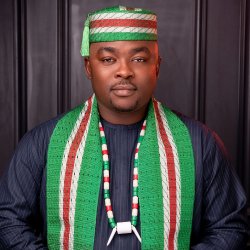
Daniel Ayuba
Academic and research departments
Centre for Vision, Speech and Signal Processing (CVSSP), Faculty of Engineering and Physical Sciences.About
My research project
AI and Robotics for Precision Agriculture using Satellites and UAVThis PhD research project will investigate the use of Artificial Intelligence (AI)
technologies such as robotics, computer vision and deep learning to aid in precision
agriculture (PA). PA is slowly becoming the standard way for farms to remain competitive
in the agricultural marketplace. It allows for higher yields at reduced cost by enabling
farmers and other agricultural stakeholders to monitor the state of a field or crop. This
monitoring comes in many forms, from built-in soil sensors to weather stations, satellites
and, more recently, active robotic platforms such as unmanned aerial vehicles (UAVs)
equipped with multi-spectral sensors. The use of AI has also become key; as more and more
data becomes available, standard human-driven analysis is slowly becoming unfeasible. The
project will focus on leveraging AI technologies to develop key areas of research for PA,
including crop health monitoring, soil quality estimation, as well as other key indicators
of crop yield.
Supervisors
This PhD research project will investigate the use of Artificial Intelligence (AI)
technologies such as robotics, computer vision and deep learning to aid in precision
agriculture (PA). PA is slowly becoming the standard way for farms to remain competitive
in the agricultural marketplace. It allows for higher yields at reduced cost by enabling
farmers and other agricultural stakeholders to monitor the state of a field or crop. This
monitoring comes in many forms, from built-in soil sensors to weather stations, satellites
and, more recently, active robotic platforms such as unmanned aerial vehicles (UAVs)
equipped with multi-spectral sensors. The use of AI has also become key; as more and more
data becomes available, standard human-driven analysis is slowly becoming unfeasible. The
project will focus on leveraging AI technologies to develop key areas of research for PA,
including crop health monitoring, soil quality estimation, as well as other key indicators
of crop yield.
Publications
The use of a pretrained image classification model (trained on cats and dogs, for example) as a perceptual loss function for hyperspectral super-resolution and pansharpening tasks is surprisingly effective. However, RGB-based networks do not take full advantage of the spectral information in hyperspectral data. This inspired the creation of HyperKon, a dedicated hyperspectral Convolutional Neural Network backbone built with self-supervised contrastive representation learning. HyperKon uniquely leverages the high spectral continuity, range, and resolution of hyperspectral data through a spectral attention mechanism. We also perform a thorough ablation study on different kinds of layers, showing their performance in understanding hyperspectral layers. Notably, HyperKon achieves a remarkable 98% Top-1 retrieval accuracy and surpasses traditional RGB-trained backbones in both pansharpening and image classification tasks. These results highlight the potential of hyperspectral-native backbones and herald a paradigm shift in hyperspectral image analysis.