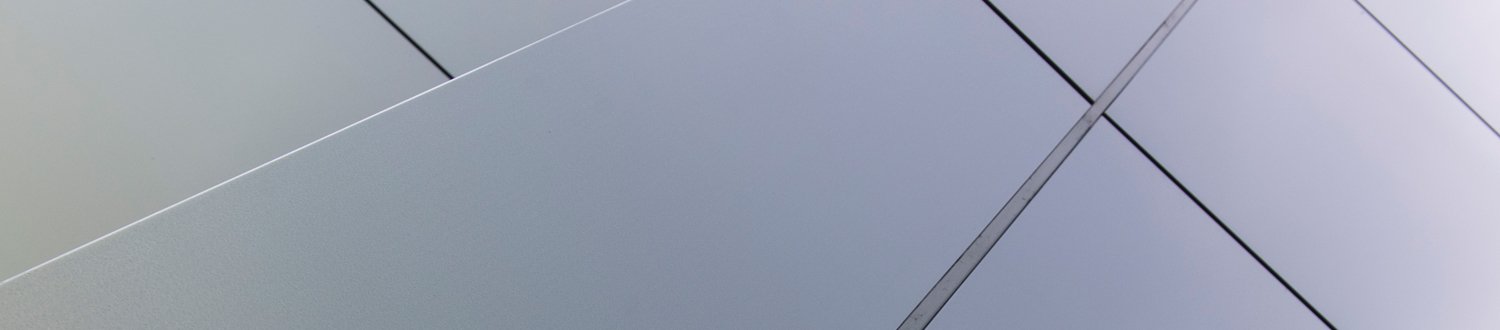
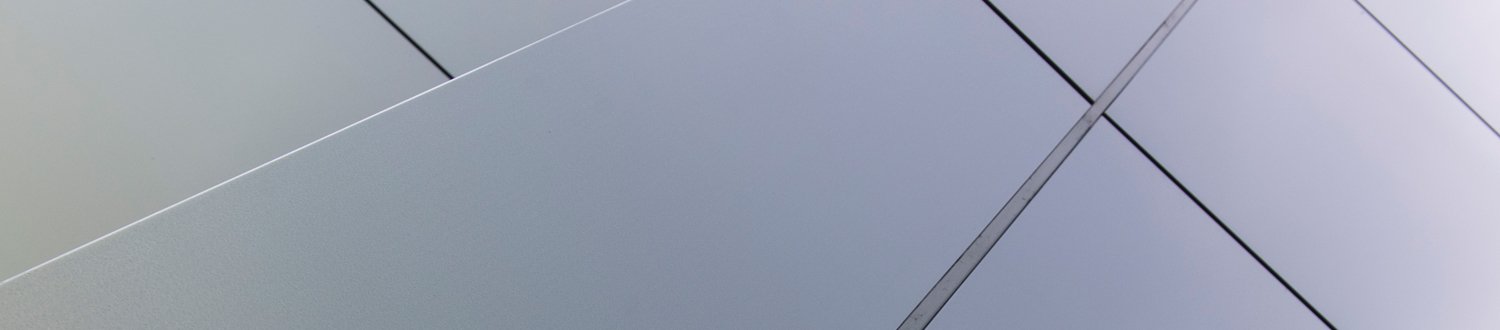
Analysing survey data
The qualitative innovations in CAQDAS (QUIC) project focused on how computer assisted qualitative data analysis (CAQDAS) software packages support mixed methods by integrating qualitative and quantitative data.
Support materials
The support materials below were developed as part of our work on the use of selected CAQDAS packages to conduct secondary analysis of qualitative data on the social factors in response to natural environmental risk arising from climate change. The materials are not necessarily relevant to the preparation or analysis of other types of qualitative data.
This diagram (PDF) illustrates how the individual sections fit together as a resource. The software tools discussed below are illustrated with examples from the post flooding event survey.
About the sample data
This section sets out the main features of the dataset that was used to explore the features and capabilities of the CAQDAS packages under review in the analysing survey data section.
A wide variety of different datasets could fall within the description of 'open-ended survey question data' making it impossible to cover a comprehensive range of possible scenarios. We hope that the dataset we used includes sufficient features to help most users find something of interest.
Background to the survey
The survey was carried out by British Market Research Bureau (BMRB) during 2001. It was commissioned by the Environment Agency (EA) as part of a series of research into the effects of serious flooding events on households and communities. This particular survey was called a 'post event' survey because it was focussed on communities that had experienced significant flooding events in the preceding 12 months. The survey data is characterised by a fairly large number of short statements.
Facts and figures
The data was collected in 12 separate areas, all in England. Samples were drawn from addresses within the EA’s 'at risk' register, these were the addresses of properties that might be expected to be flooded under some circumstances, e.g. homes in flood plains. Interviews were carried out face to face by experienced BMRB interviewers and the responses were keyed into laptop computers as the interview progressed. Most of the questions were closed question type with pre-set multiple choice answer categories. At various points in the interview schedule these were supplemented with open-ended questions, where the interviewers were asked to type the responses into free-text fields in the software.
The open-ended questions were not asked of every interviewee. Only those who responded appropriately to a previous closed-type filtering question, thus indicating their willingness to contribute this sort of answer, were asked such questions. In all some 1,257 respondents were identified as having been asked some of these open-ended questions. Eight such questions were selected for further analysis, yielding a total of 1,456 responses. The number of responses to each question ranged from 52 to 361. None of the respondents provided an answer to all 8 of these particular questions, a few answered 7 of them, and some were not recorded as answering any of them.
The length of the recorded responses varied from a couple of words to about 145 words, although most were a single short sentence. The mean length was 66 characters, or about 15 words.
Socio-demographic data was used from the quantitative dataset that had been set up in SPSS. Each case was identifiable using a 5 digit serial number.
Supplementary observations
It is apparent from the data collected that the interviewers’ typing skills varied considerably as some have frequent spelling errors. This is understandable since most survey interviews require single keystroke (often numeric) actions to take down the data. The skill required to obtain and carry-out a quantitative survey interview would not generally include typing verbatim speech in real time.
It is also apparent that some interviewers typed comments from their own point of view, sometimes even referencing themselves with phrases like "Interviewer note ...", also some typed an obvious précis of the response using third person pronouns.
If accuracy of data capture is a significant requirement of such face to face survey interview research then serious consideration should be given to making audio recordings of the actual responses and transcribing these separately.
Planning CAQDAS project work
This discussion is written in specific reference to the following versions of CAQDAS packages; ATLAS.ti version 6; MAXqda version 2007; NVivo version 8; and QDA Miner version 3.2. The information presented here is likely to be relevant to both earlier and later versions of these packages, and may also be useful as the basis of consideration for other CAQDAS packages.
In this section we discuss whether to structure open-ended survey data by creating a 'document per case or a document per question' for analysis in CAQDAS packages: ATLAS.ti, MAXqda, NVivo or QDA Miner. This is a preliminary aspect of preparing textual data derived from open-ended questions to surveys for analysis.
For further information on the specifics of data preparation of open-ended question data for each individual package, and other background information which contextualises this discussion, see the relevant section on this page.
A choice has to be made at some stage
When preparing data from open-ended survey questions for analysis in NVivo or Atlas.ti, a crucial initial decision is whether to organise material on the basis of a ‘document per question’ (all of the responses to one question in a single document) or a ‘document per respondent’ (a separate document for each respondent which includes their answers to all of the open-ended questions).
It is important in NVivo and Atlas.ti
The following paragraphs consider the advantages and disadvantages of each approach. Some points are more significant in relation to certain programs than others. NVivo and ATLAS.ti both require textual data to be held in documents, which effectively freeze the layout decision at the point of data preparation. This is why practical considerations of data handling have to be considered at the outset. Both MAXqda and QDA Miner both use a flexible database structure which allows data to be displayed in a variety of ways during analysis independently of the form in which it was imported. Therefore when using these programs the choice of how to read the texts is more flexible and possibly less consciously made.
Some may find it hard
The ‘document per respondent’ approach is likely to be the initial expectation of experienced qualitative researchers who are familiar with this way of analysing in-depth interview transcripts. It is often intuitive for qualitative researchers to think of the individual respondent or research participant as the basic unit of observation. In contrast, the ‘document per question’ approach may seem more natural to researchers with a quantitative background where a comparison across large numbers of cases according to the particular questions asked is often an important requirement. It is not meaningful to describe either approach as 'right' or 'wrong' but some mental adjustment may be needed for a researcher to be persuaded to use an unfamiliar or seemingly counter-intuitive layout because it is more efficient from a software point of view. Here we set out aspects for consideration so that informed decisions can be reached at an early stage of work.
Consider any existing formatting
An important factor to consider at the outset is the format in which the data is currently available to the researcher, because reorganising large quantities of data is likely to take considerable time and effort, as well as creating risks of error and data corruption. Ideally this issue would be considered before data is collected, so that subsequent processing tasks may be kept to a minimum. However if survey data have already been collected, and the responses to the open-ended questions have already been assembled in some particular form, then the first efforts should be directed to planning a way to use that form so far as possible.
A spectrum of situations in terms of data volume
The next consideration concerns the quantities of data to be analysed. It is possible to imagine a spectrum of situations between (say) semi-structured interviews using 20 questions with 25 respondents towards one end of the scale and two open-ended questions included within a survey of 5,000 respondents towards the other. The former situation is likely best handled on a ‘document per respondent’ basis, while the latter would almost certainly utilise two large documents with one for each question. The problem is deciding at what point along the spectrum the switch-over between approaches should be placed, and this is a matter of judgement. The more significant factor is likely to be the number of respondents, rather than the number of questions, and a limiting condition is likely to be the time it takes to process each document for packages like ATLAS.ti or NVivo, which require data to be held as documents. In database programs, such as MAXqda or QDA Miner, a more relevant factor may be the number of mouse clicks or keystrokes required to display each successive group of texts.
Example
To illustrate this point an experiment was carried out to test the extraction of texts on a ‘document per respondent’ basis from SPSS via a spreadsheet application (in this case, Microsoft Excel) into a word processing application (in this case, Microsoft Word) so that they could be saved for use in NVivo or ATLAS.ti. On average, two documents on the respondent basis could be created per minute. The full data set in this instance included 1,257 respondents and 8 questions so an extrapolation indicates that a minimum of 10 hours concentrated effort would be required to set up this data on the ‘document per respondent’ basis. The eight ‘document per question’ files were created from the same spreadsheet within less than 40 minutes in total. Beyond this there may be other time penalties with the ‘document per respondent’ approach when working within some CAQDAS packages, because operations that involve drawing data from multiple files for retrieval purposes may take an appreciable amount of time. Consider, for example, the time required for 1,257 separate files to be opened, read, and closed by the computer – even at the high speeds we expect of automated procedures. In the end this will be a judgement decision for each researcher to make but we would expect few to adopt the ‘document per respondent’ approach for open-ended survey data when the number of respondents is more than 100 times larger than the number of questions answered by each.
Research design and methodology
Leaving practical issues to one side for a moment, it seems sensible to examine this from a theoretical point of view. The project design and analytical approach should be considered. Here we focus on a key methodological issue involving this type of data integration. This discussion is concerned with open-ended questions which have been asked within a survey context, and that context may have created some framework for the respondents, whether the survey was carried out with telephone or face to face interviews, self-completion on paper or online. The responses to any one question should have quite a lot in common with each other, because the question has probably been asked in the same way for each respondent, it should have fallen at about the same stage in each questionnaire, coming after certain questions and before certain others. In a survey the respondents should exhibit a spread of characteristics, however, if they are to be representative of a population. Thus the analytic strategy is likely to be to read all of the responses to a single question, in order to identify similarities and differences amongst them. It would make less sense to read the responses to all of the questions for each respondent in turn – unless it is felt that those responses are strongly linked together. This appears to provide some inclination towards the ‘document per question’ solution.
Separate questions or a conversation?
However, there may be other factors which affect the decision. For example, open-ended questions may have been spread throughout a survey questionnaire with the intention of gaining richer insights to illustrate the quantitative data being collected by other, closed, questions. In other situations a block of open-ended questions may have been compiled, perhaps creating a more in-depth conversation episode, quite possibly at the end of a structured interview. It seems likely that the former situation would lend itself better to the ‘document per question’ style as the open-ended questions are being used to add context to individual survey answers. However, in the latter situation the ‘document per respondent’ style of analysis may be more appropriate as it will likely be useful to keep all of the open-ended questions together because they relate to one another and to the particular experiences and opinions of individual research respondents.
Paraphrase or a full transcription?
A practical problem of data-collection technology may also have a bearing on this decision, concerning the method by which the response is ‘captured’ or recorded. Where an open-ended question is included in a fairly standard quantitative interview, then it is likely that the interviewer will be expected to type the respondent’s answer into a free-text field in the database. However, where more emphasis is given to the open-ended elements of the study, an audio recording of the whole interview may be generated and subsequently fully transcribed. The former is likely to be represented by short statements and brief paraphrases of what the interviewer heard, the latter can be expected to yield richer and longer texts. Once again the former type of data would probably be more fruitfully analysed with a ‘document per question’ approach, and the latter might sometimes be better read the other way.
Relationship to other data
A final matter for consideration will be to look at the whole research project and to think about how the analysis of these open-ended questions fits into it. The advantages of organising data in ways that are compatible with other parts of a project will probably outweigh the difficulties of working in an unfamiliar layout, using a work-around, or using software in a sub-optimal way. Additional factors may come into play in a longitudinal study, if you want conduct within-case as well as between-case analysis, for example to track differences in one respondent over time as well as to consider the similarities and differences between individuals at any given time point. Alternatively, in a mixed-methods study you may have some material which is clearly ‘document per respondent’ oriented and other material which is better handled using the ‘document per question’ approach. In such situations, some ingenuity may be needed to provide links between the two.
Overall objectives
As with most decisions regarding the use of CAQDAS packages, it is important for researchers to make an informed decision with regards to individual processes, based on an appreciation of the most important factors for the project in any given situation. Either approach will necessitate a balance to be reached between the analytical needs of the project and the practical and technical efficiencies afforded by the use of particular software packages. Good planning and clarity of objectives should inform the decision-making process.
Practical issues in CAQDAS packages
It has been established that it is practically possible to analyse data that has been prepared in either of the two formats under discussion with the four programs reviewed in detail on this page. Detailed guidance on data preparation and analysis strategies for each of these programs can also be found on this page. The ‘document per respondent’ approach may be seen as the most intuitive and common method of operation for these programs amongst many qualitative researchers, and where necessary specific work-arounds have been established to demonstrate ways of working with a ‘document per question’ approach in each program. However in ATLAS.ti and NVivo there are consequences that may impinge upon the analysis whichever decision is made and these should perhaps also be taken into consideration before a final choice is made. In QDA Miner and MAXqda this decision is only required when the researcher begins to read the texts and start the analysis. For all of these programs a limited outline of the suggested procedures is shown below in order to inform the decision-making process.
Atlas.ti
In ATLAS.ti this issue is complicated. Variable information about respondents’ characteristics is usually stored in 'primary document families', which only work correctly if the 'document per respondent' approach has been adopted. In order to have some selective reporting options that use variable characteristics in the 'document per question' method, it is necessary to insert a structured alpha-numeric string next to each response and then apply autocode routines to allocate thematic-type codes to the texts associated with them. This work-around can work well, even for a dataset with a large number of respondents, but there is subsequently no practical way to extract, report or work with the responses for just a single respondent other than by running a text search for their unique ID and using it to call those responses to screen one at a time. It is probably not really practical to use a thematic code and autocode routine for each individual respondent when there are very many of them.
Furthermore, because ATLAS.ti stores the data outside the main project file (or, in ATLAS.ti terminology, the Hermeneutic Unit), having a very large number of separate files representing a very large number of respondents on a ‘document per respondent’ basis could give considerable problems of data management during the period of active analysis if the implications of the external storage of files are not fully understood.
In addition, either choice made for ATLAS.ti has a consequential inflexibility to add further data during the analysis phase of the project. If the 'document per question' approach has been adopted it will be found that the selection of characteristics to be coded-in can only be extended with considerable labour, while if the ‘document per respondent’ approach is used it will be difficult to add data for an additional question at a later stage. In summary, ATLAS.ti can be used to work in either way with this sort of data but there are some inflexibilities and disadvantages with each method.
NVivo
In NVivo, when using the ‘document per question’ approach, providing a unique identification string has been placed adjacent to each separate response, it is possible to use an autocoding procedure to link each response text to its appropriate respondent (or 'case' in NVivo terminology). It is then possible to extract all of the responses for any one case, thus creating the 'document per respondent' as a separate viewable item within the program. It is possible to allocate thematic/conceptual codes ('nodes' in NVivo terminology) to sections of text on screen within this view, thus the full functionality of the 'document per respondent' can be reproduced giving the analyst the possibility of both views. However, users may experience problems when they need to switch between the list of cases and the list of thematic codes in the Listview pane, so it would be more efficient to organise the data in documents that reflect the way it will be analysed in this package. Subsequently, by using the functionality of NVivo’s 'casebook' which can hold variable-type data about each respondent, it is possible to extract texts satisfying any combination of variables and thematic codes for all or selected questions. For detailed information on preparing open-ended survey data for analysis using NVivo.
QDA Miner
The other two programs considered here, QDA Miner and MAXqda, both use a fundamentally different approach for this type of data. These programs store each response to each question separately, and then present them to the analyst in any order or grouping requested, including sequenced by case or by question.
QDA Miner takes this slightly further than MAXqda in this respect. The main display screen only shows a single text at a time, i.e. one respondent’s answer to one question. However, it is easy to generate a Text retrieval report to show all of the responses for one question in a scrollable report window, which links interactively with the main data panel so that thematic coding can be carried out straightforwardly. So the 'document per question' view is quite simple.
However, it is not such a simple matter to extract or display all of the responses for one particular respondent in QDA Miner. It is possible to generate a Text retrieval report for the entire dataset, showing all responses for all respondents, which will be sorted into case number sequence and so, by scrolling this report, it is possible to view the full set of responses for any particular person grouped together. Alternatively, by selecting the required person in the Cases panel and then clicking on each question’s document tab in turn it is possible to view that person’s set of responses one by one. For detailed information on preparing open-ended survey data for analysis using QDA Miner.
MAXqda
MAXqda readily shows both the 'document per respondent' and 'document per question' formats within different browsers simultaneously. The data has to be prepared and imported into the program in a specific way but, when this has been done, it is straight-forward to 'activate' simultaneously all respondents for one question in order to create the desired display. The full set of responses to the activated question code will appear in the 'retrieved segments' panel as a single scrollable list, and when any item in this list is selected with a mouse-click the full set of responses made by that particular respondent are displayed in the Text browser panel above. It thus becomes an almost unconscious decision for the researcher as to which panel is used and in which sequence the texts are read and coded. It is possible to alternate between the two, but for practical reasons, and in the interests of consistency, the choice will inevitably have to be made one way or the other for specific analytic purposes. For detailed information on preparing open-ended survey data for analysis using MAXqda.
