the instance class. Then, the feature scoring information is used to initialize the population. Also, a new crossover and mutation operator are designed in this paper to guide the crossover and mutation process based on feature scoring information to improve the search direction of MOFS-RFGA in search space and enhance the convergence performance. In the experiments, MOFS-RFGA is compared with seven advanced multi-objective feature selection algorithms on 20 datasets, and the results show that MOFS-RFGA can fully utilize the advantages of filter and wrapper methods, beating the excellent performance of the comparison algorithms on a large number of datasets, and ensuring good classification performance while cutting a large number of features.
This paper proposes a novel and unconventional Memetic Computing approach for solving continuous optimization problems characterized by memory limitations. The proposed algorithm, unlike employing an explorative evolutionary framework and a set of local search algorithms, employs multiple exploitative search within the main framework and performs a multiple step global search by means of a randomized perturbation of the virtual population corresponding to a periodical randomization of the search for the exploitative operators. The proposed Memetic Computing approach is based on a populationless (compact) evolutionary framework which, instead of processing a population of solutions, handles its statistical model. This evolutionary framework is based on a Differential Evolution which cooperatively employs two exploitative search operators: the first is based on a standard Differential Evolution mutation and exponential crossover, and the second is the trigonometric mutation. These two search operators have an exploitative action on the algorithmic framework and thus contribute to the rapid convergence of the virtual population towards promising candidate solutions. The action of these search operators is counterbalanced by a periodical stochastic perturbation of the virtual population, which has the role of "disturbing" the excessively exploitative action of the framework and thus inhibits its premature convergence. The proposed algorithm, namely Disturbed Exploitation compact Differential Evolution, is a simple and memory-wise cheap structure that makes use of the Memetic Computing paradigm in order to solve complex optimization problems. The proposed approach has been tested on a set of various test problems and compared with state-of-the-art compact algorithms and with some modern population based meta-heuristics. Numerical results show that Disturbed Exploitation compact Differential Evolution significantly outperforms all the other compact algorithms present in literature and reaches a competitive performance with respect to modern population algorithms, including some memetic approaches and complex modern Differential Evolution based algorithms. In order to show the potential of the proposed approach in real-world applications, Disturbed Exploitation compact Differential Evolution has been implemented for performing the control of a space robot by simulating the implementation within the robot micro-controller. Numerical results show the superiority of the proposed algorithm with respect to other modern compact algorithms present in literature. (C) 2011 Elsevier Inc. All rights reserved.
In this paper, a recently proposed single-solution memetic computing optimization method, namely three stage optimization memetic exploration (3SOME), is used to implement a self-tuning PID controller on board of a mobile robot. More specifically, the optimal PID parameters minimizing a measure of the following error on a path-following operation are found, in real-time, during the execution of the control loop. The proposed approach separates the control and the optimization tasks, and uses simple operating system primitives to share data. The system is able to react to modifications of the trajectory, thus endowing the robot with intelligent learning and self-configuration capabilities. A popular commercial robotic tool, i.e. the Lego Mindstorms robot, has been used for testing and implementing this system. Tests have been performed both in simulations and in a real Lego robot. Experimental results show that, compared to other online optimization techniques and to empiric PID tuning procedures, 3SOME guarantees a robust and efficient control behaviour, thus representing a valid alternative for self-tuning control systems. (C) 2012 Elsevier B.V. All rights reserved.
Spiking Neural P Systems are Neural System models characterized by the fact that each neuron mimics a biological cell and the communication between neurons is based on spikes. In the Spiking Neural P systems investigated so far, the application of evolution rules depends on the contents of a neuron (checked by means of a regular expression). In these P systems, a specified number of spikes are consumed and a specified number of spikes are produced, and then sent to each of the neurons linked by a synapse to the evolving neuron. In the present work, a novel communication strategy among neurons of Spiking Neural P Systems is proposed. In the resulting models, called Spiking Neural P Systems with Communication on Request, the spikes are requested from neighboring neurons, depending on the contents of the neuron (still checked by means of a regular expression). Unlike the traditional Spiking Neural P systems, no spikes are consumed or created: the spikes are only moved along synapses and replicated (when two or more neurons request the contents of the same neuron). The Spiking Neural P Systems with Communication on Request are proved to be computationally universal, that is, equivalent with Turing machines as long as two types of spikes are used. Following this work, further research questions are listed to be open problems.
This paper is the part H of a paper composed of two parts. In the part I a memetic approach consisting of applying a local search to the scale factor of a Differential Evolution framework in order to generate an off-spring with a high quality was proposed. The part H proposes the application of the scale factor local search within a Differential Evolution framework which integrates a self-adaptive update of the control parameters. In other words, unlike for the part 1, the scale factor local search is applied to a an algorithmic framework characterized by multiple scale factors over the individuals of the population and scale factor updates during the evolution. Two simple local search logics have been tested, the first one employs the golden section search and the second one a hill-climber. The local search algorithms thus assist the global search and generates offspring with high performance which are subsequently supposed to promote the generation of better solutions within the evolutionary framework. Numerical results show that the hybridization is beneficial and able to outperform in many cases both the classical Differential Evolution and a Self-Adaptive Differential Evolution recently proposed in literature.
This article proposes a compact algorithm for optimisation in noisy environments. This algorithm has a compact structure and employs differential evolution search logic. Since it is a compact algorithm, it does not store a population of solutions but a probabilistic representation of the population. This kind of algorithmic structure can be implemented in those real-world problems characterized by memory limitations. The degree of randomization contained in the compact structure allows a robust behaviour in the presence of noise. In addition the proposed algorithm employs the noise analysis survivor selection scheme. This scheme performs an analysis of the noise and automatically performs a re-sampling of the solutions in order to ensure both reliable pairwise comparisons and a minimal cost in terms of fitness evaluations. The noise analysis component can be reliably used in noise environments affected by Gaussian noise which allow an a priori analysis of the noise features. This situation is typical of problems where the fitness is computed by means of measurement devices. An extensive comparative analysis including four different noise levels has been included. Numerical results show that the proposed algorithm displays a very good performance since it regularly succeeds at handling diverse fitness landscapes characterized by diverse noise amplitudes.
This paper proposes the application of Differential Evolution with Scale Factor Local Search to optimally design the control system of a permanent-magnet synchronous motor which is part of the UPR probe, element of the more complex PAIS system. The PAIS system is an innovative Wireless Sensor Network for the environmental monitoring whose main purpose is to reduce the wildfire damages with early detection and suppression. To operate a complete environmental monitoring, each UPR probe is usually located in wild areas and exposed to severe weather conditions, which can substantially degrade the performance of the whole system and, in particular, of the motor drive. Under such conditions, an automatic technique to periodically check and improve the performance of the motor moving the UPR is needed. Numerical results show that the Differential Evolution with Scale Factor Local Search offers excellent results in terms of convergence speed and final solution detected, serving as a perfect tool for the self-commissioning of the UPR position control.
In the realm of practical problem-solving, multi-objective optimisation problems with redundant variables and indefinite objective functions (MOPRVIF) are becoming increasingly prevalent. MOPRVIF involve determining the optimal decision variables that optimise multiple objectives, leveraging the relational data of a set of variables and multiple objectives. For these problems, this paper focuses on the following two issues: one is the demand for a unified computational model to solve this problem; the other is how to improve the algorithm's deep intelligent search capability. In this regard,this paper designs a dual data-driven multi-objective optimisation method. The method used consisted of four parts: elimination of redundant variables (ERV), objective function construction (OFC), selection evolution operator (SEO), and multi-objective evolutionary algorithm (MOEA). MOEA was the main focus of the method. ERV is data preparation and variable selection according to multiple objectives. OFC involves constructing the relationship model between variables and objectives, and a high-accuracy model is important for guaranteeing reliable results. Furthermore, SEO can adjust the evolution operator during a deep search. This is an important guarantee for deep, intelligent search. MOEA combined OFC and SEO to form the final solution algorithm—Dual Data Driven Multi-Objective Evolutionary Algorithm (DDMOEA). DDMOEA was explored using two different disciplinary problems of drug compound optimisation and wild blueberry cultivation and benchmarks were selected. The first two problem domains are distinct. The first problem is more complex than the second; however, both encompass redundant variables and indefinite objective functions. Benchmarks are utilised independently to gauge the profound intelligent search capability. The experiments affirm that the dual data-driven optimization approach proposed in this paper is effective, practical, and scalable.
Intuitively, a set is considered to be discrete if it is composed of isolated elements, whereas it is considered to be continuous if it is composed of infinite and contiguous elements and does not contain “holes”.
Memetic Algorithms (MAs) are population-based metaheuristics composed of an evolutionary framework and a set of local search algorithms which are activated within the generation cycle of the external framework, see [376]. The earliest MA implementation has been given in [621] in the context of the Travelling Salesman Problem (TSP) while an early systematic definition has been presented in [615]. The concept of meme is borrowed from philosophy and is intended as the unit of cultural transmission. In other words, complex ideas can be decomposed into memes which propagate andmutate within a population.Culture, in this way, constantly undergoes evolution and tends towards progressive improvements. Strong ideas tend to resist and be propagated within a community while weak ideas are not selected and tend to disappear. In the metaphor, the ideas are the search operators: the fittest tend to be employed while the inadequate ones are likely to disappear.
Recently, nature has stimulated many successful techniques, algorithms, and computational applications allowing conventionally difficult problems to be solved through novel computing systems. Nature-Inspired Informatics for Intelligent Applications and Knowledge Discovery: Implications in Business, Science, and Engineering provides the latest findings in nature-inspired algorithms and their applications for breakthroughs in a wide range of disciplinary fields. This defining reference collection contains chapters written by leading researchers and well-known academicians within the field, offering readers a valuable and enriched accumulation of knowledge. Recently, nature has stimulated many successful techniques, algorithms, and computational applications allowing conventionally difficult problems to be solved through novel computing systems. Nature-Inspired Informatics for Intelligent Applications and Knowledge Discovery: Implications in Business, Science, and Engineering provides the latest findings in nature-inspired algorithms and their applications for breakthroughs in a wide range of disciplinary fields. This defining reference collection contains chapters written by leading researchers and well-known academicians within the field, offering readers a valuable and enriched accumulation of knowledge.
Feature selection (FS) is an important data pre-processing technique in classification. In most cases, FS can improve classification accuracy and reduce feature dimension, so it can be regarded as a multi-objective optimization problem. Many evolutionary computation techniques have been applied to FS problems and achieved good results. However, an increase in data dimension means that search difficulty also greatly increases, and EC algorithms with insufficient search ability maybe only find sub-optimal solutions in high probability. Moreover, an improper initial population may negatively affect the convergence speed of algorithms. To solve the problems highlighted above, this paper proposes MOEA-ISa: a multi-objective evolutionary algorithm with interval based initialization and self-adaptive crossover operator for large-scale FS. The proposed interval based initialization can limit the number of selected features for solution to improve the distribution of the initial population in the target space and reduce the similarity of the initial population in the decision space. The proposed self-adaptive crossover operator can determine the number of nonzero genes in offspring according to the similarity of parents, and it combines with the feature weights obtained by ReliefF method to improve the quality of offspring. In the experiments, the proposed algorithm was compared with six other algorithms on 13 benchmark UCI datasets and two benchmark LIBSVM datasets, and an ablation experiment was performed on MOEA-ISa. The results show that MOEA-ISa's performance is better than the six other algorithms for solving large-scale FS problems, and the proposed interval based initialization and self-adaptive crossover operator can effectively improve the performance of MOEA-ISa. The source code of MOEA-ISa is available on GitHub at https://github.com/xueyunuist/MOEA-ISa.
To guarantee their locomotion, biped robots need to walk stably. The latter is achieved by a high performance in joint control. This article addresses this issue by proposing a novel human-simulated fuzzy (HF) membrane control system of the joint angles. The proposed control system, human-simulated fuzzy membrane controller (HFMC), contains several key elements. The first is an HF algorithm based on human-simulated intelligent control (HSIC). This HF algorithm incorporates elements of both multi-mode proportional-derivative (PD) and fuzzy control, aiming at solving the chattering problem of multi-mode switching while improving control accuracy. The second is a membrane architecture that makes use of the natural parallelisation potential of membrane computing to improve the real-time performance of the controller. The proposed HFMC is utilised as the joint controller for a biped robot. Numerical tests in a simulation are carried out with the planar and slope walking of a five-link biped robot, and the effectiveness of the HFMC is verified by comparing and evaluating the results of the designed HFMC, HSIC and PD. Experimental results demonstrate that the proposed HFMC not only retains the advantages of traditional PD control but also improves control accuracy, real-time performance and stability.
An intrusion detection system (IDS) is a software application or hardware appliance that monitors traffic on networks and systems to search for suspicious activity and known threats, sending up alerts when it finds such items. In these recent years, attention has been focused on artificial neural networks (ANN) techniques, especially Deep Learning approach on anomaly-based detection techniques; because of the huge and unbalanced datasets, IDS encounters real data processing problems. Thus, different techniques have been presented which can handle this problem. In this paper, a deep learning model or technique based on the Convolutional Neural Network (CNN) is proposed to improve the accuracy and precisely detect intrusions. The entire proposed model is divided into four stages: data collection, data pre-processing, the training and testing stage, and performance evaluation.
The super-fit scheme, consisting of injecting an individual with high fitness into the initial population of an algorithm, has shown to be a simple and effective way to enhance the algorithmic performance of the population-based algorithm. Whether the super-fit individual is based on some prior knowledge on the optimization problem or is derived from an initial step of pre-processing, e.g. a local search, this mechanism has been applied successfully in various examples of evolutionary and swarm intelligence algorithms. This paper presents an unconventional application of this super-fit scheme, where the super-fit individual is obtained by means of the Covariance Adaptation Matrix Evolution Strategy (CMA-ES), and fed to a single solution local search which perturbs iteratively each variable. Thus, compared to other super-fit schemes, the roles of super-fit individual generator and global optimizer are switched. To prevent premature convergence, the local search employs a re-sampling mechanism which inherits parts of the best individual while randomly sampling the remaining variables. We refer to such local search as Re-sampled Inheritance Search (RIS). Tested on the CEC 2013 optimization benchmark, the proposed algorithm, named CMA-ES-RIS, displays a respectable performance and a good balance between exploration and exploitation, resulting into a versatile and robust optimization tool.
The search for the optimum in a mixed continuous-combinatorial space is a challenging task since it requires operators that handle both natures of the search domain. Instance reduction (IR), an important pre-processing technique in data science, is often performed in separated stages, combining instance selection (IS) first, and subsequently instance generation (IG). This paper investigates a fast optimisation approach for IR considering the two stages at once. This approach, namely Accelerated Pattern Search with Variable Solution Size (APS-VSS), is characterised by a variable solution size, an accelerated objective function computation, and a single-point memetic structure designed for IG. APS-VSS is composed of a global search crossover and three local searches (LS). The global operator prevents premature convergence to local optima, whilst the three LS algorithms optimise the reduced set (RS). Furthermore, by using the k-nearest neighbours algorithm as a base classifier, APS-VSS exploits the search logic of the LS to accelerate, by orders of magnitude, objective function computation. The experiments show that APS-VSS outperforms existing algorithms using the single-point structure, and is statistically as competitive as state-of-the-art IR techniques regarding accuracy and reduction rates, while reducing significantly the runtime.
In data mining, instance reduction is a key data preprocessing step that simplifies and cleans raw data, by either selecting or creating new samples, before applying a learning algorithm. This usually yields to a complex large scale and computationally expensive optimisation problem which has been typically tackled by sophisticated population-based metaheuristics. Unlike the recent literature, in order to accomplish this target, this article proposes the use of a simple local search algorithm and its integration with an optional surrogate assisted model. This local search, in accordance with variable decomposition techniques for large scale problems, perturbs an n-dimensional vector along the directions identified by its design variables one by one. Empirical results in 40 small data sets show that, despite its simplicity, the proposed baseline local search on its own is competitive with more complex algorithms representing the state-of-the-art for instance reduction in classification problems. The use of the proposed local surrogate model enables a reduction of the computationally expensive objective function calls with accuracy test results overall comparable with respect to its baseline counterpart.
This paper proposes a novel Differential Evolution based algorithmic structure for solving continuous global optimization problems. The proposed structure makes use of the recently introduced concept of compact Differential Evolution as a search unit. Several compact units evolve simultaneously and interact in order to solve the optimization problem. In other words, the compact units are supposed to explore the decision space from diverse perspectives. The search work performed by the compact units is coordinated by a global supervision unit which processes by means of a global search the achievements obtained by the various compact units. More specifically, each compact unit performs a step of compact Differential Evolution and then feeds the achieved results to a global optimizer which recombines during one generation the candidate solutions and returns the improved genotypes to the corresponding compact units. In this implementation we selected as a global supervision unit a Differential Evolution algorithm with self-adaptive control parameters previously proposed in literature. The concept of global supervision, here introduced, appears to be very promising as it allows the improvement and development of the results locally obtained by each compact unit, thus preventing premature convergence of each unit and promoting a successful continuation of the search. Numerical results show that the resulting algorithm considered in this study displays a promising performance for a set of challenging test problems and is competitive with the-state-of-the-art Differential Evolution based algorithms.
The Moving Picture Experts Group (MPEG) video-based point cloud compression (V-PCC) standard encodes a dynamic point cloud by first converting it into one geometry video and one color video and then using a video coder to compress the two video sequences. We first propose analytical models for the distortion and bitrate of the V-PCC reference software, where the models’ variables are the quantization step sizes used in the encoding of the geometry and color videos. Unlike previous work, our analytical models are functions of the quantization step sizes of all frames in a group of frames. Then, we use our models and an implementation of the differential evolution algorithm to efficiently minimize the distortion subject to a constraint on the bitrate. Experimental results on six dynamic point clouds show that, compared to the state-of-the-art, our method achieves an encoding with a smaller error to the target bitrate (4.65% vs. 11.94% on average) and a slightly lower rate-distortion performance (on average, the increase in Bjøntegaard delta (BD) distortion is 0.27, and the increase in BD rate is 8.40%).
This paper proposes a novel distributed differential evolution algorithm, namely Distributed Differential Evolution with Explorative-Exploitative Population Families (DDE-EEPF). In DDE-EEPF the sub-populations are grouped into two families. Sub-populations belonging to the first family have constant population size, are arranged according to a ring topology and employ a migration mechanism acting on the individuals with the best performance. This first family of sub-populations has the role of exploring the decision space and constituting an external evolutionary framework. The second family is composed of sub-populations with a dynamic population size: the size is progressively reduced. The sub-populations belonging to the second family are highly exploitative and are supposed to quickly detect solutions with a high performance. The solutions generated by the second family then migrate to the first family. In order to verify its viability and effectiveness, the DDE-EEPF has been run on a set of various test problems and compared to four distributed differential evolution algorithms. Numerical results show that the proposed algorithm is efficient for most of the analyzed problems, and outperforms, on average, all the other algorithms considered in this study.
