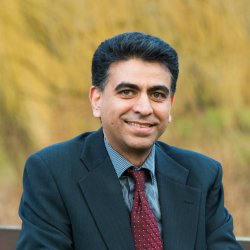
Dr Alireza Tamaddoni-Nezhad
About
Biography
Alireza joined the Department of Computer Science in 2017 as Lecturer in Machine Learning and Computational Intelligence. Prior to this appointment, he was a Research Fellow at the Department of Computing, Imperial College London. He completed his PhD in Computer Science (Machine Learning) at Imperial College where he also worked as a Research Associate on several research projects applying Machine Learning to real-world problems from Biology, Medicine and Ecology. His principal research interests are in the areas of Artificial Intelligence and Machine Learning, in particular the theory, implementations and real-world applications of Relational and Logic-based Machine Learning (also known as Inductive Logic Programming). His current research interests also include: Third Wave AI and Human-Like Computing (e.g. Human-Machine Learning), Explainable AI (e.g. Comprehensible Machine Learning), Data Science, Automated Scientific Discovery, Computational Systems Biology and Bioinformatics, Machine Learning of Biological Networks, and Genetic and Evolutionary Computation.
He was Co-Director of the Surrey Institute for People-Centred AI and currently leading the 'Trustworthy AI' and 'Sustainable AI' research themes at the AI Institute. He is also Co-Investigator and Technical Director of the EPSRC NetworkPlus Grant on Human-Like Computing (HLC) to support the development of a UK-wide multi-disciplinary community of researchers within the EPSRC priority area of Human-Like Computing. He is Principle Investigator of the multi-disciplinary EPSRC project on 'Human-machine learning of ambiguities to support safe, effective, and legal decision making'. He is also Deputy Chair, University Ethics Committee (UEC).
Alireza has been collaborating with researchers within academia and industry and played a central role in developing several Machine Learning approaches for a range of applications in Data Science and Systems Biology (from genetic & metabolic networks to ecosystems). For example, his machine learning and text-mining approach for automated discovery & validation of probabilistic interaction networks from ecological data is regarded as the first successful application of machine learning from large-scale agricultural data. The results include novel food-web hypotheses, some confirmed by subsequent experimental studies (e.g. DNA analysis). Similarly, his work on machine learning of metabolic networks was regarded as the first successful application of machine learning for modeling inhibition in metabolic networks and the method developed in this project was used in studies by other researchers. The results of his research and the above mentioned collaborations have been published in Computer Science journals and proceedings (e.g. Machine Learning Journal) as well as high impact Biological and Ecological Journals (e.g. Advances in Ecological Research, IF=6.3; Trends in Ecology and Evolution, IF=16.7; and Nature Ecology & Evolution).
He is currently Lead Guest Editor for Machine Learning Journal, Special Issue on Learning and Reasoning
ResearchResearch interests
My main research contributions are in the area of Machine Learning, in particular the theory, implementations and real-world applications of Relational and Logic-based Machine Learning aka Inductive Logic Programming (ILP). My research achievements include:
A1. Developing a novel machine learning approach for automated discovery and validation of ecological / microbial interaction networks (selected output [13])
I have developed [13] a novel approach for automated discovery and validation of ecological interaction networks (e.g. food-webs) from ecological abundance data (e.g. census or DNA sequence data) using a combination of logic-based machine learning (which included human-encoded ecological background knowledge) and text-mining from scientific publications (to corroborate and identify novel hypotheses). In an award-winning paper [49], I introduced a new probabilistic Abductive ILP method for approximating the probabilities of hypothetical trophic links. We evaluated this approach on large scale agricultural census data and many of the machine-learned trophic links were corroborated by the literature [16]. In some cases, previously unobserved but high probability links were suggested by machine learning and subsequently confirmed by experimental studies (e.g. DNA analysis). These novel machine-learned food-webs have been the basis of several studies published in high impact scientific journals, including a joint study published [4] in Nature. This machine learning approach was also the basis of several follow-up research grants including Next-Generation Biomonitoring (NGB) [3, 7, 8] where I was a Surrey PI & UK Consortium Lead. As part of this project, we extended our learning approach for the discovery of microbial interaction networks [42,38,1]. Papers [13], [3], [16], [8] and [7] currently have 62, 82, 92, 121 and 200 citations respectively (as of 2024). Papers [38] also received Best Application Paper Award. Papers [4], [7] and [8] also achieved 4*/3*, 4*, 4* scores respectively in Annual Output Review (AOR). Paper [4] was submitted to REF 2021.