Conclusions
Whichever software package you choose to use, you will have to make a further choice at some stage as to whether you will read and analyse the texts in question order within respondent groups or in respondent order within question groups. The more factors you can consider as you make that decision, the fewer surprises you will get as you put it into practice.
For further information on the specifics of data preparation of open-ended question data for each individual package, and other background information which contextualises this discussion, see the relevant section on this page.In this section we discuss whether to structure open-ended survey data by creating a 'document per case or a document per question' for analysis in CAQDAS packages: ATLAS.ti, MAXqda, NVivo or QDA Miner. This is a preliminary aspect of preparing textual data derived from open-ended questions to surveys for analysis.
A choice has to be made at some stage
When preparing data from open-ended survey questions for analysis in NVivo or Atlas.ti, a crucial initial decision is whether to organise material on the basis of a ‘document per question’ (all of the responses to one question in a single document) or a ‘document per respondent’ (a separate document for each respondent which includes their answers to all of the open-ended questions).
It is important in NVivo and Atlas.ti
The following paragraphs consider the advantages and disadvantages of each approach. Some points are more significant in relation to certain programs than others. NVivo and ATLAS.ti both require textual data to be held in documents, which effectively freeze the layout decision at the point of data preparation. This is why practical considerations of data handling have to be considered at the outset. Both MAXqda and QDA Miner both use a flexible database structure which allows data to be displayed in a variety of ways during analysis independently of the form in which it was imported. Therefore when using these programs the choice of how to read the texts is more flexible and possibly less consciously made.
Some may find it hard
The ‘document per respondent’ approach is likely to be the initial expectation of experienced qualitative researchers who are familiar with this way of analysing in-depth interview transcripts. It is often intuitive for qualitative researchers to think of the individual respondent or research participant as the basic unit of observation. In contrast, the ‘document per question’ approach may seem more natural to researchers with a quantitative background where a comparison across large numbers of cases according to the particular questions asked is often an important requirement. It is not meaningful to describe either approach as 'right' or 'wrong' but some mental adjustment may be needed for a researcher to be persuaded to use an unfamiliar or seemingly counter-intuitive layout because it is more efficient from a software point of view. Here we set out aspects for consideration so that informed decisions can be reached at an early stage of work.
Consider any existing formatting
An important factor to consider at the outset is the format in which the data is currently available to the researcher, because reorganising large quantities of data is likely to take considerable time and effort, as well as creating risks of error and data corruption. Ideally this issue would be considered before data is collected, so that subsequent processing tasks may be kept to a minimum. However if survey data have already been collected, and the responses to the open-ended questions have already been assembled in some particular form, then the first efforts should be directed to planning a way to use that form so far as possible.
A spectrum of situations in terms of data volume
The next consideration concerns the quantities of data to be analysed. It is possible to imagine a spectrum of situations between (say) semi-structured interviews using 20 questions with 25 respondents towards one end of the scale and two open-ended questions included within a survey of 5,000 respondents towards the other. The former situation is likely best handled on a ‘document per respondent’ basis, while the latter would almost certainly utilise two large documents with one for each question. The problem is deciding at what point along the spectrum the switch-over between approaches should be placed, and this is a matter of judgement. The more significant factor is likely to be the number of respondents, rather than the number of questions, and a limiting condition is likely to be the time it takes to process each document for packages like ATLAS.ti or NVivo, which require data to be held as documents. In database programs, such as MAXqda or QDA Miner, a more relevant factor may be the number of mouse clicks or keystrokes required to display each successive group of texts.
Example
To illustrate this point an experiment was carried out to test the extraction of texts on a ‘document per respondent’ basis from SPSS via a spreadsheet application (in this case, Microsoft Excel) into a word processing application (in this case, Microsoft Word) so that they could be saved for use in NVivo or ATLAS.ti. On average, two documents on the respondent basis could be created per minute. The full data set in this instance included 1,257 respondents and 8 questions so an extrapolation indicates that a minimum of 10 hours concentrated effort would be required to set up this data on the ‘document per respondent’ basis. The eight ‘document per question’ files were created from the same spreadsheet within less than 40 minutes in total. Beyond this there may be other time penalties with the ‘document per respondent’ approach when working within some CAQDAS packages, because operations that involve drawing data from multiple files for retrieval purposes may take an appreciable amount of time. Consider, for example, the time required for 1,257 separate files to be opened, read, and closed by the computer – even at the high speeds we expect of automated procedures. In the end this will be a judgement decision for each researcher to make but we would expect few to adopt the ‘document per respondent’ approach for open-ended survey data when the number of respondents is more than 100 times larger than the number of questions answered by each.
Research design and methodology
Leaving practical issues to one side for a moment, it seems sensible to examine this from a theoretical point of view. The project design and analytical approach should be considered. Here we focus on a key methodological issue involving this type of data integration. This discussion is concerned with open-ended questions which have been asked within a survey context, and that context may have created some framework for the respondents, whether the survey was carried out with telephone or face to face interviews, self-completion on paper or online. The responses to any one question should have quite a lot in common with each other, because the question has probably been asked in the same way for each respondent, it should have fallen at about the same stage in each questionnaire, coming after certain questions and before certain others. In a survey the respondents should exhibit a spread of characteristics, however, if they are to be representative of a population. Thus the analytic strategy is likely to be to read all of the responses to a single question, in order to identify similarities and differences amongst them. It would make less sense to read the responses to all of the questions for each respondent in turn – unless it is felt that those responses are strongly linked together. This appears to provide some inclination towards the ‘document per question’ solution.
Separate questions or a conversation?
However, there may be other factors which affect the decision. For example, open-ended questions may have been spread throughout a survey questionnaire with the intention of gaining richer insights to illustrate the quantitative data being collected by other, closed, questions. In other situations a block of open-ended questions may have been compiled, perhaps creating a more in-depth conversation episode, quite possibly at the end of a structured interview. It seems likely that the former situation would lend itself better to the ‘document per question’ style as the open-ended questions are being used to add context to individual survey answers. However, in the latter situation the ‘document per respondent’ style of analysis may be more appropriate as it will likely be useful to keep all of the open-ended questions together because they relate to one another and to the particular experiences and opinions of individual research respondents.
Paraphrase or a full transcription?
A practical problem of data-collection technology may also have a bearing on this decision, concerning the method by which the response is ‘captured’ or recorded. Where an open-ended question is included in a fairly standard quantitative interview, then it is likely that the interviewer will be expected to type the respondent’s answer into a free-text field in the database. However, where more emphasis is given to the open-ended elements of the study, an audio recording of the whole interview may be generated and subsequently fully transcribed. The former is likely to be represented by short statements and brief paraphrases of what the interviewer heard, the latter can be expected to yield richer and longer texts. Once again the former type of data would probably be more fruitfully analysed with a ‘document per question’ approach, and the latter might sometimes be better read the other way.
Relationship to other data
A final matter for consideration will be to look at the whole research project and to think about how the analysis of these open-ended questions fits into it. The advantages of organising data in ways that are compatible with other parts of a project will probably outweigh the difficulties of working in an unfamiliar layout, using a work-around, or using software in a sub-optimal way. Additional factors may come into play in a longitudinal study, if you want conduct within-case as well as between-case analysis, for example to track differences in one respondent over time as well as to consider the similarities and differences between individuals at any given time point. Alternatively, in a mixed-methods study you may have some material which is clearly ‘document per respondent’ oriented and other material which is better handled using the ‘document per question’ approach. In such situations, some ingenuity may be needed to provide links between the two.
Overall objectives
As with most decisions regarding the use of CAQDAS packages, it is important for researchers to make an informed decision with regards to individual processes, based on an appreciation of the most important factors for the project in any given situation. Either approach will necessitate a balance to be reached between the analytical needs of the project and the practical and technical efficiencies afforded by the use of particular software packages. Good planning and clarity of objectives should inform the decision-making process.
Practical issues in CAQDAS packages
It has been established that it is practically possible to analyse data that has been prepared in either of the two formats under discussion with the four programs reviewed in detail on this page. Detailed guidance on data preparation and analysis strategies for each of these programs can also be found on this page. The ‘document per respondent’ approach may be seen as the most intuitive and common method of operation for these programs amongst many qualitative researchers, and where necessary specific work-arounds have been established to demonstrate ways of working with a ‘document per question’ approach in each program. However in ATLAS.ti and NVivo there are consequences that may impinge upon the analysis whichever decision is made and these should perhaps also be taken into consideration before a final choice is made. In QDA Miner and MAXqda this decision is only required when the researcher begins to read the texts and start the analysis. For all of these programs a limited outline of the suggested procedures is shown below in order to inform the decision-making process.
Atlas.ti
In ATLAS.ti this issue is complicated. Variable information about respondents’ characteristics is usually stored in 'primary document families', which only work correctly if the 'document per respondent' approach has been adopted. In order to have some selective reporting options that use variable characteristics in the 'document per question' method, it is necessary to insert a structured alpha-numeric string next to each response and then apply autocode routines to allocate thematic-type codes to the texts associated with them. This work-around can work well, even for a dataset with a large number of respondents, but there is subsequently no practical way to extract, report or work with the responses for just a single respondent other than by running a text search for their unique ID and using it to call those responses to screen one at a time. It is probably not really practical to use a thematic code and autocode routine for each individual respondent when there are very many of them.
Furthermore, because ATLAS.ti stores the data outside the main project file (or, in ATLAS.ti terminology, the Hermeneutic Unit), having a very large number of separate files representing a very large number of respondents on a ‘document per respondent’ basis could give considerable problems of data management during the period of active analysis if the implications of the external storage of files are not fully understood.
In addition, either choice made for ATLAS.ti has a consequential inflexibility to add further data during the analysis phase of the project. If the 'document per question' approach has been adopted it will be found that the selection of characteristics to be coded-in can only be extended with considerable labour, while if the ‘document per respondent’ approach is used it will be difficult to add data for an additional question at a later stage. In summary, ATLAS.ti can be used to work in either way with this sort of data but there are some inflexibilities and disadvantages with each method.
NVivo
In NVivo, when using the ‘document per question’ approach, providing a unique identification string has been placed adjacent to each separate response, it is possible to use an autocoding procedure to link each response text to its appropriate respondent (or 'case' in NVivo terminology). It is then possible to extract all of the responses for any one case, thus creating the 'document per respondent' as a separate viewable item within the program. It is possible to allocate thematic/conceptual codes ('nodes' in NVivo terminology) to sections of text on screen within this view, thus the full functionality of the 'document per respondent' can be reproduced giving the analyst the possibility of both views. However, users may experience problems when they need to switch between the list of cases and the list of thematic codes in the Listview pane, so it would be more efficient to organise the data in documents that reflect the way it will be analysed in this package. Subsequently, by using the functionality of NVivo’s 'casebook' which can hold variable-type data about each respondent, it is possible to extract texts satisfying any combination of variables and thematic codes for all or selected questions. For detailed information on preparing open-ended survey data for analysis using NVivo.
QDA Miner
The other two programs considered here, QDA Miner and MAXqda, both use a fundamentally different approach for this type of data. These programs store each response to each question separately, and then present them to the analyst in any order or grouping requested, including sequenced by case or by question.
QDA Miner takes this slightly further than MAXqda in this respect. The main display screen only shows a single text at a time, i.e. one respondent’s answer to one question. However, it is easy to generate a Text retrieval report to show all of the responses for one question in a scrollable report window, which links interactively with the main data panel so that thematic coding can be carried out straightforwardly. So the 'document per question' view is quite simple.
However, it is not such a simple matter to extract or display all of the responses for one particular respondent in QDA Miner. It is possible to generate a Text retrieval report for the entire dataset, showing all responses for all respondents, which will be sorted into case number sequence and so, by scrolling this report, it is possible to view the full set of responses for any particular person grouped together. Alternatively, by selecting the required person in the Cases panel and then clicking on each question’s document tab in turn it is possible to view that person’s set of responses one by one. For detailed information on preparing open-ended survey data for analysis using QDA Miner.
MAXqda
MAXqda readily shows both the 'document per respondent' and 'document per question' formats within different browsers simultaneously. The data has to be prepared and imported into the program in a specific way but, when this has been done, it is straight-forward to 'activate' simultaneously all respondents for one question in order to create the desired display. The full set of responses to the activated question code will appear in the 'retrieved segments' panel as a single scrollable list, and when any item in this list is selected with a mouse-click the full set of responses made by that particular respondent are displayed in the Text browser panel above. It thus becomes an almost unconscious decision for the researcher as to which panel is used and in which sequence the texts are read and coded. It is possible to alternate between the two, but for practical reasons, and in the interests of consistency, the choice will inevitably have to be made one way or the other for specific analytic purposes. For detailed information on preparing open-ended survey data for analysis using MAXqda.
Conclusions
Whichever software package you choose to use, you will have to make a further choice at some stage as to whether you will read and analyse the texts in question order within respondent groups or in respondent order within question groups. The more factors you can consider as you make that decision, the fewer surprises you will get as you put it into practice.
In this section we discuss the selection of appropriate quantitative variables from survey data for use in analysing open-ended questions in CAQDAS packages.
This page is written in the context of a discussion about using CAQDAS packages to analyse the responses to open-ended questions asked in a survey. Such data can take a wide variety of forms depending, amongst other things, on the mode of data collection, the design of the survey questionnaire, and the size of the sample drawn. In this discussion we are imagining situations similar to that shown in the examples in the 'analysing survey data' section of this website – i.e. there is a large number of respondents who have provided fairly short answers to a limited number of open questions. The texts are sufficiently ‘rich’ to justify a qualitative approach to the analysis, but there is also a sufficient number of them to justify looking for patterns and relationships according to some of the characteristics of the respondents and what they said. Thus decisions have to be made over which characteristics to use and how they should be structured to best effect.
Compromises needed
It is with considerable trepidation that we venture into the potential minefield of this topic. To some qualitative researchers the very word 'variable' is almost unmentionable, whilst most quantitative researchers will want to retain the possibility of using as many variables as possible. But, in the particular mixing of methods that is inherent in analysing responses to open-ended survey questions, compromises probably have to be made on both sides.
Sort texts by characteristics
The big advantage of analysing this data in a CAQDAS program is that access to the words used by the respondents is almost always quickly available. By including relevant attribute variables it should be possible to sort and group those words according to some characteristics of the respondents. It should always be remembered that CAQDAS programs are not statistical analysis programs, and so the range of mathematical functions available in them will generally be limited. There will be some occasions when it will make sense to export some of the coding information to a statistical program in order to carry out a sophisticated quantitative analysis. But the compromise in that situation is that contact with the full texts will probably be lost in the process.
Hidden costs of too much detail
The disadvantage of analysing this data in a CAQDAS program is that retaining the finest grain of detail for a lot of variables may impose hidden costs in the operation of that program. In particular, in some programs it is unlikely to be useful to retain all of the values of a scaled variable, such as age, and it may be better to recode that data into an ordinal variable based on a few (age) groups. The most common use of variables within CAQDAS is in cross-tabulation tables, generally linked to the underlying texts related to each cell in the table, so variables with a wide range of possible values will generate very large and unwieldy tables. Once the variable data has been brought into the CAQDAS environment it may be difficult to alter, and so any adjustments to make it more usable within CAQDAS need to be made before it is brought in.
Use theory in the choices
In common with most research analysis, the primary consideration of variables to include will be governed by existing theories about the topic. This may be seen as similar to the decision taken, when embarking on in-depth interviews, as to which socio-demographic characteristics should be noted for each respondent. The difference is that in the survey situation those data collection decisions may have been taken by other researchers and the analyst feels that they are presented with a ‘fait accompli’. However not everything has been fixed and the analyst still has considerable flexibility of judgement. As a practical step it is probably a good idea to separate the selection of characteristics from decisions about their form.
Selecting variables
Three groups to consider
Firstly, it is very likely that common basic data is going to be used, and age and gender fall into this group. Next a short list of frequently used variables may be drawn up for further consideration, this might include ethnicity, employment status, social class, educational background, religion, and marital status. It is likely that some of these will be rejected as not relevant to the topic. If you are not sure whether to include a particular item maybe you could ask yourself what you would do with the information should you find that respondents in different categories of that variable appeared to say different things about some analysis theme. Finally, you may need to use more imagination to identify less obvious potential factors that might have an influence on your topic. In our example about experiences of flooding we included data on advance warnings received by the respondents, how seriously they had been flooded, and how long they had lived at the affected property.
Use restraint
Whatever the temptation to include more and more variables of interest, we advise you to exercise restraint and limit the selection to the variables that you actually anticipate using meaningfully in the analysis. There will be time costs for you in preparing each variable’s data for inclusion, so the fewer you use the sooner you will be ready to start the analysis. You are unlikely to be able to see all of the variables on screen at the same time as the relevant texts, so the inclusion of speculative variables may obscure relationships in the data that should be more interesting to you.
Take care from the outset
You also need to consider the significance of your choice of CAQDAS program in which you will carry out the analysis. As explained in the detailed advice on data preparation for each program, there may be difficulties in some programs if you need to add an extra variable after you have begun the analysis, this is particularly true of ATLAS.ti. But in all of these programs it will involve a disproportionate amount of time and trouble to add one extra variable separately compared to the time and trouble of including it in the main data preparation phase.
Formatting variable data
Values and labels
In quantitative programs, like SPSS, data may have two aspects; a 'value' and a 'label'. This is because the program finds the values easier to process and humans find the labels easier to interpret. In CAQDAS packages typically only one aspect is used and, as more interpretation by humans is involved, this is generally the label. Thus where gender may be coded in a statistics program as 1=Male and 2=Female (where 1 and 2 are the values, 'Male' and 'Female' are the labels) in a CAQDAS program the abbreviations 'M' and 'F' may be the most appropriate form, being brief but easily understood. Brevity of the labels may be particularly important for users of MAXqda and NVivo because of the way those programs display the attributes table in a separate window, and also in ATLAS.ti because of the work-around that is necessary to get the data into that program. So it will generally be important that the data is formatted in a way that is easily understood by human readers, whilst at the same time being brief and unambiguous. This may be achieved by editing the data labels for the selected variables in SPSS before exporting the data to Microsoft Excel and saving the labels only.
Scaled variables
Some variables may have been collected as scales, for example age or a physical measurement such as height or weight, where any value is acceptable so long as it is within the expected range. CAQDAS programs have fewer capabilities of processing such data than statistics programs and so these may be recoded into ordinal variables (such as age in 10 year groups). There is a loss of detail and precision in such a transformation, and an aspect of arbitrariness in the selection of the group boundaries, but so long as the full detail is retained in the data version for the statistics program the possibility of using it in a subsequent statistical analysis is retained (for example if coding data is moved from CAQDAS to SPSS). In some circumstances it may be useful to have both the scale value and the ordinal groups, MAXqda, NVivo and QDA Miner can all report text retrievals based on a comparison like 'Age greater than 45', but you are more likely to be looking at cross-tabulations of thematic code frequencies against age-groups. Using the work-arounds suggested on this site for ATLAS.ti means that there is no practical way to utilise the scale values in that program. QDA Miner may be capable of making more use of the scaled values through its Simstat module.