The design of each agent composing a Memetic Algorithm (MA) is a delicate task which often requires prior knowledge of the problem to be effective. This paper proposes a method to analyse one feature of the fitness landscape, that is the epistasis, with the aim of designing efficient local search algorithms for Memetic Frameworks. The proposed Analysis of Epistasis performs a sampling of points within the basin of attraction and builds a data set containing those candidate solutions whose objective function value falls below a threshold.The covariance matrix associated with this data set is then calculated. The eigenvectors of this covariance matrix are then computed and used as the reference system for the local search: a change of variables is performed and then the local search is performed on the new variables. The Analysis of Epistasis has been implemented on the three local search algorithms composing a popular MA called Multiple Trajectory Search (MTS). Numerical results show that the three modified local search algorithms outperform their original counterparts.
In this paper the Authors examine the optimization of the design of unequally spaced grounding grids equipped with rods. To this end, the genetic algorithms method proposed optimizes both the grounding grid cost and the maximum touch voltage it generates. The method has been successfully applied to a real case.
This paper proposes re-sampled inheritance search (RIS), a novel algorithm for solving continuous optimization problems. The proposed method, belonging to the class of Memetic Computing, is very simple and low demanding in terms of memory employment and computational overhead. The RIS algorithm is composed of a stochastic sample mechanism and a deterministic local search. The first operator randomly generates a solution and then recombines it with the best solution detected so far (inheritance) while the second operator searches in an exploitative way within the neighbourhood indicated by the stochastic operator. This extremely simple scheme is shown to display a very good performance on various problems, including hard to solve multi-modal, highly-conditioned, large scale problems. Experimental results show that the proposed RIS is a robust scheme that competitively performs with respect to recent complex algorithms representing the-state-of-the-art in modern continuous optimization. In order to further prove its applicability in real-world cases, RIS has been used to perform the control system tuning for yaw operations on a helicopter robot. Experimental results on this real-world problem confirm the value of the proposed approach.
This paper proposes a novel implementation of memetic structure for continuous optimization problems. The proposed algorithm, namely Differential Evolution with Concurrent Fitness Based Local Search (DEcfbLS), enhances the DE performance by including a local search concurrently applied on multiple individuals of the population. The selection of the individuals undergoing local search is based on a fitness-based adaptive rule. The most promising individuals are rewarded with a local search operator that moves along the axes and complements the normal search moves of DE structure. The application of local search is performed with a shallow termination rule. This design has been performed in order to overcome the limitations within the search logic on the original DE algorithm. The proposed algorithm has been tested on various problems in multiple dimensions. Numerical results show that the proposed algorithm is promising candidate to take part to competition on Real-Parameter Single Objective Optimization at CEC-2013. A comparison against modern meta-heuristics confirms that the proposed algorithm robustly displays a good performance on the testbed under consideration.
T his article performs a study on correlation between pairs of variables in dependence on the problem dimensionality. Two tests, based on Pearson and Spearman coefficients, have been designed and used in this work. In total, 86 test problems ranging between 10 and 1000 variables have been studied. If the most commonly used experimental conditions are used, the correlation between pairs of variables appears, from the perspective of the search algorithm, to consistently decrease. This effect is not due to the fact that the dimensionality modifies the nature of the problem but is a consequence of the experimental conditions: the computational feasibility of the experiments imposes an extremely shallow search in case of high dimensions. An exponential increase of budget and population with the dimensionality is still practically impossible. Nonetheless, since real- world application may require that large scale problems are tackled despite of the limited budget, an algorithm can quickly improve upon initial guesses if it integrates the knowledge that an apparent weak correlation between pairs of variables occurs, regardless the nature of the problem.
In this article, various metaheuristics for a numerical optimization problem with application to Electric Impedance Tomography are tested and compared. The experimental setup is composed of a real valued Genetic Algorithm, the Differential Evolution, a self adaptive Differential Evolution recently proposed in literature, and two novel Memetic Algorithms designed for the problem understudy. The two proposed algorithms employ different algorithmic philosophies in the field of Memetic Computing. The first algorithm integrates a local search into the operations of the offspring generation, while the second algorithm applies a local search to individuals already generated in the spirit of life-time learning. Numerical results show that the fitness landscape and difficulty of the optimization problem heavily depends on the geometrical configuration, as well the proposed Memetic Algorithms seem to be more promising when the geometrical conditions make the problem harder to solve.
A fast adaptive memetic algorithm (FAMA) is proposed which is used to design the optimal control system for a permanent-magnet synchronous motor. The FAMA is a memetic algorithm with a dynamic parameter setting and two local searchers adaptively launched, either one by one or simultaneously, according to the necessities of the evolution. The FAMA has been tested for both offline and online optimization. The former is based on a simulation of the whole system-control system and plant-using a model obtained through identification tests. The online optimization is model free because each fitness evaluation consists of an experimental test on the real motor drive. The proposed algorithm has been compared with other optimization approaches, and a matching analysis has been carried out offline and online. Excellent results are obtained in terms of optimality, convergence, and algorithmic efficiency. Moreover, the FAMA has given very robust results in the presence of noise in the experimental system.
This paper proposes an artificial player for the Turtle Race game, with the goal of creating an opponent that will provide some amount of challenge to a human player. Turtle Race is a game of imperfect information, where the players know which one of the game pieces is theirs, but do not know which ones belong to the other players and which ones are neutral. Moreover, movement of the pieces is determined by cards randomly drawn from a deck. The artificial player is based oil a non-linear neural network whose training is performed by means of a novel parallel evolutionary algorithm with fitness diversity adaptation. The algorithm handles, in parallel, several populations which cooperate with each other 133, exchanging individuals when a population registers a diversity loss. Four Popular evolutionary algorithms have been tested for the proposed parallel framework. Numerical results show that an evolution strategy can be very efficient for the problem under examination and that the proposed adaptation tends to improve upon the algorithmic performance Without any addition in computational overhead. The resulting artificial player displayed a high performance against other artificial players and a challenging behavior for expert human players.
Fitness landscape analysis (FLA) refers to a set of techniques that allow for the characterisation, visualisation and comprehension of the trends of objective functions within their decision spaces. Two of the important features estimated through FLA are ruggedness, i.e. the number and distribution of optima within the decision space, and neutrality, i.e. the width, distribution and frequency of areas with one objective function value. Recent studies have proposed the application of FLA to the training problem in supervised learning. The present paper extends previous results on ruggedness and neutrality to investigate the loss landscape of LeNet-5. More specifically, we demonstrate that the neutrality threshold is an important metaparameter and that its optimisation is required to correctly assess the ruggedness and neutrality of a problem. Furthermore, this study investigates the difference between the landscape perceived by a learning algorithm and the actual landscape. The obtained numerical results indicate that the division of datasets into batches causes an increase in perceived ruggedness. As a result, modern training algorithms that make use of division into batches, such as Adam, overestimate ruggedness.
This paper proposes a speed-sensorless stator field orientation control (SFOC) of IM drives based on a delayed-state Kalman filter (DSKF) optimally tuned with the differential evolution (DE) algorithm. The DSKF estimates the stator flux components in the stationary reference frame, using the derivatives of the stator flux components as mathematical model and the stator voltage equations as observation model. The DE algorithm gives the values of the covariance matrices of model and measurement errors to obtain optimal performance from the DSKF. The DE is a reliable and versatile function optimizer that has not yet been widely implemented in the field of electrical drives. It performs extremely well for the problem under analysis. The DE outperformed the best known GAs on proposed optimization problem. The paper investigates the responses to a load speed reversal as well as to a training test at low speed and the experiments show the low-speed performance of the sensorless control scheme using the new optimized DSKF.
Compact algorithms are Estimation of Distribution Algorithms which mimic the behavior of population-based algorithms by means of a probabilistic representation of the population of candidate solutions. Compared to an actual population, a probabilistic model requires a much smaller memory, which allows algorithms with limited memory footprint. This feature is extremely important in some engineering applications, e.g. robotics and real-time control systems. This paper proposes a compact implementation of Bacterial Foraging Optimization (cBFO). cBFO employs the same chemotaxis scheme of population-based BFO, but without storing a swarm of bacteria. Numerical results, carried out on a broad set of test problems with different dimensionalities, show that cBFO, despite its minimal hardware requirements, is competitive with other memory saving algorithms and clearly outperforms its population-based counterpart.
We show some mathematical links between partially observable (PO) games in which information is regu larly revealed, and simultaneous actions games. Using this, we study the extension of Monte-Carlo Tr ee Search algorithms to PO games and to games with simultaneous actions. We apply the results to Urb an Rivals, a free PO internet card game with more than 10 millions of registered users.
This chapter studies and analyzes Memetic Differential Evolution (MDE) Frameworks for designing digital filters, which aim at detecting paper defects produced during an industrial process. MDE Frameworks employ the Differential Evolution (DE) as an evolutionary framework and a list of local searchers adaptively coordinated by a control scheme. Here, three different variants of MDE are taken into account and their features and performance are compared. The binomial explorative features of the DE framework in contraposition to the exploitative features of the local searcher are analyzed in detail in light of the stagnation prevention problem, typical for the DE. Much emphasis in this chapter is given to the various adaptation systems and to their applicability this image processing problem.
The optimization of robot controller parameters is a crucial task for enhancing robot performance, yet it often presents challenges due to the complexity of multi-objective, multi-dimensional multi-parameter optimization. This paper introduces a novel approach aimed at efficiently optimizing robot controller parameters to enhance its motion performance. While spiking neural P systems have shown great potential in addressing optimization problems, there has been limited research and validation concerning their application in continuous numerical, multi-objective, and multi-dimensional multi-parameter contexts. To address this research gap, our paper proposes the Entropy-Weighted Numerical Gradient Optimization Spiking Neural P System, which combines the strengths of entropy weighting and spiking neural P systems. First, the introduction of entropy weighting eliminates the subjectivity of weight selection, enhancing the objectivity and reproducibility of the optimization process. Second, our approach employs parallel gradient descent to achieve efficient multi-dimensional multi-parameter optimization searches. In conclusion, validation results on a biped robot simulation model show that our method markedly enhances walking performance compared to traditional approaches and other optimization algorithms. We achieved a velocity mean absolute error at least 35% lower than other methods, with a displacement error two orders of magnitude smaller. This research provides an effective new avenue for performance optimization in the field of robotics.
This paper proposes a novel algorithm for addressing multi-objective optimisation problems, by employing a progressive preference articulation approach to decision making. This enables the interactive incorporation of problem knowledge and decision maker preferences during the optimisation process. A novel progressive preference articulation mechanism, derived from a statistical technique, is herein proposed and implemented within a multi-objective framework based on evolution strategy search and hypervolume indicator selection. The proposed algorithm is named the Weighted Z-score Covariance Matrix Adaptation Pareto Archived Evolution Strategy with Hypervolume-sorted Adaptive Grid Algorithm (WZ-HAGA). WZ-HAGA is based on a framework that makes use of evolution strategy logic with covariance matrix adaptation to perturb the solutions, and a hypervolume indicator driven algorithm to select successful solutions for the subsequent generation. In order to guide the search towards interesting regions, a preference articulation procedure composed of four phases and based on the weighted z-score approach is employed. The latter procedure cascades into the hypervolume driven algorithm to perform the selection of the solutions at each generation. Numerical results against five modern algorithms representing the state-of-the-art in multi-objective optimisation demonstrate that the proposed WZ-HAGA outperforms its competitors in terms of both the hypervolume indicator and pertinence to the regions of interest.
At an abstract level, memetic algorithms can be seen as a broad class of populationbased stochastic local search (SLS) methods, where a main theme is “exploiting allavailableknowledge about a problem,” see also Moscato and Cotta [618], page 105. The most wide-spread implementation of this theme is probably that of improving some or all individuals in the population by some local search method. This combination of a population-based, global search and a single-solution local search is a very appealing one. The global search capacity of the evolutionary part of a memetic algorithm takes care of exploration, trying to identify the most promising search space regions; the local search part scrutinizes the surroundings of some initial solution, exploiting it in this way. This idea is not only an appealing one, it is also practically a very successful one. In fact, for a vast majority of combinatorial optimization problems and, as it is also becoming more clear in recent research, also for many continuous optimization problems this combination leads to some of best performing heuristic optimization algorithms.
This paper presents an experimental study on memetic strategies to enhance the performance of population-based metaheuristics for multimodal optimisation. The purpose of this work is to devise some recommendations about algorithmic design to allow a successful combination of local search and niching techniques. Six memetic strategies are presented and tested over five population-based algorithms endowed with niching techniques. Experimental results clearly show that local search enhances the performance of the framework for multimodal optimisation in terms of both peak ratio and success rate. The most promising results are obtained by the variants that employ an archive that pre-selects the solutions undergoing local search thus avoiding computational waste. Furthermore, promising results are obtained by variants that reduce the exploitation pressure of the population-based framework by using a simulated annealing logic in the selection process, leaving the exploitation task to the local search.
The file attached to this record is the authors final peer reviewed version. The publisher's final version can be found by following the DOI link. Three Stage Optimal Memetic Exploration (3SOME) is a recently proposed algorithmic framework which sequentially perturbs a single solution by means of three operators. Although 3SOME proved to be extremely successful at handling high-dimensional multi-modal landscapes, its application to non-separable fitness functions present some flaws. This paper proposes three possible variants of the original 3SOME algorithm aimed at improving its performance on non-separable problems. The first variant replaces one of the 3SOME operators, namely the middle distance exploration, with a rotation-invariant Differential Evolution (DE) mutation scheme, which is applied on three solutions sampled in a progressively shrinking search space. In the second proposed mechanism, a micro-population rotation-invariant DE is integrated within the algorithmic framework. The third approach employs the search logic (1+1)-Covariance Matrix Adaptation Evolution Strategy, aka (1+1)-CMA-ES. In the latter scheme, a Covariance Matrix adapts to the landscape during the optimization in order to determine the most promising search directions. Numerical results show that, at the cost of a higher complexity, the three approaches proposed are able to improve upon 3SOME performance for non-separable problems without an excessive performance deterioration in the other problems.
The impetus behind data analytics and integration is the need for greater insight and data visibility, but since a growing share of our data is multimedia, there is a parallel need for methods that can align multimedia data. This paper explores georeferencing, which is used to combine spatial datasets and used here to align map images to 2D GIS models. This paper surveys various approaches for building the key components of a georeferencing solution, notes their strengths and weaknesses, and comments on their trajectory to help orient future work. The implementation presented here uses Hough transforms for feature detection, nearest neighbor correspondences with simplistic similarity measures, and a population based optimizer. The comparison among metaheuristics has shown that Differential Evolution (DE) frameworks appear especially suited for this problem. In particular, the controlled randomization of DE parameters appears to display the best performance in terms of execution time and competitive performance in terms of function evaluations even with respect to more complex memetic implementations.
In this paper the Authors suggest a software based on the genetic algorithms to optimize the design of a road lighting installation so as to reduce the use of the energy per year. The method has been applied to a real case.
Point clouds are sets of points used to visualize three-dimensional (3D) objects. Point clouds can be static or dynamic. Each point is characterized by its 3D geometry coordinates and attributes such as color. High-quality visualizations often require millions of points, resulting in large storage and transmission costs, especially for dynamic point clouds. To address this problem, the moving picture experts group has recently developed a compression standard for dynamic point clouds called video-based point cloud compression (V-PCC). The standard generates two-dimensional videos from the geometry and color information of the point cloud sequence. Each video is then compressed with a video coder, which converts each frame into frequency coefficients and quantizes them using a quantization parameter (QP). Traditionally, the QPs are severely constrained. For example, in the low-delay configuration of the V-PCC reference software, the quantization parameter values of all the frames in a group of pictures are set to be equal. We show that the rate-distortion performance can be improved by relaxing this constraint and treating the QP selection problem as a multi-variable constrained combinatorial optimization problem, where the variables are the QPs. To solve the optimization problem, we propose a variant of the differential evolution (DE) algorithm. Differential evolution is an evolutionary algorithm that has been successfully applied to various optimization problems. In DE, an initial population of randomly generated candidate solutions is iteratively improved. At each iteration, mutants are generated from the population. Crossover between a mutant and a parent produces offspring. If the performance of the offspring is better than that of the parent, the offspring replaces the parent. While DE was initially introduced for continuous unconstrained optimization problems, we adapt it for our constrained combinatorial optimization problem. Also, unlike standard DE, we apply individual mutation to each variable. Furthermore, we use a variable crossover rate to balance exploration and exploitation. Experimental results for the low-delay configuration of the V-PCC reference software show that our method can reduce the average bitrate by up to 43% compared to a method that uses the same QP values for all frames and selects them according to an interior point method.
In many modern machine learning models, attention mechanisms play a crucial role in processing data and identifying significant parts of the inputs, whether these are text or images. This selective focus enables subsequent stages of the model to achieve improved classification performance. Traditionally, attention mechanisms are applied as a preprocessing substructure before a neural network, such as in encoder/decoder architectures. In this paper, we extend the application of attention mechanisms to intermediate stages of data propagation within machine learning models. Specifically, we propose a Gen-eralised Attention Mechanism (GAM), which can be integrated before each layer of a neural network for classification tasks. The proposed GAM allows for at each layer/step of the machine learning architecture identification of the most relevant sections of the intermediate results. Our experimental results demonstrate that incorporating the proposed GAM into various machine learning models consistently enhances the accuracy of these models. This improvement is achieved with only a marginal increase in the number of parameters, which does not significantly affect the training time.
Machine learning algorithms are commonly used for quickly and efficiently counting people from a crowd. Test-time adaptation methods for crowd counting adjust model parameters and employ additional data augmentation to better adapt the model to the specific conditions encountered during testing. The majority of current studies concentrate on unsupervised domain adaptation. These approaches commonly perform hundreds of epochs of training iterations, requiring a sizable number of unannotated data of every new target domain apart from annotated data of the source domain. Unlike these methods, we propose a meta-test-time adaptive crowd counting approach called CrowdTTA, which integrates the concept of test-time adaptation into the meta-learning framework and makes it easier for the counting model to adapt to the unknown test distributions. To facilitate the reliable supervision signal at the pixel level, we introduce uncertainty by inserting the dropout layer into the counting model. The uncertainty is then used to generate valuable pseudo labels, serving as effective supervisory signals for adapting the model. In the context of meta-learning, one image can be regarded as one task for crowd counting. In each iteration, our approach is a dual-level optimization process. In the inner update, we employ a self-supervised consistency loss function to optimize the model so as to simulate the parameters update process that occurs during the test phase. In the outer update, we authentically update the parameters based on the image with ground truth, improving the model’s performance and making the pseudo labels more accurate in the next iteration. At test time, the input image is used for adapting the model before testing the image. In comparison to various supervised learning and domain adaptation methods, our results via extensive experiments on diverse datasets showcase the general adaptive capability of our approach across datasets with varying crowd densities and scales.