A2. Contributing to the framework of Meta-Interpretive Learning (MIL) (selected publication [11])
I have contributed to the theory, implementation, and real-world applications of Meta-Interpretive Learning (MIL) and was co-author on the first publications on MIL [11, 12]. MIL has been applied in challenging problems including grammatical inference, learning string transformations, learning robot strategies and Logical Vision (LV). Due to its symbolic paradigm and background knowledge guided mid-level features extraction, high-level visual concepts learning can be simply implemented by logic programming. Initial experiments conducted on learning simple shapes (e.g. regular polygons) suggested that this technique outperforms existing state-of-the-art object recognition methods. We also investigated [5] methods for extending these initial experiments to real-world images and videos, where my implementation of noise-tolerant MIL (i.e. MetagolNT) was used for one-shot learning from (noisy) images. The Stochastic Refinement approach [51] introduced in my PhD, was adapted for a Bayesian framework for MIL (i.e. MetaBayes) [48] which is shown to be useful in challenging problems such as learning from large scale textual data. MIL has been also used in the experiments on human comprehensibility and ultra-strong machine learning [6]. It was also shown [12] that MIL can learn context-free grammars efficiently and accurately from a small number of examples. The original MIL framework introduced in [11, 12] has been adapted by other research groups, including researchers from Google DeepMind (Evans & Grefenstette, JAIR, 61:1-64, 2018). The number of citations for [11], [12], [6] and [5] are 279, 163, 129 and 39 respectively (as of 2024). Papers [11], [12], [5] and [6] also achieved 4 x 4*/3* in Annual Output Review (AOR). Paper [12] was submitted to REF 2021.
A3. Explainable machine learning for Integrative Systems Biology (selected publication [21])
I played a central role in developing a series of logic-based machine learning methods for integrative systems biology applications ranging from modelling inhibition in metabolic pathways for predictive toxicology [19, 21] to modelling genotype-phenotype relations for novel vaccine discovery [15, 17]. I used a logic-based representation and a combination of Abduction and Induction to model biological systems. In general, the integration of abduction and induction is required when the given background knowledge is incomplete. This condition holds in several challenging problems in systems biology. For example, in modelling inhibition in metabolic pathways (important in drug discovery), background knowledge, which represents the present state of understanding (known pathways or gene functions), is incomplete. The proposed Abductive ILP method and the case study described in [21] is regarded as the first successful application of machine learning for modelling inhibition in metabolic networks and was also used in several follow-up studies by other researchers. The underlying logic-based representation also allows the integration of diverse background knowledge and data (e.g. genetic, metabolic, etc). I also extended this approach to use with other biological data. For example, in [15] a mixture of metabolic data, gene expression and mutant data (gene knockout experiments) were used together to model genotype-phenotype relations in biosynthetic pathways for capsular polysaccharides of Campylobacter jejuni. My research is this area demonstrated the feasibility of a process of scientific model development through logical abduction / induction. The original approach described in [21] has been adapted in follow-up studies by other researchers. Paper [21] currently has 114 citations (as of 2024).