Specific difficulties in ATLAS.ti
If the analysis is to be carried out in ATLAS.ti it will be necessary to ensure that there is no possibility of confusing values from two different variables. This is a consequence of the way that we recommend the data is auto-coded in ATLAS.ti. It is recommended that some text is included in time referents to remove any possible ambiguity. Thus in the example used to illustrate all of this material we used the prefixes 'Age' and 'Prp' in the group labels to distinguish length of life from length of residence.
Conclusion
It has to be acknowledged that it is very difficult to get the selection and format for all attribute variables right first time. Fortunately, most of the CAQDAS packages will allow you to change your mind and add further variables during the analysis. Where this is the case you need not agonise for too long over this initial choice. But where you cannot see a secure method of adding more attribute data then it is obviously important to get these decisions right. It is also helpful to save and document data at the intermediate steps as this will help you to rectify any omissions that you find you have made.
In this section we discuss why it may be very important to re-check your coding work.
Where it is planned to use coding frequency statistics, for example to report the percentage of respondents who referred to a particular topic, or to export code data to a statistical program like SPSS, it is important to apply procedures to check the accuracy of the coding process.
If coding has been done manually, that is by a human researcher reading each response before making a decision as to which codes are applied to it, then it is likely that the range of meanings to which each code is attached will have been extended as the work has proceeded. Thus there is a danger that a code was not applied to some texts that were read early in the process (because they seemed somehow to refer to something slightly different) but that code was applied to similar texts later in the process (because when more were found it became possible to rationalise their connection to the topic). Therefore multiple coding passes may be necessary to achieve consistent coding.
If coding has been done automatically, say by using text searches and autocoding routines, then there is a possibility that, say, positive and negative comments about some concept have been coded to the same theme although their meanings are completely opposite. Passages may have been coded together because they include certain key words but which actually have quite different meanings.
For these, and other, reasons it is recommended that two types of coding check are carried out before any statistical output of coding information is used. Consistency checks are required to confirm that all of the passages given any particular code have got sufficient meaning in common to justify being grouped together. Omission checks are required to minimise the risk of excluding a passage from coding data which should really have been included because of its similarity to the rest of that group of texts.
This page sets out examples of such procedures for each CAQDAS package reviewed here. These are not necessarily the only or even the best ways of checking your coding, because the potential range of coding methods and errors is very large, but these notes should help you to think about how best to confirm the accuracy of your coding data before you or others start to rely on it.
Consistency checks
Consistency checks are generally quite straightforward to set up in CAQDAS programs. All of these programs have facilities to report all of the passages that have been attached to a particular code. Coding reports are standard operations. You will need to decide whether to display them out on screen or use printouts, and this will be a matter of personal preference. However, it is worth pointing out that, as the original coding was almost certainly done on-screen, a change of mode to paper format may help to flag up subtle differences of meaning. What has to be borne in mind as you check for consistency is that you are looking for similarities and differences at the same time. You need to ask yourself two questions about each group of texts and about any borderline texts in particular:
- Are these passages sufficiently similar to justify treating them as equivalent to each other?
- Are there any differences here that indicate that some respondents had quite different ideas about this topic?
At the end of each code consistency check you should be in a position to write a concise definition of the meaning of that code in terms of the way you have interpreted each of the responses that you have grouped together with that code. If you cannot write such a definition then you probably have not got a code that can be used effectively or reported accurately in a statistical way.
Tip: You may also find it helpful at this point to make a note of key words and phrases that respondents have used in the coded passages, these will be the search terms for the omission checks to be carried out later.
Coding report commands
- ATLAS.ti: in the code manager window - highlight the code + select output / quotations for selected code(s) – send output to editor (on-screen checking) or printer (paper checking). The report displays the details of the code and texts.
- MAXqda: activate all of the texts in the relevant text group in the document system (right click on the parent level text and select "activate all texts") + activate the code in the code system. The relevant texts are displayed in the retrieved segments panel, and can be printed from there using the print icon in that panel.
- NVivo: either – open the nodes area from the navigation pane, navigate to the relevant tree node or free node section, expanding sub-nodes if necessary, and double click on the node / code required – all the relevant passages from all document sources will appear as references in the detail pane. Or – open the queries section, open a new / coding query, in the "simple" tab under "Search for content coded at:" highlight the node button, click on the select button, navigate to and highlight the required code and click OK, back on the coding query dialogue use the pull-down menu beside "in:" to pick "selected items", then click on the select button navigate to and tick the source document(s) required and click OK, and finally click "run". The output in the detail pane can be printed using the file / print command. Note that neither the displayed nor printed data shows the node/code name, so you will have to remember it or make a manual annotation.
- QDA Miner: from the top menu bar select "analyse / coding retrieval" + in the dialogue screen by "search in:" choose the document(s) required using the pull-down menu + by "codes:" choose the code required using the pull-down menu or the tree diagram and tick boxes + click on "search". Use the "multi-lines" tick box to display longer passages in full. The code name is shown on each detailed line. To repeat the exercise for other codes simply alternate the tabs between "search expression" and "search hits" and vary the code as required. Hard copy can be obtained by using the printer icon on the search hits page.
Omission checks
Omission checks are much more difficult to carry out, and in some CAQDAS packages potentially helpful routines are not obvious. The main problem is that these require looking for a negative, something that is not in a group where it should be. It is suggested that these are better carried out after consistency checks have been done, when the key words and phrases noted during those checks are available.
A basic approach, having selected a code to check, is to exclude all of the texts that have been linked already to that code and then search the remainder for words or phrases that might indicate a connection to that concept. This requires a combination of coding, filtering and text searching. The suggested procedures for each CAQDAS package reviewed here are set out in summary form below (full details may be found on the "qualitative analysis strategies" page for each program).
- ATLAS.ti: create a complex expression in the query tool to list all of the responses to one question which have not had the required code allocated to them. Then, either scan the output from that query for apparent omissions or save it as a new document, assign that new document to the project, run a variety of text searches on that document looking for words associated with the code of interest and investigate any positive results as likely omissions. Where errors are found they will have to be corrected in the original responses document. Finally, disconnect the temporary document from the project to prevent unnecessary clutter from accumulating.
- MAXqda: create a complex text retrieval expression to retrieve all of the responses to the question which have not had the required code allocated to them. These will be displayed in the retrieved segments window, where lexical searches and word frequency functions can be applied to them and, if errors are found, correcting codings can be applied directly.
- NVivo: create a complex compound query which combines a text search for key words associated with the code of interest with a coding query for that code, linked by the operator "AND NOT", in the relevant question document. All positive results from such a query should be investigated as potential errors. Re-run the query as necessary to check for different key words.
- QDA Miner: Use the text retrieval function to list all of the responses to one question and apply a code identifying that question to all of the hits. Then run a code retrieval to search in the question document for all of the instances where the question code "is not enclosing" the thematic code of interest. Either scan the search hits from this retrieval visually on screen or after printing it out, or activate WordStat from the hits page to run word frequency functions on that output.
It is in the iterative processes described above that the thematic codes, and their meaning in the context of the data being analysed, are refined. In this work the CAQDAS program should be used to do what computers do best: searching, counting, and grouping. It is the role of the analyst to do what humans do better than computers: deciding what is useful, important, or interesting to distinguish subtle differences of meaning between similar phrases. If the processes described here seem to be complicated, it is because the task is difficult. If the survey design has given respondents the opportunity to answer in their own terms then the analyst must anticipate receiving the full range of complexity in the language used. Each of these programs provides powerful assistance in that task, but to be used effectively some investment of time and effort will be required to develop the appropriate skills.
About the software packages
This section summarises the design features, software architecture and terminology of CAQDAS software as relevant to the analysis of open-ended questions from surveys.
Basic architecture and terminology
Here we describe some relevant features of four selected CAQDAS packages to create a context for understanding why the suggested approach to analysing data from open-ended questions asked in surveys differs across those packages.
This material should help to inform decisions where users have the opportunity to choose which one of these programs they will use in a particular project that involves a substantial element of open-ended survey questions. The particular packages examined here are ATLAS.ti 6, MAXQDA 2010, NVivo 8, and QDA Miner 3.2.
It is our opinion that responses to open-ended questions asked in surveys will often be analysed one question at a time (rather than one case at a time, as may be more common in qualitative work). This has significant implications for how the architecture of the software impacts on the analysis process. We also consider that in the survey situation the use of semi-automation tools, such as word frequency and autocoding routines, will be useful aids to the analysis.Detailed instructions for procedures and processes in each package can be found elsewhere on this page, these notes provide background material only. Emphasis is placed on the elements of each package that are considered most relevant to the analysis of open-ended survey questions. This is not an attempt to provide a comprehensive introduction to these programs. Each program has its own terminology, which we use here where relevant, so the tables below show where specific terms have equivalent meanings.
ATLAS.ti (v6)
Element | Altas.ti terminology |
Container for the analysis | Hermaneutic Unit (HU) |
Location of response data | External folder |
Unit of material displayed in main screen | Primary Document |
Process for introducing material to be analysed | Assign Documents |
Themes used to categorise data | Codes |
Passages of data to which codes are attached | Quotations |
Characteristics or descriptors of respondents | Primary Documents Families (or Codes) |
A unique feature of ATLAS.ti, in comparison with the three other packages reviewed here, is that the data materials are stored outside the project container or hermeneutic unit (HU). The HU stores links to the data files and uses these links to mark the boundaries of the quotations to which codes are attached. A consequence of this structure is that the data are not reorganised by the program when they are introduced (or assigned), so the layout and format in which the material has been prepared is the one that is viewed during the coding phase of the analysis. (This is in contrast with some of the other packages). It is important that the data files that have been assigned to an HU are not moved or altered in any way outside ATLAS.ti.
When the data are organised with all the responses to one question in a single primary document then word frequency, text search and autocoding tools can be applied effectively to that document. However, with this way of organising the data there is no way of extracting code frequencies at the level of cases after the initial analysis so it will not be practicable to export such data back to a statistics package for further analysis with the remaining quantitative data from the same survey.
With version 6.2 ATLAS.ti introduced a new data import routine specifically for survey data. This uses a different architecture, in particular it stores all of the responses inside the HU and it arranges them with a separate primary document for each case, with document families to store socio-demographic variables. Unfortunately this architecture cannot at present be used to apply the automation tools (word frequency etc) to the responses for a single question, so the coding analysis may have to be done entirely manually. On the other hand, this architecture does facilitate the eventual export of code frequencies at the case level for further statistical analysis. It thus follows that an early decision is needed between the different approaches to open ended questions in ATLAS.ti v6.2.
MAXqda 2010
Element | MAXqda terminology |
Container for the data and analysis | Project |
Individual respondent | Textname |
Unit of material displayed in main screen | Text |
Process for introducing material to be analysed | Import Texts |
Themes used to categorise data | Codes |
Passages of data to which codes are attached | Segments |
Characteristics or descriptors of respondents | Attributes/Variables |
MAXqda stores text data, such as open-ended survey question responses, in the same project file as the codes and analysis materials. Provided certain data preparation steps are followed, it can use a relational database to store each response to each question separately. It is then possible to display the responses by one case to all of the open-ended questions in the text browser panel, at the same time as displaying the responses to one question by all of the cases in the retrieved segments panel. This gives the analyst complete flexibility to read the data in whichever way they like.
The word frequency function is included in the MAXDictio module, which is included in the MAXqda+ version of the software, so it is not part of the basic program package. When this has been purchased it integrates effectively with the main program so that text searches and autocoding routines can be repeated easily to generate codings quickly.
Socio-demographic or variable data can be imported from a spreadsheet and associated with the cases. This can then be displayed in a separate window, interactively linked to the main text browser, or used in crosstabulations with code frequencies.
Outputs of coding frequencies per case or per question can be generated and exported to MS Excel, facilitating reporting and re-use in statistical packages.
NVivo (v8)
Element | Altas.ti terminology |
Container for the data and analysis | Project |
Individual respondent | Case |
Unit of material displayed in main screen | Source (generally an Internal Source) |
Process for introducing material to be analysed | Import Sources |
Themes used to categorise data | Nodes (Free Nodes or Tree Nodes) |
Passages of data to which codes are attached | References |
Characteristics or descriptors of respondents | Attributes (held in the Casebook) |
NVivo stores text data, such as open-ended survey question responses, in the same project file as the codes and analysis materials. It is essentially a document based system, so when a source is opened for reading and analysing it appears in the same format and layout as that of the document from which it was imported. This means that in order to analyse all of the responses to one question systematically it is necessary to organise those responses in a single document source.
Provided certain preparations are carried out in setting up the source documents using respondent identifiers and specific heading levels, it is possible to link each response to its appropriate case. It is then possible to import a set of socio-demographic attribute data for each case and store it in the casebook. The casebook can be displayed in a separate window beside the main analysis in the detailed view pane, but these panels do not link interactively and have to be scrolled separately in order to relate a specific response to the attributes of that respondent. The case identifiers can be used in a query to display all of the responses from a single case.
When using a separate document for each question’s responses the word frequency, text searching and autocoding tools can be applied effectively to one question at a time.
Outputs of coding frequencies per case or per question can be generated and exported to MS Excel, facilitating reporting and re-use in statistical packages.
With version 9 of NVivo (released in 2010) a new survey import function was added. This imports data from a spreadsheet layout and reproduces that layout in a single source document within NVivo where it can be analysed. At the time of writing it appears that the word frequency, text search and autocode functions can only work on all of the texts within this source, so it does not appear possible to use these effectively when a separate analysis of each question is required.
QDA Miner
Element | Altas.ti terminology |
Container for the data and analysis | Project |
Individual repsondent | Case |
Unit of material displayed in main screen | Intersection of selected Case and Document |
Process for introducing material to be analysed | Import or Append |
Themes used to categorise data | Codes & Categories |
Passages of data to which codes are attached | Text Segments |
Characteristics or descriptors of respondents | Variables |
QDA Miner uses a relational database structure to store the response texts and socio-demographic attribute variable data within a set of integrated project files. The data can be imported from a single spreadsheet file or from a variety of other sources. Within the project the main screen only displays a single response at a time, i.e. the answer from one case to one question. However a simple retrieval tool can be used to generate a display of all the responses to one question, or the responses by all cases to all questions sorted in case number order, so that there is full flexibility to read the data by case or by question. The socio-demographic variable data for the case being read are displayed within the main screen by default and so is fully integrated.
Whilst some text search, thesaurus, and autocoding tools are available within the basic QDA Miner program, the separate module called "WordStat" contains a suite of sophisticated content analysis tools, of which word frequency calculation is one of the simplest. A further module called "SimStat" is a powerful statistical package in its own right, so it may not be necessary to export code frequency data for further quantitative analysis, however that option is also available if required.
QDA Miner is capable of handling very large volumes of data, ie particularly large numbers of cases in a survey, with fast processing speeds.
Summary
These four packages have a considerable amount in common. They were all designed to assist with the core tasks of working with qualitative data: applying thematic codes to sections of data and facilitating the identification of meanings and patterns in that data. The differences between them are minor in comparison to that common core. However there are differences, and these may have a bearing on which package is best suited to certain situations in particular data. Here we focus on the implications for dealing with open-ended questions asked in surveys.
If you have an extremely large number of cases or respondents, say many thousands, then QDA Miner is likely to be the only package to work efficiently. If you have a large number of cases, say several hundred or a few thousand, then MAXqda and NVivo will be able to handle the data almost as well as QDA Miner and may have other features that are useful. ATLAS.ti may prove difficult to use with large volumes of cases, in part because of its method of storing primary data outside its hermaneutic unit, and in part because of its method of handling the variable characteristics of respondents.
If your data are particularly ‘rich’, with thoughtful responses fully transcribed, possibly closer to a semi-structured interview, then ATLAS.ti can be more effective with its tools to assist inductive reasoning. NVivo also has modelling tools but does not keep the user quite as close to the data. However, it is likely that data originating in a survey situation may be formed of shorter statements, with fewer possibilities for considering subtle nuances of meaning, but with more likely requirements to generate quantifying summaries, and for these situations MAXqda, NVivo and QDA Miner have more useful functions.
The biggest differences between these packages lie in the formats in which they accept and store the data. ATLAS.ti and NVivo take the data in documents, whereas MAXqda and QDA Miner take each separate question response as a data unit. There may be considerable differences in the effort required to prepare the data before introducing it to your chosen CAQDAS program, depending on the form it takes at the start of the process and the program chosen to use with it. That is why we have included detailed guidance on these processes below.
We would like to emphasise that, whichever software is available to you, it can be used to analyse open-ended survey question data. So, if you are already familiar with one of these programs, then that would probably be the easiest one for you to use for a one-off project. However, if you are likely to analyse open ended questions on a regular basis, then it would be worth studying all of the materials in this section of our website to help you choose the program that will be most helpful in the long run.
In this section you will find a summary comparison of ATLAS.ti, MAXqda, NVivo and QDA Miner in the context of the analysis of open-ended questions from surveys. We draw some comparisons between the four selected CAQDAS packages as an aid for users who may be considering which program to use for analysing the responses to open-ended survey questions.
There is no question of identifying which package is the ‘best’ because they each have different strengths and weaknesses, which may interact differently with the very wide range of circumstances that may be covered by the description "open-ended survey questions". In view of the time that it can take to become familiar with a new software program, we would suggest that if you have been using one of these packages already then that would probably be the best one for you to consider first. All four programs have been used successfully with the trial data so there are few critical weaknesses.
The particular packages examined here are ATLAS.ti 6, MAXqda 2010, NVivo 8, and QDA Miner 3.2. Of these only QDA Miner could be described as having been designed for this particular application. The other three programs were all designed initially for mainstream qualitative data analysis, generally involving a moderate number of lengthy text documents. Here we are considering the problems arising from analysing a large number of short texts, which are typical with open ended questions.
In our view, a key advantage of using a CAQDAS program to analyse this sort of data is the availability of semi-automation tools, such as word frequency counts, text searches, and autocoding. These tools can be combined in an inductive approach to derive concepts from the words used in the responses and identify accurately the cases that have used those concepts. Further benefit may be gained when data about the respondents, such as socio-demographic and other relevant variables, is combined with the thematic codes to reveal potential patterns of co-occurrence. But the real power of CAQDAS programs is the facility to keep close to the words recorded in the data capture process at all stages of the analysis, so that emerging ideas of possible relationships in the data can be tested against readings of the language used. All of these features are combined in an iterative process where the analyst uses a variety of program routines all guided by judgment decisions and interpretations based on skill and experience. Thus there is no single procedural path of steps to follow to achieve a ‘correct’ analysis, and it follows that differing styles of working practice between individual analysts will integrate differently with the programs reviewed here.