Real-world optimisation problems pose domain specific challenges that often require an ad-hoc algorithmic design to be efficiently addressed. The present paper investigates the optimisation of a key stage in data mining, known as instance reduction, which aims to shrink the input data prior to applying a learning algorithm. Performing a smart selection or creation of a reduced number of samples that represent the original data may become a complex large-scale optimisation problem, characterised by a computationally expensive objective function, which has been often tackled by sophisticated population-based metaheuristics that suffer from a high runtime. Instead, by following the Ockham’s Razor in Memetic Computing, we propose a Memetic Computing approach that we refer to as fast Single-Point Memetic Structure with Accelerated Local Search (SPMS-ALS). Using the k-nearest neighbours algorithm as base classifier, we first employ a simple local search for large-scale problems that exploits the search logic of Pattern Search, perturbing an -dimensional vector along the directions identified by its design variables one by one. This point-by-point perturbation mechanism allows us to design a strategy to re-use most of the calculations previously made to compute the objective function of a candidate solution. The proposed Accelerated Local Search is integrated within a single-point memetic framework and coupled with a resampling mechanism and a crossover. A thorough experimental analysis shows that SPMS-ALS, despite its simplicity, displays an excellent performance which is as good as that of the state-of-the-art while reducing up to approximately 85% of the runtime with respect to any other algorithm that performs the same number of function calls.
Echo state networks (ESNs), belonging to the family of recurrent neural networks (RNNs), are suitable for addressing complex nonlinear tasks due to their rich dynamic characteristics and easy implementation. The reservoir of the ESN is composed of a large number of sparsely connected neurons with randomly generated weight matrices. How to set the structural parameters of the ESN becomes a difficult problem in practical applications. Traditionally, the design of the parameters of the ESN structure is performed manually. The manual adjustment of the ESN parameters is not convenient since it is an extremely challenging and time-consuming task. This paper proposes an ensemble of five particle swarm optimization (PSO) strategies to design the structure of ESN and then reduce the manual intervention in the design process. An adaptive selection mechanism is used for each particle in the evolution to select a strategy from the strategy candidate pool for evolution. In addition, leaky integration neurons are used as reservoir internal neurons, which are added within the adaptive mechanism for optimization. The root mean squared error (RMSE) is adopted as the evaluation criterion. The experimental results on Mackey-Glass time series benchmark dataset show that the proposed method outperforms other traditional evolutionary methods. Furthermore, experimental results on electrocardiogram dataset show that the proposed method on the ensemble of PSO displays an excellent performance on real-world problems.
An artificial compound eye system is the bionic system of natural compound eyes with much wider field-of-view, better capacity to detect moving objects and higher sensitivity to light intensity than ordinary single-aperture eyes. In recent years, renewed attention has been paid to the artificial compound eyes, due to their better characteristics inheriting from insect compound eyes than ordinary optical imaging systems. This paper provides a comprehensive survey of the state-of-the-art work on artificial compound eyes. This review starts from natural compound eyes to artificial compound eyes including their system design, theoretical development and applications. The survey of artificial compound eyes is developed in terms of two main types: planar and curved artificial compound eyes. Finally, the most promising future research developments are highlighted.
Deep convolutional neural networks (CNNs) are widely used for image classification. Deep CNNs often require a large memory and abundant computation resources, limiting their usability in embedded or mobile devices. To overcome this limitation, several pruning methods have been proposed. However, most of the existing methods focus on pruning parameters and cannot efficiently address the computation costs of deep CNNs. Additionally, these methods ignore the connections between the feature maps of different layers. This paper proposes a multi-objective pruning based on feature map selection (MOP-FMS). Unlike previous studies, we use the number of floating point operations (FLOPs) as a pruning objective in addition to the accuracy of the pruned network. First, we propose an encoding method based on feature map selection with a compact and efficient search space. Second, novel domain-specific crossover and mutation operators with reparation are designed to generate new individuals and make them meet the constraint rules. Then, decoding and pruning methods are proposed to prune networks based on the results of feature map selection. Finally, multi-objective optimisation is used for evaluation and individual selection. Our method has been tested with commonly used network structures. Numerical results demonstrate that the proposed method achieves better results than other state-of-the-art methods in terms of pruning rate without decreasing the accuracy rate to a high degree. •Considering the relation between the feature maps from different layers, the pruning problem is formulated as a bi-objective optimisation problem with feature map selection, and the accuracy rate and computation cost are simultaneously optimised.•A novel feature map-based encoding method and a unique decoding method are proposed for pruning common structures or networks with additive aggregation.•Special initialisation, crossover and mutation operators are designed with the quick reparation method to satisfy the encoding constraints of this specific problem.
In every day life, we always have to make decisions, e.g. the path to choose in order to go back home from work, the brand of milk in a supermarket, whether to watch football or a movie on TV, etc. Some of these choices appear to us obvious while some other choices require some thinking. Regardless of the context, decisions are usually made in order to reach a certain goal or satisfy a given necessity. For example, in the case of going back home from work, a reasonable goal would be to choose a path which leads us back home in the shortest possible time. Let us assume that the path should be performed by walking. In this case, the solution for the problem is likely to be the shortest path. This would be a simple optimization problem. If the goal would be to be at home at the earliest time after having bought something in the city center, e.g. a visit a shop, we have to exclude some of the possible paths. More specifically, we have to take into account only the paths which pass through the shop. The path having the latter features are said to be feasible while all the others are infeasible. The newly stated problem is a constrained optimization problem. If an additional goal, beside being back at home in the shortest possible time, is to take the opportunity for having some physical activities by means of a long walk, two conflicting objectives must be taken into account and a compromise must be accepted (e.g. a path that is not too long as to get home reasonably early but also not too short as to have at least some physical activity). Due to the presence of two simultaneous and conflicting goals, the latter is a multi-objective optimization problem.
Dropout is an effective method of mitigating over-fitting while training deep neural networks (DNNs). This method consists of switching off (dropping) some of the neurons of the DNN and training it by keeping the remaining neurons active. This approach makes the DNN general and resilient to changes in its inputs. However, the probability of a neuron belonging to a layer to be dropped, the 'dropout rate', is a hard-to-tune parameter that affects the performance of the trained model. Moreover, there is no reason, besides being more practical during parameter tuning, why the dropout rate should be the same for all neurons across a layer. This paper proposes a novel method to guide the dropout rate based on an evolutionary algorithm. In contrast to previous studies, we associate a dropout with each individual neuron of the network, thus allowing more flexibility in the training phase. The vector encoding the dropouts for the entire network is interpreted as the candidate solution of a bi-objective optimisation problem, where the first objective is the error reduction due to a set of dropout rates for a given data batch, while the second objective is the distance of the used dropout rates from a prearranged constant. The second objective is used to control the dropout rates and prevent them from becoming too small, hence ineffective; or too large, thereby dropping a too-large portion of the network. Experimental results show that the proposed method, namely GADropout, produces DNNs that consistently outperform DNNs designed by other dropout methods, some of them being modern advanced dropout methods representing the state-of-the-art. GADroput has been tested on multiple datasets and network architectures.
Effective implementations of Memetic Algorithms often integrate, within their design, problem-based pieces of information. When no information is known, an efficient MA can still be designed after a preliminary analysis of the problem. This approach is usually referred to as Fitness Landscape Analysis (FLA). This paper proposes a FLA technique to analyse the epistasis of continuous optimisation problems and estimate those directions, within a multi-dimensional space, associated with maximum and minimum directional derivatives. This estimation is achieved by making use of the covariance matrix associated with a distribution of points whose objective function value is below (in case of minimisation) a threshold. The eigenvectors and eigenvalues of the covariance matrix provide important pieces of information about the geometry of the problem and are then used to design a memetic operator that is a local search belonging to the family of generalised Pattern Search. A restarting mechanism enables a progressive characterisation of the fitness landscape. Numerical results show that the proposed approach successfully explore ill-conditioned basins of attractions and outperforms the standard pattern search as well as a pattern search recently proposed in the literature and partially based on a similar design logic. The proposed local search based on FLA also displays a performance competitive with that of other types of local search.
The present study is conducted in two phases. In the first phase we analyze the different aspects of gray image watermarking in a colored host. Robustness and imperceptibility are used as analysis parameters. The approaches explored and compared in this study are - watermark embedding with any one of the three RGB (Red-Green-Blue) components (single channel embedding), multichannel watermark embedding (same watermark with all channels) and multichannel embedding with equally segmented watermark. SVD (Singular Value Decomposition) is used to calculate the singular values of host image and then appropriate scaling factor isused to embed the watermark and the watermarked image is subjected to different attacks. To secure the watermark from an unauthorized access Arnold transform is implemented. From the simulation results it is observed that segmented watermark approach is better than the other two approaches in terms of both robustness and imperceptibility. In the second phase, change of robustness and imperceptibility is studied with the change of scaling factor for which PSO (Particle swarm optimization) is employed to determine the optimal values of scaling factor. The results here indicate that the use of different scaling factors (optimal) for each RGB component provides better result in comparison to a single (optimal) scaling factor in segmented multichannel approach. Overall, the experimental analysis shows that the equal distribution of gray watermark over RGB components with PSO optimized scaling factors provides significant improvement in the quality of watermarked image and the quality of retrieved watermark even from the distorted watermarked image.
This paper presents a signal processing technique for segmenting short speech utterances into unvoiced and voiced sections and identifying points where the spectrum becomes steady. The segmentation process is part of a system for deriving musculoskeletal articulation data from disordered utterances, in order to provide training feedback. The functioning of the signal processing technique has been optimized by selecting the parameters of the model. The optimization has been carried out by testing and comparing multiple Differential Evolution implementations, including a standard one, a memetic one, and a controlled randomized one. Numerical results have also been compared with a famous and efficient swarm intelligence algorithm. For the given problem, Differential Evolution schemes appear to display a very good performance as they can quickly reach a high quality solution. The binomial crossover appears, for the given problem, beneficial with respect to the exponential one. The controlled randomization appears to be the best choice in this case. The overall optimized system proved to segment well the speech utterances and efficiently detect its uninteresting parts.
Although Differential Evolution is an efficient and versatile optimizer, it has a wide margin of improvement. During the latest years much effort of computer scientists studying Differential Evolution has been oriented towards the improvement of the algorithmic paradigm by adding and modifying components. In particular, two modifications lead to important improvements to the original algorithmic performance. The first is the super-fit mechanism, that is the injection at the beginning of the optimization process of a solution previously improved by another algorithm. The second is the progressive reduction of the population size during the evolution of the population. Recently, the algorithmic paradigm of compact Differential Evolution has been introduced. This class of algorithm does not process a population of solutions but its probabilistic representation. In this way, the Differential Evolution can be employed on a device characterized by a limited memory, such as microcontroller or a Graphics Processing Unit. This paper proposes the implementation of the two modifications mentioned above in the context of compact optimization. The compact versions of memetic super-fit mechanism and population size reduction have been tested in this paper and their benefits highlighted. The main finding of this paper is that although separately these modifications do not robustly lead to significant performance improvements, the combined action of the two mechanism appears to be extremely efficient in compact optimization. The resulting algorithm succeeds at handling very diverse fitness landscapes and appears to improve on a regular basis the performance of a standard compact Differential Evolution.
Overhead contact lines (OCLs) are electric transmission lines that power railways, which are constantly threatened by external weather and environmental factors due to their outdoor location. Hence, for the long-term functioning of railway lines, a weather-driven risk predictor is an essential tool. Current prediction methods mainly adopt a single-point estimation system with fixed weights of neural networks and therefore cannot propagate the uncertainties within the data and model, resulting in unreliable predictions. To enhance safety-risk prevention capabilities, this paper proposes an uncertainty-aware trustworthy weather-driven failure-risk approach for OCLs, in a probabilistic deep multitask learning framework. Firstly, a deep Gaussian process is employed as the backbone model to deal with imbalanced weather-related failure datasets with limited fault samples. Moreover, a multi-task learning framework is embedded to simultaneously predict the multiple weather-driven failure risks under lightning, wind and haze. Finally, the predictive uncertainty is decomposed into epistemic and aleatory uncertainties, where epistemic and aleatory uncertainties account for the uncertainty within the model and data, respectively. Extensive experiments on actual OCLs are carried out to demonstrate the effectiveness of the proposed approach, which can effectively capture the predictive uncertainty and provide trustworthy predictive decisions of mitigating operational risk for railway operators.
This chapter proposes a neural network based approach for solving the resource discovery problem in Peer to Peer (P2P) networks and an Adaptive Global Local Memetic Algorithm (AGLMA) for performing in training of the neural network. The neural network, which is a multi-layer perceptron neural network, allows the P2P nodes to efficiently locate resources desired by the user. The necessity of testing the network in various working conditions, aiming to obtain a robust neural network, introduces noise in the objective function. The AGLMA is a memetic algorithm which employs two local search algorithms adaptively activated by an evolutionary framework. These local searchers, having different features according to the exploration logic and the pivot rule, have the role of exploring decision space from different and complementary perspectives. Furthermore, the AGLMA makes an adaptive noise compensation by means of explicit averaging on the fitness values and a dynamic population sizing which aims to follow the necessity of the optimization process. The numerical results demonstrate that the proposed computational intelligence approach leads to an efficient resource discovery strategy and that the AGLMA outperforms an algorithm classically employed for executing the neural network training.
We propose Multi-Strategy Coevolving Aging Particles (MS-CAP), a novel population-based algorithm for black-box optimization. In a memetic fashion, MS-CAP combines two components with complementary algorithm logics. In the first stage, each particle is perturbed independently along each dimension with a progressively shrinking (decaying) radius, and attracted towards the current best solution with an increasing force. In the second phase, the particles are mutated and recombined according to a multi-strategy approach in the fashion of the ensemble of mutation strategies in Differential Evolution. The proposed algorithm is tested, at different dimensionalities, on two complete black-box optimization benchmarks proposed at the Congress on Evolutionary Computation 2010 and 2013. To demonstrate the applicability of the approach, we also test MS-CAP to train a Feedforward Neural Network modeling the kinematics of an 8-link robot manipulator. The numerical results show that MS-CAP, for the setting considered in this study, tends to outperform the state-of-the-art optimization algorithms on a large set of problems, thus resulting in a robust and versatile optimizer.
This chapter proposes the integration of fitness diversity adaptation techniques within the parameter setting of Differential Evolution (DE). The scale factor and crossover rate are encoded within each genotype and self-adaptively updated during the evolution by means of a probabilistic criterion which takes into account the diversity properties of the entire population. The population size is also adaptively controlled by means of a novel technique based on a measurement of the fitness diversity. An extensive experimental setup has been implemented by including multivariate problems and hard to solve fitness landscapes. A comparison of the performance has been conducted by considering both standard DE and modern DE based algorithms, recently proposed in the literature. Available numerical results show that the proposed approach seems to be very promising for some fitness landscapes and still competitive with modern algorithms in other cases. In most cases analyzed the proposed self-adaptation is beneficial in terms of algorithmic performance and can be considered a useful tool for enhancing the performance of a DE scheme.
This paper proposes a novel Memetic Algorithm consisting of an Adaptive Evolutionary Algorithm (AEA) with three Intelligent Mutation Local Searchers (IMLSs) for designing optimal multidrug Structured Treatment Interruption (STI) therapies for Human Immunodeficiency Virus (HIV) infection. The AEA is an evolutionary algorithm with a dynamic parameter setting. The adaptive use of the local searchers helps the evolutionary process in the search of a global optimum. The adaptive rule is based on a phenotypical diversity measure of the population. The proposed algorithm has been tested for determining optimal 750-day pharmacological protocols for HIV patients. The pathogenesis of HIV is described by a system of differential equations including a model for an immune response. The multidrug therapies use reverse transcriptase inhibitor and protease inhibitor anti-HIV drugs. The medical protocol designed by the proposed algorithm leads to a strong immune response; the patient reaches a "healthy" state in one and half years and after this the STI medications can be discontinued. A comparison with a specific heuristic method and a standard Genetic Algorithm (GA) has been performed. Unlike the heuristic, the AEA with IMLSs does not impose any restrictions on the therapies in order to reduce the dimension of the problem. Unlike the GA, the AEA with IMLSs can overcome the problem of premature convergence to a suboptimal medical treatment. The results show that the therapies designed by the AEA lead to a "healthy" state faster than with the other methods. The statistical analysis confirms the computational effectiveness of the algorithm.
This article proposes a distributed differential evolution which employs a novel self-adaptive scheme, namely scale factor inheritance. In the proposed algorithm, the population is distributed over several sub-populations allocated according to a ring topology. Each sub-population is characterized by its own scale factor value. With a probabilistic criterion, that individual displaying the best performance is migrated to the neighbor population and replaces a pseudo-randomly selected individual of the target sub-population. The target sub-population inherits not only this individual but also the scale factor if it seems promising at the current stage of evolution. In addition, a perturbation mechanism enhances the exploration feature of the algorithm. The proposed algorithm has been run on a set of various test problems and then compared to two sequential differential evolution algorithms and three distributed differential evolution algorithms recently proposed in literature and representing state-of-the-art in the field. Numerical results show that the proposed approach seems very efficient for most of the analyzed problems, and outperforms all other algorithms considered in this study.
This paper proposes the application of Memetic Algorithms employing Differential Evolution as an evolutionary framework in order to achieve optimal design of the control system for a permanent-magnet synchronous motor. Two Memetic Differential Evolution frameworks have been considered in this paper and their performance has been compared to a standard Differential Evolution, a standard Genetic Algorithm and a Memetic Algorithm presented in literature for solving the same problem. All the algorithms have been tested on a simulation of the whole system (control system and plant) using a model obtained through identification tests. Numerical results show that the Memetic Differential Evolution frameworks seem to be very promising in terms of convergence speed and has fairly good performance in terms of final solution detected for the real-world problem under examination. In particular, it should be remarked that the employment of a meta-heuristic local search component during the early stages of the evolution seems to be very beneficial in terms of algorithmic efficiency.
This paper presents a signal processing technique for segmenting short speech utterances into unvoiced and voiced sections and identifying points where the spectrum becomes steady. The segmentation process is part of a system for deriving musculoskeletal articulation data from disordered utterances, in order to provide training feedback for people with speech articulation problem. The approach implement a novel and innovative segmentation scheme using artificial neural network mixture model (ANNMM) for identification and capturing of the various sections of the disordered (impaired) speech signals. This paper also identify some salient features that distinguish normal speech from impaired speech of the same utterances. This research aim at developing artificial speech therapist capable of providing reliable text and audiovisual feed back progress report to the patient.