A4. Novel theoretical framework and implementations for efficient and explainable machine learning using a combination of logic-based and stochastic approaches (selected publication [18])
Searching a bounded hypothesis space is the basis of several state-of-the-art ILP systems (e.g. Progol, Aleph) which have been applied to many real-world problems. As part of my PhD, I analysed the lattice structure and refinement operators for the bounded hypothesis space in ILP and introduced the concept of bounded subsumption (i.e. subsumption relative to a bottom clause) [18]. I have also shown that the bounded subsumption lattice is isomorphic to atomic lattice and that the general subsumption testing, which is NP-complete, can be reduced to unification which has a linear time complexity. I also developed novel stochastic search methods including a Genetic Algorithm approach for ILP [20, 59, 60, 61]. The theoretical results were also the basis of several novel refinement operators & implementations in ILP, including Asymmetric Relative Minimal Generalisation (or ARMG) implemented in ProGolem [52]. Unlike Plotkin’s RLGG where clause length grows exponentially in the number of examples, in ARMGs clause length is bounded by the length of the initial bottom clause. ProGolem has been successfully applied (e.g. Santos et al, BMC Bioinformatics, 13(162), 2012) in challenging real-world problems such as learning of complex chemical properties for drug discovery. We have recently developed a novel and efficient implementation of this approach in PyGol [38]. The number of current citations for papers [52], [18], [20], [59] and [61] are 68, 46, 41, 35, 35 respectively (as of 2024). Paper [20] also received the MLJ's Best Theory Paper Award.
Research projects
Role: PI, Funder: EPSRC, Dates: 2023-2026, Total amount = £1.1M
Role: CoI and Technical Director, Funder: EPSRC, Dates: 2018-202, Total amount = £1.6M
Research interests
My main research contributions are in the area of Machine Learning, in particular the theory, implementations and real-world applications of Relational and Logic-based Machine Learning aka Inductive Logic Programming (ILP). My research achievements include:
A1. Developing a novel machine learning approach for automated discovery and validation of ecological / microbial interaction networks (selected output [13])
I have developed [13] a novel approach for automated discovery and validation of ecological interaction networks (e.g. food-webs) from ecological abundance data (e.g. census or DNA sequence data) using a combination of logic-based machine learning (which included human-encoded ecological background knowledge) and text-mining from scientific publications (to corroborate and identify novel hypotheses). In an award-winning paper [49], I introduced a new probabilistic Abductive ILP method for approximating the probabilities of hypothetical trophic links. We evaluated this approach on large scale agricultural census data and many of the machine-learned trophic links were corroborated by the literature [16]. In some cases, previously unobserved but high probability links were suggested by machine learning and subsequently confirmed by experimental studies (e.g. DNA analysis). These novel machine-learned food-webs have been the basis of several studies published in high impact scientific journals, including a joint study published [4] in Nature. This machine learning approach was also the basis of several follow-up research grants including Next-Generation Biomonitoring (NGB) [3, 7, 8] where I was a Surrey PI & UK Consortium Lead. As part of this project, we extended our learning approach for the discovery of microbial interaction networks [42,38,1]. Papers [13], [3], [16], [8] and [7] currently have 62, 82, 92, 121 and 200 citations respectively (as of 2024). Papers [38] also received Best Application Paper Award. Papers [4], [7] and [8] also achieved 4*/3*, 4*, 4* scores respectively in Annual Output Review (AOR). Paper [4] was submitted to REF 2021.
A2. Contributing to the framework of Meta-Interpretive Learning (MIL) (selected publication [11])
I have contributed to the theory, implementation, and real-world applications of Meta-Interpretive Learning (MIL) and was co-author on the first publications on MIL [11, 12]. MIL has been applied in challenging problems including grammatical inference, learning string transformations, learning robot strategies and Logical Vision (LV). Due to its symbolic paradigm and background knowledge guided mid-level features extraction, high-level visual concepts learning can be simply implemented by logic programming. Initial experiments conducted on learning simple shapes (e.g. regular polygons) suggested that this technique outperforms existing state-of-the-art object recognition methods. We also investigated [5] methods for extending these initial experiments to real-world images and videos, where my implementation of noise-tolerant MIL (i.e. MetagolNT) was used for one-shot learning from (noisy) images. The Stochastic Refinement approach [51] introduced in my PhD, was adapted for a Bayesian framework for MIL (i.e. MetaBayes) [48] which is shown to be useful in challenging problems such as learning from large scale textual data. MIL has been also used in the experiments on human comprehensibility and ultra-strong machine learning [6]. It was also shown [12] that MIL can learn context-free grammars efficiently and accurately from a small number of examples. The original MIL framework introduced in [11, 12] has been adapted by other research groups, including researchers from Google DeepMind (Evans & Grefenstette, JAIR, 61:1-64, 2018). The number of citations for [11], [12], [6] and [5] are 279, 163, 129 and 39 respectively (as of 2024). Papers [11], [12], [5] and [6] also achieved 4 x 4*/3* in Annual Output Review (AOR). Paper [12] was submitted to REF 2021.