Presentation of the data and ease of reading the texts
As mentioned previously, a key analytical decision with open ended survey question data is whether to read and code the responses on a case by case or question by question basis (see above). The case by case approach is central to qualitative analytical work and so all of the programs handle that efficiently. But in survey situations it will often be more useful to analyse all of the responses to a single question together in order to make effective comparisons between them, and for this approach the packages have different strengths and weaknesses.
In this respect MAXqda has a clear advantage over the other three programs as it can display all of the responses to one question in one part of its main window and all of the responses by one case in another part of the window, with both panels linked interactively so that selecting a response in the question part causes the same response to be shown in the case part within the context of the other answers by that case. QDA Miner is almost as flexible but the user has to configure text search routines to generate the required lists within a separate output window, which is then linked interactively with the data appearing in the main window. NVivo and ATLAS.ti are both less flexible in this respect as these programs have a different architecture and the data needs to be organised into documents whose presentation style is then used unaltered when those documents are read within the software.
As the analysis proceeds and codes are attached to the responses to signify the presence of specific concepts in the texts, it becomes important to be able to see where codes have or have not been applied. All of the programs use coloured coding markers in a margin, but NVivo is less flexible than the other three programs, in that program the user has no control over the colour for any particular code, and the user has to specify which coding stripes are to be displayed at any one time. In ATLAS.ti, MAXQDA and QDA Miner it is possible to define a separate colour for each code as it is created (or common colours for different groups of codes), and when the response texts are displayed in the main working window all the codes that have been applied to any paragraph are shown by default. (NVivo v9 has added some user controlled colour functionality for codes).
Use of semi-automation tools
In most qualitative analysis work it would be normal practice for codes to be applied entirely under the manual control of the analyst, often by using a combination of mouse highlighting and clicking to select carefully identified passages of text and then to apply one or more codes to that marked segment. All of these programs handle that way of working with similar efficiency. But, as commented above, in the survey situation it can be advantageous to apply some codes automatically on the basis of the presence of specific words or phrases. We have identified three basic steps where computer power can be harnessed by the analyst to speed up this process for open ended question data.
All four programs have a word frequency function, however in MAXqda and QDA Miner this is only available through an additional module of the package (MAXDictio in MAXqda+, and WordStat with QDA Miner). In ATLAS.ti and NVivo the word frequency (and subsequent text searching) tool can only be restricted to the responses of a single question if those responses have been imported or assigned to the project in a single document, without any other question responses in the same document. QDA Miner’s WordStat module also includes a "phrase finder" which searches for and counts groups of words occurring together in the data, and this can be very effective with open ended question responses. It is often useful to be able to exclude trivial or unhelpful words through the application of a ‘stop-list’. NVivo has a fixed list of words to exclude for each language (which can be effectively turned off by setting the language to ‘none’), whereas the other three programs all allow users to create their own stopword lists, if required, separately from a default list.
When a word has been identified as occurring frequently in the responses, and thus may be an indicator of a common theme or concept, it will be necessary to read a sample of the those instances in order to judge whether it has been used with sufficient consistency to be the basis for a code. QDA Miner in its WordStat module has a "keyword in context" (or KWIC) function which not only lists all of the instances but can also be sorted alphabetically according to either the word preceding the key word or the word following it and this can be very useful with open ended question responses. MAXqda has very good integration of the word frequency and text searching functions, using separate windows for each which can be kept open and re-used easily, but each instance is displayed separately in its full context in the main window. In NVivo it is possible to view all of the instances in which a word has been used as a list derived by selecting that word from the word frequency report, but that list only shows a fixed number of words before and after the key word, although it can be linked interactively with the source data via a context menu, which makes this slightly less useful. ATLAS.ti does not integrate its word frequency tool with other functions, so separate text searches have to be run to explore the usage of any particular word in the data.
The third semi-automation tool is autocoding. By this we mean using a single command in the program to attach a particular code to all of the responses that match some specified criterion, most often those that include a specified word. This is most useful when it can be done in conjunction with a text search so that the analyst has the opportunity to check at least some of the selected responses immediately prior to the coding process. ATLAS.ti does not integrate the text search and autocode routine, but does allow the autocode to be run with user intervention at each ‘hit’ (to select code or skip). NVivo uses different options within a saved query to show a ‘preview’ list of search finds or to code all of the search finds, but this makes it difficult to exclude a small number of unwanted ‘hits’. MAXqda and QDA Miner both make it possible for the analyst to exclude the unwanted ‘hits’ at the viewing stage and then to apply the code to all of the remaining ‘hits’.
A further aspect of the autocoding process is the decision as to how much text to code in the automation process. In MAXqda and QDA Miner, the two relational database programs, the option to code the whole paragraph should generally capture the whole of each relevant response, with the identity of the respondent being always attached to any paragraph. In ATLAS.ti, provided the data has been prepared in the way we suggest, the option to code until the next "multi hard return” effectively captures the whole response and the case identity. We have found it more difficult to find a combination of data preparation and query parameters to achieve this in NVivo, although the paragraph setting in the query does capture all the response text and the matrix coding queries can relate the thematic codes to the attributes in the casebook after autocoding by paragraph, it is just harder to locate a specific hit manually in the source document.
In some ways the analysis of open ended questions may be seen as akin to content analysis, and there are more sophisticated procedures and tools available in that approach. QDA Miner with its WordStat module has a considerable array of further tools that are not matched in the other three programs reviewed here. However, MAXqda with its MAXDictio module does have a dictionary function that may be roughly equivalent to the thesaurus function in the main QDA Miner program. These facilitate analysis by allowing users to build up sets of words that are likely to signify the presence of identified thematic concepts in response texts. This approach is laborious at first but is very powerful and effective if a similar analysis has to be repeated on different data, such as repeated surveys.
Looking for patterns amongst thematic codes and personal attributes
Although originally most CAQDAS programs were not developed with quantitative analysis techniques in mind, because generally the necessary conditions of sample size and randomness for quantitative abstraction are not found in qualitative research, some matrix tables are now available and their use can be justified in the open ended question situation where the remainder of the survey is quantitative.
It is anticipated that a common output required from such analysis will be a table of the frequencies with which the thematic codes have been applied to the responses to certain questions. With the data preparation strategies recommended in this section of this website, we have been able to generate these in report format and as a spreadsheet export in all four programs.
Another possible output is the export of thematic code frequencies at the case level (i.e. separately for each case) so that these can be imported into a more sophisticated statistics package for further analysis in conjunction with other data collected in the survey. This can be achieved effectively in QDA Miner, MAXqda and NVivo (in increasing order of processing time when the number of respondents is large), but is not possible in ATLAS.ti when our suggested method of data preparation has been used.
We have identified effective ways of incorporating personal attributes, such as socio-demographic data, into the project datasets in the four CAQDAS programs, and these can be used effectively to create crosstabulation tables of thematic codes against the set of values for any one attribute in all of these programs. For ATLAS.ti this involves a fairly extensive workaround at the data preparation stage, while the other three programs can all import such data directly from a spreadsheet layout. All of the programs have facilities to extract all of the segments in the response texts that match a selected combination of theme and attribute value, and this should assist in the interpretation of any quantitative patterns that may be observed in the tables.
Ease of use
This sort of comparison is quite subjective and opinions may vary on the comments below. However, it is important to make some effort to consider this aspect, because of the iterative approach which seems to be necessary with this sort of data. To analyse open ended questions effectively will require the use of several different tools in the chosen software, the application of human judgment, and the development of skills to move rapidly between these.
We have found NVivo to be quite laborious because of the extensive use of dialog screens and the need to save exploratory queries; it can do all of the tasks we require but it does take a lot of user effort to work out how to achieve the desired effects. ATLAS.ti can also require an investment of time to learn, and it is not ideally suited to the quantitative aspects of these tasks, but its large area of working screen is useful when the analysis has to be done by reading and manual coding. MAXqda is probably the easiest program to learn, of the four reviewed here, and many of its functions seem to operate as one intuitively expects as well as integrating well with each other. QDA Miner is the most sophisticated and the most powerful when the number of cases is very large, (but it is considerably more expensive than the others) however the sheer range of options and facilities can be daunting for the novice user so it does require some investment of time and effort to identify what is useful and meaningful in relation to your particular data.
Further developments
Subsequent to much of the work on which these web pages are based, NVivo and ATLAS.ti introduced new versions of their software with special "survey import” functions added. These have not been included in the comments above, or generally in other sections of this website. Our preliminary review of both of these new routines indicates that they are only marginally helpful, because in both programs the data that is imported with the new routine is not readily available for analysis with the aid of the semi automation tools on the basis of one question at a time. The new routine in ATLAS.ti v6.2 does create a project file from which one can output the code frequencies on a case by case basis (the one expected output that was not possible with the way we have recommended preparing the data for earlier versions of ATLAS.ti), but before that stage is reached the user will either have to apply all codes manually, or separate each question’s responses into a separate hermeneutic unit if autocoding is to be used while keeping each question distinct. There is a similar problem in NVivo v9, as the data imported with its survey import routine is treated as a single source. The potential workaround for both new routines of using separate projects for each question will probably work satisfactorily at one level but may cause understanding of the broader phenomena being studied to become fragmented and so be less effective.
Data preparation instructions for analysing open-ended survey questions
The following material provides step-by-step guidance for preparing the texts, collected from a large number of respondents answering several open-ended questions in a survey situation, for qualitative analysis using MAXqda software. |
There are several stages in this procedure, some of which may not be relevant in your circumstances. Some users may have alternative methods or short-cuts, in which case please use your own judgment as to which elements from below to apply. Our purpose here is to provide a comprehensive guide, which has been tested and proved to work, for the benefit of those who have not achieved this task successfully before.
Unlike some other CAQDAS packages, MAXqda includes specific routines for working with this sort of data and so the steps outlined below do not represent a workaround but a mainstream activity.
1. Create a spreadsheet containing all of the survey texts and respondent identifiers
This step is illustrated here using Microsoft Excel, however it is likely that almost any spreadsheet program could be used for these tasks as no specialised functions are required.
The recommended structure to aim for is one in which you arrange the data in a table, or grid, with a separate row for each respondent (or case). The columns should separately hold both the unique identifier for each respondent and the texts of the answers to the open ended questions.
TipBefore carrying out any data processing, try to examine data in their most original format in order to identify some of the longest individual responses and to look for unusual characters in the texts. The longer responses may get truncated in some conversion processes (for example on being brought into SPSS if the 256 character default was not changed) and it is useful to be able to check that you have the fullest versions of all responses before you carry out the analysis work. Unusual characters, other than basic alpha-numeric and punctuation ones, sometimes affect conversion processes so it is a good idea to check that these have been copied faithfully before doing analysis work. |
MAXqda has specific requirements for the first row and the first two columns of the table. The first row must contain the unique identification names for the separate questions, these will appear in the code section of the program and should be short and unambiguous. The first column must contain the name of the "textgroup" that will become a container for all of the respondents in MAXqda, and the second column must contain the unique identifiers for the respondents. This is illustrated in Figure 1 below.
Figure 1: Spreadsheet of survey texts prepared for MAXqda
Please note the following in Figure 1:
- Cell A1 must contain the word "textgroup", and cell B1 must contain "textname". The strings in C1, D1 etc are the names of the separate questions in the survey.
- All the rest of the cells in column A have the word "survey", this is the group name under which all of the respondents will appear. You may choose any word here, the important point is that the same word must be used throughout the column.
- Column B contains the unique identifier for each respondent. This has been formatted as the survey serial number prefixed with "R". There are many acceptable formats for this but it is recommended that a consistent length and layout is used. The letter prefix causes the spreadsheet program to interpret each item as a text string instead of a number. MAXqda accepts purely numerical identifiers, so the "R" prefix is not strictly necessary. It is essential, however, that each respondent has a unique identifier and serial numbers are probably more reliable for this purpose.
- In columns C and D the format has been set to word wrap and auto-fit row-height so that the longer responses can still be seen. Before proceeding any further, you should check that any particularly long responses are included in full.
- It is not necessary for there to be a response text in every cell in the table, for example in this illustration respondent R.26222 did not answer question QBETT2.
- The inadvertent inclusion of a blank row or column in this table does not appear to cause a problem when the data is imported into MAXqda.
After preparing this table, save it in spreadsheet format as a back up and in case further editing may be necessary.
TipWe have found it difficult at a later stage to add a further set of question texts to an existing MAXqda project, and so we recommend that you take care in this first step to include all of the response materials that you will need to analyse in this project. |
2. Create another spreadsheet with socio-demographic attribute variables and respondent identifiers
The second table is similar to the first in some respects but contains the attributes of the respondents which may be relevant to the subsequent analysis.
TipIt is quite possible to add further attributes for all respondents during the analysis, so the decision over which attributes to include at this point is not an irrevocable one with MAXqda. However it is worth making sure that you use all of the data that you can reasonably expect to consider during the analysis, as it is easier to incorporate it at this stage. |
For further comments and guidance on selecting variables to use in such analysis please see the notes above.
Figure 2: Spreadsheet of attribute data prepared for MAXqda
Please note the following in Figure 2:
- Columns A and B should be identical to those set up for the texts in Figure 1.
- The first row should contain the names of the attributes.
- There should be data in every cell of this table. When this table is imported into MAXqda any blank rows or columns will be ignored, however an empty cell will remain blank and may not be recognised as missing data in subsequent analysis reports, so it may be best to enter a specific missing data label in such cells.
TipIt may be advisable to do some work to recode scale variables into ordinal groups, as shown for the age variable above. Some routines within MAXqda will work with a numerically scaled variable but these are more likely to be used for filtering data or cross-tabulations than in regression calculations. |
After preparing this table, save it in spreadsheet format as a backup and in case further editing may be necessary.
3. Save both spreadsheets as tab-delimited text files
Each of the spreadsheets created in steps 1 and 2 needs to be saved as a separate tab-delimited text file for the import into MAXqda. If you have saved both tables as worksheets within a single Microsoft Excel workbook you will need to copy each to a new workbook and then save those separately. (In Microsoft Excel you can easily copy a worksheet by right-clicking on the name tab at the bottom of the screen, selecting the move or copy command in the menu that this brings up, clicking a tick into the create a copy box, and selecting "new book" from the pull-down list at "to book": – see figure 3.
Figure 3: Copy worksheet to a new file in Microsoft Excel
Use the "save as" command with the field "save as type" set to "text (tab delimited)" and select a file name and storage location which you will be able to access from MAXqda at the import stage (see Figure 4).
Figure 4: Save As command in Microsoft Excel
4. Import the survey text file into MAXqda using a designated routine
Open a new project in MAXqda. From the menu in the top bar select "text / import structured text" from Microsoft Excel. You will be presented with an information screen which tells you how to structure the Microsoft Excel spreadsheet, and the instructions above should prove to be consistent with these, so press OK to continue. The next dialogue prompts you to navigate to the location where you stored the text file created at step 3 above (the file with the response texts is the one you need at this point), and when you have selected the correct file click on "open".
The next dialogue asks if you want to ignore empty cells, but gives no clue over the significance of this decision, we have obtained satisfactory results by clicking "yes" at this point.
TipTesting our trial data with both answers to this dialogue has revealed the effects. If you answer “Yes” to ignore empty cells the import process will be quicker because a “No” answer means that the program creates a coded segment for every cell in the spreadsheet grid whether it has data in it or not. This will also affect the coding and analysis stages by clogging your screen with empty segments. |
The import process may seem to be slower in MAXqda than in other CAQDAS programs, but a lot of work is being done by the computer where the equivalent has to be done manually in ATLAS.ti or NVivo. In our example a data file with 1,257 respondents and 8 questions took around 15 minutes to process on a fairly high specification PC (current models will be faster). It is apparent how the process slows down as it builds the database because the textnames are displayed in the document system panel as they are completed. The number displayed in the top right corner of the document system panel is the total number of coded segments in the system. In Figure 5 this can be seen to be 1,456, and this represents the total number of separate question responses made in this dataset. The highlighted respondent, R.97117, answered two questions (shown by the "2" in that column) whereas case R.97116 did not answer any of these questions. Each respondent’s response to each question represents one segment. The same total appears in the code system panel below, where the segments are sorted and coded according to the question names from the top row of the spreadsheet – for example in Figure 5 the question QBLO2 was answered by 87 respondents.
Figure 5: MAXqda immediately after importing a set of Survey texts
5. Import the attributes file into MAXqda using a designated routine
The process to import the attributes is started from the main menu by selecting attributes / import. The dialogue screen requires you to navigate to and select the tab-delimited text file with the attribute variables that you saved in step 3 above. Having done that, click on "open" and the process will run.
TipThis procedure is much faster than the text import, if you watch closely you should observe a counter in the middle of the screen clocking up to the total number of respondents. |
To confirm that the data has been imported you should open the attributes table. The command attributes / edit shows an icon which is also located on the top toolbar (the grid symbol just beneath the attributes command in Figure 5 above), use either the full command or the toolbar button to open the attributes table. The table may need some editing and arranging to obtain a satisfactory display. It is shown in a separate window which can be moved and resized to suit your preferences, and you can choose which variables are to be visible. Locate the button in the attributes window which toggles between variable view and data view (the third from the left in the attributes table view in Figure 6 below). In variable view tick just the variables that you want to see displayed and untick the ones that you want hidden, then switch back to data view to see the effect. You can also adjust the width of any column by dragging its border in the title bar.
Figure 6: MAXqda with attributes table open in data view
In Figure 6, note that all of the survey text group documents have been activated. When activated, the texts are linked interactively to the data in the attributes window. Selecting a respondent in the document system (R.97117 in this illustration) causes the attributes for that respondent to be highlighted in yellow in the attributes window.
6. Verify the accuracy of the data transfers
The first checks to confirm that the data has been imported correctly are those that confirm the total number of texts and respondents. In Figure 6 above, note that in the top right corner of the attributes window "1257 texts" is displayed. This is confirmation of the number of respondents whose attributes have been imported. When you activate all of the texts in the group (by right clicking on the group header, "survey", and selecting activate all texts the number beside the first icon in the extreme bottom left corner of the screen shows the total number of texts (i.e. respondents) in the group (also 1257 in this example). These are two indications of successful data imports.
Next, you should check that the longest response texts which you identified in the first step above have been copied successfully. Move the attributes window to one side so that you can see the text browser and retrieved segments panels. Deactivate all of the texts if they are currently activated, and activate all of the question codes in the code system panel instead. Now, when you activate a single text you will be able to see all of that informant’s responses in the retrieved segments panel. Use this method to call to screen the longest individual response texts and confirm that they are complete.
Finally, you may check the import of attributes by scrolling through the attributes window quickly, looking for obvious gaps, blanks, or out of pattern cells.