This paper introduces two lightweight variants of ISPO, a Single Particle Optimization algorithm recently proposed in the literature. The goal of this work is to improve upon the performance of the original ISPO, still bearing in mind its admirable algorithmic simplicity. The first variant, namely ISPO-restart, combines in a memetic fashion the logics of ISPO with a partial restart mechanism similar to the binomial crossover typically used in Differential Evolution. The second variant, named VISPO, builds on top of the restart process a very simple learning stage which tries to adapt the algorithm behaviour to the (non)-separability of the problem. Numerical results obtained on three complete optimization benchmarks show that not only the two algorithms are able to improve, incrementally, upon the performance of ISPO, but also they show respectable performance in comparison with modern complex state-of-the-art methods, especially when the problem dimensionality increases.
This paper proposes the super-fit memetic differential evolution (SFMDE). This algorithm employs a differential evolution (DE) framework hybridized with three meta-heuristics, each having different roles and features. Particle Swarm Optimization assists the DE in the beginning of the optimization process by helping to generate a super-fit individual. The two other meta-heuristics are local searchers adaptively coordinated by means of an index measuring quality of the super-fit individual with respect to the rest of the population. The choice of the local searcher and its application is then executed by means of a probabilistic scheme which makes use of the generalized beta distribution. These two local searchers are the Nelder mead algorithm and the Rosenbrock Algorithm. The SFMDE has been tested on two engineering problems; the first application is the optimal control drive design for a direct current (DC) motor, the second is the design of a digital filter for image processing purposes. Numerical results show that the SFMDE is a flexible and promising approach which has a high performance standard in terms of both final solutions detected and convergence speed.
The emergence of different metaheuristics and their new variants in recent years has made the definition of the term Evolutionary Algorithms unclear. Originally, it was coined to put a group of stochastic search algorithms that mimic natural evolution together. While some people would still see it as a specific term devoted to this group of algorithms, including Genetic Algorithms, Genetic Programming, Evolution Strategies, Evolutionary Programming, and to a lesser extent Differential Evolution and Estimation of Distribution Algorithms, many others would regard "Evolutionary Algorithms" as a general term describing population-based search methods that involve some form of randomness and selection. In this chapter, we re-visit the fundamental question of "what is an Evolutionary Algorithm?" not only from the traditional viewpoint but also the wider, more modern perspectives relating it to other areas of Evolutionary Computation. To do so, apart from discussing the main characteristics of this family of algorithms we also look at Memetic Algorithms and the Swarm Intelligence algorithms. From our discussion, we see that establishing semantic borders between these algorithm families is not always easy, nor necessarily useful. It is anticipated that they will further converge as the research from these areas cross-fertilizes each other.
This paper studies the problem of the optimal control design of permanent magnet synchronous motor (PMSM) drives taking into account the noise due to sensors and measurement devices. The problem is analyzed by means of an experimental approach which considers noisy data returned by the real plant (on-line). In other words, each fitness evaluation does not come from a computer but from a real laboratory experiment. In order to perform the optimization notwithstanding presence of the noise, this paper proposes an Adaptive Prudent- Daring Evolutionary Algorithm (APDEA). The APDEA is an evolutionary algorithm with a dynamic parameter setting. Furthermore, the APDEA employs a dynamic penalty term and two cooperative-competitive survivor selection schemes. The numerical results show that the APDEA robustly executes optimization in the noisy environment. In addition, comparison with other meta-heuristics shows that behavior of the APDEA is very satisfactory in terms of convergence velocity. A statistical test confirms the effectiveness of the APDEA.
Differential evolution (DE) has become a prevalent tool for global optimization problems since it was proposed in 1995. As usual, when applying DE to a specific problem, determining the most proper strategy and its associated parameter values is time-consuming. Moreover, to achieve good performance, DE often requires different strategies combined with different parameter values at different evolution stages. Thus integrating several strategies in one algorithm and determining the application rate of each strategy as well as its associated parameter values online become an ad-hoc research topic. This paper proposes a novel DE algorithm, called multicriteria adaptive DE (MADE), for global numerical optimization. In MADE, a multicriteria adaptation scheme is introduced to determine the trial vector generation strategies and the control parameters of each strategy are separately adjusted according to their most recently successful values. In the multicriteria adaptation scheme, the impacts of an operator application are measured in terms of exploitation and exploration capabilities and correspondingly a multi-objective decision procedure is introduced to aggregate the impacts. Thirty-eight scale numerical optimization problems with various characteristics and two real-world problems are applied to test the proposed idea. Results show that MADE is superior or competitive to six well-known DE variants in terms of solution quality and convergence performance.
This paper compares three different fitness diversity adaptations in Multimeme Algorithms (MmAs). These diversity indexes have been integrated within a MmA present in literature, namely Fast Adaptive Memetic Algorithm. Numerical results show that it is not possible to establish a superiority of one of these adaptive schemes over the others and choice of a proper adaptation must be made by considering features of the problem under study. More specifically, one of these adaptations outperforms the others in the presence of plateaus or limited range of variability in fitness values, another adaptation is more proper for landscapes having distant and strong basins of attraction, the third one, in spite of its mediocre average performance can occasionally lead to excellent results.
The authors propose a hierarchical evolutionary algorithm (HEA) to solve structural optimization problems. The HEA is composed by a lower level evolutionary algorithm (LLEA) and a higher level evolutionary algorithm (HLEA). The HEA has been applied to the design of grounding grids for electrical safety. A compact representation to describe the topology of the grounding grid is proposed. An analysis of the decision space is carried out and its restriction is obtained according to some considerations on the physical meaning of the individuals. Due to the algorithmic structure and the specific class of problems under study, the fitness function of the HLEA is noisy. A statistical approach to analyze the behavior and the reliability of the fitness function is done by applying the limit theorems of the probability theory. The comparison with the other method of grounding grid design shows the validity and the efficiency of the HEA.
Purpose As well known, in the finite element method, the calculation and the location of the elements of the matrix C of the coefficients requires a lot of calculation times and memory employment especially for 3D problems. Besides, once the matrix C is properly filled, the solution of the system of linear equations is computationally expensive. Designmethodologyapproach The paper consists of two parts. In the first part, to quickly calculate and store only the nonnull terms of the matrix of the system, a geometrical analysis on threedimensional domains has been carried out. The second part of the paper deals with the solution of the system of linear equations and proposes a procedure for increasing the solution speed the traditional method of the conjugate gradient is hybridized with an adequate genetic algorithm Genetic Conjugate Gradient. Findings The proposed geometrical procedure allows us to calculate the nonnull terms and their location within the matrix C by simple recursive formulas. The results concerning the genetic conjugate gradient show that the convergence to the solution of the linear system is obtained in a much smaller number iterations and the calculation time is also significantly decreased. Originalityvalue The approach proposed to analyze the geometrical space has been turned out to be very useful in terms of memory saving and computational cost. The genetic conjugate gradient is an original hybrid method to solve large scale problems quicker than the traditional conjugate gradient. An application of the method has been shown for current fields generated by grounding electrodes.
—Generative adversarial networks (GANs) are machine learning algorithms that can efficiently generate data such as images. Although GANs are very popular, their training usually lacks stability, with the generator and discriminator networks failing to converge during the training process. To address this problem and improve the stability of GANs, in this paper, we automate the design of stable GANs architectures through a novel approach: differentiable architecture search with attention mechanisms for generative adversarial networks (DAMGAN). We construct a generator supernet and search for the optimal generator network within it. We propose incorporating two attention mechanisms between each pair of nodes in the supernet. The first attention mechanism, down attention, selects the optimal candidate operation of each edge in the supernet, while the second attention mechanism, up attention, improves the training stability of the supernet and limits the computational cost of the search by selecting the most important feature maps for the following candidate operations. Experimental results show that the architectures searched by our method obtain a state-of-the-art inception score (IS) of 8.99 and a very competitive Fréchet inception distance (FID) of 10.27 on the CIFAR-10 dataset. Competitive results were also obtained on the STL-10 dataset (IS = 10.35, FID = 22.18). Notably, our search time was only 0.09 GPU days. Index Terms—Generative adversarial networks, neural architecture search, attention mechanism, generative model.
This paper proposes a neural network based approach for solving the resource discovery problem in Peer to Peer (P2P) networks and an Adaptive Global Local Memetic Algorithm (AGLMA) for performing the training of the neural network. This training is very challenging due to the large number of weights and noise caused by the dynamic neural network testing. The AGLMA is a memetic algorithm consisting of an evolutionary framework which adaptively employs two local searchers having different exploration logic and pivot rules. Furthermore, the AGLMA makes an adaptive noise compensation by means of explicit averaging on the fitness values and a dynamic population sizing which aims to follow the necessity of the optimization process. The numerical results demonstrate that the proposed computational intelligence approach leads to an efficient resource discovery strategy and that the AGLMA outperforms two classical resource discovery strategies as well as a popular neural network training algorithm.
Exact methods for Agglomerative Hierarchical Clustering (AHC) with average linkage do not scale well when the number of items to be clustered is large. The best known algorithms are characterized by quadratic complexity. This is a generally accepted fact and cannot be improved without using specifics of certain metric spaces. Twister tries is an algorithm that produces a dendrogram (i.e, outcome of a hierarchical clustering) which resembles the one produced by AHC, while only needing linear space and time. However, twister tries are sensitive to rare, but still possible, hash evaluations. These might have a disastrous effect on the final outcome. We propose the use of a metaheuristic algorithm to overcome this sensitivity and show how approximate computations of dendrogram quality can help to evaluate the heuristic within reasonable time. The proposed metaheuristic is based on an evolutionary framework and integrates a surrogate model of the fitness within it to enhance the algorithmic performance in terms of computational time.
Memetic computing is a subject in computer science which considers complex structures as the combination of simple agents, memes, whose evolutionary interactions lead to intelligent structures capable of problem-solving. This paper focuses on memetic computing optimization algorithms and proposes a counter-tendency approach for algorithmic design. Research in the field tends to go in the direction of improving existing algorithms by combining different methods or through the formulation of more complicated structures. Contrary to this trend, we instead focus on simplicity, proposing a structurally simple algorithm with emphasis on processing only one solution at a time. The proposed algorithm, namely three stage optimal memetic exploration, is composed of three memes; the first stochastic and with a long search radius, the second stochastic and with a moderate search radius and the third deterministic and with a short search radius. The bottom-up combination of the three operators by means of a natural trial and error logic, generates a robust and efficient optimizer, capable of competing with modern complex and computationally expensive algorithms. This is suggestive of the fact that complexity in algorithmic structures can be unnecessary, if not detrimental, and that simple bottom-up approaches are likely to be competitive is here invoked as an extension to memetic computing basing on the philosophical concept of Ockham’s Razor. An extensive experimental setup on various test problems and one digital signal processing application is presented. Numerical results show that the proposed approach, despite its simplicity and low computational cost displays a very good performance on several problems, and is competitive with sophisticated algorithms representing the-state-of-the-art in computational intelligence optimization.
Compact algorithms are Estimation of Distribution Algorithms which mimic the behavior of population-based algorithms by means of a probabilistic representation of the population of candidate solutions. These algorithms have a similar behaviour with respect to population-based algorithms but require a much smaller memory. This feature is crucially important in some engineering applications, especially in robotics. A high performance compact algorithm is the compact Differential Evolution (cDE) algorithm. This paper proposes a novel implementation of cDE, namely compact Differential Evolution light (cDElight), to address not only the memory saving necessities but also real-time requirements. cDElight employs two novel algorithmic modifications for employing a smaller computational overhead without a performance loss, with respect to cDE. Numerical results, carried out on a broad set of test problems, show that cDElight, despite its minimal hardware requirements, does not deteriorate the performance of cDE and thus is competitive with other memory saving and population-based algorithms. An application in the field of mobile robotics highlights the usability and advantages of the proposed approach.
Sets out a method for determining the dangerous areas on the soil surface. The touch voltages are calculated by a Maxwell's subareas program. The search for the areas in which the touch voltages are dangerous is performed by a suitably modified genetic algorithm. The fitness is redefined so that the genetic algorithm does not lead directly to the only optimum solution, but to a certain number of solutions having pre-arranged "goodness" characteristics. The algorithm has been called "quasi-genetic" algorithm and has been successfully applied to various grounding systems.
Memetic computing is a subject in computer science which considers complex structures such as the combination of simple agents and memes, whose evolutionary interactions lead to intelligent complexes capable of problem-solving. The founding cornerstone of this subject has been the concept of memetic algorithms, that is a class of optimization algorithms whose structure is characterized by an evolutionary framework and a list of local search components. This article presents a broad literature review on this subject focused on optimization problems. Several classes of optimization problems, such as discrete, continuous, constrained, multi-objective and characterized by uncertainties, are addressed by indicating the memetic "recipes" proposed in the literature. In addition, this article focuses on implementation aspects and especially the coordination of memes which is the most important and characterizing aspect of a memetic structure. Finally, some considerations about future trends in the subject are given. (C) 2011 Elsevier B.V. All rights reserved.
This article proposes an Enhanced Memetic Differential Evolution (EMDE) for designing digital filters which aim at detecting defects of the paper produced during an industrial process. Defect detection is handled by means of two Gabor filters and their design is performed by the EMDE. The EMDE is a novel adaptive evolutionary algorithm which combines the powerful explorative features of Differential Evolution with the exploitative features of three local search algorithms employing different pivot rules and neighborhood generating functions. These local search algorithms are the Hooke Jeeves Algorithm, a Stochastic Local Search, and Simulated Annealing. The local search algorithms are adaptively coordinated by means of a control parameter that measures fitness distribution among individuals of the population and a novel probabilistic scheme. Numerical results confirm that Differential Evolution is an efficient evolutionary framework for the image processing problem under investigation and show that the EMDE performs well. As a matter of fact, the application of the EMDE leads to a design of an efficiently tailored filter. A comparison with various popular metaheuristics proves the effectiveness of the EMDE in terms of convergence speed, stagnation prevention, and capability in detecting solutions having high performance.
This paper compares the binomial crossover used in the Differential Evolution with a variant named the contiguous binomial crossover. In the latter, a contiguous block of variables is used for selecting which variables are exchanged, in a fashion similar to that of the exponential crossover, allowing to using a single, normally-distributed random number to decide the number of exchanged variables. Experimental results show that this variant of the binomial crossover exhibits in general similar or better performance than the original one, and allows to increase significantly the execution speed of the Differential Evolution, especially in higher dimension problems.
Automatic target detection and tracking systems are used extensively in complex scenes. In long-term tracking, some visual attributes of objects are changing, such as illumination, size, profile, and so on. To address the issue, it is particularly important to describe the essential properties of the objects in tracking. An enhanced kernelized correlation filter tracking strategy fused multiple features with location prediction is proposed. To make the object appearance models more accuracy and robustness, based on the original histogram of oriented gradient features, we integrate the hue, saturation, value, and grayscale information to construct a new descriptor to represent the target appearance. Moreover, location prediction and bi-linear interpolation are employed to obtain the more accurate target position. Experiments show that the proposed strategy can obtain superior or competitive performance in challenging benchmark data sets. In practice, the algorithm is applied to track shuttle bus targets in the airport apron.
Differential Evolution (DE) is a simple and efficient optimizer, especially for continuous optimization. For these reasons DE has often been employed for solving various engineering problems. On the other hand, the DE structure has some limitations in the search logic, since it contains too narrow a set of exploration moves. This fact has inspired many computer scientists to improve upon DE by proposing modifications to the original algorithm. This paper presents a survey on DE and its recent advances. A classification, into two macro-groups, of the DE modifications is proposed here: (1) algorithms which integrate additional components within the DE structure, (2) algorithms which employ a modified DE structure. For each macro-group, four algorithms representative of the state-of-the-art in DE, have been selected for an in depth description of their working principles. In order to compare their performance, these eight algorithm have been tested on a set of benchmark problems. Experiments have been repeated for a (relatively) low dimensional case and a (relatively) high dimensional case. The working principles, differences and similarities of these recently proposed DE-based algorithms have also been highlighted throughout the paper. Although within both macro-groups, it is unclear whether there is a superiority of one algorithm with respect to the others, some conclusions can be drawn. At first, in order to improve upon the DE performance a modification which includes some additional and alternative search moves integrating those contained in a standard DE is necessary. These extra moves should assist the DE framework in detecting new promising search directions to be used by DE. Thus, a limited employment of these alternative moves appears to be the best option in successfully assisting DE. The successful extra moves are obtained in two ways: an increase in the exploitative pressure and the introduction of some randomization. This randomization should not be excessive though, since it would jeopardize the search. A proper increase in the randomization is crucial for obtaining significant improvements in the DE functioning. Numerical results show that, among the algorithms considered in this study, the most efficient additional components in a DE framework appear to be the population size reduction and the scale factor local search. Regarding the modified DE structures, the global and local neighborhood search and self-adaptive control parameter scheme, recently proposed in literature, seem to be the most promising modifications.
Spiking neural P systems (SN P systems), inspired by biological neurons, are introduced as symbolical neural-like computing models that encode information with multisets of symbolized spikes in neurons and process information by using spike-based rewriting rules. Inspired by neuronal activities affected by enzymes, numerical variants of SN P systems called enzymatic numerical spiking neural P systems (ENSNP systems) are proposed wherein each neuron has a set of variables with real values and a set of enzymatic activation-production spiking rules, and each synapse has an assigned weight. By using spiking rules, ENSNP systems can directly implement mathematical methods based on real numbers and continuous functions. Furthermore, ENSNP systems are used to model ENSNP membrane controllers for robots implementing wall following. The trajectories, distances from the wall, and wheel speeds of robots with ENSNP membrane controllers for wall following are compared with those of a robot with a membrane controller for wall following. The average error values of the designed ENSNP membrane controllers are compared with three recently fuzzy logical controllers with optimization algorithms for wall following. The experimental results showed that the designed ENSNP membrane controllers can be candidates as efficient controllers to control robots implementing the task of wall following.
Multi-objective optimisation is a prominent subfield of optimisation with high relevance in real-world problems, such as engineering design. Over the past 2 decades, a multitude of heuristic algorithms for multi-objective optimisation have been introduced and some of them have become extremely popular. Some of the most promising and versatile algorithms have been implemented in software platforms. This article experimentally investigates the process of interpreting and implementing algorithms by examining multiple popular implementations of three well-known algorithms for multi-objective optimisation. We observed that official and broadly employed software platforms interpreted and thus implemented the same heuristic search algorithm differently. These different interpretations affect the algorithmic structure as well as the software implementation. Numerical results show that these differences cause statistically significant differences in performance.
