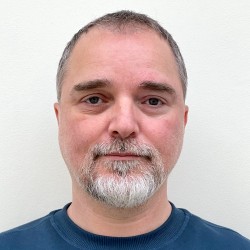
Professor Bogdan Vrusias
Academic and research departments
Computer Science Research Centre, School of Computer Science and Electronic Engineering, Faculty of Engineering and Physical Sciences.About
Biography
My professional career started here, at the University of Surrey, back in September 1994 as an undergraduate student for Computing and Information Technology. After I graduated, I continued as Research Officer, conducting research and transferring technology from the University to businesses. During that time I also started my PhD in the area of multi modal information retrieval, where I suggested a network of synchronously trained Artificial Neural Networks, a kind of an encoder / decoder multi-net for making text queries and retrieving images and vice versa (maybe one could call it an early Transformer model for Generative AI).
Following my PhD I continued at the University as a Lecturer in Intelligent Systems. I started teaching modules such as basic and advanced Artificial Intelligence, Natural Language Processing and Web Technologies (and many more afterwards). I became the Director of Studies for both UG and PG courses and I built new courses such as the Web Hacking Countermeasures, which was unique in UK at that time.
After several years as an academic and around 2007, I created a spinoff company where I was trying to materialise and commercialise my research activities, specifically utilising NLP to scrape information from the unstructured web and build business signals / insights.
After completing my MBA in 2015, I moved out of the University and followed my entrepreneur dream and creating another two businesses. I still kept contact with the University and using the practical knowledge that I had accumulated I continued teaching part-time (until 2023!) the Computer Networking and the new revamped Natural Language Processing course.
During the last 9 years, from 2015 until today (2024) I consulted and supported many startups and I led the most elite team (for 5 years) within Amazon Web Services, the AI / ML Specialist Solution Architects, across Europe, Middle East and Africa, and we built AI / ML solutions for the largest organisations World Wide.
In my current role, since December 2023, as the Global Head of AI and Data Engineering at The Economist, I now have the responsibility to transform the business, complying with the new era of Generative AI and modern data needs. I also have the honour to be back as a Visiting Professor at the Computer Science Research Centre, where I am working on ensuring that data science and data engineering subjects are kept up to date, but I also conduct research activities in the area of large language models, foundation models, and generative AI.
Areas of specialism
My qualifications
Publications
As shared electronic data increases, it has become more difficult to manage it successfully and the demand for scalable and efficient mechanisms for managing and retrieving data effectively becomes essential. In this paper a more effective P2P architecture is presented, aiming to improve existing resource discovery processes. The proposed architecture is organised as a hierarchical super-peer structure, where super-peers of the network represent network's knowledge that is formalised dynamically using its peers' resources. The main focus of this paper is the creation of an adaptive hierarchical concept-based P2P topology using collective intelligence methods. In that process, unmanageable data is transformed into a structured knowledge based repository of semantic resources. Therefore, the network takes the form of an ontology of conceptually related entities of resource information, as provided by the peers. This knowledge driven approach has benefits over traditional load driven architectures, as the user query context is usually the main driver for managing the performance of the network, and in a way the network can be characterised as proactive rather than reactive. A number of experiments have been undertaken and results demonstrate the advantages of the proposed concept-based architecture over other popular architectures.
The increasing amount of online information demands for effective, scalable and accurate mechanisms to manage and search this information. Distributed semantic-enabled architectures, which enforce semantic web technologies for resource discovery, could satisfy these requirements. In this work a semantic-driven adaptive architecture is presented, aiming to improve existing resource discovery processes. The P2P network is organised in a two-layered super-peer architecture. The network formation of super-peers is a conceptual representation of the network’s knowledge, which is shaped from the information provided by the nodes using collective intelligence methods. The main focus of the paper is on the creation of a dynamic hierarchical semantic-driven P2P topology using the network’s collective intelligence. The unmanageable amounts of data are therefore transformed into a repository of semantic knowledge, transforming the network into an ontology of conceptually related entities of information collected from the resources located in the peers. Appropriate experiments have been undertaken through a case study, by simulating the proposed architecture and evaluating the results.
In this paper we explore the distribution of training of self-organised maps (SOM) on grid middleware. We propose a two-level architecture and discuss an experimental methodology comprising ensembles of SOMs distributed over a grid with periodic averaging of weights. The purpose of the experiments is to begin to systematically assess the potential for reducing the overall time taken for training by a distributed training regime against the impact on precision. Several issues are considered: (i) the optimum number of ensembles; (ii) the impact of different types of training data; and (iii) the appropriate period of averaging. The proposed architecture has been evaluated in a grid environment, with clock-time performance recorded.
The need for a generic and adaptable object detection and recognition method in images, is becoming a necessity today, given the rapid development of the internet and multimedia databases in general. This paper compares the state-of-the-art in object recognition and proposes a method based on adaptable models for detecting thematic categories of objects. Furthermore, automatically constructed semantics are used for filtering false positive objects. The classification of objects into categories is performed by the popular Adaboost. The method has been used for identifying car objects and so far has indicated not only accurate recognition performance, but also good adaptability to new objects types.
'Integrated' classification refers to the conjunctive or competitive use of two or more (neural) classifiers. A cooperative neural network system comprising two independently trained Kohonen networks and co-operating with the help of a Hebbian network, is described. The effectiveness of such a network is demonstrated by using it to retrieve images and related texts from a multi-media database. Preliminary results of such an approach appear to be encouraging.
In this paper we explore the distribution of training of self-organised maps (SOM) on Grid middleware. We propose a two-level architecture and discuss an experimental methodology comprising ensembles of SOMs distributed over a Grid with periodic averaging of weights. The purpose of the experiments is to begin to systematically assess the potential for reducing the overall time taken for training by a distributed training regime against the impact on precision. Several issues are considered: (i) the optimum number of ensembles; (ii) the impact of different types of training data; and (iii) the appropriate period of averaging. The proposed architecture has been evaluated in a Grid environment, with clock-time performance recorded.
This paper deals with the problem of semantic transcoding of CCTV video footage. A framework is proposed that combines Computer Vision algorithms that extract visual semantics, together with Natural Language Processing that automatically builds the domain ontology from unstructured text annotations. The final aim is a system that will link the visual and text semantics in order to routinely annotate video sequences with the appropriate keywords of the domain experts ' terminology. © 2007 IEEE.
Visualisation techniques focus on reducing high dimensional data to a low dimensional surface or a cube. Similar dimensional reduction is attempted in the so-called 'self-organising maps'. A number of techniques have been developed to visualise categories learnt by these maps through and exemplified by the term sequential clustering. An evaluation of the techniques is presented using the learning capability of the self-organising maps as a baseline for building systems that learn to visualise complex data.