The following material provides step-by-step guidance for preparing the texts, collected from a large number of respondents answering several open-ended questions in a survey situation, for qualitative analysis using NVivo software. |
These instructions assume that the decision has been made to organise these texts into a separate document for each question, with many respondents included in each document. (For a discussion of the arguments for and against such a decision please see the 'document per case' vs 'document per question' section above). This way of organising the data is not the mainstream procedure in NVivo and so these instructions may be seen as a workaround to achieve a satisfactory basis for analysis.
There are many stages in this procedure, some of which may not be relevant in certain circumstances. Some users may have alternative methods or short-cuts, in which case please use your own judgment as to which elements from these instructions to apply. Our purpose here is to provide a comprehensive guide, which has been tested and proved to work, for the benefit of those who have not achieved this task successfully before. These instructions refer to Microsoft Excel and Microsoft Word and are illustrated with screenshots from those programs, however the operations involved are not particularly sophisticated and any equivalent spreadsheet and word-processing programs would probably be usable as alternatives.
TipIf you have several batches of response texts to analyse it will be most efficient to repeat each stage for all the batches before moving on to the next step. However, it may also be a good idea to take the first batch right through the whole process to make sure that it is working correctly with your data and software versions before committing the time to process all batches. |
1. Locate the set of response texts and a unique identifier (ID) for each respondent and copy them into Microsoft Excel
This step may not be necessary if the response texts are already organised in a Microsoft Word document with appropriate identifiers for each respondent, in which case please skip to step 3 and continue from there. If, on the other hand, the texts are being extracted from an SPSS data file or some other database format then this step can be a useful means of organising the data in the first instance.
TipBefore carrying out any data processing, examine the data in their most original format in order to identify some of the longest individual responses and to look for unusual characters in the texts. The longer responses may get truncated in some conversion processes (for example on being brought into SPSS if the 256 character default was not changed) and it is useful to be able to check that you have the fullest versions before analysis begins. Unusual characters, other than basic alpha-numeric and punctuation, sometimes affect conversion processes so it is a good idea to check that these have been copied faithfully before proceeding. |
Create a separate Excel worksheet for each open-ended question, grouped into a single workbook using the sheet name tabs to identify them. Each worksheet should have two columns, one with the unique respondent identifiers, and the other with the response texts. Each row represents an individual respondent. Adjust column widths and use the Word-wrap and Autofit row-height commands (or their equivalent in your spreadsheet program) to display the longest responses in full.
2. If necessary, reformat the IDs
The identifier for each respondent (or "case" in NVivo’s terminology) will be very significant in this process, in order to link the socio-demographic characteristics with the analysis of the responses and later to link the responses to different questions made by each speaker. Therefore careful thought about identifiers will be helpful at this point. Each separate response must have some means of identifying the respondent who made it. Generally in quantitative surveys there will be a serial number, not necessarily in a completely unbroken sequence as it may originate in a sampling procedure, whereas qualitative researchers are more used to using forenames or initials to identify their informants. For the purposes of the sort of analysis envisaged here it is very important that each identifier (ID) is unique and serial numbers are probably more reliable for this purpose.
For some later purposes it will be useful if the ID is in the form of a text string, rather than a pure number, so it is important to prefix the serial numbers with a few letters, such as "RESP". This can be done quite simply in Microsoft Excel by using the concatenation function, as illustrated below.
If cell B3 has a serial number in it (say 741), in cell C3 type the following logic:
=("RESP.0"&B3)
Cell C3 will then display the result as RESP.0741
and this may be copied down the column as many times as necessary.
Finally, the whole of column C should be copied and pasted into column D using the paste special command set to paste values. This removes the underlying logic and stores the strings as pure text which can be copied and pasted to any other location without trouble. These steps are illustrated in Figure 1 below (using Microsoft Excel 2007).
Figure 1: Converting serial numbers into text strings
Avoid using characters like hyphens or colons in these text strings as they can sometimes cause problems in later processes in NVivo v7. Having reformatted IDs in this way ensure they are in place beside the corresponding response texts in each question’s worksheet. Save the workbook.
3. Export the response texts and IDs to Microsoft Word for formatting
In Microsoft Excel highlight the two column block of IDs and their associated response texts for one question, click on the copy icon (or press Ctrl + C), open a new Microsoft Word document in portrait layout, and click on the paste icon (or press Ctrl + V). The data should appear as a two column table in the Microsoft Word document.
It is then very important that you set all of the IDs to a consistent style (we find "heading 2" works well, but it could be any heading level) that is different to the style used for the responses. This can be done quite simply whilst the data is in table layout by highlighting the whole column of IDs and applying a format command to it.
TipYou may find it helpful, when it comes to reading the response texts in the analysis phase, if you set this particular heading style to be a different colour from the main text (in the examples below, blue has been used for this purpose, see Figure 11) as it helps the eye to concentrate on the significant material and is useful when retrieving data according to thematic/conceptual codes later on. It may also be worth creating a template with the desired colour and font settings for use with the subsequent question documents. |
Next you should convert the table to text, using the Table/Convert/Table to Text command while the cursor is positioned within the table, see Figure 2 below, (in the MS Office 2007 version of Word the command Convert to text is to be found at the right hand end of the Table Tools / Layout ribbon). It is important that you set the command to separate text with Paragraph marks, as illustrated in Figure 3 below. This command will generate a layout with alternate lines of case ID and response text.
This combination of heading style and paragraph mark separators is essential in the NVivo autocoding process to be applied later.
Figure 2: Convert table to text (Microsoft Office 97-2003 version)
Figure 3: Set separators to paragraph marks
Save the file as a Microsoft Word document, locating it in the directory where the rest of your project data is stored, and using a short name that unambiguously identifies the question to which the responses belong. This name will be used by NVivo as the source name once the file has been imported.
4. Select the socio-demographic attribute variables that will be required to be available in NVivo to inform the qualitative analysis and copy them into Microsoft Excel
This step is similar to the first in some respects but contains the attributes of the respondents which may be relevant to the subsequent analysis. It is quite possible to add further attributes for all respondents during the analysis, so the decision over which attributes to include at this point is not an irrevocable one with NVivo.
TipHowever it is worth making sure that you use all of the data that you can reasonably expect to consider during the analysis, as it is easier to incorporate it at this stage. |
For further comments and guidance on selecting variables to use in such analysis please see the notes here.
It is quite likely that the variable data is available to you in a statistical program like SPSS, in which case there is a straightforward procedure for transferring the data to Microsoft Excel. However, before you start the transfer it may be worth considering doing some recoding within SPSS in order to simplify the variables. It is possible to alter some strings in Microsoft Excel, by using "find and replace" commands, but it is certainly easier and less error prone to do this in SPSS. In SPSS terminology, it is the "value labels" that you will be exporting and so these are the items that need to be made short and clear. The number of different values each variable can take may be significant so reducing these by grouping some together may be helpful. Again, more guidance is given on this page.
The procedure for exporting data from SPSS to Excel uses the save as command in SPSS.
TipIt can be done in a single step but a two stage process provides a mid-point to return to if something goes wrong. |
Having completed the selection and recoding decisions and processes and saved the full dataset, start the save as command to create a new data file with all of the cases but only the particular variables that are wanted for analysis in NVivo. Click on the variables button to open the dialogue screen that allows you to select a subset of the variables into a new dataset. It will probably be best to click on the drop all button first, to uncheck every variable box, and then manually tick the box for each variable that you have decided to use (in its recoded form if you made that a new variable). Make sure that you include the ID or serial number variable in the list. When this selection operation is complete, click on the continue button, enter an appropriate new name and path for the file to be stored at (you don’t want to overwrite the master data file and thus delete valuable data), leave the file type as "*.sav", and save the file.
Now open the new data file that you have just created in SPSS and close the master data file (which will still be open if you are using SPSS v15 or higher), and check that you have all of the data that you expect to bring into NVivo. If necessary repeat the last steps to correct any omissions or errors. When you are satisfied that all is correct, start another save as command. This time on the main dialogue screen change the save as type field to "Excel 97 through 2003 (*.xls)" by using the drop-down menu. When this is selected, two further options below come into use. You should tick both of them – write variable names to spreadsheet and save value labels where defined instead of data values. The first of these will provide helpful identification of each attribute as column headers in Microsoft Excel, the second makes sure that you can interpret the attribute in a qualitative environment ("mother" makes more sense than "1"). Finally enter a file name, which can be similar to the one used for the temporary SPSS file because it will have a different extension (.xls instead of .sav) and hit the save button. (If you have Microsoft Office 2007 available you can use that version to save to "*.xlsx").
5. Edit the variables in Excel as necessary then save as a Unicode Text file
Using Microsoft Excel, open the file that you have just created.
It is very important that the case IDs in this file exactly match those used in the response text documents. So, if you reformatted the serial numbers as ID strings for the response text documents, you will need to repeat the process (from step 2 above) here so that the IDs in the Casebook can be connected to the IDs in the response texts.
TipYou may find it helpful to edit the variable names, which appear at the top of each column, to be more easily interpreted within NVivo later. Check also that the value labels for each variable are simple, clear, and as short as possible. Both the variable and value names can be edited with the find and replace command if necessary. |
The ID strings must appear in the first column in order for the automatic case creation procedure in NVivo to work correctly. There should be no empty columns or rows within the table, and only the data that you want to import into NVivo should be present. See Figure 4 for an illustration of these points.
Figure 4: Variables after being edited in Microsoft Excel
Now select the file / save as command, locate the directory where the rest of the project data is stored, choose a suitable name (including the word "casebook" to avoid uncertainty) and select the "unicode text" option from the save as type pull down menu list. See Figure 5 for an illustration of this step.
Figure 5: Save as unicode text for import into NVivo
6. Import the unicode text file into the NVivo casebook, and create the cases
At last it is time to open NVivo and create a new project.
TipOur recommendation here is to use the casebook data to create all of the cases before importing the response text documents and autocoding them to those cases. This is because the casebook file has a row for every case in the project, whereas the response text files each carry a subset of cases (those who answered that particular question), so all of the required cases will be created in a single process by the casebook import instead of incrementally through the text file imports. |
However, if the response texts have already been imported into an NVivo project and thematic/conceptual coding has begun, then it would be possible to import the casebook data file into the project later on, provided the case names are exactly the same as the ID strings in the table.
Due to the way the autocoding routine works in NVivo, it is necessary to create the cases as a group beneath a ‘header’ or ‘top level’ case so the first task in the new project is to create a single case.
Figure 6: Create header case in NVivo project
In the navigation pane, select nodes and then choose the cases subfolder. From the new drop-down menu, select new case in this folder to open the new case dialogue box (Figure 6). Enter a name for the set of cases into the name field, in this example we used "serials". No other entries are absolutely necessary in this dialogue box, so hit OK.
Select Tools / Casebook / Import casebook ... from the main menu to open the dialogue illustrated in Figure 7. Using the Browse button, locate the unicode text file that you created at the end of step 5 for the import from field. There should be no need to alter the defaults in the format fields. For the three fields under the heading options, ensure that create unmatched attributes and create unmatched cases are checked, but leave the first one blank because there should be no existing attribute values in a new project. Finally, and most importantly, the case location field needs to be set to the name of the single case you created in the instruction above by using the select button, in our example it is "cases / serials". It is necessary to use some care with this selection dialogue; you have to click on the cases folder in the left hand panel to display the serials case on the right, where the case node checkbox can be ticked. Only then will the OK button become active and return you to the main dialogue box, where you should confirm that the field correctly displays the "cases / serials" path, before clicking the final OK to effect the whole import command.
Figure 7: Import casebook to NVivo project
The procedure will take some time, depending on the number of cases and variables being imported. A process bar will appear in the bottom left corner of the NVivo window and, when the process is complete, the expected total number of cases (plus one for the ‘header’ case) should appear there as well (you may need to click on the expand button "+" to the left of the serials case node to reveal the individual sub-nodes).
TipSome variations on the above routine may be used in different circumstances. For example, if you are adding further variables to an existing casebook you will only need the Create unmatched attributes option to be ticked. If you are re-importing the casebook to correct erroneous data you will need to tick the replace existing attribute values option. If adding further data to an existing project, such as a longitudinal study, it is advisable to generate a secure project back-up before commencing this operation. |
To check the import has worked successfully, open the casebook from the Tools / Casebook / Open casebook ...menu and investigate any cells in the table which show "unassigned". This value should only appear in the header case (see the first row in Figure 8), unless you are aware of missing data for some cases.
Figure 8: Check NVivo casebook
Note how similar this is to the Microsoft Excel spreadsheet. However, NVivo has altered the order of the variables so that the columns appear in alphabetical order according to the variable names.
Save the project.
7. Import the response text documents into NVivo and autocode by case IDs
The text documents are imported into NVivo’s Sources area within the "internals" folder in the same way as interview transcripts would be. Click on sources and then internals in the navigation panel. Then select the command Project / Import internals from the main menu bar. This brings up a dialogue screen like the one shown in Figure 9. Click on the browse button to open a standard navigation dialogue and use it to locate the files that you created at step 3 above. It is possible to import several files at the same time by using the shift or control keys with the mouse clicks.
Of the three supplementary questions in this dialogue screen we recommend that you tick the first and third but not the second. When importing files one at a time, ticking the text option to create descriptions will cause the program to prompt you to fill in a description field in the properties box for each NVivo source (it is a good idea to include the full text of the question that was used to generate the responses in each file as part of this description). If importing multiple files simultaneously, ticking this option will automatically create source descriptions by turning the first paragraph of each file into the corresponding source description. You do not want to code these sources as new cases because you have structured them to contain many cases, so leave that option blank. The final option, to create as read-only is recommended in order to protect the response texts from accidental alteration – it does not affect coding operations. Click on OK to effect the import command.
Figure 9: Import documents into NVivo project
The next step, the autocode routine, can be carried out separately for each source or for several sources together.
Within sources / internals, click on a single source to highlight it, or multiple sources using the shift or control keys, and then select the command code / auto code... to open the dialogue screen shown in Figure 10 (note its icon which can be found towards the right end of the coding toolbar – and is highlighted in yellow near the top right corner of Figure 10).
This is the point at which the heading style, which you applied to the respondents’ IDs at step 3 above, becomes relevant. In our example we used "heading 2" to format the IDs so that option has to be moved into the right-hand box as the selected paragraph style. It is then important to get the correct settings for code at nodes. All of the cases have already been created in step 6 above, so you need the existing node setting, and the dialogue behind the select button here works similarly to the one used to create those cases in step 6 above although the screen display (when you have selected the correct header case as before) is subtly different as can be seen if you compare Figure 7 and Figure 10. Click the OK button to effect the command and watch the green processor bar display.
When the process has completed you should see the number of responses in the question document appear in both the nodes and references columns in the top half of the main window. In the example shown in Figure 10, the first seven documents have already been autocoded and so the numbers of nodes and references contained in each source are listed in the adjacent columns, whereas the final document ("QVAL2") is about to be processed.
Figure 10: Auto code the response documents by cases
TipA final check on the success of the autocoding process can be made by opening a document, turning on the coding stripes to "nodes recently coding", and scrolling to the bottom of the document. This should display something like Figure 11 at which point you can confirm that the coding stripes do not overlap, so that every part of the document has been coded to exactly one case. |
Figure 11: Checking coding stripes
TipIf you open the cases folder within nodes and click on the expand "+" button beside the header case ("serials" in our example) you should be able to see the beginning of your full list of respondents. The number appearing in each row beneath "sources" and "references" indicates the number of questions answered by that respondent. In Figure 12 it can be seen that respondent number 04806 has answered 4 questions of the 8 used in this example. |
Figure 12: Check cases after autocoding
Save the project. You are now ready to begin the analysis phase of your project.
The following material provides step-by-step guidance for preparing the texts, collected from a large number of respondents answering several open-ended questions in a survey situation, for qualitative analysis using QDA Miner software. |
There are several stages in this procedure, some of which may not be relevant in your circumstances. Some users may have alternative methods or short-cuts, in which case please use your own judgment as to which elements from below to apply. Our purpose here is to provide a comprehensive guide, which has been tested and proved to work, for the benefit of those who have not achieved this task successfully before.
Unlike some other CAQDAS packages, QDA Miner was designed with this sort of task in mind and so the steps outlined below do not represent a workaround but a mainstream activity.
1. Select the software to use in an alternative in an intermediate process
QDA Miner can import data in a wide range of formats so there is considerable flexibility over this step.
TipBefore you do any data preparation it may be a good idea to explore the possibilities available to you. |
Start the QDA Miner program and, at the first dialogue screen, select the create a new project option. This takes you to the dialogue screen shown in Figure 1 below.
Figure 1: New project options in QDA Miner
It is most likely that the third option above will be useful to you, so explore that one by selecting it first – import from an existing data file. Move straight to the drop-down menu beside the field files of type: near the bottom of the dialogue box and click on the down arrow to display the full list of data formats which can be imported directly into the program. Figure 2 shows the list in version 3.2.2 of the software at the time of writing (July 2009), you may have different possibilities available in your version.
Figure 2: Existing data file formats available for import into QDA Miner (v. 3.2.2)
If your data is already set up in one of these formats then that will be the option to explore first, otherwise you should consider which of these formats might be the most convenient to use as an intermediate step between your existing data format and QDA Miner. Obvious choices to consider are the spreadsheet options (Microsoft Excel or Lotus 1-2-3) or the statistical program (SPSS for Windows).
TipFor this purpose it is most unlikely that you would want to use one of the other CAQDAS programs listed here as they generally have more difficulty handling open-ended survey question data, those options are made available for converting other types of project which have been started in another software package. |
Two of these options will be discussed in the sections below, Microsoft Excel and SPSS, as it is considered that these are the ones most users of this website are likely to use. There is some guidance in the QDA Miner help file which may also be worth consulting.
Note also an alternative option from the dialogue in Figure 1, create a project from a list of documents / images. This is the route that many conventional qualitative analysis projects would take, whereby groups of documents are imported into a QDA Miner project and variable data about their cases is added later.
TipIt is not recommended that this option is used where you have a large number of cases each making brief comments because this situation is much more efficiently addressed by the methods explained below. |
2. Locate and organise all of the data to be used in the analysis in a logical structure
We will look at the spreadsheet method first because this is probably the least software specific method available. This will be illustrated with reference to Microsoft Excel, although any other spreadsheet program can be expected to work in a similar fashion and no specialised functions are required.
The recommended structure to aim for is one in which you arrange the data in a table, or grid, with a separate row for each respondent (or case). The columns should hold both the texts of the answers to the open ended questions and the variable data about each respondent which may be relevant to the analysis.