An extremely natural, yet efficient design pattern in memetic computing optimisation is the sequential structure algorithms composed of few simple memes executed sequentially, each one with its own specific role, have proven to be robust and versatile on various optimisation problems with diverse features and dimensionality values. This principle of non-complexity, which can be seen as an application of the Ockham’s Razor in memetic computing, leads us to create shrinking three-stage optimal memetic exploration (S-3SOME), a scheme which progressively perturbs a candidate solution by alternating three search operators, the first one being a stochastic global search, the second a random sampling within progressive narrowing hyper-volume, and the third a deterministic local search. Numerical results show that the proposed S-3SOME, despite its simplicity, is competitive not only with other memory-saving schemes recently proposed in literature, but also with complex state-of-the-art population-based algorithms characterised by high computational overhead and memory employment.
Differential Evolution is a population based stochastic algorithm with less number of parameters to tune. However, the performance of DE is sensitive to the mutation and crossover strategies and their associated parameters. To obtain optimal performance, DE requires time consuming trial and error parameter tuning. To overcome the computationally expensive parameter tuning different adaptive/self-adaptive techniques have been proposed. Recently the idea of ensemble strategies in DE has been proposed and favorably compared with some of the state-of-the-art self-adaptive techniques. Compact Differential Evolution (cDE) is modified version of DE algorithm which can be effectively used to solve real world problems where sufficient computational resources are not available. cDE can be implemented on devices such as micro controllers or Graphics Processing Units (GPUs) which have limited memory. In this paper we introduced the idea of ensemble into cDE to improve its performance. The proposed algorithm is tested on the 30D version of 14 benchmark problems of Conference on Evolutionary Computation (CEC) 2005. The employment of ensemble strategies for the cDE algorithms appears to be beneficial and leads, for some problems, to competitive results with respect to the-state-of-the-art DE based algorithms
This article proposes a Memetic Differential Evolution (MDE) for designing digital filters which aim at detecting defects of the paper produced during an industrial process. The MDE is an adaptive evolutionary algorithm which combines the powerful explorative features of Differential Evolution (DE) with the exploitative features of two local searchers. The local searchers are adaptively activated by means of a novel control parameter which measures fitness diversity within the population. Numerical results show that the DE framework is efficient for the class of problems under study and employment of exploitative local searchers is helpful in supporting the DE explorative mechanism in avoiding stagnation and thus detecting solutions having a high performance.
Memetic Algorithms are traditionally composed of an evolutionary framework and one or more local search elements. However, modern generation Memetic Algorithms do not necessarily follow a pre-established scheme and are hybrid structures of various types. By following these modern trends, the present paper proposes an original and unconventional adaptive memetic structure generated by the hybridisation of a set of theoretical computational models, namely P Systems, and an evolutionary algorithm employing adaptation rules and moving operators inspired by Evolution Strategies. The resulting memetic algorithm, namely Adaptive Optimisation Spiking Neural P System (AOSNPS), is a tailored algorithm to solve optimisation problems with binary encoding.More specifically AOSNPS is composed of a family of parallel spiking neural P systems, each of them generating a binary vector representing a candidate solution on the basis of internal probability parameters and an adaptive Evolutionary Guider Algorithm that evolves the probabilities encoded in each P system. Numerical result shows that the proposed approach is effective to solve the 0/1 knapsack problem and outperforms various algorithms proposed in the literature to solve the same class of problems.
In the fashion of the Ockham's Razor principle for Memetic Computing approaches, this paper proposes an extremely simple and yet very efficient algorithm composed of two operators. The proposed approach employs a deterministic local search operator that periodically perturbs by means of a stochastic search component. The perturbation occurs by re-sampling the initial solution within the decision space. The deterministic local search is stopped by means of a precision based criterion and started over by means of the stochastic re-sampling. Although the concept of multi-start local search is not new in the optimization environment the proposed algorithm is shown to be extremely efficient on a broad set of diverse problems and competitive with complex algorithms representing the-state-of-the-art in computational intelligence optimization.
This paper proposes the use of two algorithms based on the parallel differential evolution. The first algorithm proposes the use of endemic control parameters within a parallel differential evolution algorithm; the differential evolution running at each subpopulation is associated with randomly initialised scale factor and crossover rate, which are then repeatedly updated during the optimisation process. The second algorithm proposes decomposing the search space of large-scale problems into lower-dimensionality subspaces, and associating each of these to one subpopulation of a parallel differential evolution algorithm. Each subpopulation is running a modified differential evolution algorithm, where the crossover function is limited to components of the subpopulation’s associated subspace. According to numerical results, both algorithms seem to be clear improvements over the original parallel distributed evolution; they are simple, robust, and efficient algorithms suited for various applications.
— Biped robots have received increasing attention due to their human-like mechanical structure and good environmental adaptability. In this paper, a new Human-Simulated Intelligent Walking Control (HIWC) scheme is proposed to solve the stability problem of the most popular proportional differential (PD) control under model inaccuracy and disturbance, and further improve its control performance. Specifically, based on Human-Simulated Intelligent Control (HSIC), HIWC is a hierarchical control structure composed of a foot placement compensation (FPC) strategy at the high-level planning layer, and a multi-mode compensation controller (MCC) at the low-level (execution) layer. In FPC, a foot placement compensation algorithm is proposed to plan and correct the swing foots trajectory in real time. MCC consists of a PD and two adaptive compensation algorithms. MCC under bounded uncertainty is proven to be stable in this paper using the Lyapunov theorem. HIWC was tested and compared with PD and model predictive control (MPC) in three experiments on a physical robot platform for planar walking, push-pull, and uneven-ground walking. Experimental results show that the proposed HIWC is more flexible and accurate in controlling the robot's movement. Note to Practitioners—This paper builds on the fact that PD controllers cannot be easily proven to be stable and do not provide accurate control for the biped robot walking problem. To address these issues, this paper proposes a novel control scheme namely Human-Simulated Intelligent Walking Control (HIWC) and belonging to the family of Human-Simulated Intelligent Control (HSIC) schemes. The proposed HIWC system has been compared against the model predictive control (MPC) and a proportional differential (PD) controller. The proposed HIWC, unlike PD controllers, is rigorously proven to be stable in the Manuscript presence of model inaccuracy and disturbance. Furthermore, the experiments carried out on a real-world biped robot demonstrate the superiority of HIWC over PD and MPC in terms of control performance.
—Neural architecture search (NAS) is a popular method that can automatically design deep neural network structures. However, designing a neural network using NAS is computationally expensive. This paper proposes a gradient-guided evolutionary neural architecture search (GENAS) to design convolutional neural networks for image classification. GENAS is a hybrid algorithm that combines evolutionary global and local search operators to evolve a population of subnets sampled from a supernet. Each candidate architecture is encoded as a table describing which operations are associated with the edges between nodes signifying feature maps. Besides, evolutionary optimisation employs novel crossover and mutation operators to manipulate the subnets using the proposed tabular encoding. Every n generations, the candidate architectures undergo a local search inspired by differentiable neural architecture search. GENAS is designed to overcome the limitations of both evolutionary and gradient-descent NAS. This algorithmic structure enables the performance assessment of the candidate architecture without retraining, thus limiting the NAS calculation time. Furthermore, subnet individuals are decoupled during evaluation to prevent strong coupling of operations in the supernet. The experimental results indicate that the searched structures achieve test errors of 2.45%, 16.86%, and 23.9% on CIFAR-10/100/ImageNet datasets and it costs only 0.26 GPU days on a graphic card. GENAS can effectively expedite the training and evaluation processes and obtain high-performance network structures.
Generative adversarial networks have made remarkable achievements in generative tasks. However, instability and mode collapse are still frequent problems. We improve the framework of evolutionary generative adversarial networks (E-GANs), calling it phased evolutionary generative adversarial networks (PEGANs), and adopt a self-attention module to improve upon the disadvantages of convolutional operations. During the training process, the discriminator will play against multiple generators simultaneously, where each generator adopts a different objective function as a mutation operation. Every time after the specified number of training iterations, the generator individuals will be evaluated and the best performing generator offspring will be retained for the next round of evolution. Based on this, the generator can continuously adjust the training strategy during training, and the self-attention module also enables the model to obtain the modeling ability of long-range dependencies. Experiments on two datasets showed that PEGANs improve the training stability and are competitive in generating high-quality samples.
In classification tasks, feature selection (FS) can reduce the data dimensionality and may also improve classification accuracy, both of which are commonly treated as the two objectives in FS problems. Many meta-heuristic algorithms have been applied to solve the FS problems and they perform satisfactorily when the problem is relatively simple. However, once the dimensionality of the datasets grows, their performance drops dramatically. This paper proposes a self-adaptive multi-objective genetic algorithm (SaMOGA) for FS, which is designed to maintain a high performance even when the dimensionality of the datasets grows. The main concept of SaMOGA lies in the dynamic selection of five different crossover operators in different evolution process by applying a self-adaptive mechanism. Meanwhile, a search stagnation detection mechanism is also proposed to prevent premature convergence. In the experiments, we compare SaMOGA with five multi-objective FS algorithms on sixteen datasets. According to the experimental results, SaMOGA yields a set of well converged and well distributed solutions on most data sets, indicating that SaMOGA can guarantee classification performance while removing many features, and the advantage over its counterparts is more obvious when the dimensionality of datasets grows.
Adam is an adaptive gradient descent approach that is commonly used in back-propagation (BP) algorithms for training feed-forward neural networks (FFNNs). However, it has the defect that it may easily fall into local optima. To solve this problem, some metaheuristic approaches have been proposed to train FFNNs. While these approaches have stronger global search capabilities enabling them to more readily escape from local optima, their convergence performance is not as good as that of Adam. The proposed algorithm is an ensemble of differential evolution and Adam (EDEAdam), which integrates a modern version of the differential evolution algorithm with Adam, using two different sub-algorithms to evolve two sub-populations in parallel and thereby achieving good results in both global and local search. Compared with traditional algorithms, the integration of the two algorithms endows EDEAdam with powerful capabilities to handle different classification problems. Experimental results prove that EDEAdam not only exhibits improved global and local search capabilities, but also achieves a fast convergence speed.
This paper proposes the employment of the differential evolution (DE) to offline optimize the covariance matrices of a new reduced delayed-state Kalman-filter (DSKF)-based algorithm which estimates the stator-flux linkage components, in the stationary reference frame, to realize sensorless control of induction motors (IMs). The DSKF-based algorithm uses the derivatives of the stator-flux components as mathematical model and the stator-voltage equations as observation model so that only a vector of four variables has to be offline optimized. Numerical results, carried out using a low-speed training test, show that the proposed DE-based approach is very promising and clearly outperforms a classical local search and three popular metaheuristics in terms of quality of the final solution for the problem considered in this paper. A novel simple stator-flux-oriented sliding-mode (SFO-SM) control scheme is online used in conjunction with the optimized DSKF-based algorithm to improve the robustness of the sensorless IM drive at low speed. The SFO-SM control scheme has closed loops of torque and stator-flux linkage without proportional-plus-integral controllers so that a minimum number of gains has to be tuned.
This paper proposes a Differential Evolution based algorithm for numerical optimization in the presence of noise. The proposed algorithm, namely Noise Analysis Differential Evolution (NADE), employs a randomized scale factor in order to overcome the structural difficulties of a Differential Evolution in a noisy environment as well as a noise analysis component which determines the amount of samples required for characterizing the stochastic process and thus efficiently performing pairwise comparisons between parent and offspring solutions. The NADE has been compared, for a benchmark set composed of various fitness landscapes tinder several levels of noise bandwidth, with a classical evolutionary algorithm for noisy optimization and two recently proposed metaheuristics. Numerical results show that the proposed NADE has a very good performance in detecting high quality solutions despite the presence of noise. The NADE seems, in most cases, very fast and reliable in detecting promising search directions and continuing evolution towards the optimum.
The file attached to this record is the authors final peer reviewed version. The publisher's final version can be found by following the DOI link. Three Stage Optimal Memetic Exploration (3SOME) is a single-solution optimization algorithm where the coordinated action of three distinct operators progressively perturb the solution in order to progress towards the problem's optimum. In the fashion of Memetic Computing, 3SOME is designed as an organized structure where the three operators interact by means of a success/failure logic. This simple sequential structure is an initial example of Memetic Computing approach generated by means of a bottom-up logic. This paper compares the 3SOME structure with a popular adaptive technique for Memetic Algorithms, namely Meta-Lamarckian learning. The resulting algorithm, Meta-Lamarckian Three Stage Optimal Memetic Exploration (ML3SOME) is thus composed of the same three 3SOME operators but makes use a different coordination logic. Numerical results show that the adaptive technique is overall efficient also in this Memetic Computing context. However, while ML3SOME appears to be clearly better than 3SOME for low dimensionality values, its performance appears to suffer from the curse of dimensionality more than that of the original 3SOME structure.
The ensemble structure is a computational intelligence supervised strategy consisting of a pool of multiple operators that compete among each other for being selected, and an adaptation mechanism that tends to reward the most successful operators. In this paper we extend the idea of the ensemble to multiple local search logics. In a memetic fashion, the search structure of an ensemble framework cooperatively/ competitively optimizes the problem jointly with a pool of diverse local search algorithms. In this way, the algorithm progressively adapts to a given problem and selects those search logics that appear to be the most appropriate to quickly detect high quality solutions. The resulting algorithm, namely Ensemble of Parameters and Strategies Differential Evolution empowered by Local Search (EPSDE-LS), is evaluated on multiple testbeds and dimensionality values. Numerical results show that the proposed EPSDE-LS robustly displays a very good performance in comparison with some of the state-of-the-art algorithms.
Memetic Computing (MC) structures are algorithms composed of heterogeneous operators (memes) for solving optimization problems. In order to address these problems, this study investigates and proposes a simple yet extremely efficient structure, namely Parallel Memetic Structure (PMS). PMS is a single solution optimization algorithm composed of tree operators, the first one being a stochastic global search which explores the entire decision space searching for promising regions. In analogy with electrical networks, downstream of the global search component there is a parallel of two alternative elements, i.e. two local search algorithms with different features in terms of search logic, whose purpose is to refine the search in the regions detected by the upstream element. The first local search explores the space along the axes, while the second performs diagonal movements in the direction of the estimated gradient. The PMS algorithm, despite its simplicity, displays a respectable performance compared to that of popular meta-heuristics and modern optimization algorithms representing the state-of-the-art in the field. Thanks to its simple structure, PMS appears to be a very flexible algorithm for various problem features and dimensionality values. Unlike modern complex algorithm that are specialized for some benchmarks and some dimensionality values, PMS achieves solutions with a high quality in various and diverse contexts, for example both on low dimensional and large scale problems. An application example in the field of magnetic sensors further proves the potentials of the proposed approach. This study confirms the validity of the Ockham's Razor in MC: efficiently designed simple structures can perform as well as (if not better than) complex algorithms composed of many parts. (C) 2012 Elsevier Inc. All rights reserved.
Lightning is a rapidly evolving phenomenon, exhibiting both mesoscale and microscale characteristics. Its prediction significantly relies on timely and accurate data observation. With the implementation of new generation weather radar systems and lightning detection networks, radar reflectivity image products, and lightning observation data are becoming increasingly abundant. Research focus has shifted towards lightning nowcasting (prediction of imminent events), utilizing deep learning (DL) methods to extract lightning features from very large data sets. In this paper, we propose a novel spatio-temporal fusion deep learning lightning nowcasting network (STF-LightNet) for lightning nowcasting. The network is based on a 3-dimensional U-Net architecture with encoder-decoder blocks and adopts a structure of multiple branches as well as the main path for the encoder block. To address the challenges of feature extraction and fusion of multi-source data, multiple branches are used to extract different data features independently, and the main path fuses these features. Additionally, a spatial attention (SA) module is added to each branch and the main path to automatically identify lightning areas and enhance their features. The main path fusion is conducted in two steps: the first step fuses features from the branches, and the second fuses features from the previous and current levels of the main path using two different methods—the weighted summation fusion method and the attention gate fusion method. To overcome the sparsity of lightning observations, we employ an inverse frequency weighted cross-entropy loss function. Finally, STF-LightNet is trained using observations from the previous half hour to predict lightning in the next hour. The outcomes illustrate that the fusion of both the multi-branch and main path structures enhances the network's ability to effectively integrate features from diverse data sources. Attention mechanisms and fusion modules allow the network to capture more detailed features in the images.
Fitness landscape analysis for optimisation is a technique that involves analysing black-box optimisation problems to extract pieces of information about the problem, which can beneficially inform the design of the optimiser. Thus, the design of the algorithm aims to address the specific features detected during the analysis of the problem. Similarly, the designer aims to understand the behaviour of the algorithm, even though the problem is unknown and the optimisation is performed via a metaheuristic method. Thus, the algorithmic design made using fitness landscape analysis can be seen as an example of explainable AI in the optimisation domain. The present paper proposes a framework that performs fitness landscape analysis and designs a Pattern Search (PS) algorithm on the basis of the results of the analysis. The algorithm is implemented in a restarting fashion: at each restart, the fitness landscape analysis refines the analysis of the problem and updates the pattern matrix used by PS. A computationally efficient implementation is also presented in this study. Numerical results show that the proposed framework clearly outperforms standard PS and another PS implementation based on fitness landscape analysis. Furthermore, the two instances of the proposed framework considered in this study are competitive with popular algorithms present in the literature.
This paper proposes a novel trajectory tracking control approach for nonholonomic wheeled mobile robots. In this approach, the integration of feed-forward and feedback controls is presented to design the kinematic controller of wheeled mobile robots, where the control law is constructed on the basis of Lyapunov stability theory, for generating the precisely desired velocity as the input of the dynamic model of wheeled mobile robots; a proportional-integral-derivative based membrane controller is introduced to design the dynamic controller of wheeled mobile robots to make the actual velocity follow the desired velocity command. The proposed approach is defined by using an enzymatic numerical membrane system to integrate two proportional-integral-derivative controllers, where neural networks and experts' knowledge are applied to tune parameters. Extensive experiments conducted on the simulated wheeled mobile robots show the effectiveness of this approach.
This paper proposes the employment of continuous probability distributions instead of step functions for adaptive coordination of the local search in fitness diversity based Memetic Algorithms. Two probability distributions are considered in this study: the beta and exponential distributions. These probability distributions have been tested within two memetic frameworks present in literature. Numerical results show that employment of the probability distributions can be beneficial and improve performance of the original Memetic Algorithms on a set of test functions without varying the balance between the evolutionary and local search components.
