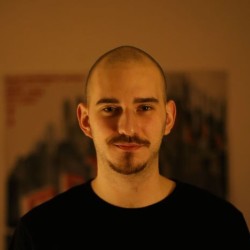
Davide Berghi
Publications
Sound Event Localization and Detection (SELD) is a task that involves detecting different types of sound events along with their temporal and spatial information, specifically, detecting the classes of events and estimating their corresponding direction of arrivals at each frame. In practice, real-world sound scenes might be complex as they may contain multiple overlapping events. For instance, in DCASE challenges task 3, each clip may involve simultaneous occurrences of up to five events. To handle multiple overlapping sound events, current methods prefer multiple output branches to estimate each event, which increases the size of the models. Therefore, current methods are often difficult to be deployed on the edge of sensor networks. In this paper, we propose a method called Probabilistic Localization and Detection of Independent Sound Events with Transformers (PLDISET), which estimates numerous events by using one output branch. The method has three stages. First, we introduce the track generation module to obtain various tracks from extracted features. Then, these tracks are fed into two transformers for sound event detection (SED) and localization, respectively. Finally, one output system, including a linear Gaussian system and regression network, is used to estimate each track. We give the evaluation resn results of our model on DCASE 2023 Task 3 development dataset.
Leveraging machine learning techniques, in the context of object-based media production, could enable provision of personalized media experiences to diverse audiences. To fine-tune and evaluate techniques for personalization applications, as well as more broadly, datasets which bridge the gap between research and production are needed. We introduce and publicly release such a dataset, themed around a UK weather forecast and shot against a blue-screen background, of three professional actors/presenters – one male and one female (English) and one female (British Sign Language). Scenes include both production and research-oriented examples, with a range of dialogue, motions, and actions. Capture techniques consisted of a synchronized 4K resolution 16-camera array, production-typical microphones plus professional audio mix, a 16-channel microphone array with collocated Grasshopper3 camera, and a photogrammetry array. We demonstrate applications relevant to virtual production and creation of personalized media including neural radiance fields, shadow casting, action/event detection, speaker source tracking and video captioning.