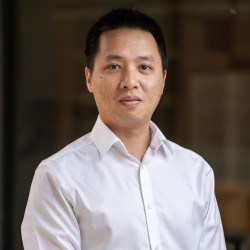
Dr Cuong Nguyen
About
Biography
Cuong Nguyen is a Research Fellow at the Centre for Vision, Speech and Signal Processing, University of Surrey. Before that, he was a Research Associate at The University of Adelaide from April 2022 to January 2024. He received his Ph.D. in Computer Science from The University of Adelaide in March 2022, his M.Phil. in Electronic Engineering also from The University of Adelaide in January 2018, and his B.S. in Mechanical Engineering from Portland State University in June 2012.
My qualifications
Previous roles
ResearchResearch interests
Artificial intelligence, machine learning, Bayesian inference and probabilistic programming
Research interests
Artificial intelligence, machine learning, Bayesian inference and probabilistic programming
Supervision
Postgraduate research supervision
- Arpit Garg (Ph.D. student at the University of Adelaide, co-supervisor)
Teaching
The University of Adelaide, Australia:
- Grand challenges in Computer Science - semester 2/2023
- Grand challenges in Computer Science - semester 2/2022
Publications
Additional publications
- Cuong Nguyen, Thanh-Toan Do and Gustavo Carneiro (2023) Task weighting in meta-learning with trajectory optimisation. In Transactions on Machine Learning Research.
- Cuong Nguyen, Thanh-Toan Do and Gustavo Carneiro (2022) PAC-Bayes meta-learning with implicit task-specific posteriors. In IEEE Transactions on Pattern Analysis and Machine Intelligence, vol. 45, no. 1, pp. 841-851, 1 Jan. 2023, doi: 10.1109/TPAMI.2022.3147798
- Cuong Nguyen, Thanh-Toan Do and Gustavo Carneiro (2021) Probabilistic task modelling for meta-learning. In Uncertainty in Artificial Intelligence. PMLR, pp. 781–791.
- Cuong Nguyen, Thanh-Toan Do and Gustavo Carneiro (2020) Similarity of classification tasks. In NeurIPS 2020 Meta-learning Workshop.
- Cuong Nguyen, Thanh-Toan Do and Gustavo Carneiro (2020) Uncertainty in model-agnostic meta-learning using variational inference. In IEEE/CVF Winter Conference on Applications of Computer Vision.