This paper proposes a novel algorithm for large-scale optimization problems. The proposed algorithm, namely shuffle or update parallel differential evolution (SOUPDE) is a structured population algorithm characterized by sub-populations employing a Differential evolution logic. The sub-populations quickly exploit some areas of the decision space, thus drastically and quickly reducing the fitness value in the highly multi-variate fitness landscape. New search logics are introduced into the sub-population functioning in order to avoid a diversity loss and thus premature convergence. Two simple mechanisms have been integrated in order to pursue this aim. The first, namely shuffling, consists of randomly rearranging the individuals over the sub-populations. The second consists of updating all the scale factors of the sub-populations. The proposed algorithm has been run on a set of various test problems for five levels of dimensionality and then compared with three popular meta-heuristics. Rigorous statistical and scalability analyses are reported in this article. Numerical results show that the proposed approach significantly outperforms the meta-heuristics considered in the benchmark and has a good performance despite the high dimensionality of the problems. The proposed algorithm balances well between exploitation and exploration and succeeds to have a good performance over the various dimensionality values and test problems present in the benchmark. It succeeds at outperforming the reference algorithms considered in this study. In addition, the scalability analysis proves that with respect to a standard Differential Evolution, the proposed SOUPDE algorithm enhances its performance while the dimensionality grows.
This paper presents the optimization of a vector controlled Permanent Magnet Synchronous Motor (PMSM). The optimization is carried out by a Surrogate Assisted Hooke-Jeeves Algorithm (SAHJA). The SAHJA is a local searcher having a steepest descent pivot rule which employs both the real fitness and an approximated model (surrogate), in a cooperative way, in order to perform the optimization saving calculation time. The real fitness of each set of control parameters is evaluated by means of a simulated test which takes a few seconds to be executed. In a combined way, a computationally cheap approximated function, generated by means of the least square method, is employed. The numerical results show that the usage of the SAHJA leads to a significant reduction in terms of computational cost with respect to the classical Hooke-Jeeves algorithm, still maintaining high performance in terms of reliability.
Spiking neural P systems (abbreviated as SNP systems) are models of computation that mimic the behavior of biological neurons. The spiking neural P systems with communication on request (abbreviated as SNQP systems) are a recently developed class of SNP system, where a neuron actively requests spikes from the neighboring neurons instead of passively receiving spikes. It is already known that small SNQP systems, with four unbounded neurons, can achieve Turing universality. In this context, ‘unbounded’ means that the number of spikes in a neuron is not capped. This work investigates the dependency of the number of unbounded neurons on the computation capability of SNQP systems. Specifically, we prove that (1) SNQP systems composed entirely of bounded neurons can characterize the family of finite sets of numbers; (2) SNQP systems containing two unbounded neurons are capable of generating the family of semilinear sets of numbers; (3) SNQP systems containing three unbounded neurons are capable of generating nonsemilinear sets of numbers. Moreover, it is obtained in a constructive way that SNQP systems with two unbounded neurons compute the operations of Boolean logic gates, i.e., OR, AND, NOT, and XOR gates. These theoretical findings demonstrate that the number of unbounded neurons is a key parameter that influences the computation capability of SNQP systems.
In this paper, we propose a novel Reinforcement Learning (RL) algorithm for robotic motion control, that is, a constrained Deep Deterministic Policy Gradient (DDPG) deviation learning strategy to assist biped robots in walking safely and accurately. The previous research on this topic highlighted the limitations in the controller's ability to accurately track foot placement on discrete terrains and the lack of consideration for safety concerns. In this study, we address these challenges by focusing on ensuring the overall system's safety. To begin with, we tackle the inverse kinematics problem by introducing constraints to the damping least squares method. This enhancement not only addresses singularity issues but also guarantees safe ranges for joint angles, thus ensuring the stability and reliability of the system. Based on this, we propose the adoption of the constrained DDPG method to correct controller deviations. In constrained DDPG, we incorporate a constraint layer into the Actor network, incorporating joint deviations as state inputs. By conducting offline training within the range of safe angles, it serves as a deviation corrector. Lastly, we validate the effectiveness of our proposed approach by conducting dynamic simulations using the CRANE biped robot. Through comprehensive assessments, including singularity analysis, constraint effectiveness evaluation, and walking experiments on discrete terrains, we demonstrate the superiority and practicality of our approach in enhancing walking performance while ensuring safety. Overall, our research contributes to the advancement of biped robot locomotion by addressing gait optimisation from multiple perspectives, including singularity handling, safety constraints, and deviation learning.
This paper proposes a period representation for modeling the multidrug HIV therapies and an Adaptive Multimeme Algorithm (AMmA) for designing the optimal therapy. The period representation offers benefits in terms of flexibility and reduction in dimensionality compared to the binary representation. The AMmA is a memetic algorithm which employs a list of three local searchers adaptively activated by an evolutionary framework. These local searchers, having different features according to the exploration logic and the pivot rule, have the role of exploring the decision space from different and complementary perspectives and, thus, assisting the standard evolutionary operators in the optimization process. Furthermore, the AMmA makes use of an adaptation which dynamically sets the algorithmic parameters in order to prevent stagnation and premature convergence. The numerical results demonstrate that the application of the proposed algorithm leads to very efficient medication schedules which quickly stimulate a strong immune response to HIV. The earlier termination of the medication schedule leads to lesser unpleasant side effects for the patient due to strong antiretroviral therapy. A numerical comparison shows that the AMmA is more efficient than three popular metaheuristics. Finally, a statistical test based on the calculation of the tolerance interval confirms the superiority of the AMmA compared to the other methods for the problem under study.
Neural architecture search (NAS), a promising method for automated neural architecture design, is often hampered by its overwhelming computational burden, especially the architecture evaluation process in evolutionary neural architecture search (ENAS). Although there are surrogate models based on regression or ranking to assist or replace the neural architecture evaluation process in ENAS to reduce the computational cost, these surrogate models are still affected by poor architectures and are not able to accurately find good architectures in a search space. To solve the above problems, we propose a novel surrogate-assisted NAS approach, which called similarity surrogate-assisted evolutionary neural architecture search with dual encoding strategy (SSENAS). We propose a surrogate model based on similarity measurement to select excellent neural architectures from a large number of candidate architectures in a search space. Furthermore, we propose a dual encoding strategy for architecture generation and surrogate evaluation in ENAS to achieve better exploration of well-performing neural architectures in a search space and sufficiently informative representations of neural architec-tures, respectively. We perform experiments on NAS benchmarks to verify the effectiveness of the proposed algorithm. The experimental results show that SSENAS can accurately find the best neural architecture in the NAS-Bench-201 search space after only 400 queries of the tabular benchmark. In the NAS-Bench-101 search space, it can also get results comparable to other algorithms. In addition, we conduct a large number of experiments and analyses on the proposed algorithm, showing that the surrogate model measured by similarity can gradually search for excellent neural architectures in a search space.
The design of complex and deep neural networks is often performed by identifying and combining building blocks and progressively selecting the most promising combination. Neuroevolution automates this process by employing evolutionary algorithms to guide the search. Within this field, grammar-based evolutionary algorithms have been demonstrated to be powerful tools to describe and thus encode complex neural architectures effectively. Following this trend, the present work proposes a novel grammar-based multi-objective neuroevolutionary for generating Fully Convolutional Networks. The proposed method, named Multi-Objective gRammatical Evolution for FUlly convolutional Networks (MOREFUN), includes a new efficient way to encode skip connections, facilitating the description of complex search spaces and the injection of domain knowledge in the search procedure, generation of fully convolutional networks, upsampling of lower-resolution inputs in multi-input layers, usage of multi-objective fitness, and inclusion of data augmentation and optimiser settings to the grammar. Our best networks outperformed previous grammar evolution algorithms, achieving 90.5% accuracy on CIFAR-10 without using transfer learning, ensembles, or test-time data augmentation. Our best models had 13.39±5.25 trainable parameters and the evolutionary process required 90 min per generation.
Despite the constant growth of the computational power in consumer electronics, very simple hardware is still used in space applications. In order to obtain the highest possible reliability, in space systems limited-power but fully tested and certified hardware is used, thus reducing fault risks. Some space applications require the solution of an optimization problem, often plagued by real-time and memory constraints. In this paper, the disturbance to the base of a robotic arm mounted on a spacecraft is modeled, and it is used as a cost function for an online trajectory optimization process. In order to tackle this problem in a computationally efficient manner, addressing not only the memory saving necessities but also real-time requirements, we propose a novel compact algorithm, namely compact Differential Evolution light (cDElight). cDElight belongs to the class of Estimation of Distribution Algorithms (EDAs), which mimic the behavior of population-based algorithms by means of a probabilistic model of the population of candidate solutions. This model has a more limited memory footprint than the actual population. Compared to a selected set of memory-saving algorithms, cDElight is able to obtain the best results, despite a lower computational overhead.
Within memetic computing frameworks, the structure as well as a correct choice of memes are important elements that drive successful optimization algorithms. This paper studies variations of a promising yet simple search operator, the S Algorithm, which can easily be integrated within a memetic framework to improve candidate solutions. S is a single-solution optimizer that iteratively perturbs variables and conditionally evaluates solutions along the axes. The first S variant, namely S2, unconditionally evaluates solutions in both directions while S3 maintains D uncorrelated step sizes that are either expanded in the direction of improving fitness or else redirected and contracted. Numerical results from the CEC2010 and CEC2014 benchmarks show that the variants outperform S in terms of the number of function evaluations for a given fitness value and, further, that S3 outperforms S in terms of final fitness against a wide range of problems and dimensionality.
This paper proposes a novel implementation of micro-Differential Evolution (μDE) that incorporates within the DE scheme an extra search move that attempts to improve the best solution by perturbing it along the axes. These extra moves complement the DE search logic and allows the exploration of the decision space from an alternative perspective. In addition, these extra moves at subsequent activations tend to explore a progressively narrowing area. This mechanism increases the exploitation of the original scheme thus helping μDE to prevent from stagnation. An experimental set-up including various test problems and dimensionality values has been considered. Numerical results show that the proposed algorithm enhances upon the original μDE performance and, despite its simplicity, is competitive with modern complex DE based algorithms.
Real-world problems often involve the optimisation of multiple conflicting objectives. These problems, referred to as multi-objective optimisation problems, are especially challenging when more than three objectives are considered simultaneously. This paper proposes an algorithm to address this class of problems. The proposed algorithm is an evolutionary algorithm based on an evolution strategy framework, and more specifically, on the Covariance Matrix Adaptation Pareto Archived Evolution Strategy (CMA-PAES). A novel selection mechanism is introduced and integrated within the framework. This selection mechanism makes use of an adaptive grid to perform a local approximation of the hypervolume indicator which is then used as a selection criterion. The proposed implementation, named Covariance Matrix Adaptation Pareto Archived Evolution Strategy with Hypervolumesorted Adaptive Grid Algorithm (CMA-PAES-HAGA), overcomes the limitation of CMA-PAES in handling more than two objectives and displays a remarkably good performance on a scalable test suite in five, seven, and ten-objective problems. The performance of CMA-PAES-HAGA has been compared with that of a competition winning meta-heuristic, representing the state-of-the-art in this sub-field of multi-objective optimisation. The proposed algorithm has been tested in a seven-objective real-world application, i.e. the design of an aircraft lateral control system. In this optimisation problem, CMA-PAES-HAGA greatly outperformed its competitors.
Spiking neural P systems are a class of third generation neural networks belonging to the framework of membrane computing. Spiking neural P systems with communication on request (SNQ P systems) are a type of spiking neural P system where the spikes are requested from neighboring neurons. SNQ P systems have previously been proved to be universal (computationally equivalent to Turing machines) when two types of spikes are considered. This paper studies a simplified version of SNQ P systems, i.e. SNQ P systems with one type of spike. It is proved that one type of spike is enough to guarantee the Turing universality of SNQ P systems. Theoretical results are shown in the cases of the SNQ P system used in both generating and accepting modes. Furthermore, the influence of the number of unbounded neurons (the number of spikes in a neuron is not bounded) on the computation power of SNQ P systems with one type of spike is investigated. It is found that SNQ P systems functioning as number generating devices with one type of spike and four unbounded neurons are Turing universal.
This paper proposes the compact differential evolution (cDE) algorithm. cDE, like other compact evolutionary algorithms, does not process a population of solutions but its statistic description which evolves similarly to all the evolutionary algorithms. In addition, cDE employs the mutation and crossover typical of differential evolution (DE) thus reproducing its search logic. Unlike other compact evolutionary algorithms, in cDE, the survivor selection scheme of DE can be straightforwardly encoded. One important feature of the proposed cDE algorithm is the capability of efficiently performing an optimization process despite a limited memory requirement. This fact makes the cDE algorithm suitable for hardware contexts characterized by small computational power such as micro-controllers and commercial robots. In addition, due to its nature cDE uses an implicit randomization of the offspring generation which corrects and improves the DE search logic. An extensive numerical setup has been implemented in order to prove the viability of cDE and test its performance with respect to other modern compact evolutionary algorithms and state-of-the-art population-based DE algorithms. Test results show that cDE outperforms on a regular basis its corresponding population-based DE variant. Experiments have been repeated for four different mutation schemes. In addition cDE outperforms other modern compact algorithms and displays a competitive performance with respect to state-of-the-art population-based algorithms employing a DE logic. Finally, the cDE is applied to a challenging experimental case study regarding the on-line training of a nonlinear neural-network-based controller for a precise positioning system subject to changes of payload. The main peculiarity of this control application is that the control software is not implemented into a computer connected to the control system but directly on the micro-controller. Both numerical results on the test functions and experimental results on the real-world problem are very promising and allow us to think that cDE and future developments can be an efficient option for optimization in hardware environments characterized by limited memory.
Compact algorithms are optimization algorithms belonging to the class of Estimation of Distribution Algorithms (EDAs). Compact algorithms employ the search logic of population-based algorithms but do not store and process an entire population and all the individuals therein, but on the contrary make use of a probabilistic representation of the population in order to perform the optimization process. This probabilistic representation simulates the population behaviour as it extensively explores the decision space at the beginning of the optimization process and progressively focuses the search on the most promising genotypes and narrows the search radius. In this way, a much smaller amount of parameters must be stored in the memory. Thus, a run of these algorithms requires much more limited memory devices compared to their corresponding standard population-based algorithms. This class of algorithms is especially useful for those applications characterized by a limited hardware, e.g. mobile systems, industrial robots, etc. This chapter illustrates the history of compact optimization by giving a description of the main paradigms proposed in literature and a novel interpretation of the subject as well as a design procedure. An application to space robotics is given in order to show the applicability of compact algorithms.
Pattern search is a family of single solution deterministic optimisation algorithms for numerical optimisation. Pattern search algorithms generate a new candidate solution by means of an archive of potential moves, named pattern. This pattern is generated by a basis of vectors that span the domain where the function to optimise is defined. The present article proposes an adaptive implementation of pattern search that performs, at run-time, a fitness landscape analysis of the problem to determine the pattern and adapt it to the geometry of the problem. The proposed algorithm, called Adaptive Covariance Pattern Search (ACPS) uses at the beginning the fundamental orthonormal basis (directions of the variables) to build the pattern. Subsequently, ACPS saves the successful visited solutions, calculates the covariance matrix associated with these samples, and then uses the eigenvectors of this covariance matrix to build the pattern. ACPS is a restarting algorithm that at each restart recalculates the pattern that progressively adapts to the problem to optimise. Numerical results show that the proposed ACPS appears to be a promising approach on various problems and dimensions.
The present study, proposes an optimization algorithm for solving the continuous global optimization problems. The basic framework selected for modeling the algorithm is Artificial Bee Colony (ABC). The proposed variant is called ABC with changing factor or CF-ABC. The proposed CF-ABC tries to maintain a tradeoff between exploration and exploitation so as to obtain reasonably good results. The proposed algorithm is implemented on the six benchmark functions and four engineering design problems. Simulated results illustrate the efficiency of the CF-ABC in terms of convergence speed and mean value.
This paper presents a neural system-based technique for segmenting short impaired speech utterances into silent, unvoiced, and voiced sections. Moreover, the proposed technique identifies those points of the (voiced) speech where the spectrum becomes steady. The resulting technique thus aims at detecting that limited section of the speech which contains the information about the potential impairment of the speech. This section is of interest to the speech therapist as it corresponds to the possibly incorrect movements of speech organs (lower lip and tongue with respect to the vocal tract). Two segmentation models to detect and identify the various sections of the disordered (impaired) speech signals have been developed and compared. The first makes use of a combination of four artificial neural networks. The second is based on a support vector machine (SVM). The SVM has been trained by means of an ad hoc nested algorithm whose outer layer is a metaheuristic while the inner layer is a convex optimization algorithm. Several metaheuristics have been tested and compared leading to the conclusion that some variants of the compact differential evolution (CDE) algorithm appears to be well-suited to address this problem. Numerical results show that the SVM model with a radial basis function is capable of effective detection of the portion of speech that is of interest to a therapist. The best performance has been achieved when the system is trained by the nested algorithm whose outer layer is hybrid-population-based/CDE. A population-based approach displays the best performance for the isolation of silence/noise sections, and the detection of unvoiced sections. On the other hand, a compact approach appears to be clearly well-suited to detect the beginning of the steady state of the voiced signal. Both the proposed segmentation models display outperformed two modern segmentation techniques based on Gaussian mixture model and deep learning.
This paper proposes a metaheuristic approach to solve a complex large scale optimization problem that originates from a recently introduced Positron Emission Tomography (PET) data analysis method that provides an estimate of tissue heterogeneity. More specifically three modern metaheuristics have been tested. These metaheustics are based on Differential Evolution, Particle Swarm Optimization, and Memetic Computing. On the basis of a preliminary analysis of the fitness landscape, an intelligent initialization technique has been proposed in this paper. More specifically, since the fitness landscape appears to have a strong basin of attraction containing a multimodal landscape, a local search method is applied to one solution at the beginning of the optimization process and inserted into a randomly generated population. The resulting "doped" population is then processed by the metaheuristics. Numerical results show that the application of the local search at the beginning of the optimization process leads to significant benefits in terms of algorithmic performance. Among the metaheuristics analyzed in this study, the DE based algorithm appears to display the best performance.
The Authors suggest a new method for quickly determining the maximum touch voltage generated by a grounding system leaking a known current. The touch voltages in the points of the soil surface are calculated by the Maxwell’s subareas method and the search for the maximum touch voltage is carried out by an ad hoc genetic program which is based both on the “one-point crossover” technique and on a mutation having a pre-arranged probability rate. The method has been successfully tested on some grounding systems.