A3. Explainable machine learning for Integrative Systems Biology (selected publication [21])
I played a central role in developing a series of logic-based machine learning methods for integrative systems biology applications ranging from modelling inhibition in metabolic pathways for predictive toxicology [19, 21] to modelling genotype-phenotype relations for novel vaccine discovery [15, 17]. I used a logic-based representation and a combination of Abduction and Induction to model biological systems. In general, the integration of abduction and induction is required when the given background knowledge is incomplete. This condition holds in several challenging problems in systems biology. For example, in modelling inhibition in metabolic pathways (important in drug discovery), background knowledge, which represents the present state of understanding (known pathways or gene functions), is incomplete. The proposed Abductive ILP method and the case study described in [21] is regarded as the first successful application of machine learning for modelling inhibition in metabolic networks and was also used in several follow-up studies by other researchers. The underlying logic-based representation also allows the integration of diverse background knowledge and data (e.g. genetic, metabolic, etc). I also extended this approach to use with other biological data. For example, in [15] a mixture of metabolic data, gene expression and mutant data (gene knockout experiments) were used together to model genotype-phenotype relations in biosynthetic pathways for capsular polysaccharides of Campylobacter jejuni. My research is this area demonstrated the feasibility of a process of scientific model development through logical abduction / induction. The original approach described in [21] has been adapted in follow-up studies by other researchers. Paper [21] currently has 114 citations (as of 2024).
A4. Novel theoretical framework and implementations for efficient and explainable machine learning using a combination of logic-based and stochastic approaches (selected publication [18])
Searching a bounded hypothesis space is the basis of several state-of-the-art ILP systems (e.g. Progol, Aleph) which have been applied to many real-world problems. As part of my PhD, I analysed the lattice structure and refinement operators for the bounded hypothesis space in ILP and introduced the concept of bounded subsumption (i.e. subsumption relative to a bottom clause) [18]. I have also shown that the bounded subsumption lattice is isomorphic to atomic lattice and that the general subsumption testing, which is NP-complete, can be reduced to unification which has a linear time complexity. I also developed novel stochastic search methods including a Genetic Algorithm approach for ILP [20, 59, 60, 61]. The theoretical results were also the basis of several novel refinement operators & implementations in ILP, including Asymmetric Relative Minimal Generalisation (or ARMG) implemented in ProGolem [52]. Unlike Plotkin’s RLGG where clause length grows exponentially in the number of examples, in ARMGs clause length is bounded by the length of the initial bottom clause. ProGolem has been successfully applied (e.g. Santos et al, BMC Bioinformatics, 13(162), 2012) in challenging real-world problems such as learning of complex chemical properties for drug discovery. We have recently developed a novel and efficient implementation of this approach in PyGol [38]. The number of current citations for papers [52], [18], [20], [59] and [61] are 68, 46, 41, 35, 35 respectively (as of 2024). Paper [20] also received the MLJ's Best Theory Paper Award.
Research projects
Role: PI, Funder: EPSRC, Dates: 2023-2026, Total amount = £1.1M
Role: CoI and Technical Director, Funder: EPSRC, Dates: 2018-202, Total amount = £1.6M
Supervision
Postgraduate research supervision
Human-Machine Learning and Reasoning Lab
2017-24: Supervised 19 PhD students, 7 as primary supervisor (1 successfully completed, 4 passed confirmation) and 12 as secondary supervisor (5 successfully completed, 4 passed confirmation). Supervised 16 MSc project and 22 UG final projects to successful completion.