TipBefore carrying out any processing of the data, try to examine it in its most original format in order to identify some of the longest individual responses and to look for unusual characters in the texts. The longer responses may get truncated in some conversion processes (for example on being brought into SPSS if the 256 character default was not changed) and it is useful to be able to check that you have the fullest versions of these before you carry out the analysis work. Unusual characters, other than basic alpha-numeric and punctuation ones, sometimes affect conversion processes so it is a good idea to check that these have been copied faithfully before doing analysis work. |
It may be easier to understand if you keep the variable information in the left-hand columns and put the question response texts to the right. There should be no blank rows or columns in the table at the point of transfer to QDA Miner, so check for that problem from time to time. (If you have a blank row the import process will create an empty case for it, and a blank column will become an empty variable in QDA Miner). When you import the data into QDA Miner the program will add a sequential case identifier number of its own to each case but, if you anticipate wanting to relate some data from this analysis to other materials stored outside the program, you may wish to retain your existing case identifiers as a string variable – no specific format is required for these. The first row of the table should carry the variable names and there should be no duplications amongst these.
TipIt will be possible to import further variables or sets of response texts at a later stage, so the decision as to what to include at this point is not an irrevocable one. However it is worth making sure that you use all of the data that you can reasonably expect to consider during the analysis, as it is easier to incorporate it at this stage. |
See more discussion and advice see the selection of variables / attributes to use in CAQDAS section above.
An illustration of a spreadsheet prepared for data transfer to QDA Miner is shown in Figure 3. In this example column A has been used to store an ID for each respondent, columns B to H hold demographic and other variable data about the respondents, and columns I onwards hold the response texts. Note how the formatting of columns I to K includes word wrap and autofit row height functions so that the longer texts can be seen in full. In this presentation it is difficult to see if there are any blank rows so it can be useful to turn this formatting on and off for different viewpoints.
Figure 3: Microsoft Excel spreadsheet prepared for import into QDA Miner
TipQDA Miner will distinguish tetween socio-demographic variables and texts for analysis on the basis of the number of characters in the data cells. It is best to keep the variable labels short, say less than 30 characters. |
Having prepared the spreadsheet carefully, save it to a location where it can be easily located for importing into QDA Miner.
As an alternative to working through a spreadsheet program like Microsoft Excel above, it may be possible to take data straight from SPSS for Windows to QDA Miner. At Figure 2 above you can see that the format "SPSS for windows (*.sav)" is an option. However it is not necessarily a problem free operation and some preparation may be needed for this. In particular the frequency with which new versions of SPSS are issued increases the possibility of incompatibilities arising in such a transfer.
TipAs a preliminary point, if you have a very large data set in SPSS it may be worth filtering off a modest sized sample of cases and variables into a temporary file and exploring how well it is imported into QDA Miner. If any problems are identified with this subset of the data you will save yourself time by not processing large amounts of data fruitlessly. When you have proved that a set of procedures works successfully for the sample, you can apply them to the full dataset with confidence. It is also recommended that you look carefully at some of the longest responses in your dataset to see if they have been truncated in SPSS by a default character number limit. If possible check back to an earlier source of the data before it was copied into SPSS. |
3. Import all of the data into QDA Miner in a single operation
If necessary, open a new project and, as shown in Figure 1, select the option import from an existing data file. You will have to change the files of type: field to the right setting for the program you have used in the above preparation step.
If you are importing from Microsoft Excel, select that from the pull-down menu (see Figure 2) and then navigate to the location where you saved your input file in the previous step, select it and click on the "open" command. The dialogue screen changes subtly as you are asked to provide a filename for the new project. Next you will be asked whether you want to import a whole worksheet or a specified range. It is likely that you do want to import the whole sheet so leave the default range on "all", otherwise specify the appropriate range for the data table, and click on "import". If in doubt, click on cancel and check your source spreadsheet before starting the import process again.
TipYou can observe the progress of the import as the screen shows a count of the number of cases imported. As a further control check, on completion of the routine the bottom left hand corner of the working window shows 1 / #### (where #### stands for the total number of cases imported – in Figure 4 this appears as "2/1257", the 2nd case out of 1,257 is highlighted), so you can confirm that the number of cases you expected has been imported successfully. |
Figure 4: Data has been imported from Microsoft Excel into QDA Miner
One way of interpreting the desktop layout illustrated in Figure 4 is that some elements of the spreadsheet layout have been retained. The cases are held in rows, as shown with the column of case numbers in the cases window. The documents are held in columns, as shown by the row of tab labels at the top of the Documents window. But only one cell’s contents are visible at a time – so the text "leaflet from the environment agency" which can be seen in Figure 4 is the response by case #2 (highlighted) to question QADVI2 (emboldened tab label). From this position, moving the highlighter line down the cases window will bring other responses to question QADVI2 into view in the document window, and clicking on other tab labels will bring case #2’s responses to other questions into view.
TipNote in Figure 4 how QDA Miner has added its own case identifiers ("case #2" is highlighted) and shows the imported identifiers as the first variable ("ID Resp.04403" in this example). |
4. Check the accuracy of the import procedure
Having confirmed that the correct number of cases is showing at the bottom of the screen, you should next check the number of variables. The variables panel to the left of the main window can be enlarged to help display a long list of variables. The list of variables should be in the same sequence as the columns in your source spreadsheet, and this should aid checking its accuracy.
A significant point is that the text variables, which contain your response texts, should have received different treatment from the demographic variables. In the variables panel these items should show the word "[document]" where the other variables show the appropriate value for the current case (which is highlighted in the cases panel above), and these document variables should also be visible as tab labels at the top of the documents panel. This is illustrated in Figure 4 above, which shows the situation just after a data import from an Excel spreadsheet.
However, on some occasions the import process does not quite work correctly for all document variables. In the example shown in Figure 5 below, one text variable has not been fully recognised as a document during the process. Compare Figure 5 with Figure 4 and note two significant differences.
Figure 5: A problem with a data import
The data import shown in Figure 5 has not been fully successful because the document called "QLOC2" has not been fully recognised as a text document. This is apparent in the variables panel where the space to the right of the variable name is blank, and also in the documents panel where there is no tab showing "QLOC2".
The best way to rectify this situation is to adjust the setting in the Project / Program Setup command for "Import as document text longer than:" to a low value (but greater than the length of your longest socio-demographic variable), say 50 characters, and then re-import your data into another new project.
Alternatively, in this situation the problem can be resolved quickly with the following procedure:
- In the variables panel, left click in the empty space to the right of the document name (a dotted field outline appears)
- Right click in the same place to open a menu (Figure 6)
Figure 6: Right click to open a menu
- Left click on "transform xxx" in the menu (Figure 6 - xxx will be replaced by the name of your variable, this is context sensitive so do not proceed if this is showing the wrong name)
- Left click on "string > document" (Figure 7)
Figure 7: Left click on "string > document" (Figure 7)
- At the dialogue shown in Figure 8 leave the default on "overwrite existing variable" and click on OK.
Figure 8: Overwrite existing variable
After a brief time of processing, dependent on the size of the document, the word "[document]" should appear beside the affected variable name and a new tab should be inserted in the appropriate place at the top of the documents panel – see Figure 9.
Figure 9: After correction of import error for document QLOC2
TipNote also that the sequence in which the variables appear can be changed, by using the "reorder" command which is visible in Figure 6 above. It makes sense to have the socio-demographic variables at the top of the list, where they will usually be visible, and the text variables at the bottom, where they can be safely obscured when a larger panel for codes is needed. You may also notice that the word "document" sometimes appears in the variables panel in capital letters, and sometimes in lower case letters. The capital letter version indicates that there is a response text for that document from this respondent, whereas the lower case version indicates that there was no response. If you look at the data in the Simstat module you will observe a similar pattern with "TEXT" and "Text". |
Finally, to check the accuracy with which the texts have been imported it is recommended that you generate some text retrieval reports where you can verify that the longest responses have been imported successfully. To view all of the responses for one question use the command analyse / text retrieval, select the document with the drop-down menu by search in, set the search unit to paragraphs and click the radio button by retrieve all units, then hit search. On the search hits results page, click the check box by multilines grid in order to see the longer responses wrapped over multiple lines (you can adjust the size of the window and the width of the text column to see more material if you like). You could check a specific text by clicking on the appropriate case number and document tab but the re-numbering of cases during the data import process may have made locating a particular respondent more difficult.
Qualitative and quantitative analysis strategies
There are many different ways to analyse responses to open-ended questions. Our guides are a series of observations about how the features of each CAQDAS package might interact with a particular type of dataset. They are not step-by-step instructions on how to conduct analysis. Each guide should be read in context with related guides to using each CAQDAS package for analysing open-ended survey questions. Also note the data preparation instructions, since the quantitative strategies outlined below can only be effected after the data have been imported and coded systematically in a particular CAQDAS project.
This page was edited when the current version of ATLAS.ti was 6.2.16. If you cannot find some of the facilities mentioned it may be because you are using an earlier or later version of the software. |
1. Reading the texts - by respondent or by question?
In ATLAS.ti the decision as to which way to read the texts had to be taken at the data preparation stage. If the texts were organised in the way suggested in the data preparation instructions on this website, that is to say grouped by the question to which they refer, then there is no effective way of displaying them in the opposite way once they have been assigned to a hermeneutic unit (HU) and coded by the attribute variables. All that can be done to read all of the responses made by any one respondent is to carry out a text search across all of the documents using that respondent’s unique ID string as the search term. This will locate their responses one at a time, within the source context of the question documents and so will prove to be a tedious method to use other than very occasionally.
If the texts have been imported using the "survey import" routine that was added to ATLAS.ti in version 6.2 then a separate primary document will have been created for each respondent and a thematic code will have been applied to identify each separate question within those documents. By using the output for a single question code it will be possible to read through all of the responses for that question isolated from the other question responses and this may be an effective basis for manual coding of the themes within a single question. However it will not be possible to use the automation tools (to be described below) effectively with the data in this form. So, where the number of respondents is very large and a degree of automation is desirable, it is still worth considering preparing the data on a document per question basis and using the procedures described below to speed-up the work of analysis.
The remainder of this document will look at the strategies that can be used in ATLAS.ti to analyse a large number of response texts that have been organised in the document per question format. For a discussion of the merits of this approach versus the document per case approach see the relevant section above. For this approach it is a simple matter to open a primary document containing all of the answers to a single question and read through them systematically by scrolling the document in the main screen.
Figure 1: A basic working screen layout in ATLAS.ti
Figure 1, above, shows an initial layout of window panels in ATLAS.ti as the analysis work begins. The central panel displays a primary document containing response texts (in black font) and the ID/socio-demographic strings associated with them (see the data preparation instructions for guidance on how to create this document structure). To the left two of the object manager windows have been opened and arranged. At the top is the primary document manager showing the set of documents containing the responses to eight separate questions. Below that is the code manager showing some thematic codes and some socio-demographic codes (using colour to distinguish these). To the right is the "margin" area of the display, which scrolls with the central panel, here showing the quotation brackets and codes for the seven socio-demographic codes which have been attached to each response (coloured pale pink).
TipThere is a potential difficulty with the socio-demographic codes, which will be needed later for investigating patterns of response amongst various sub-groups of the survey respondents, because they may obscure clear sight of the thematic codes as these are applied to the response texts. Two alternative suggestions may be helpful here. |
As shown in Figure 1, use may be made of the facility in ATLAS.ti to set different colours for code labels. In the code manager window highlight a block of codes using the mouse and then click on the circular colour icon in the code manager toolbar to select an appropriate colour for that set of codes. To see those colours used in the margin area it is necessary to right-click in the margin area and select "use object colours" from the context menu that will be displayed there. Selecting pale colours for the socio-demographic codes and bold colours for the thematic codes will help the latter to show up more clearly.
A second suggestion is to hide the socio-demographic codes with a filter command so that only the thematic codes will be visible in the margin area. This involves several steps which may not seem straightforward to those who are not experienced users of ATLAS.ti, so more detailed guidance on those steps may be found in the qualitative analysis strategies for ATLAS.ti below.
2. Developing a coding scheme – manually or by using word frequency tools?
The nature of the analytic strategy will affect whether it is appropriate to develop a coding scheme manually or by using word frequency tools. If working deductively a coding scheme may be derived from, or informed by, existing (theoretical) frameworks; in these situations the following comments will not really be relevant. If, on the other hand, you are working inductively and therefore intend to generate coding categories from the ideas mentioned in the response texts themselves then you have a choice as to how to proceed. You may work ‘manually’ by reading the texts and choosing categories that seem to be mentioned in those texts or alternatively let the software help by creating a list of the most frequently used words in the texts and allow code development to be informed by this list.
The manual, or maybe that could be termed "human", method will be required at some stage if really accurate coding is needed, because only human readers can detect all of the subtleties of human expression involving multiple ways of phrasing any particular idea. However to get started, particularly in a large dataset, it may be worth trying the word count method to get an early idea of the range and salience of words used. The most frequently used words of interest (ie ignoring trivial words) may be expected to provide indications of the most frequently expressed concepts, although multiple possible meanings for some words can complicate this assumption.
In ATLAS.ti the word count function is found in the Tools / Word cruncher menu option or by using the icon from that menu which can be found on the main toolbar. The dialog box for this command is illustrated in Figure 2.
Figure 2: Word cruncher dialog box
Taking the check boxes shown in Figure 2 in turn, the following comments may be helpful:
- Include selected PD only: this governs the extent of the material to be included in the count, a single document (the document currently on view), all of the documents in the project, or the set of documents limited by a current filter. The approach taken in this example is to just analyse the responses to a single question, QADVI2, so that document has been opened (behind the dialog box), and this option is ticked.
- Use built-in tool: this option is a matter of judgement. By leaving it ticked you will stay within ATLAS.ti and the limited facilities to sort and display the output from this process, whereas by unchecking this box you will create a spreadsheet outside the program where you may be able to exploit many more sorting and displaying functions. We would recommend staying within ATLAS.ti at first and only exporting to Excel when you are comfortable that the word counting approach is suitable and you need more sophisticated tools to process the output. However, if you have selected more than one document in the choice above, you will be forced to export the results because the built-in tool can only handle a single document at a time.
- Use stoplist: this function allows you to exclude certain selected trivial words from the calculation and should help you to ‘see the wood for the trees’ more clearly. The default stoplist is very short, and hardly excludes anything, so, if you choose to use it, you will probably need to set up your own list and edit it for each document that you need to analyse. To edit the stoplist, first cancel this command and then select extras / explorer / user system folder to open a folder window where you should be able to see the file "stoplist.txt". This can be edited in Windows Notepad by typing additional words to exclude in capitals at the end of the list. If you save the amended stoplist file under a different name you will need to amend the file name in the Word cruncher settings dialog to match it. In this illustration it has been saved as "FloodStoplist.txt".
- Clean text before counting: this shows a set of characters to be ignored in the counting process, in most cases this seems likely to be a sensible option to use.
- Ignore case: again this is likely to be a useful option so that a word which sometimes starts with a capital letter will be counted along with the occasions when it is all in lower case.
An example of the output from the word cruncher calculation is shown in Figure 3. The table can be re-sorted by clicking in the header area of each column, in this illustration this has been set to decreasing order of the count column, so the most frequently used words are at the top of the list. It may often be useful to sort alphabetically by clicking on the "Word" header in order to see misspellings and plurals of words side by side. In this example it can be seen that the word "sandbags" appeared 41 times, but the alphabetical list showed that "sandbag" appeared twice, "sanbags" once, and "sand bags" a further 7 times. This information will be invaluable if text searches or autocoding routines are used to locate and code the responses with a code for this theme.
Figure 3: Word count output
The data which was "crunched" for Figure 3 related to advice respondents had been given about preparing for being flooded. In this context several potential coding themes can be seen in this extract. Sandbags have already been mentioned but "upstairs", "pack", "valuables", "furniture" and "leaflets" all look worthy of further exploration. Knowing all of the actual spellings used for each word from this output helps the researcher to investigate the uses of these words in an efficient way.
This illustration may also demonstrate why it pays to be really thoughtful about adding words to the stoplist. In Figure 3 it can be seen that the word "off" appeared 35 times. Whilst it would be understandable to exclude such a short word in many situations, in this case it was found that many of these 35 uses were next to words like "electricity", "gas", "mains", or "power" and that this little word was a useful way of locating most of these different phrases which referred to the single important idea of turning off utility supplies in the event of a flood.
Another inductive method of developing coding themes from the content of the texts themselves is for the researcher to read the texts systematically, noting ideas that appear to be important or relevant, and then creating codes to represent those ideas. There is no reason why open ended survey questions should not be analysed with this frequently used qualitative approach. However, where the number of responses to a single question is very large, and it is suspected that there is considerable repetition of ideas amongst those responses, then this approach may be found to be unnecessarily time consuming. ATLAS.ti has tools which, if used with imagination and skill, can provide powerful and thorough assistance for such tasks.
As you develop the ideas for your coding scheme you should also consider two other aspects of this work. Do you want to create any form of structure for your codes so that they can be located in the code manager in groups? (Note that it will be possible to make multiple groupings of codes later, with the code families tool, to reflect developing theories about relationships between the themes represented by the codes.) And, secondly, do you want to create a separate set of codes for each question in your data? There are no firm solutions to these questions, it depends on your own preferences and working practices, the nature of the data and your analytic approach. But, as ATLAS.ti has no visible structures for hierarchies of codes in its main code listing, you may need to consider using a system of letter prefixes in order to create some visible structure for yourself.
It is possible to create a ‘cosmetic’ coding structure in ATLAS.ti by prefixing groups of codes with a common letter. In this example we placed an ‘A’ in front of all codes used in the analysis of document QMORE and a ‘C’ in front of all the codes used in the analysis of document QADVI2. This makes it easier to locate the correct code when allocating them manually whilst reading the document on screen.
If the survey questions were largely unrelated to each other, perhaps because they were widely spaced apart at different points in the questionnaire, then a separate code group for each question may be suitable. However if you expect to be interested in the way some themes arose across multiple questions, then having a more unified coding structure may suit you better.
3. Text searching and autocoding
Before carrying out any coding activity, some thought should be given to the decision of exactly how much text should be coded, or in the terms used by ATLAS.ti, how much text should be included in each quotation. The significance of this decision will only become fully apparent when you start to retrieve sets of texts which have been allocated to a specific code or combination of codes. The issue to be decided is whether to include the whole response with the socio-demographic ID strings or just the phrase which is relevant to the concept being coded.
In simple terms, if you only code the minimum text or just the relevant phrase, it may be easier to check an output for consistency (because there will be less material to read) but it may be harder to locate an omitted code, an incorrectly coded passage or to consider a quotation in the light of its speaker’s characteristics (because there will be less contextual material available in most outputs). If the majority of the texts in your document are very short it would probably be better to include the whole response and the full ID string (i.e. the same quotation as was used for the attribute coding when the data was first prepared for CAQDAS - see analysing open-ended survey question data section for more information), because then all of the attributes will always be readily accessible in any set of quotations that are output. On the other hand if you have quite lengthy responses and you want to consider nuances of meaning within them then more precise coding and quotations may be appropriate. As far as the program is concerned there is no difficulty with shorter quotations because ATLAS.ti will be able to identify the attribute codes within which they are nested using the query tool.
