Fundamental Mathematics Group
Pioneering Mathematical Knowledge
This group hosts the fundamental mathematics in the School. It involves both pure and applied mathematics and covers any area of mathematics that enriches the discipline of mathematics, and includes algebra, analysis, differential equations, geometry, and their offshoots.
Welcome from the head of group
Our group carries out research into fundamental mathematics across the subject from pure to applied. Topics include nonlinear partial differential equations (including infinite-dimensional dynamical systems, analysis, calculus of variations and asymptotics), fluid dynamics (hydrodynamic stability, breaking water waves, oceanographic patterns, mathematical
Dr Bin Cheng
Head of Group
News from our group members
Study with us
If you have a query regarding our new MSc degree programme, then please get in touch with any member of academic staff of our group.
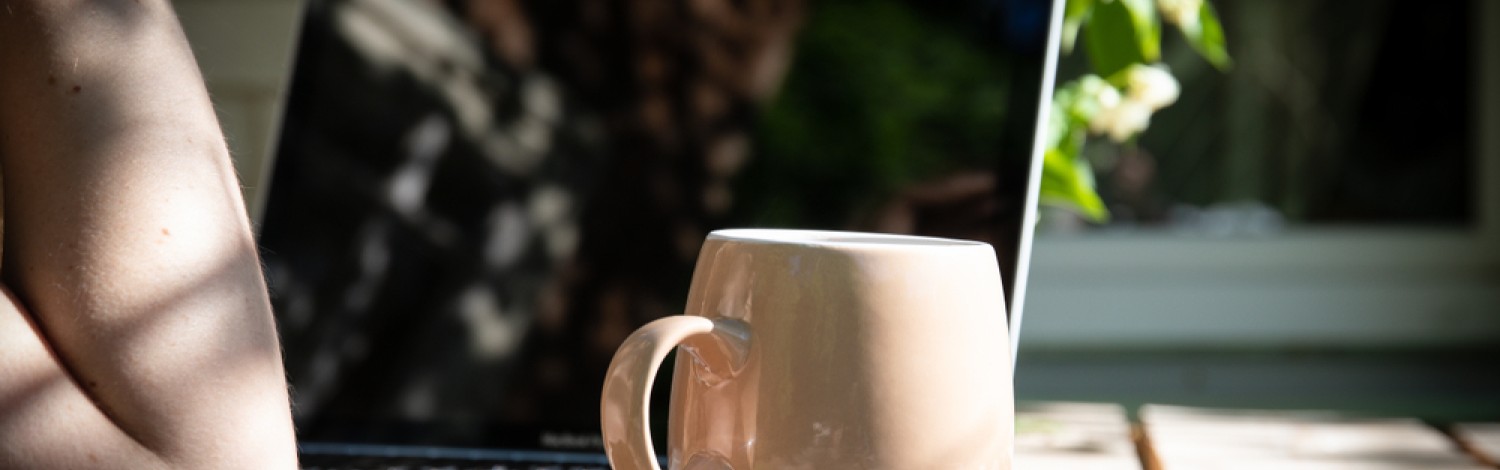
Find out about the latest in our blog
Our blog collects updates from all of us about journal articles, conference talks, and much more!