Teaching
2020-Present: Machine Learning and Data Mining (COMM055) [Module Leader]
2017-2019: Practical Business Analytics (COM3018/COMM053) [Module Leader]
Publications
[ A more up to date list and pdf files are available from: https://hmlr-lab.github.io/publications ]
Selected Journal Articles
[1] D Barroso-Bergada, A Tamaddoni-Nezhad, D Varghese, C Vacher, N Galic, V Laval, F Suffert, and D Bohan, Unravelling the web of dark interactions: explainable inference of the diversity of microbial interactions, Advances in Ecological Research, 68:155-183, 2023
[2] A. Soliman, J. O'Connell, A. Tamaddoni-Nezhad, Data-driven revenue characterisation and analysis of long-haul low-cost carriers in the Southeast Asian market, Jour. of Air Trans. Mang, 103 ,2022
[3] A. Makiola, Z. Compson, D. Baird, M. Barnes.., A. Tamaddoni-Nezhad..,Key questions for next-generation biomonitoring, Frontiers in Env. Sci., 7:197, Frontiers, 2020.
[4] A. Ma, X. Lu, C. Gray, A. Raybould, A. Tamaddoni-Nezhad, G. Woodward, D. Bohan. Ecological networks reveal resilience of agro-ecosystems to changes in farming management. Nature Ecology & Evolution 3:260-264, 2019
[5] S.H. Muggleton, W-Z. Dai, C. Sammut, A. Tamaddoni-Nezhad, J. Wen and Z-H. Zhou. Meta-interpretive learning from noisy images. Machine Learning, 107:1097-1118, 2018.
[6] S.H. Muggleton, U. Schmid, C. Zeller, A. Tamaddoni-Nezhad, and Besold. Ultra-strong machine learning - comprehensibility of programs learned with ILP. Machine Learning, 107:1119-1140, 2018
[7] D. Bohan, C. Vacher, A. Tamaddoni-Nezhad, A. Raybould, A. Dumbrell and G. Woodward, Next- Generation Global Biomonitoring: Large-scale, automated reconstruction of ecological networks. Trends in Ecology and Evolution, 32(7):477-487, 2017
[8] C. Vacher, A. Tamaddoni-Nezhad, S. Kamenova, N. Peyrard, Y. Moalic, R. Sabbadin, L. Schwaller, J. Chiquet, M. Smith, J. Vallance, V. Fievet, D. Bohan, Learning Ecological Networks from Next-Generation Sequencing Data. Advances in Ecol. Res., 54, 1-39, 2016.
[9] M. Pocock, D. Evans, C. Fontaine, M. Harvey, R. Julliard, Ó. McLaughlin, J. Silvertown, A. Tamaddoni-Nezhad, P. White, and D. Bohan, The visualisation of ecological networks, and their use as a tool for engagement, advocacy and management, Adv. in Ecol. Research, 54, 1-39, 2016.
[10] QUINTESSENCE Consortium, Networking our way to better Ecosystem Service provision. Trends in Ecology and Evolution, 31(2):105-115, 2016.
[11] S.H. Muggleton, D. Lin and A. Tamaddoni-Nezhad, Meta-interpretive learning of higher-order dyadic datalog: predicate invention revisited, Machine Learning, 100(1):49-73, 2015. (Citations: 279)
[12] S.H. Muggleton, D. Lin, N. Pahlavi, and A. Tamaddoni-Nezhad. Meta-Interpretive Learning: application to Grammatical Inference. Machine Learning, 94:25-49, 2014
[13] A. Tamaddoni-Nezhad, G. Afroozi Milani, A. Raybould, S. Muggleton and D.Bohan, Construction and Validation of Agricultural Food-webs using Logic-based Machine Learning and Text-mining, Advances in Ecological Research, vol. 49, pages 224-290, 2013.
[14] D. Bohan, A. Raybould, C. Mulder, G. Woodward, A. Tamaddoni-Nezhad, N. Bluthgen, M. Pocock, S. Muggleton, D. Evans, J. Astegiano, F. Massol, N. Loeuille, Networking Agroecology: Integrating the diversity of agroecosystem interactions, Adv. in Eco. Res., vol. 49, pages 2-67, 2013.
[15] M. Sternberg, A. Tamaddoni-Nezhad, V. Lesk, E. Kay, P. Hitchen, A. Cootes, L. Alphen, M. Lamoureux, H. Jarrell, C. Rawlings, E. Soo, C. Szymanski, A. Dell, B. Wren, S. Muggleton. Gene function hypotheses for the Campylobacter jejuni glycome generated by a logic-based approach. Jour of Mol. Bio., 425(1):186-197, 2013.