Although many qualitative analysts may naturally prefer to do all coding work manually it is quite reasonable in some circumstances to use ATLAS.ti’s autocoding process. For example quite a lot of responses may be simply "don’t know" because the open-question has not triggered a specific response. Coding such material individually would be tedious work but by checking with a text search for that phrase (probably first with and then without the apostrophe, or both together by searching for "dont|don’t") and then using the autocode function these can be allocated to a code quickly and efficiently. With practice more positive common concepts may be identified and also rapidly coded in this way. It should be easier to follow this procedure and then eliminate any incorrect codings, following a consistency check, than to code a large number of very similar statements manually.
The text search function can be initiated in any one of several ways. The option edit / search can be used from the main menu bar, or else the shortcut keystroke Ctrl + F or the toolbar icon (both of which can be seen in the edit menu) can be used. This brings up a simple dialog box where the required string(s) can be entered and the currently open document searched forwards (using the "next" button) or backwards (using the "previous" button). With each click on next or previous the document will be scrolled and a further instance of the search word will be highlighted in the text where it can be read in context and its meaning can be assessed.
If you decide that a word or phrase has been used with sufficient frequency and consistency to justify an automated coding procedure then the autocode routine can be used. This is the same routine as the one used to allocate codes for socio-demographic attributes in the data preparation stage (see data preparation for ATLAS.ti for more information). However in this context it can be used a little differently because several variations of words and spelling can be used in a single coding pass. The procedure can be started with the codes / coding / autocoding option, and new codes can be created within the dialog box, but it is probably a good idea to create the required code beforehand and then concentrate in the dialog box on the variations for the search string.
Following on from the example used above, it was noted in the word cruncher output that there were a variety of references to gas, electricity and power. The first few text searches indicated that many of these referred to advice that these utilities should be switched off or disconnected before floodwater got into the house. These could all be autocoded in a single operation. As shown in Figure 4, below.
Figure 4: Autocoding using a category search
In Figure 4 it can be seen that the search expression is "electric*|gas|power". This will locate and code all sentences in the selected document which include any of these words, and the asterisk at the end of the first word means that "electrical" and "electricity" will also be located and coded. The word cruncher output had shown that there were no variations on the spelling of "gas" or "power".
Other points to note in Figure 4 are as follows:
- The scope of the search has been restricted to a single selected primary document, i.e. the one that is currently open; this fits with a working practice (used here) of coding one question’s responses at a time so that the meaning of a phrase is interpreted in the context of that single question.
- The quotations coded with this command will be extended to include the full response and the respondent’s ID and socio-demographic characteristics (indicated by selecting the "multi hard returns" setting).
- The case sensitive check box has been left blank so that instances where the first letter has been capitalised will also be located and coded.
- The Use GREP check box has been left blank because this search is not based around character structure in the texts.
- The "confirm always" box has been left blank so that all of the ‘hits’ will be coded automatically and the procedure will run quickly. Alternatively, by ticking this box it is possible to review each located ‘hit’ in turn and take a separate decision to ‘code it’ or ‘skip it’ using the buttons below the ‘start’ button, and even to vary the size of the quotation coded each time, but these options will slow the process down. The choice between fully- and semi-automatic working will depend on personal preference and the nature and quantity of data to be analysed.
- It is possible to save an auto coding search expression for re-use later, by adding a name or label for the search, a colon, and the equals sign in front of the expression. Examples of this can be seen in Figure 4 and are offered by the program when you use this function.
TipWhen this option was run it was noted that 28 quotations were added to the code “A Utilities”, this was fewer than expected as the Word Cruncher output had shown 38 occurrences of the variations of the words in all. A check on the actual quotations revealed that there were 10 responses where both electricity and gas were mentioned and this explained the difference. |
The autocoding process will not complete the task if data reduction to accurate quantities of references is the goal of the analysis (see section 4 below). The number of different ways in which a concept may be expressed will frequently exceed the number of ways the analyst expects to find it. So a combination of autocoding and human interpretation is needed to achieve a high level of accuracy. But time can undoubtedly be saved through the use of well-directed search and autocoding routines.
4. Coding – data indexing versus data reduction
The actual techniques of manually applying codes to segments of text are not discussed here. They are common to all applications of the program and are clearly explained in ATLAS.ti’s help manual and in other sources. However, the possible uses to which the analysis of responses to open-ended survey questions may be put is a matter worth discussing further.
As a coding scheme is developed and applied to textual data, the analyst will inevitably encounter uncertainty and doubt. Does one particular text represent something different from others read before which mentioned a particular keyword? A common solution to this is to be generous and inclusive, applying specific codes to a range of comments that initially appear to be connected to those concepts, with the good intention of returning later and checking the work. This activity may be described as "data indexing" as it facilitates the retrieval of various passages that appear to relate to a particular topic.
When open-ended questions have been asked in survey situations it may be anticipated that the analyst will often be asked to generate numerical summaries of the data, probably in the form of statements of the type "X% of responses to this question mentioned Y". The obvious source of the numbers for this output is the coding of concept "Y". However the statement will only be valid if the use of that concept in every one of the responses allocated that code is consistent and equivalent, because the code that is used in this way has effectively replaced the words recorded for each respondent. The original textual data has been reduced to the code label.
When put this way it should be apparent that work needs to be done by the analyst to refine the inclusive indexing codes before they can be safely used as ‘summarising reducing’ codes. In the example used above "upstairs" was the most frequently used word in the document, relating to advice to move valuable items to a higher part of the house in order to protect them from flood damage. It would be straightforward to autocode all of the responses that included this word with a code to reflect that theme. However a closer check revealed that, while most of the responses were indeed along the lines of "take everything important upstairs", one response was actually "I have a file upstairs which gave various useful tips as to what to do". It would appear that this latter comment is mentioning ‘upstairs’ in a different context to the others and so should probably be excluded from that coded group. Another respondent added a different dimension to the theme with "move stuff upstairs ! but no upstairs here -single floor flat", apparently acknowledging the common advice but drawing attention to its irrelevance in his particular circumstances. For some interpretations at least, this response also should probably be excluded from the main group to avoid counts of the occurrence of the word ‘upstairs’ being misleading.
5. Checking summarising codes – consistency and omissions
There are a variety of tools in ATLAS.ti to assist with the refinement of codes when they have to be reduced to summarise what was originally said. Two particular aspects should be considered, firstly confirmation that all of the passages connected to any one code are all sufficiently similar to be treated as equivalent, and secondly confirmation that no other passages that are also equivalent have been omitted from that code.
The first step in confirming consistency or equivalence is to extract all of the passages that have been allocated to a code and read them carefully looking for differences of meaning that might justify exclusion from this code group. An obvious way of doing this in ATLAS.ti is to generate an output report through the code manager window. Open this by clicking on the "codes" button in the object managers toolbar, select the code of interest in the code manager, then from the options list in that window select the option output / quotations for selected code(s), and then choose where to send the report from the subsequent dialog box ("editor" for screen display and maybe subsequent printing, or "printer" for immediate hardcopy).
Figure 5: Output - quotations for selected code
Figure 5, above, shows the first four quotations listed for the output of code "C Upstairs" (to continue the example). It may not be immediately apparent as to what is the significance of each element in this report. There are several lines of header information before the first quotation. At first glance it may appear that the quotation in square brackets in this line is identical to the similar text 3 or 4 lines below, but this is not quite correct. The text in the first line is the shortened quote name used in the quotation manager list, while beneath that is the full quotation. When anything longer than a brief phrase has been coded then the full quotation will be longer than the list name. (The number of characters included in the list name is controlled by a setting in extras / preferences / general preferences – general tab and has been set here to 50).
It is important that quotations are examined in full in order to maximise the probability of identifying inconsistent or incorrect applications of the code. So you should read the plain text versions to make that judgement because it is easier to compare quotations in this format. If you find a code that has been incorrectly applied you should note the paragraph number on which it occurs (the "(10:10)" section after the list name for the first reference in Figure 5) in order to locate it manually in the document. (ATLAS.ti does not have an interactive link between the report editor and the document to which it refers).
The second line for each quotation in this report is about the other codes that have been applied to this particular quotation and the code families to which those codes belong.
TipPlease note that this only shows the codes for this precise quotation, if it is nested within a longer quotation then the codes applied to the larger quotation will not be shown in this report. |
Whilst checking all of the quotations linked to a single code it should be possible to write a concise definition of that code. There is a place for this in the lower half of the code manager window. Highlight the code of interest in the top half, click the cursor into the bottom half and type the definition there. If you find it difficult to write a concise definition of a code then it may be inferred that you should not refer to the number of references to that code in any data reducing statements.
TipAnother way of checking the consistency with which a particular code has been applied involves using the network tool. Open a new network, probably with the label of the code as its name, and drag the code in. Then right click on the code in the network and select "Import neighbors" from the context menu, this will bring in all of the quotations for that code as a cascading series of tags. You may need to alter a display setting to read the full quotations, this is controlled in the network window by the command display / quotation verbosity / +full text . An advantage of this method is that the tags can be moved around in the network display and grouped according to similarities or differences, this may be helpful if a code theme is still being developed or the code needs to be split into sub-themes. This will not be so practical if a code has a large number of quotations. Also, by right-clicking on any quotation in the network and selecting display in context it is possible to jump to the source document (although in survey data this would only be helpful if the quotations are short extracts from the responses, and not the full responses). |
It is more difficult to search for code omissions; passages which are closely equivalent to those already allocated to a particular code but which have not yet been allocated themselves. One possible method for doing this is to filter-out all of the segments which have been allocated the code and then carry out a series of text searches using key words connected to that code on the remaining text passages. This is not a simple operation in ATLAS.ti but it is possible to do it quite efficiently using the following set of procedures.
In outline, the suggested method is to build a complex query (using the query tool) to identify all the potentially relevant responses which have not had the code of interest allocated to them, save the output from that query as a new document, assign that document to the project, run a variety of text searches looking for words associated with the code of interest, and investigate any results which appear to indicate that the response has not been coded despite containing an associated word. Finally, the temporary document can be "disconnected", to remove it from the project in order to prevent unnecessary clutter accumulating.
The specific query structure will depend crucially on whether you have uniformly applied thematic codes and relevant socio-demographic codes to exactly the same quotation, or at times have coded shorter phrases to thematic codes (see section 3 above). Some queries for the former coding method will be simpler than for the latter.
First, let us consider the simpler situation, where there exists only one quotation per response with both thematic and socio-demographic variables applied to it (i.e. the former of the two cases above). Open the query tool, using the binoculars icon in the main toolbar or the menu option tools / query tool. The query needs to extract just the responses in the relevant document that have not been allocated to the code of interest. In the example that follows the code of interest we are testing is called "A Accurate" and the texts are stored in primary document "P7 QMORE". So we will be looking for all of the quotations in P7 QMORE that are not coded with A Accurate.
If the "accurate" code has consistently been applied to the same size quotations as the socio-demographic codes then the query should proceed as follows:
- Using the "scope" button at the bottom of the query screen, limit the query to just the document(s) that you require by double clicking on the relevant document (in this case just QMORE) and close that dialog with "OK".
- Select the A Accurate code in the lower left panel with a double click and see it also appear on the right.
- Select the "NON" operator (the fourth icon down the left margin in Figure 6, a rotated L symbol) with a click.
- The query in the upper panel should now read NOT("A Accurate"), the list of quotations in the bottom right panel should now be everything in the selected primary document(s) which has not been allocated to the specified code of interest, in our example "A Accurate". The number of these quotations is shown in the bottom left corner of the query tool window and should be the total number of responses to this question less the number coded to the code of interest (361 – 32 = 329).
Figure 6: Using the query tool to check for omitted codings (thematic code quotations exactly match socio-demographic variable quotations)
Pick up the instructions to proceed from this point below Figure 7 (after the more complicated notes on handling shorter thematic quotations).
Next, let us consider the more complex situation, where only short extracts from the responses have been coded with the code of interest.
The first step, if it has not already been done for another purpose (such as hiding some code labels as outlined at the end of Section 1 above), is to create a code family for one of your socio-demographic variables. This will be used to define the full set of responses to any one question in a way that can be used by the query tool. Probably the variable with the fewest number of members (values) will be that for gender, so that should be the quickest to create (but it is important that there are no cases in the data with missing values for the selected variable). From the code manager window click on the yellow ring icon, or from the main menu line select codes / edit families / open family manager. Click on the create new family icon, enter a simple name (e.g. "ZB Gender" – using the same prefix letters as were used in the codes that will be its members) and hit OK, then select each of the codes that you have set up for that variable (i.e. "ZB Male" and "ZB Female") in the right hand panel below and move them to the left hand panel using the arrow button in the middle. No special save command is required to complete this process.
Next open the query tool, using the binoculars icon in the main toolbar or the command tools / query tool. The query needs to extract just the responses in the relevant document that have not been allocated to the code of interest. In the example that follows the code of interest we are testing is called "C Upstairs", the socio-demographic code family is called "ZB Gender" and the texts are stored in primary document "P1 QADVI2". So we will be looking for all of the quotations in P1 QADVI2 that are in family ZB Gender (which should be all of them) but excluding those that are also allocated to code "C Upstairs". See Figure 7 below for an illustration of the query tool window at the end of this process.
- Using the "scope" button at the bottom of the query screen, limit the query to just the document(s) that you require by double clicking on the relevant document (in this case just QADVI2) and close that dialog with "OK".
- Select the ZB Gender family in the top left panel with a double click and see that name appear in the panels on the right.
- Select the C Upstairs code in the lower left panel with a double click and see it also appear on the right.
- Select the "ENCLOSES" operator (9th button down on the left of Figure 7). The query expression in the top right panel should now read ENCLOSES("ZB Gender", "C Upstairs") the order of the terms is important with this operator.
- Select the ZB Gender family by double clicking in the top left panel again – this may seem counter-intuitive but trust us, it is correct!
- Select the "XOR" symbol (a "V" with a dot in the middle, 2nd button down on the left of Figure 7). The expression in the top panel should now read XOR(ENCLOSES("ZB Gender", "C Upstairs"),"ZB Gender").
- The list of quotations in the bottom right panel should now be all of the complete responses in the selected primary document which do not include anything that has been allocated to the specified code.
See Figure 7, below, for an illustration of the query tool screen at the end of this latter procedure. In the bottom line of the panel you can see "Result: 249"; this is confirming that 249 quotations are selected by the current query. It should be possible to use this information to confirm that the query is delivering what you expect, so in this example with 324 responses to question QADVI2 and 77 quotations coded to "C Upstairs" we would expect 324 – 77 = 247 quotations in the result – the difference of 2 items is accounted for by the fact that in two places the upstairs code has been applied to two separate phrases within a single response. As you build the query using steps like those outlined above this result figure adjusts at each step to count the hits found by the latest step in the process. It is also useful to observe that the restricted scope of the query is confirmed in this bottom bar.
Figure 7: Using the query tool to check for omitted codings (thematic code quotations shorter than socio-demographic variable quotations)
Whatever the length of your thematic quotations, from this point there are several possible ways to proceed.
- The simplest may be to click on each item in the results window within the query tool panel in turn and examine the interactively linked text in the source document that will be highlighted in the main working window.
- Alternatively you may choose to print the list of quotations in order to read the hard copy search result for references that have been omitted from the relevant code – to do this click on the printer icon in the query tool panel. Next you will see a small menu of choices for the content of the report, experience will help you in the future but for now we would recommend the "full content – no meta" option as the first to try as this will give you the report that is easiest to read. This particular option brings up another dialog, the "poor man’s reporter" with five options – of these you should uncheck "clip quotation contents" because it is important to get the full quotations in the report, it is probably also useful to uncheck "include source references" as this should save you unnecessary clutter in the report. Finally you get another dialog asking where to send the output to: "editor" sends it to screen, from where you can print it if you are happy with its appearance, "printer" sends it direct to hard copy.
TipNote that reports generated from the query tool may not appear in the same sequence of responses as in your primary documents. The responses will in fact be grouped according to the order in which the first socio-demographic variable was autocoded during the data preparation phase, because that process created the initial quotations within the document. |
- The final option brings you the possibility of more computer assistance. Follow the "print" notes above until the choice of output destination is reached, then choose the "file" option. This brings up a dialog for you to name the file and choose a location to save it in. You should edit the suggested name to uniquely identify the report (in this example, say, "QADVI2 Not C Upstairs.rtf"), save it in rich text format, and put it in the same location as the rest of your data files for the current project. It will then be a simple operation to Assign that file as a new primary document in the project.
- The advantage of this is that you can run text searches as many times as you like on this document, searching for keywords related to the concept behind the code, until you are satisfied that no relevant responses have been omitted. Remember that a nil result on a text search of this kind is a useful result as it confirms that you have already coded all instances of the search term.
- Then it is possible to remove the temporary primary document by using the document / disconnect command as it has no further use in your analysis.
- Note that if you do observe a response where an appropriate code has been omitted, you will need to use the information from the report to locate that text in the main primary document in order to apply the code there – it will be no use applying the code to the temporary report document which you are going to disconnect later.
Each of these processes may seem to involve a lot of work, so judgement will be necessary to decide how much is appropriate. These checks are important if you are going to use the code frequencies in any statistical analysis or reporting, they are not so significant if you are merely indexing the ideas in your data. If you started with a clear coding scheme and precise definitions of the codes before you began interpreting the texts then you may be more confident that you have applied the codes consistently and accurately. However, if you have worked more inductively, gradually refining the meanings and uses of the codes with ideas found within the texts, then you are more likely to have inconsistencies between the coding you did on earlier readings and those you did later. It is also important to check for these types of error if more than one person has been involved in coding any particular set of texts.
6. Looking for similarities or differences?
When analysing the responses to open ended survey questions it may well be easy to slip into the expectation that the most frequently used codes, or rather the concepts to which they refer, are the most important. After all, these are the items that seem to have the most interpretive and statistical ‘weight’. However, it should always be worth looking out for contributions which are different from the common ideas. One-off comments will never feature in the quantitative tables because, by definition, they lack numerical support. But a small number of individuals may well take the opportunity of an open-ended question to add an unexpected thought and these contributions represent a challenge and an opportunity for the analyst.
It is worth considering what the purpose behind the inclusion of an open ended question in the survey was. In many situations previous research will have revealed the most likely answers and these will have been included as response categories in closed questions asked elsewhere in the survey, but then an open question has been included to pick up other ideas. In these situations it is the unusual answers which may be of most interest. It is for this reason that it is worth analysing the open-ended questions systematically.
