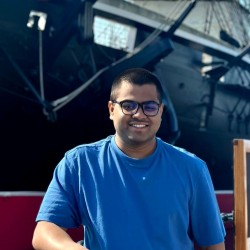
Anindya Mondal
Academic and research departments
Surrey Institute for People-Centred Artificial Intelligence (PAI), Centre for Vision, Speech and Signal Processing (CVSSP).About
My research project
Integrating Auxiliary Information for Representation Learning for the Natural WorldRecent strides in the field of computer vision have been significantly propelled by the availability of meticulously annotated datasets. However, in the natural world, despite the wealth of available data, the process of manual annotation remains a time-consuming and resource-intensive task. This thesis tackles the pivotal question of how to effectively harness the wealth of auxiliary information at our disposal to enhance the learning of visual representations. A transformative paradigm shift is underway in contemporary research, where integrating multi-modal data is emerging as a potent remedy for addressing the scarcity of labeled data. Our research capitalizes on this shift by employing multimodal fusion techniques to address critical challenges in visual representation learning for the natural world. Additionally, we adapt foundational models such as CLIP and SAM, harnessing their remarkable zero-shot capabilities. Through these contributions, we aspire to unlock the untapped potential of auxiliary information to confront the multifaceted challenges that characterize the natural world and beyond.
Recent strides in the field of computer vision have been significantly propelled by the availability of meticulously annotated datasets. However, in the natural world, despite the wealth of available data, the process of manual annotation remains a time-consuming and resource-intensive task. This thesis tackles the pivotal question of how to effectively harness the wealth of auxiliary information at our disposal to enhance the learning of visual representations. A transformative paradigm shift is underway in contemporary research, where integrating multi-modal data is emerging as a potent remedy for addressing the scarcity of labeled data. Our research capitalizes on this shift by employing multimodal fusion techniques to address critical challenges in visual representation learning for the natural world. Additionally, we adapt foundational models such as CLIP and SAM, harnessing their remarkable zero-shot capabilities. Through these contributions, we aspire to unlock the untapped potential of auxiliary information to confront the multifaceted challenges that characterize the natural world and beyond.
Teaching
Demonstrator for EEEM068, APPLIED MACHINE LEARNING - 2023/4
Demonstrator for EEEM071, Advanced Topics in Computer Vision and Deep Learning - 2024
Publications
Existing action recognition methods are typically actor-specific due to the intrinsic topological and apparent differences among the actors. This requires actor-specific pose estimation (e.g., humans vs. animals), leading to cumbersome model design complexity and high maintenance costs. Moreover, they often focus on learning the visual modality alone and single-label classification whilst neglecting other available information sources (e.g., class name text) and the concurrent occurrence of multiple actions. To overcome these limitations, we propose a new approach called ‘actor-agnostic multi-modal multi-label action recognition,’ which offers a unified solution for various types of actors, including humans and animals. We further formulate a novel Multi-modal Semantic Query Network (MSQNet) model in a transformer-based object detection framework (e.g., DETR), characterized by leveraging visual and textual modalities to represent the action classes better. The elimination of actor-specific model designs is a key advantage, as it removes the need for actor pose estimation altogether. Extensive experiments on five publicly available benchmarks show that our MSQNet consistently outperforms the prior arts of actor-specific alternatives on human and animal single- and multi-label action recognition tasks by up to 50%. Code is made available at https://github.com/mondalanindya/MSQNet.