[16] D. A. Bohan, G. Caron-Lormier, S. Muggleton, A. Raybould and A. Tamaddoni-Nezhad. Automated Discovery of Food Webs from Ecological Data Using Logic-Based Machine Learning. PloS One, vol. 6, pp. e29028, 2011.
[17] E Kay, V Lesk, A. Tamaddoni-Nezhad, P Hitchen, A Dell, M. Sternberg, S. Muggleton, B Wren. Systems analysis of bacterial glycomes. Bioch. Soc. Trans., 38(5), pp.1290–1293, 2010.
[18] A. Tamaddoni-Nezhad and S.H. Muggleton. The lattice structure and refinement operators for the hypothesis space bounded by a bottom clause. Machine Learning, 76(1):37-72, 2009.
[19] A. Tamaddoni-Nezhad, R. Chaleil, A. Kakas, M. Sternberg, J. Nicholson, and S. Muggleton. Modeling the effects of toxins in metabolic networks. IEEE Engineering in Medicine and Biology, 26:37-46, 2007.
[20] S.H. Muggleton and A.Tamaddoni-Nezhad. QG/GA: A stochastic search approach for Progol. Machine Learning, 70 (2–3):123–133, 2007. (MLJ's Best Theory Paper Award)
[21] A. Tamaddoni-Nezhad, R. Chaleil, A. Kakas, and S.H. Muggleton. Application of abductive ILP to learning metabolic network inhibition from temporal data. Machine Learning, 64:209–230, 2006.
Selected Book Chapters / Edited Book
[22] A. Tamaddoni-Nezhad, D. Bohan, G. Milani, A. Raybould and S. Muggleton. Human-Machine Scientific Discovery. Book chapter in Human-Like Machine Intelligence, Oxford University Press, 2021
[23] D. Bohan, D. Gravel, A. Tamaddoni-Nezhad, C. Vacher, S. Robin, Eds. A Next-Generation of Biomonitoring to Detect Global Ecosystem Change. Frontiers Media SA. 2020.
[24] A. Tamaddoni-Nezhad, D. Lin, H. Watanabe, J. Chen and S. Muggleton, Machine Learning of Biological Networks using Abductive ILP, In Eds. Cerro &Inoue, Logical Modeling of Biological Systems, pp 363-401, ISTE-Wesley, 2014
Selected Refereed Conference and Workshop Papers
[25] D. Varghese, D. Cyrus, S. Patsantzis, J. Trewern, A. Treloar, A. Hunter and A. Tamaddoni-Nezhad, One-Shot Learning of Autonomous Behaviour: A Meta-interpretive Learning approach, In Proc. of the 33rd Int. Conf. on Inductive Logic Programming (ILP), Springer, 2024 (Accepted).
[26] S. Patsantzis and A. Tamaddoni-Nezhad, From model-based learning to model-free behaviour with Meta-Interpretive Learning, In Proc. of the 33rd Int. Conf. on Ind. Log. Prog (ILP), Springer, 2024 (Accepted).
[27] Z. Chaghazardi, S. Fallah, and A. Tamaddoni-Nezhad, Trustworthy Vision for Autonomous Vehicles: A Robust Logic-infused Deep Learning Approach, In Proc. of the IEEE Int. Conf. Intel. Tran. Sys (ITSC), 2024 (Accepted).
[28] D. Cyrus, D. Varghese, and A. Tamaddoni-Nezhad, An Inductive Logic Programming approach for feature-range discovery, In Proc. of the 33rd Int. Conf. on ILP, Springer, 2024 (Accepted, Best Student Paper Award)
[29] Z. Chaghazardi, S. Fallah, and A. Tamaddoni-Nezhad, Leveraging Inductive Logic Programming and Deep Learning for Trustworthy Vision, In Proc. of the 33rd Int. Conf. on ILP, Springer, 2024 (Accepted).