Fitness Landscape Analysis (FLA) for loss/gain functions for Machine Learning is an emerging research trend in Computational Intelligence that offered an alternative view on how learning algorithms work and should be designed. The vast majority of these recent studies investigate supervised learning whereas reinforcement learning remains so far nearly unaddressed. This paper performs a FLA on the reinforcement learning of a deep neural network for a simulated robot control task and focuses on ruggedness and neutrality. Two configurations of the physical system under investigation are considered and studied separately to highlight differences and similarities. Furthermore, the results of the performed FLA are put into relation with performance of the learning algorithm with the aim of achieving an understanding of most suitable parameter setting. Numerical results indicate a correlation between ruggedness and exploration to enable more optimal reinforcement learning. In the presence of high ruggedness the algorithm displays its best performance when the control parameters are set to enable a high degree of exploration. Conversely, when the landscape appears less rugged a less exploratory behaviour seems to contribute to the best performance of the learning algorithm.
This paper proposes a computational prototype for automatic design of optimization algorithms. The proposed scheme makes an analysis of the problem that estimates the degree of separability of the optimization problem. The separability is estimated by computing the Pearson correlation indices between pairs of variables. These indices are then manipulated to generate a unique index that estimates the separability of the entire problem. The separability analysis is thus used to design the optimization algorithm that addresses the needs of the problem. This prototype makes use of two operators arranged in a Parallel Memetic Structure. The first operator performs moves along the axes while the second simultaneously perturbs all the variables to follow the gradient of the fitness landscape. The resulting algorithmic implementation, namely Separability Prototype for Automatic Memes (SPAM), has been tested on multiple testbeds and various dimensionality levels. The proposed computational prototype proved to be a flexible and intelligent framework capable to learn from a problem and, thanks to this learning, to outperform modern meta-heuristics representing the-state-of-the-art in optimization. (C) 2014 Elsevier Inc. All rights reserved.
This paper proposes the Noise Analysis compact Genetic Algorithm (NAcGA). This algorithm integrates a noise analysis component within a compact structure. This fact makes the proposed algorithm appealing for those real-world applications characterized by the necessity of a high performance optimizer despite severe hardware limitations. The noise analysis component adaptively assigns the amount of fitness evaluations to be performed in order to distinguish two candidate solutions. In this way, it is assured that computational resources are not wasted and the selection of the most promising solution is correctly performed. The noise analysis employed in this algorithm spouses very well the pair-wise comparison logic typical of compact evolutionary algorithms. Numerical results show that the proposed algorithm significantly improves upon the performance, in noisy environments, of the standard compact genetic algorithm. Two implementation variants based on the elitist strategy have been tested in this studies. It is shown that the nonpersistent strategy is more robust to the noise than the persistent one and therefore its implementation seems to be advisable in noisy environments.
This paper proposes the employment of multiple scale factor values within distributed differential evolution structures. Four different scale factor schemes are proposed, tested, compared and analyzed. Two schemes simply employ multiple scale factor values and two also include an update logic during the evolution. The four schemes have been integrated for comparison within three recently proposed distributed differential evolution structures and tested on several various test problems. Numerical results show that, on average, the employment of multiple scale factors is beneficial since in most cases it leads to significant improvements in performance with respect to standard distributed algorithms. Although proper choice of a scale factor scheme appears to be dependent on the distributed structure, any of the proposed simple schemes has proven to significantly improve upon the single scale factor distributed differential evolution algorithms. (C) 2011 Elsevier Inc. All rights reserved.
This chapter proposes a novel adaptive memetic approach for solving multi-objective optimization problems. The proposed approach introduces the novel concept of crossdominance and employs this concept within a novel probabilistic scheme which makes use of the Wigner distribution for performing coordination of the local search. Thus, two local searchers are integrated within an evolutionary framework which resorts to an evolutionary algorithm previously proposed in literature for solving multi-objective problems. These two local searchers are a multi-objective version of simulated annealing and a novel multi-objective implementation of the Rosenbrock algorithm. Numerical results show that the proposed algorithm is rather promising and, for several test problems, outperforms two popular meta-heuristics present in literature. A realworld application in the field of electrical engineering, the design of a control system of an electric motor, is also shown. The application of the proposed algorithm leads to a solution which allows successful control of a direct current motor by simultaneously handling the conflicting objectives of the dynamic response.
The file attached to this record is the authors final peer reviewed version. The publisher's final version can be accessed via the DOI link. Memetic Computing (MC) is a discipline which studies optimization algorithms and sees them as structures of operators, the memes. Although the choice of memes is crucial for an effective algorithmic design, special attention should be paid also to the coordination amongst the memes. This paper presents a study on a basic sequential structure, namely Three Stage Optimal Memetic Exploration (3SOME). The 3SOME algorithm is composed of three operators (or memes) which progressively perturbs a single solution. The first meme, long distance exploration is characterized by a long search radius and is supposed to detect promising areas of the decision space. The second meme, middle distance exploration, is characterized by a moderate search radius and is supposed to focus the search in the the most promising basins of attraction. The third meme, short distance exploration, is characterized by a short search radius and had the role of performing the local optimal search in the areas detected by the first two memes. To assess the importance of the structure within MC we compare the performance of 3SOME with two modified versions of it over two complete benchmarks. In both cases, while retaining the 3SOME structure, we replace one of the three original components (short distance exploration) with an alternative deterministic local search, respectively Rosenbrock and Powell methods. Numerical results show that, regardless of the choice of the specific memes, as far as the 3SOME structure contains memes which perform long, middle, and short distance explorations a similar performance is achieved. These results remark that besides the intuitive finding that a proper choice of operators is fundamental for the algorithmic success, the structure composing them also plays a crucial role.
In Evolutionary Computing, Swarm Intelligence, and more generally, populationbased algorithms diversity plays a crucial role in the success of the optimization. Diversity is a property of a group of individuals which indicates how much these individuals are alike. Clearly, a group composed of individuals similar to each other is said to have a low diversity whilst a group of individuals dissimilar to each other is said to have a high diversity. In computer science, in the context of population-based algorithms the concept of diversity is more specific: the diversity of a population is a measure of the number of different solutions present, see [239].
In video-based point cloud compression (V-PCC), one geometry video and one color video are generated from a dynamic point cloud. Then, the two videos are compressed independently using a state-of-the-art video coder. In the Moving Picture Experts Group (MPEG) V-PCC test model, the quantization parameters for a given group of frames are constrained according to a fixed offset rule. For example, for the low-delay configuration, the difference between the quantization parameters of the first frame and the quantization parameters of the following frames in the same group is zero by default. We show that the rate-distortion performance of the V-PCC test model can be improved by lifting this constraint and considering the ratedistortion optimization problem as a multi-variable constrained combinatorial optimization problem where the variables are the quantization parameters of all frames. To solve the optimization problem, we use a variant of the differential evolution algorithm. Experimental results for the low-delay configuration show that our method can achieve a Bjøntegaard delta bitrate of up to -43.04% and more accurate rate control (average bitrate error to the target bitrate of 0.45% vs. 10.75%) compared to the state-of- the-art method, which optimizes the rate-distortion performance subject to the test model default offset rule. We also show that our optimization strategy can be used to improve the rate-distortion performance of two-dimensional video coders.
This paper proposes a novel adaptive local search algorithm for tackling real-valued (or continuous) dynamic optimization problems. The proposed algorithm is a simple single-solution based metaheuristic that perturbs the variables separately to select the search direction for the following step and adapts its step size to the gradient. The search directions that appear to be the most promising are rewarded by a step size increase while the unsuccessful moves attempt to reverse the search direction with a reduced step size. When the environment is subject to changes, a new solution is sampled and crosses over the best solution in the previous environment. Furthermore, the algorithm makes use of a small archive where the best solutions are saved. Experimental results show that the proposed algorithm, despite its simplicity, is competitive with complex population-based algorithms for tested dynamic optimization problems.
This paper proposes the scale factor local search differential evolution (SFLSDE). The SFLSDE is a differential evolution (DE) based memetic algorithm which employs, within a self-adaptive scheme, two local search algorithms. These local search algorithms aim at detecting a value of the scale factor corresponding to an offspring with a high performance, while the generation is executed. The local search algorithms thus assist in the global search and generate offspring with high performance which are subsequently supposed to promote the generation of enhanced solutions within the evolutionary framework. Despite its simplicity, the proposed algorithm seems to have very good performance on various test problems. Numerical results are shown in order to justify the use of a double local search instead of a single search. In addition, the SFLSDE has been compared with a standard DE and three other modern DE based metaheuristic for a large and varied set of test problems. Numerical results are given for relatively low and high dimensional cases. A statistical analysis of the optimization results has been included in order to compare the results in terms of final solution detected and convergence speed. The efficiency of the proposed algorithm seems to be very high especially for large scale problems and complex fitness landscapes.
This paper proposes a novel algorithm for solving continuous complex optimization problems with a relatively low memory consumption. The proposed approach, namely Composed compact Differential Evolution, consists of a set of compact Differential Evolution units which simultaneously search the decision space from various perspectives. A randomization in the virtual population allows the algorithm to behave, on one hand, as a multiple local search with a multi-start logic integrated within it. On the other hand, the compact units communicate among each other by means of a ring topology and propagation of information. More specifically, the most promising elite solutions and scale factor values of each compact unit are migrated to the neighbour unit so that the search of the global optimum is performed. In other words, while each single compact unit performs a local search by exploiting the direction suggested by each elite solution, the entire structure combines the achievement of each local search operation towards the direction of the global search. The proposed algorithm is characterized by a limited memory consumption and is memory-wise equivalent to a population-based algorithm with a small population. Numerical results show that the proposed approach outperforms other compact algorithms and various modern population-based structures.
In recent years, much attention has been paid to the electronic cluster eye (eCley), a new type of artificial compound eyes, because of its small size, wide field of view (FOV) and sensitivity to motion objects. An eCley is composed of a certain number of optical channels organized as an array. Each optical channel spans a small and fixed field of view (FOV). To obtain a complete image with a full FOV, the images from all the optical channels are required to be fused together. The parallax from unparallel neighboring optical channels in eCley may lead to reconstructed image blurring and incorrectly estimated depth. To solve this problem, this paper proposes a geometry based three-dimensional image processing method (G3D) for eCley to obtain a complete focused image and dense depth map. In G3D, we derive the geometry relationship of optical channels in eCley to obtain the mathematical relation between the parallax and depth among unparallel neighboring optical channels. Based on the geometry relationship, all of the optical channels are used to estimate the depth map and reconstruct a focused image. Subsequently, by using an edge-aware interpolation method, we can further gain a sharply focused image and a depth map. The effectiveness of the proposed method is verified by the experimental results.
This paper proposes to use a differential evolution (DE) algorithm to obtain the optimal covariance matrices of a new reduced delayed-state Kalman filter (DSKF) based algorithm to realize sensorless induction motor drives. We propose to use a novel simple stator flux oriented-sliding mode (SFOSM) control scheme in conjunction with the optimized DSKF-based algorithm, estimating the stator flux linkage components. This control scheme has closed loops of torque and stator flux without PI-type controllers and a minimum number of controller gains is required to obtain good performance without fine tuning. The DSKF-based algorithm estimates the stator flux components in the stationary reference frame, using the derivatives of the stator flux components as mathematical model and the stator voltage equations as observation model. The experiments show a comparison between DE and genetic algorithms (GAs) with different settings. The DE outperformed the best known GAs on proposed optimization problem. The paper concentrates on a low-speed training test and the experiments show the low-speed performance of the sensorless control scheme using the new optimized DSKF-based algorithm.
Differential evolution (DE) is a prominent stochastic optimization technique for global optimization. After its original definition in 1995, DE frameworks have been widely researched by computer scientists and practitioners. It is acknowledged that structuring a population is an efficient way to enhance the algorithmic performance of the original, single population (panmictic) DE. However, only a limited amount of work focused on Distributed DE (DDE) due to the difficulty of designing an appropriate migration strategy. Since a proper migration strategy has a major impact on the performance, there is a large margin of improvement for the DDE performance. In this paper, an enhanced DDE algorithm is proposed for global numerical optimization. The proposed algorithm, namely DDE with Multicultural Migration (DDEM) makes use of two migration selection approaches to maintain a high diversity in the subpopulations, Target Individual Based Migration Selection (TIBMS) and Representative Individual Based Migration Selection (RIBMS), respectively. In addition, the diversity amongst the individuals is controlled by means of the proposed Affinity Based Replacement Strategy (ABRS) mechanism. Numerical experiments have been performed on 34 diverse test problems. The comparisons have been made against DDE algorithms using classical migration strategies and three popular DDE variants. Experimental results show that DDEM displays a better or equal performance with respect to its competitors in terms of the quality of solutions, convergence, and statistical tests.
Ensemble of parameters and mutation strategies differential evolution (EPSDE) is an elegant, promising optimization framework recently introduced in the literature. The idea behind it is that a pool of mutation and crossover strategies, along with associated pools of parameters, can flexibly adapt to a large variety of problems when a simple success based rule is introduced. Modern versions of this scheme attempts to improve upon the original performance at the cost of a high complexity. One of most successful implementations of this algorithmic scheme is the Self-adaptive Ensemble of Parameters and Strategies Differential Evolution (SaEPSDE). This paper operates on the SaEPSDE, reducing its complexity by identifying some algorithmic components that we experimentally show as possibly unnecessary. The result of this de-constructing operation is a novel algorithm implementation, here referred to as "j" Ensemble of Strategies Differential Evolution (jESDE). The proposed implementation is drastically simpler than SaEPSDE as several parts of it have been removed or simplified. Nonetheless, jESDE appears to display a competitive performance, on diverse problems throughout various dimensionality values, with respect to the original EPSDE algorithm, as well as to SaEPSDE and three modern algorithms based on Differential Evolution.
Some real-world optimization problems are plagued by a limited hardware availability. This situation can occur, for example, when the optimization must be performed on a device whose hardware is limited due to cost and space limitations. This paper addresses this class of optimization problems and proposes a novel algorithm, namely compact Particle Swarm Optimization (cPS0). The proposed algorithm employs the search logic typical of Particle Swarm Optimization (PSO) algorithms, but unlike classical PSO algorithms, does not use a swarm of particles and does not store neither the positions nor the velocities. On the contrary, cPSO employs a probabilistic representation of the swarm's behaviour. This representation allows a modest memory usage for the entire algorithmic functioning, the amount of memory used is the same as what is needed for storing five solutions. A novel interpretation of compact optimization is also given in this paper. Numerical results show that cPSO appears to outperform other modern algorithms of the same category (i.e. which attempt to solve the optimization despite a modest memory usage). In addition, cPSO displays a very good performance with respect to its population-based version and a respectable performance also with respect to some more complex population-based algorithms. A real world application in the field of power engineering and energy generation is given. The presented case study shows how, on a model of an actual power plant, an advanced control system can be online and real-time optimized. In this application example the calculations are embedded directly on the real-time control system. (C) 2013 Elsevier Inc. All rights reserved.
This paper proposes an algorithm to solve the CEC2013 benchmark. The algorithm, namely Super-fit Multicriteria Adaptive Differential Evolution (SMADE), is a Memetic Computing approach based on the hybridization of two algorithmic schemes according to a super-fit memetic logic. More specifically, the Covariance Matrix Adaptive Evolution Strategy (CMAES), run at the beginning of the optimization process, is used to generate a solution with a high quality. This solution is then injected into the population of a modified Differential Evolution, namely Multicriteria Adaptive Differential Evolution (MADE). The improved solution is super-fit as it supposedly exhibits a performance a way higher than the other population individuals. The super-fit individual then leads the search of the MADE scheme towards the optimum. Unimodal or mildly multimodal problems, even when non-separable and ill-conditioned, tend to be solved during the early stages of the optimization by the CMAES. Highly multi-modal optimization problems are efficiently tackled by SMADE since the MADE algorithm (as well as other Differential Evolution schemes) appears to work very well when the search is led by a super-fit individual.
Purpose This paper aims to propose a reliable local search algorithm having steepest descent pivot rule for computationally expensive optimization problems. In particular, an application to the design of Permanent Magnet Synchronous Motor PMSM drives is shown. Designmethodologyapproach A surrogate assisted HookeJeeves algorithm SAHJA is proposed. The SAHJA is a local search algorithm with the structure of the HookeJeeves algorithm, which employs a local surrogate model dynamically constructed during the exploratory move at each step of the optimization process. Findings Several numerical experiments have been designed. These experiments are carried out both on the simulation model offline and at the actual plant online. Moreover, the offline experiments have been considered in nonnoisy and noisy cases. The numerical results show that use of the SAHJA leads to a saving in terms of computational cost without requiring any extra hardware components. Originalityvalue The surrogate approach in the design of electric drives is novel. In addition, implementation of the proposed surrogate model allows the algorithm not only to reduce computational cost but also to filter noise caused by the sensors and measurement devices.
One of the main challenges in algorithmics in general, and in Memetic Computing, in particular, is the automatic design of search algorithms. A recent advance in this direction (in terms of continuous problems) is the development of a software prototype that builds up an algorithm based upon a problem analysis of its separability. This prototype has been called the Separability Prototype for Automatic Memes (SPAM). This article modifies the SPAM by incorporating within it an adaptive model used in hyper-heuristics for tackling optimization problems. This model, namely Adaptive Operator Selection (AOS), rewards at run time the most promising heuristics/memes so that they are more likely to be used in the following stages of the search process. The resulting framework, here referred to as SPAM-AOS, has been tested on various benchmark problems and compared with modern algorithms representing the-state-of-the-art of search for continuous problems. Numerical results show that the proposed SPAM-AOS is a promising framework that outperforms the original SPAM and other modern algorithms. Most importantly, this study shows how certain areas of Memetic Computing and Hyper-heuristics are very closely related topics and it also shows that their combination can lead to the development of powerful algorithmic frameworks.
This paper proposes a novel adaptation scheme for Differential Evolution (DE) frameworks. The proposed algorithm, namely Estimation Distribution Differential Evolution (EDDE), is based on a DE structure and employs randomized scale factor ad crossover rate values. These values are sampled from truncated Gaussian probability distribution functions. These probability functions adaptively vary during the optimization process. At the beginning of the optimization the truncated Gaussian functions are characterized by a large standard deviation values and thus are similar to uniform distributions. During the later stages of the evolution, the probability functions progressively adapt to the most promising values attempting to detect the optimal working conditions of the algorithm. The performance offered by the proposed algorithm has been compared with those given by three modern DE based algorithms which represent the state-of-the-art in DE. Numerical results show that the proposed EDDE, despite its simplicity, is competitive with the other algorithms and in many cases displays a very good performance in terms of both final solution detected and convergence speed.
