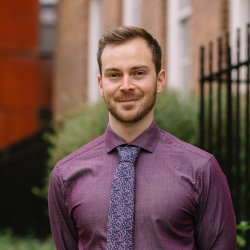
Publications
By weakening Shannon's original axioms to allow for attributes of the choice environment to differ in their associated learning costs, this paper provides an axiomatic foundation for Multi-Attribute Shannon Entropy, a natural multi-parameter generalization of Shannon En-tropy. Sufficient conditions are also provided for a simple dataset that provides a closed-form solution for the Multi-Attribute Shannon Entropy cost function for information by analysing stochastic choice data produced by a rationally inattentive agent that is picking between pairs of options when relatively few states of the world have a positive probability of being realized.
This paper studies a new measure for the cost of learning that allows the different attributes of the options faced by an agent to differ in their associated learning costs. The new measure maintains the tractability of Shannon's classic measure but produces richer choice predictions and identifies a new form of informational bias significant for welfare and counterfactual analysis that is conducted with the multinomial logit model. Necessary and sufficient conditions are provided for optimal agent behavior under the new measure for the cost of learning.