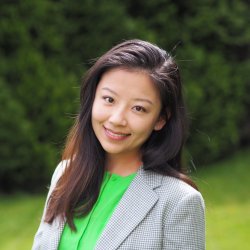
Dr Paloma Liu
Academic and research departments
Faculty of Arts and Social Sciences, Surrey Hospitality and Tourism Management.About
Biography
I am currently working as a research fellow with Dr Nikolas Thomopoulos, Professor Caroline Scarles and Dr Nicola Carey on the HEIF funded project in partnership with Nervtech UK to test the driving support needs of those aged 60+ in the UK.
I am a graduate of the University of Surrey, and after obtaining my MSc in 2017, I moved to West Yorkshire. I was awarded a PhD degree in recognition of a programme of work entitled “Exploring public acceptance of Connected and Autonomous Vehicles (CAVs) with a focus on cybersecurity and privacy risks”, where I investigated CAVs benefits and their associated risks, their regulation and implementation, as well as public perception and acceptance of this technology. This research was sponsored by Huddersfield Business School and the Artificial Intelligence Research Funding initiative of Huddersfield University.
Publications
Connected and Autonomous Vehicles (CAVs) constitute an automotive development carrying paradigm-shifting potential that may soon be embedded into a dynamically changing urban mobility landscape. The complex machine-led dynamics of CAVs make them more prone to data exploitation and vulnerable to cyber attacks than any of their predecessors increasing the risks of privacy breaches and cyber security violations for their users. This can adversely affect the public acceptability of CAVs, give them a bad reputation at this embryonic stage of their development, create barriers to their adoption and increased use, and complicate the business models of their future operations. Therefore, it is vital to identify and create an in-depth understanding of the cyber security and privacy issues associated with CAVs, and of the way these can be prioritised and addressed. This work employs 36 semi-structured elite interviews to explore the diverse dimensions of user acceptance through the lens of the well-informed CAV experts that already anticipate problems and look for their solutions. Our international interviewee sample represents academia, industry and policy-making so that all the key stakeholder voices are heard. Thematic analysis was used to identify and contextualise the factors that reflect and affect CAV acceptance in relation to the privacy and cyber security agendas. Six core themes emerged: awareness, user and vendor education, safety, responsibility, legislation, and trust. Each of these themes has diverse and distinctive dimensions and are discussed as sub-themes. We recommend that mitigating the cyber security and privacy risks embedded in CAVs require inter-institutional cooperation, awareness campaigns and trials for trust-building purposes, mandatory educational training for manufacturers and perhaps more importantly for end-users, balanced and fair responsibility-sharing, two-way dynamic communication channels and a clear consensus on what constitutes threats and solutions.
Health data are being increasingly sensed from the health-based wearable Internet of Things (IoT) devices, providing much-needed fitness and health tracking. However, data generated also present opportunities within computer security, specifically with biometric systems used for identification and authentication purposes. This article performs a systematic review of health-based IoT data collected from wearable IoT technology. This involved performing research in the underlying data sources, what they are collected for in terms of their health monitoring, and the underlying data characteristics. Furthermore, it explores existing work in computer security using these data sources, identifying key themes of work, key limitations, and challenges. Finally, key opportunities are provided as summaries to the potential of health-based IoT data, highlighting challenges that are yet to be addressed, which motivate areas of future work.
Behavioural biometrics have the potential to provide an additional or alternative authentication mechanism to those involving a shared secret (i.e., a password). Keystroke timings are the focus of this study, where key press and release timings are acquired whilst monitoring a user typing a known phrase. Many studies exist in keystroke biometrics, but there is an absence of literature aiming to understand the relationship between characteristics of password policies and the potential of keystroke biometrics. Furthermore, benchmark data sets used in keystroke biometric research do not enable useful insights into the relationship between their capability and password policy. In this work, we consider substitutions of uppercase, numeric, special characters, and their combination on passwords derived from English words. We acquire timings for 42 participants for the same 40 passwords. We implement a matching system using the Manhattan distance measure with seven different feature sets, culminating in an Equal Error Rate of between 6-11% and accuracy values between 89-94%, demonstrating comparable accuracy to other threshold-based systems. Further analysis suggests that the best feature sets are those containing all timings and trigraph press to press. Evidence also suggests that phrases containing fewer characters have greater accuracy, except for those with special character substitutions.
Fitness and activity tracking devices acquire, process and store rich behavioural data that are consumed by the end-user to learn health insights. This rich data source also enables a secondary use of being part of a biometric authentication system. However, there are many open research challenges with the use of data generated by fitness and activity trackers as a biometric source. In this article, the challenge of using data acquired from low-cost devices is tackled. This includes investigating how to best partition the data to deduce repeatable behavioural traits, while maximizing the uniqueness between participant datasets. In this exploratory research, 3 months’ worth of data (heart rate, step count and sleep) for five participants is acquired and utilized in its raw form from low-cost devices. It is established that dividing the data into 14-h segments is deemed the most suitable based on measuring coefficients of variance. Several supervised machine learning algorithms are then applied where the performance is evaluated by six metrics to demonstrate the potential of employing this data source in biometric-based security systems.