This paper proposes an image processing/machine learning system for estimating the amount of biomass in a field. This piece of information is precious in agriculture as it would allow a controlled adjustment of water and fertilizer. This system consists of a flying robot device which captures multiple images of the area under interest. Subsequently, this set of images is processed by means of a combined action of digital elevation models and multispectral images in order to reconstruct a three-dimensional model of the area. This model is then processed by a machine learning device, i.e. a support vector regressor with multiple kernel functions, for estimating the biomass present in the area. The training of the system has been performed by means of three modern meta-heuristics representing the state-of-the-art in computational intelligence optimization. These three algorithms are based on differential evolution, particle swarm optimization, and evolution strategy frameworks, respectively. Numerical Results derived by empirical simulations show that the proposed approach can be of a great support in precision agriculture. In addition, the most promising results have been attained by means of an algorithm based on the differential evolution framework.
This chapter proposes a novel algorithm for handling high dimensionality in large scale problems. The proposed algorithm, here indicated with Differential Evolution for Large Scale problems (DELS) is a Differential Evolution (DE) based Memetic Algorithm with self-adaptive control parameters and automatic population size reduction, which employs within its framework a variation operator local search. The local search algorithm is applied to the scale factor in order to generate high quality solutions. Due to its structure, the computational cost of the local search is independent on the number of dimensions characterizing the problem and thus is a suitable component for large scale problems. The proposed algorithm has been compared with a standard DE and two other modern DE based metaheuristics for a varied set of test problems. Numerical results show that the DELS is an efficient and robust algorithm for highly multivariate optimization, and the employment of the local search to the scale factor is beneficial in order to detect solutions with a high quality, convergence speed and algorithmic robustness.
This paper proposes the integration of the generalized opposition based learning into compact Differential Evolution frameworks and tests its impact on the algorithmic performance. Opposition-based learning is a technique which has been applied, in several circumstances, to enhance the performance of Differential Evolution. It consists of the generation of additional points by means of a hyper-rectangle. These opposition points are simply generated by making use of a central symmetry within the hyper-rectangle. In the population based Differential Evolution, the inclusion of this search move corrects a limitation of the original algorithm, i.e. the scarcity of search moves, and sometimes leads to benefits in terms of algorithmic performance. The opposition-based learning scheme is further improved in the generalized scheme by integrating some randomness and progressive narrowing of the search. The proposed study shows how the generalized opposition-based learning can be encoded within a compact Differential Evolution framework and displays its effect on a set of diverse problems. Numerical results show that the generalized opposition-based learning is beneficial for compact Differential Evolution employing the binomial crossover while its implementation is not always successful when the exponential crossover is used. In particular, the opposition-based logic appears to be in general promising for non-separable problems whilst it seems detrimental for separable problems.
Membrane systems (also called P systems) refer to the computing models abstracted from the structure and the functioning of the living cell as well as from the cooperation of cells in tissues, organs, and other populations of cells. Spiking neural P systems (SNPS) are a class of distributed and parallel computing models that incorporate the idea of spiking neurons into P systems. To attain the solution of optimization problems, P systems are used to properly organize evolutionary operators of heuristic approaches, which are named as membrane-inspired evolutionary algorithms (MIEAs). This paper proposes a novel way to design a P system for directly obtaining the approximate solutions of combinatorial optimization problems without the aid of evolutionary operators like in the case of MIEAs. To this aim, an extended spiking neural P system (ESNPS) has been proposed by introducing the probabilistic selection of evolution rules and multi-neurons output and a family of ESNPS, called optimization spiking neural P system (OSNPS), are further designed through introducing a guider to adaptively adjust rule probabilities to approximately solve combinatorial optimization problems. Extensive experiments on knapsack problems have been reported to experimentally prove the viability and effectiveness of the proposed neural system.
This paper aims to study the benefits and limitations in the hybridization of the Differential Evolution with local search algorithms. In order to perform this study, the performance of three Memetic Algorithms employing a Differential Evolution as an evolutionary framework and several local search algorithms adaptively coordinated by means of a fitness diversity logic have been analyzed. The performance of a standard Differential Evolution whose parameter setting has been executed only after fine tuning has also been taken into account in the comparison. The comparative analysis has been performed on a set of various test functions. Numerical results show that the Memetic Algorithms without any extensive parameter tuning are still competitive with the finely tuned plain Differential Evolution.
Solutions to real-world problems often require the simultaneous optimisation of multiple conflicting objectives. In the presence of four or more objectives, the problem is referred to as a "many-objective optimisation problem". A problem of this category introduces many challenges, one of which is the effective and efficient selection of optimal solutions. The hypervolume indicator (or s-metric), i.e. the size of dominated objective space, is an effective selection criterion for many-objective optimisation. The indicator is used to measure the quality of a non-dominated set, and can be used to sort solutions for selection as part of the contributing hypervolume indicator. However, hypervolume based selection methods can have a very high, if not infeasible, computational cost. The present study proposes a novel hypervolume driven selection mechanism for many-objective problems, whilst maintaining a feasible computational cost. This approach, named the Hypervolume Adaptive Grid Algorithm (HAGA), uses two-phases (narrow and broad) to prevent population-wide calculation of the contributing hypervolume indicator. Instead, HAGA only calculates the contributing hypervolume indicator for grid populations, i.e. for a few solutions, which are close in proximity (in the objective space) to a candidate solution when in competition for survival. The result is a trade-off between complete accuracy in selecting the fittest individuals in regards to hypervolume quality, and a feasible computational time in many-objective space. The real-world efficiency of the proposed selection mechanism is demonstrated within the optimisation of a classifier for concealed weapon detection.
This paper proposes the introduction of a generator of random individuals within the ring topology of a Parallel Differential Evolution algorithm. The generated random individuals are then injected within a sub-population. A crucial point in the proposed study is that a proper balance between migration and random injection can determine the success of a distributed Differential Evolution scheme. An experimental study of this balance is carried out in this paper. Numerical results show that the proposed Parallel Random Injection Differential Evolution seems to be a simple, robust, and efficient algorithm which can be used for various applications. An important finding of this paper is that premature convergence problems due to an excessively frequent migration can be overcome by the injection of random individuals. In this way, the resulting algorithm maintains the high convergence speed properties of a parallel algorithm with high migration but differs in that it is able to continue improving upon the available genotypes and detect high quality solutions.
Purpose - This paper aims to design an algorithm able to locate all the possible dangerous areas generated by the leaking of a fault current in a grounding system (i.e. the areas where the limits of the technical standards are not respected) and thus locate, inside each area, the point which takes locally the maximum value of touch voltage.Design methodology approach - A fast evolutionary-deterministic algorithm to solve constrained multimodal optimization problems is proposed. The algorithm is composed by three algorithmic blocks: a Quasi Genetic Algorithm to find a population of feasible solutions, a Fitness Sharing Selection to choose a subpopulation of feasible and fitter solutions having high diversity, a Hooke-Jeeves Algorithm to find all the global and local feasible maxima.Findings - The proposed algorithm has been successfully applied to various current field (i.e. to many shapes of grounding grids) problems to find the dangerous values of touch voltages generated by various grounding systems having any shape and it has turned out to be fast and reliable.Originality value - For this kind of problems, in fact, there is a lack, in literature, of multimodal optimization methods under safety constraints and the application of classical methods (e.g. genetic algorithms or deterministic methods) would be often inadequate since these methods are made so as to converge towards a single maximum point and so they unavoidably lose the information related to all the other possible maxima. On the contrary, a good application of the proposed allows the overcoming of these limits.
When dealing with real-world applications, one often faces non-linear and nondifferentiable optimization problems which do not allow the employment of exact methods. In addition, as highlighted in [104], popular local search methods (e.g. Hooke-Jeeves, Nelder Mead and Rosenbrock) can be ill-suited when the real-world problem is characterized by a complex and highly multi-modal fitness landscape since they tend to converge to local optima. In these situations, population based meta-heuristics can be a reasonable choice, since they have a good potential in detecting high quality solutions. For these reasons, meta-heuristics, such as Genetic Algorithms (GAs), Evolution Strategy (ES), Particle Swarm Optimization (PSO), Ant Colony Optimization (ACO), and Differential Evolution (DE), have been extensively applied in engineering and design problems.
This paper presents an experimental study on the efficacy of a rotation-invariant Differential Evolution (based on current-to-rand mutation) on a benchmark of test problems in its non-rotated and rotated version. Numerical results show that standard Differential Evolution outperforms rotation-invariant Differential Evolution on the benchmark under consideration for both non-rotated and rotated problems. In other words, the rotation-invariant Differential Evolution does not seem to be more efficient than its standard counterpart to address rotated problems. According to our interpretation, these experimental results show that rotated problems are simply different problems with respect to the non-rotated problems. Furthermore, rotation-invariant Differential Evolution is characterised by its moving operator: it generates an offspring by perturbing all the design variables of a candidate solution at the same time. This logic does not appear to guarantee a better performance on rotated problems.
Biological brains have a natural capacity for resolving certain classification tasks. Studies on biologically plausible spiking neurons, architectures and mechanisms of artificial neural systems that closely match biological observations while giving high classification performance are gaining momentum. Spiking neural P systems (SN P systems) are a class of membrane computing models and third-generation neural networks that are based on the behavior of biological neural cells and have been used in various engineering applications. Furthermore, SN P systems are characterized by a highly flexible structure that enables the design of a machine learning algorithm by mimicking the structure and behavior of biological cells without the over-simplification present in neural networks. Based on this aspect, this paper proposes a novel type of SN P system, namely, layered SN P system (LSN P system), to solve classification problems by supervised learning. The proposed LSN P system consists of a multi-layer network containing multiple weighted fuzzy SN P systems with adaptive weight adjustment rules. The proposed system employs specific ascending dimension techniques and a selection method of output neurons for classification problems. The experimental results obtained using benchmark datasets from the UCI machine learning repository and MNIST dataset demonstrated the feasibility and effectiveness of the proposed LSN P system. More importantly, the proposed LSN P system presents the first SN P system that demonstrates sufficient performance for use in addressing real-world classification problems.
Membrane computing models are parallel and distributed natural computing models. These models are often referred to as P systems. This paper proposes a novel multi-behaviors co-ordination controller model using enzymatic numerical P systems for autonomous mobile robots navigation in unknown environments. An environment classifier is constructed to identify different environment patterns in the maze-like environment and the multi-behavior co-ordination controller is constructed to coordinate the behaviors of the robots in different environments. Eleven sensory prototypes of local environments are presented to design the environment classifier, which needs to memorize only rough information, for solving the problems of poor obstacle clearance and sensor noise. A switching control strategy and multi-behaviors coordinator are developed without detailed environmental knowledge and heavy computation burden, for avoiding the local minimum traps or oscillation problems and adapt to the unknown environments. Also, a serial behaviors control law is constructed on the basis of Lyapunov stability theory aiming at the specialized environment, for realizing stable navigation and avoiding actuator saturation. Moreover, both environment classifier and multi-behavior coordination controller are amenable to the addition of new environment models or new behaviors due to the modularity of the hierarchical architecture of P systems. The simulation of wheeled mobile robots shows the effectiveness of this approach.
A Generative Adversarial Network (GAN) can learn the relationship between two image domains and achieve unpaired image-to-image translation. One of the breakthroughs was Cycle-consistent Generative Adversarial Networks (CycleGAN), which is a popular method to transfer the content representations from the source domain to the target domain. Existing studies have gradually improved the performance of CycleGAN models by modifying the network structure or loss function of CycleGAN. However, these methods tend to suffer from training instability and the generators lack the ability to acquire the most discriminating features between the source and target domains, thus making the generated images of low fidelity and few texture details. To overcome these issues, the present paper proposes a new method that combines Evolutionary Algorithms (EAs) and Attention Mechanisms to train GANs. Specifically, from an initial CycleGAN, binary vectors indicating the activation of the weights of the generators are progressively improved upon by means of an EA. At the end of this process, the best-performing configurations of generators can be retained for image generation. In addition, to address the issues of low fidelity and lack of texture details on generated images, we make use of the channel attention mechanism. The latter component allows the candidate generators to learn important features of real images and thus generate images with higher quality. The experiments demonstrate qualitatively and quantitatively that the proposed method, namely Attention evolutionary GAN (AevoGAN) alleviates the training instability problems of CycleGAN training. In the test results, the proposed method can generate higher-quality images and obtain better results than the CycleGAN training methods present in the literature, in terms of Inception Score (IS), Fréchet Inception Distance (FID) and Kernel Inception Distance (KID).
In recent years, incremental sampling-based motion planning algorithms have been widely used to solve robot motion planning problems in high-dimensional configuration spaces. In particular, the Rapidly-exploring Random Tree (RRT) algorithm and its asymptotically-optimal counterpart called RRT* are popular algorithms used in real-life applications due to its desirable properties. Such algorithms are inherently iterative, but certain modules such as the collision-checking procedure can be parallelized providing significant speedup with respect to sequential implementations. In this paper, the RRT and RRT* algorithms have been adapted to a bioinspired computational framework called Membrane Computing whose models of computation, a.k.a. P systems, run in a non-deterministic and massively parallel way. A large number of robotic applications are currently using a variant of P systems called Enzymatic Numerical P systems (ENPS) for reactive controlling, but there is a lack of solutions for motion planning in the framework. The novel models in this work have been designed using the ENPS framework. In order to test and validate the ENPS models for RRT and RRT*, we present two ad-hoc implementations able to emulate the computation of the models using OpenMP and CUDA. Finally, we show the speedup of our solutions with respect to sequential baseline implementations. The results show a speedup up to 6x using OpenMP with 8 cores against the sequential implementation and up to 24x using CUDA against the best multi-threading configuration.
This article proposes a simplistic algorithmic framework, namely hyperSPAM, composed of three search algorithms for addressing continuous optimisation problems. The Covariance Matrix Adaptation Evolution Strategy (CMAES) is activated at the beginning of the optimisation process as a preprocessing component for a limited budget. Subsequently, the produced solution is fed to the other two single-solution search algorithms. The first performs moves along the axes while the second makes use of a matrix orthogonalization to perform diagonal moves. Four coordination strategies, in the fashion of hyperheuristics, have been used to coordinate the two single-solution algorithms. One of them is a simple randomized criterion while the other three are based on a success based reward mechanism. The four implementations of the hyperSPAM framework have been tested and compared against each other and modern metaheuristics on an extensive set of problems including theoretical functions and real-world engineering problems. Numerical results show that the different versions of the framework display broadly a similar performance. One of the reward schemes appears to be marginally better than the others. The simplistic random coordination also displays a very good performance. All the implementations of hyperSPAM significantly outperform the other algorithms used for comparison.
Epistasis is the correlation between the variables of a function and is a challenge often posed by real-world optimisation problems. Synthetic benchmark problems simulate a highly epistatic problem by performing a so-called problem's rotation. Mutation in Differential Evolution (DE) is inherently rotational invariant since it simultaneously perturbs all the variables. On the other hand, crossover, albeit fundamental for achieving a good performance, retains some of the variables, thus being inadequate to tackle highly epistatic problems. This article proposes an extensive study on rotational invariant crossovers in DE. We propose an analysis of the literature, a taxonomy of the proposed method and an experimental setup where each problem is addressed in both its non-rotated and rotated version. Our experimental study includes 280 problems over five different levels of dimensionality and nine algorithms. Numerical results show that 1) for a fixed quota of transferred design variables, the exponential crossover displays a better performance, on both rotated and non-rotated problems, in high dimensions while the binomial crossover seems to be preferable in low dimensions; 2) the rotational invariant mutation DE/current-to-rand is not competitive with standard DE implementations throughout the entire set of experiments we have presented; 3) DE crossovers that perform a change of coordinates to distribute the moves over the components of the offspring offer high-performance results on some problems. However, on average the standard DE/rand/1/exp appears to achieve the best performance on both rotated and non-rotated testbeds.
The wide deployment of cloud-assisted electronic health (eHealth) systems has already shown great benefits in managing electronic health records (EHRs) for both medical institutions and patients. However, it also causes critical security concerns. Since once a medical institution generates and outsources the patients’ EHRs to cloud servers, patients would not physically own their EHRs but the medical institution can access the EHRs as needed for diagnosing, it makes the EHRs integrity protection a formidable task, especially in the case that a medical malpractice occurs, where the medical institution may collude with the cloud server to tamper with the outsourced EHRs to hide the medical malpractice. Traditional cryptographic primitives for the purpose of data integrity protection cannot be directly adopted because they cannot ensure the security in the case of collusion between the cloud server and medical institution. In this paper, a secure cloud-assisted eHealth system is proposed to protect outsourced EHRs from illegal modification by using the blockchain technology (blockchain-based currencies, e.g., Ethereum). The key idea is that the EHRs only can be outsourced by authenticated participants and each operation on outsourcing EHRs is integrated into the public blockchain as a transaction. Since the blockchain-based currencies provide a tamper-proofing way to conduct transactions without a central authority, the EHRs cannot be modified after the corresponding transaction is recorded into the blockchain. Therefore, given outsourced EHRs, any participant can check their integrity by checking the corresponding transaction. Security analysis and performance evaluation demonstrate that the proposed system can provide a strong security guarantee with a high efficiency.
This paper proposes a novel variant of differential evolution (DE) tailored to the optimisation of noisy fitness functions. The proposed algorithm, namely noise analysis differential evolution (NADE), combines the stochastic properties of a randomised scale factor and a statistically rigorous test which supports one-to-one spawning survivor selection that automatically selects a proper sample size and then selects, among parent and offspring, the most promising solution. The actions of these components are separately analysed and their combined effect on the algorithmic performance is studied by means of a set of numerous and various test functions perturbed by Gaussian noise. Various noise amplitudes are considered in the result section. The performance of the NADE has been extensively compared with a classical algorithm and two modern metaheuristics designed for optimisation in the presence of noise. Numerical results show that the proposed NADE has very good performance with most of the problems considered in the benchmark set. The NADE seems to be able to detect high quality solutions despite the noise and display high performance in terms of robustness.
An artificial compound eye (ACE) is a bio-inspired vision sensor which mimics a natural compound eye (typical of insects). This artificial eye is able to visualize large fields of the outside world through multi-aperture. Due to its functioning, the ACE is subject to optical flow, that is an apparent motion of the object visualized by the eye. This paper proposes a method to estimate the optical flow based on capturing multiple images (multi-aperture). In this method, based on descriptors-based initial optical flows, a unified global energy function is presented to incorporate the information of multi-aperture and simultaneously recover the optical flows of multi-aperture. The energy function imposes a compound flow fields consistency assumption along with the brightness constancy and piecewise smoothness assumptions. This formula efficiently binds the flow field in time and space, and further enables view-consistent optical flow estimation. Experimental results on real and synthetic data demonstrate that the proposed method recovers view-consistent optical flows crossed multi-aperture and performs better than other optical flow methods on the multi-aperture images.