[30] J. Trewern and S. Patsantzis and Alireza Tamaddoni-Nezhad, Meta-Interpretive learning as Second Order Resolution, In Proc. of the 33rd Int. Conf. on Inductive Logic Programming (ILP), Springer, 2024 (Accepted).
[31] D. Varghese and A. Tamaddoni-Nezhad, Towards enhancing LLMs with logic-based reasoning, In Proc. of the 4th Int. Conf. on Learn and Reasoning (IJCLR), Springer, 2024 (Accepted).
[32] D Cyrus, J Trewern, A Tamaddoni-Nezhad, Meta Interpretive Learning of Fractals. In Proc. of the 32nd Int Conf on Inductive Logic Programming, ILP 2023, pp. 166-174, Springer, 2023
[33] D Varghese, R Bauer, A Tamaddoni-Nezhad, Few-shot learning of diagnostic rules for neurodegenerative diseases using Inductive Logic Programming, In Proc. of the Int Conf on ILP, pp. 109-123, Springer, 2023
[34] Z Chaghazardi, S Fallah, A Tamaddoni-Nezhad, A Logic-based Compositional Generalisation Approach for Robust Traffic Sign Detection, In IJCAI 2023 Workshop on Knowledge-Based Compos Generalization, 2023
[35] D Cyrus, G Milani, A Tamaddoni-Nezhad, Explainable Game Strategy Rule Learning from Video. In Proc. of 17th Int. Rule Challenge Conf on RuleML+RR Challenge, 8-10, 2023.
[36] Z Chaghazardi, S Fallah, A Tamaddoni-Nezhad, Explainable and Trustworthy Traffic Sign Detection for Safe Autonomous Driving: An ILP Approach, In Proc. of Int Conf on Logic Programming, 201-212, 2023.
[37] G A Milani, D Cyrus, A Tamaddoni-Nezhad, Towards One-Shot Learning for Text Classification using ILP, In Proc of Int Conf on Logic Programming, 69-79, 2023
[38] D. Varghese, D. Barroso-Bergada, D. Bohan and A. Tamaddoni-Nezhad, Efficient Abductive Learning of Microbial Interactions using Meta Inverse Entailment, In Proc. of the 31th Int. Conf. on Inductive Logic Programming, 127-141, Springer, 2023 (Best Application Paper Award)
[39] M. Yildirim, S. Mozaffari, L McCutcheon, M Dianati, A Tamaddoni-Nezhad, S Fallah, Prediction based decision making for autonomous highway driving, In Proc. of IEEE 25th Int Conf. on Intel. Trans. Sys (ITSC), 138-145, 2022
[40] D. Varghese, U. Patel, P. Krause, A. Tamaddoni-Nezhad, Few-Shot Learning for Plant Disease Classification Using ILP. In Proc. Int Advanced Computing Conf., 321-336, 2022
[41] A. Soliman, J. O'Connell, A. Tamaddoni-Nezhad, Application of Relational Machine Learning to construct Explainable AI models from airline big data, In Proc. of the 25th Air Trans. Res. Soc., ATRS, 103:102242, 2022
[42] D. Barroso-Bergada, A. Tamaddoni-Nezhad, S. Muggleton, C. Vacher, N. Galic, D. Bohan, Machine learning of microbial interactions In Proc. of the Int. Conf. on ILP, pp 26-40, Springer-Verlag, 2022
[43] D. Varghese, R. Bauer, D. Baxter-Beard, S. Muggleton, A. Tamaddoni-Nezhad, Human-like rule learning from images using one-shot hypothesis derivation, In Proc. of the Int. Conf. on ILP, pp 234-250, Springer, 2022
[44] D. Varghese, A. Tamaddoni-Nezhad, One-Shot Rule Learning for Challenging Character Recognition, In Proc. of Int Conf. Declarative AI / RuleML Challenge, CEUR-WS, vol 2644, pages 10-27, 2020.
[45] W-Z Dai, S.H. Muggleton, J. Wen, A. Tamaddoni-Nezhad, and Z-H. Zhou. Logical vision: One-shot meta-interpretive learning from real images. In Proc. of the Int. Conf. on ILP, Springer, pp 46-62, 2018..
