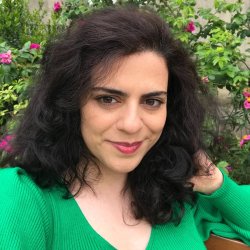
Dr Faegheh Sardari
Publications
Leveraging machine learning techniques, in the context of object-based media production, could enable provision of personalized media experiences to diverse audiences. To fine-tune and evaluate techniques for personalization applications, as well as more broadly, datasets which bridge the gap between research and production are needed. We introduce and publicly release such a dataset, themed around a UK weather forecast and shot against a blue-screen background, of three professional actors/presenters – one male and one female (English) and one female (British Sign Language). Scenes include both production and research-oriented examples, with a range of dialogue, motions, and actions. Capture techniques consisted of a synchronized 4K resolution 16-camera array, production-typical microphones plus professional audio mix, a 16-channel microphone array with collocated Grasshopper3 camera, and a photogrammetry array. We demonstrate applications relevant to virtual production and creation of personalized media including neural radiance fields, shadow casting, action/event detection, speaker source tracking and video captioning.
Leveraging machine learning techniques, in the context of object-based media production, could enable provision of personalized media experiences to diverse audiences. To fine-tune and evaluate techniques for personalization applications, as well as more broadly, datasets which bridge the gap between research and production are needed. We introduce and publicly release such a dataset, themed around a UK weather forecast and shot against a blue-screen background, of three professional actors/presenters – one male and one female (English) and one female (British Sign Language). Scenes include both production and research-oriented examples, with a range of dialogue, motions, and actions. Capture techniques consisted of a synchronized 4K resolution 16-camera array, production-typical microphones plus professional audio mix, a 16-channel microphone array with collocated Grasshopper3 camera, and a photogrammetry array. We demonstrate applications relevant to virtual production and creation of personalized media including neural radiance fields, shadow casting, action/event detection, speaker source tracking and video captioning.
We present PAT, a transformer-based network that learns complex temporal co-occurrence action dependencies in a video by exploiting multi-scale temporal features. In existing methods, the self-attention mechanism in transformers loses the temporal positional information, which is essential for robust action detection. To address this issue, we (i) embed relative positional encoding in the self-attention mechanism and (ii) exploit multi-scale temporal relationships by designing a novel non-hierarchical network, in contrast to the recent transformer-based approaches that use a hierarchical structure. We argue that joining the self-attention mechanism with multiple sub-sampling processes in the hierarchical approaches results in increased loss of positional information. We evaluate the performance of our proposed approach on two challenging dense multi-label benchmark datasets, and show that PAT improves the current state-of-the-art result by 1.1% and 0.6% mAP on the Charades and MultiTHUMOS datasets, respectively, thereby achieving the new state-of-the-art mAP at 26.5% and 44.6%, respectively. We also perform extensive ablation studies to examine the impact of the different components of our proposed network.
We present PAT, a transformer-based network that learns complex temporal co-occurrence action dependencies in a video by exploiting multi-scale temporal features. In existing methods, the self-attention mechanism in transformers loses the temporal positional information, which is essential for robust action detection. To address this issue, we (i) embed relative positional encoding in the self-attention mechanism and (ii) exploit multi-scale temporal relationships by designing a novel non hierarchical network, in contrast to the recent transformer-based approaches that use a hierarchical structure. We argue that joining the self-attention mechanism with multiple sub-sampling processes in the hierarchical approaches results in increased loss of positional information. We evaluate the performance of our proposed approach on two challenging dense multi-label benchmark datasets, and show that PAT improves the current state-of-the-art result by 1.1% and 0.6% mAP on the Charades and MultiTHUMOS datasets, respectively, thereby achieving the new state-of-the-art mAP at 26.5% and 44.6%, respectively. We also perform extensive ablation studies to examine the impact of the different components of our proposed network.