[46] A. Cropper, A. Tamaddoni-Nezhad, and S. Muggleton. Meta-interpretive learning of data transformation programs. In Proc. of the 25th Intl. Con. on Inductive Logic Programming, pages 46-59, 2016.
[47] A. Tamaddoni-Nezhad, D. Bohan, A. Raybould and S. Muggleton. Towards machine learning of predictive models from ecological data. In Proc. of the Int. Conf. on ILP, Springer, pages 154-167, 2015. (AOR 3*)
[48] S.H. Muggleton, D. Lin, J. Chen, and A. Tamaddoni-Nezhad. Metabayes: Bayesian meta-interpretative learning using higher-order stochastic refinement. In Proc. of the Int. Conf. on ILP, pages 1-17, 2014 (AOR 3*)
[49] A. Tamaddoni-Nezhad, D. Bohan, A. Raybould and S. Muggleton. Machine learning a probabilistic network of ecological interactions. In Proc. of the Int. Conf. on ILP, 332-346, 2012. (Best Application Paper Award)
[50] S.H. Muggleton, D. Lin, and A. Tamaddoni-Nezhad. MC-Toplog: Complete multi-clause learning guided by a top theory. In Proc. of the 21st Int. Conf. on ILP, LNAI 7207, pages 238-254, 2012.
[51] A. Tamaddoni-Nezhad and S.H. Muggleton. Stochastic Refinement. In Proceedings of the 20th International Conference on Inductive Logic Programming, pages 222-237, 2011.
[52] S.H. Muggleton, J. Santos, and A. Tamaddoni-Nezhad. ProGolem: a system based on relative minimal generalisation. In Proc. of the 19th Int. Conf. on ILP, pages 131-148. Springer-Verlag, 2010. (Citations: 68)
[53] S.H. Muggleton, J. Santos, and A. Tamaddoni-Nezhad. TopLog: ILP using a logic program declarative bias. In Proceedings of the Int. Conf. on Logic Programming 2008, LNCS 5366, pages 687-692. Springer-Verlag, 2010.
[54] A. Tamaddoni-Nezhad, R. Barton,..M. Sternberg, B. Wren, S. Muggleton, A logic-based approach for modeling genotype-phenotype relations in Campylobacter, In Proc. Int Con. Sys. Biology (ICSB-2008), 2008.
[55] A. Tamaddoni-Nezhad and S.H. Muggleton. A note on refinement operators for IE-based ILP systems. In Proceedings of the 18th Int. Conf. on Inductive Logic Programming, pages 297-314. Springer-Verlag, 2008.
[56] A. Tamaddoni-Nezhad, A. Kakas, S.H. Muggleton, and F. Pazos. Modelling inhibition in metabolic pathways through abduction and induction. In Proc. of the 14th Int. Conf. on ILP, pages 305-322. Springer-Verlag, 2004.
[57] S.H. Muggleton, A. Tamaddoni-Nezhad, and H. Watanabe. Induction of enzyme classes from biological databases. In Proc. of the 13th Int. Conf. on Inductive Logic Programming, pp 269-280. Springer-Verlag, 2003.
[58] A. Tamaddoni-Nezhad, S. Muggleton, and J. Bang. A Bayesian model for metabolic pathways. In Int. Joint Con. on AI (IJCAI03) Workshop on Learning Statistical Models from Relational Data, pp 50-57, 2003.
[59] A. Tamaddoni-Nezhad and S.H. Muggleton. A genetic algorithms approach to ILP. In Proceedings of the 12th International Conference on Inductive Logic Programming, pages 285-300. Springer-Verlag, 2002.
[60] A. Tamaddoni-Nezhad and S.H. Muggleton. Using genetic algorithms for learning clauses in first-order logic. In Proc. of the Genetic and Evolutionary Computation Conference, GECCO-2001, pp 639-646, 2001
[61] A. Tamaddoni-Nezhad and S.H. Muggleton. Searching the subsumption lattice by a genetic algorithm. In Proceedings of the 10th Int. Conf. on Inductive Logic Programming, pages 243-252. Springer-Verlag, 2000.