For instance, in the data used as an example for these instructions the question QADVI2 was used to ask respondents what advice they had been given in order to prepare themselves for an impending flood about which they had been warned. It may be interesting to note that out of 324 responses to this question, just three people mentioned warm clothing or blankets. Now it may be the case that for most people the need for warm clothing as you sit out a flood in an upstairs room is too obvious to be worth mentioning, but this may also be a clue that there was a potentially significant gap in the advice actually given to the flood victims in the incidents under consideration. It seems that the value of a detailed qualitative analysis of the responses to such a question is an opportunity to pick up the unexpected ideas which would be so easily overlooked in a statistical analysis.
Conclusions
There are many lengthy step-by-step instructions in the above materials. These have been included to help those who are not familiar with certain aspects of the way ATLAS.ti works. However this is not intended to imply that these are the correct / only / best ways of analysing the responses to open-ended survey questions in ATLAS.ti. These are merely examples of procedures that do work, particularly with data of the sort shown in the examples. However it will always be the case that different data may require different procedures, but we hope that these examples will help some analysts to get over the problem of using unfamiliar software or of using familiar software in an unfamiliar way.
Readers who are interested in comparing these processes across different CAQDAS packages should note that the centrality of the "quotation" in ATLAS.ti can be the source of complexity in these procedures. It is for this reason that we recommend that serious consideration be given to the decision to adopt the whole response as the standard quotation for thematic coding, unless the responses are particularly rich and full of meaning.
1. Reading the texts - by respondent or by question?
Out of the four CAQDAS packages reviewed in this section, MAXqda offers the greatest flexibility over how to read the response texts on screen. The choice between reading all of the texts respondent by respondent or reading all of the responses question by question has to be made at some stage, but in this program it is not determined by any earlier text preparation decisions and remains a free choice throughout the analysis phase.
Reading the texts by respondent
To read the texts respondent by respondent, open the first text by double-clicking on its name in the document system panel (top left in Figure 1) and all of the responses made by that respondent will be displayed in the text browser panel (top right in Figure 1). To move on to the next respondent, double click on the next name in the document system panel – the ‘open’ name is highlighted and shows a pencil icon superimposed on the text symbol.
Reading the responses by question
To read all of the responses to one question it is necessary to activate all of the texts and also to activate the code for that question, the responses will then be displayed in the retrieved segments panel (bottom right in Figure 1). Activating all of the texts can be achieved by right clicking on the group header title in the document system panel and selecting activate all texts from the context menu. Activating the question code requires a right click on the question name in the code system panel (bottom left in Figure 1) and selecting activate from that context menu. A left click on the information box beside any response text in the retrieved segments panel highlights that text, brings all of the responses by that respondent into the text browser panel, and highlights that respondent’s attributes if the attributes window has been opened.
Figure 1: MAXqda display showing the four working panels
In Figure 1, all 1,257 texts have been activated (indicated by the red icons in the document system panel), the code for question QADVI2 has been activated (red icon in the code system panel), some of the 324 responses to QADVI2 are visible in the retrieved segments panel (which has scroll buttons to display the others), the response by R.96312 has been highlighted in the retrieved segments panel and this has opened all of that person’s answers in the text browser panel (only four of the eight questions in this dataset were answered by this respondent).
2. Developing a coding scheme - manually or by using word frequency tools
The nature of the analytic strategy will affect whether it is appropriate to develop a coding scheme manually or by using word frequency tools. If working deductively a coding scheme may be derived from, or informed by, existing literature or (theoretical) frameworks; in these situations the following comments will not really be relevant. If, on the other hand, you are working inductively and therefore intend to generate coding categories from the ideas mentioned in the response texts themselves then you have a choice as to how to proceed. You may work ‘manually’ by reading the texts and choosing categories that seem to be mentioned in those texts or alternatively let the software help by creating a list of the most frequently used words in the texts and allow code development to be informed by this list.
The manual, or maybe that could be termed "human", method will be required at some stage if really accurate coding is needed, because only human readers can detect all of the subtleties of human expression involving multiple ways of phrasing any particular idea. However to get started, particularly in a large dataset, it could be worth trying the word frequency method to get an early idea of the range and salience of words used. The most frequently used words may be expected to provide indications of the most frequently expressed concepts, although multiple possible meanings for some words can complicate this assumption.
For MAXqda the word frequency functions are held within the module "MAXDictio" which is an optional add-on to the program. The word "MAXDictio" should be visible in the top menu bar if this module has been installed with your version of the software. Before selecting the initial menu option it is important to set the correct activations so that the word count is run on the passages relevant to your current work. In the context of a survey it is likely that you will want to develop a separate set of codes for each question’s responses (see section 1 above and further comments after Figure 4 below), so activate all of the texts and just one question’s code as the first step. Then select the MAXDictio menu and the word frequencies option.
Figure 2: MAXDictio - word frequency options
In Figure 2, above, the group of texts under the heading Survey have all been activated, along with the code for QADVI2, and text R.96322 has been opened. In the word frequency dialogue box, placing ticks in the first two boxes, only activated texts and only coded segments, will restrict the count to the full batch of responses to question QADVI2. The minimal number of characters field has been adjusted to length 4 in this illustration in order to reduce the number of trivial words included in the output list – this is a matter of judgement.
In Figure 3, below, the results of the procedure are illustrated. Confirmation that the words counted have been limited to those for the selected question is apparent in the number of texts included – the table shows "In 324 texts ..." and the highlighted code to the left shows that there are 324 segments in QADVI2.
Not all of the results are meaningful as indications of useful concepts, for example the fourth most frequently used word in this table is "from" and this does not seem to lend itself to a thematic code, so judgement will still be needed to select coding categories, but some useful information can be seen very quickly. This data is about advice on how to cope with the threat of being flooded, and so words like "upstairs, sandbags, valuables, furniture" can be picked out at once.
TipIt is worth noting that care should be taken over the word length threshold; for this exercise it was set at 4 letters, if it had been set any higher the word "pack" would have been excluded, yet its 44 mentions here may be interesting. |
Figure 3: MAXDictio - Word frequency output table
The data can be re-sorted according to any of the columns by clicking on the relevant column header. The example shown in Figure 3 has been sorted in descending order of frequency. It may be interesting to look at the longest words by sorting on the word length column for some complex ideas. An alphabetical sort may also reveal some minor misspellings, splitting a frequently cited word between two or more counts, for example in Figure 3 it can be seen that "leaflets" were mentioned 25 times but when that is combined with the 17 mentions of "leaflet" the total of 42 makes this an even more significant topic.
In some situations it may be useful to examine the contexts in which a frequently used word has been found in order to assess its suitability as a thematic code. A left-click on the required word in the Word Frequency table highlights it (as with "leaflets" in Figure 3 above), then a right-click opens a small context menu from which the option create index should be selected. This opens a second output window in which all occurrences of the required word are listed, identified by the text number within which they are located. The number of hits in this window may well exceed the frequency number in the other window because this index is not restricted to the single question code as before. However, by sorting the list on the paragraph column data the relevant occurrences will all be grouped together. A click on any row of this "search results" window brings that response up in the text browser window with the search word highlighted so that it can be read in its full context (see Figure 4).
TipThere is no need to close this window when you want to check another frequently used word, simply right-click on the new word in the word frequency window, reselect create index and a new list will be generated in the search results window, already sorted by paragraph number (if you had used that previously). In this way it is quite easy to examine the detailed usage of keywords before you decide whether to use them as the basis of thematic codes or not. |
Figure 4: Index of uses for a word selected from word frequency table, sorted by paragraph
As you develop ideas for your coding scheme you should also consider an important aspect of its structure. Do you want to create a separate set of codes for each question in your data or have a common coding scheme across all questions? There is no clear solution to this problem for open-ended questionnaire data, as it depends on your own preferences and working practices, the nature of your data and your analytic approach. If the survey questions were largely unrelated to each other, perhaps because they were widely spaced apart at different points in the questionnaire, then a separate group of sub-codes for each question may be suitable. However if you expect to be interested in the way themes occur across multiple questions, then having a more unified coding structure may be more suitable.
3. Text searching and autocoding
Although most qualitative analysts will naturally prefer to do all coding work manually it is quite reasonable in some circumstances to use MAXqda’s autocoding process. For example quite a lot of responses may be simply "don’t know" because the open-question has not triggered a specific response. Coding such material individually would be tedious work but by running a lexical search for that phrase (probably first with and then without the apostrophe, or both together using more sophisticated search criteria) and then using the autocode function, these can be allocated to a code quickly and efficiently. With practice more positive common concepts may be identified and also rapidly coded in this way. It should be easier to follow this procedure and then eliminate any incorrect codings, following a consistency check, than to code a large number of very similar statements manually.
In the example illustrated in Figure 5, below, the full set of response texts have been activated and the question code "QADVI2" has been activated, limiting the search to the responses to the single question but including all of the respondents. The menu option analysis / lexical search has been initiated and a new search string "environment" has been entered in the dialogue box (to locate references to "Environment Agency" in order to allocate these comments to the "EA" code). The checks in the options only in active texts and only in retrieved segments are necessary to limit the search to the responses just defined.
Figure 5: Basic lexical search to locate a common word or phrase
Click on run search to generate the output, which is illustrated in Figure 6.
The output shows that 26 texts were found in the activated group which contained the word "environment". By clicking on any line in the search results window, the full text for that respondent is brought up in the text browser panel where it can be checked for accuracy or relevance to the expected code. It is not essential to check all of the hits at this stage but it is worth making sure that your search has made sense by looking at a few of them.
Figure 6: Lexical search output
The next step will be to click on the green coding icon in the search results window to pull-up the autocode dialogue shown in Figure 7. However, before doing this it is worth clicking once on the code label (in the code system panel on the left) for the appropriate code (in this example "EA") as this will make it available in the autocode dialogue.
Figure 7: Autocode the results of a lexical search
It was the click on the code "EA" before commencing the autocode which has placed that as the expected code for this dialogue. If the code you want to use is not listed in the drop-down menu it will be necessary to cancel this command, click on the required code in the Code System panel (or create it if necessary) and then restart the autocode process. It is not necessary to close the search results window whilst doing this. For this example in the lower part of the dialogue we have chosen to autocode the whole paragraph containing the search word but shorter or longer coding options are also available. A click on the autocode button now completes the sequence of operations resulting in 26 responses being coded at once.
TipThe decision as to how much text should be included in the autocode should be considered carefully. In contrast to some other CAQDAS packages there is no penalty in terms of loss of a link to the respondent’s ID when short passages are coded, because MAXqda will always include the relevant Text number in outputs and reports. For on-screen enquiries it will always be possible to view the attributes of the respondent who contributed any coded segment under review. But by extending the code to the paragraph, as indicated here, it will be possible to read the full context of the response within which a theme has been coded. On the other hand, if your data is especially rich and you may want to investigate whether one theme is generally mentioned before another, then it will be advisable to restrict the autocoding to more precisely determined segments. |
Before moving on you should now check the accuracy of the coding just carried out. Reset the activations in the code system window (a single icon click achieves this) then activate the code you have just used ("EA" in our example) to bring all of the items allocated to that code into the retrieved segments panel. You can now read each of the texts in turn to satisfy yourself that they have been correctly coded, and you have the option of removing the code from any responses where the automatic allocation was incorrect (for example in our illustration if the word "environment" had been used in some other sense than a reference to the Environment Agency).
It is unlikely that a single search will exhaust the potential autocoding for one code, and the process can be repeated with variations on the search theme. To continue this example we searched on the word "agency" and found two more relevant responses which had used that word but not "environment". Clearly, ideas for further lexical searches may be found in the word frequency tables suggested earlier, and those tables also indicate the variations in spelling which should be included in the searches.
TipSome users find it productive to export the Word Frequency table to a spreadsheet (like MS Excel) in order to carry out more powerful sorting and grouping routines to identify related words, and this can be seen as a way of extending the rigour of the analysis. |
However, it is not necessary to run a separate search and autocode procedure for each possible word. Figure 8, below, shows how several words or phrases can be combined in a single search.
In Figure 8 four separate strings are set up for the search, and they have been combined with the "OR" function so that a hit will be located if any one of these strings is found. The check box "Find whole words" has been ticked to catch references to the Environment Agency by its acronym while excluding the many irrelevant words in which the letters ea may be found together. However this setting may prevent this search from locating variations to the other search words (for example "environmental"), but this can be accommodated by using the ‘*’ wildcard extension (which works in conjunction with the whole words setting).
When multiple strings have been used in this way the search results window shows which word or phrase has been located in each hit and indicates the number of texts which contain them without double counting the occasions when two or more terms appear in the same text (such as "environment agency").
Figure 8: Complex lexical search to locate multiple words or phrases
The autocoding process will not complete the task if data reduction to accurate quantities of references is the goal of the analysis (see section 4 below). Our searches above did not locate the responses which made reference to "floodline pack" or "rivers authority" which were eventually coded to the same "EA" code following careful reading of the full texts. So a combination of autocoding and human interpretation is needed to achieve a high level of accuracy. But time can undoubtedly be saved through the use of well-directed search and autocoding routines.
4. Coding - data indexing versus data reduction
The actual techniques of manually applying codes to segments of text are not discussed here. They are common to all applications of the program and are clearly explained in MAXqda’s help manual and in other sources. However, the possible uses to which the analysis of responses to open-ended survey questions may be put is a matter worth discussing further.
As a coding scheme is developed and applied to textual data, the analyst will inevitably encounter uncertainty and doubt. Does the text in front of me represent something different from others I have read before which mentioned a particular keyword? A common solution to this is to be generous and inclusive, applying specific codes to a range of comments that initially appear to be connected to those concepts, with the good intention of returning later and checking the work. This activity may be described as "data indexing" as it facilitates the retrieval of various passages that appear to relate to a particular topic.
When open-ended questions have been asked in survey situations it may be appropriate to generate numerical summaries of the data, probably in the form of statements of the type "X% of responses to this question mentioned Y". The obvious source of the numbers for this output is the coding of concept "Y". However the statement will only be valid if the use of that concept in every one of the responses allocated that code is consistent and equivalent, because the code that is used in this way has effectively replaced the words recorded for each respondent. The original textual data has been reduced to the code label.
When put this way it should be apparent that work needs to be done to refine the inclusive indexing codes before they can be safely used as summarising reducing codes. In this example data one respondent answered the question about the advice they had received by saying "... move self and belongings upstairs. Contact floodline. I think there were a few tags to put on things in the kitchen. The pack itself was very well done and well thought out ..." while another dismissed this as "... a flood pack stating obvious, silly stickers ...". Initial index coding may have allocated a code "given flood pack" to both of these passages but it could be potentially misleading to include both in a percentage of respondents who referred to the flood packs as though these comments are equivalent to each other.
5. Checking summarising codes - consistency and omissions
There are a variety of tools in MAXqda to assist with the refinement of codes when they have to be reduced to summarise what was originally said. Two particular aspects should be considered, firstly confirmation that all of the passages connected to any one code are all sufficiently similar to be treated as equivalent, and secondly confirmation that no other passages that are also equivalent have been omitted from that code.
The first step in confirming consistency or equivalence is to extract all of the passages that have been allocated to a code and read them carefully. In MAXqda a retrieval of this kind is achieved by activating all of the texts and just the specific code that is being checked. If the code has only been used in connection with a single question then this retrieval can be achieved using mouse clicks in the code system panel. However, if the code has been used with more than one question and the requirement is to confirm its consistent use within a single question’s responses then a more complex text retrieval operation is necessary. First activate all of the texts, the specific code to be checked, and the code for the question to which the check is to be limited. Then use the analysis / text retrieval option (or the fx button on the main toolbar) and change the function if necessary to intersection by using the drop-down menu. Next click on the all activated codes button to bring the required codes into window A of the dialogue box. The execute command should then put these operations into effect and in the retrieved segments panel display just the passages from the selected question that have been coded to the selected code.
When checking all of the passages linked to a single code it should be possible to write a concise definition of that code. MAXqda provides a memo function for each code and this is the ideal location to store that definition. A right click on the code label in the code system panel brings up a context menu, select the command code memo from this menu, and type the definition into the large text space in the lower part of the dialogue box. If the code has been applied in part by using autocoding routines then the specific words used in those routines should be listed here. When this has been done a yellow box is added to the line for that code in the code system panel and subsequently by moving the mouse pointer over this box you can bring up a temporary display of the code definition.
TipIf you find it difficult to write a concise definition of a code then it may be inferred that you should not refer to the number of references to that code in any data reducing statements. |
It is more difficult to search for code omissions, these are passages which are closely equivalent to those already allocated to a particular code but which have not yet been allocated themselves. One possible method for doing this is to filter-out all of the segments which have been allocated the code and then identify passages in the remaining data which may be equivalent.
TipThe way this is done probably varies according to the way in which you have coded initially. If you have used autocoding routines to apply the code it would be good practice to read the remaining passages as with manual coding, but if you have initially applied codes manually then it may be appropriate to use text searches using key words connected to that code on the remaining text passages to confirm the accuracy of those procedures. |
To filter-out the segments which have been allocated to a particular code it is necessary to use the fx function a little differently. We will use the example of the "EA" code described separately in the autocoding section of this page (section 3); to filter-out the responses already coded to EA, activate all of the texts, the question QADVI2 and the code EA, then select the text retrieval function (fx) to bring up a dialogue box similar to that in Figure 9. Change the function in the top part of the dialogue to if outside, select all activated codes to bring both the question and the thematic code into window A, and then highlight the thematic code and click on the remove button to take it out again. In window B click on the ... button and select the thematic code in that list. The dialogue should now look something like Figure 9 below. Note in this illustration how the Result bar shows "segments found: 296", and this is the difference between the 324 segments in QADVI2 and the 28 segments coded to EA (both visible in the Code System panel in Figure 9). Click on the execute button to apply the filter.
Figure 9: Filter-out responses to QADV12 already coded to EA
With the coded segments filtered out, it is possible to run repeated lexical searches on the remaining retrieved segments for key words that may be associated with the thematic code being checked (see instructions in section 3 above for lexical searching). Zero returns, or no "hits", are satisfactory as they confirm that no texts including the search term have been found that are not already coded to that theme.
TipIt may be even quicker to run a word frequency calculation on the remaining retrieved segments and review that for key words, again the absence of these words in the results would be satisfactory. |
6. Looking for similarities or differences?
When analysing the responses to open ended survey questions it may well be easy to slip into the expectation that the most frequently used codes, or rather the concepts to which they refer, are the most significant. After all, these are the items that seem to have the most statistical importance. However, it should always be worth looking out for contributions which are different from the common ideas. One-off comments will never feature in the quantitative tables because, by definition, they lack numerical support. But a small number of individuals may well take the opportunity of an open-ended question to add an unexpected thought and these contributions represent a challenge and an opportunity for the analyst.
Perhaps it is worth asking yourself what was the purpose behind the inclusion of an open ended question in the survey. In many situations previous research will have revealed the most likely answers and these will have been included as response categories in closed questions asked elsewhere in the survey, but then an open question has been included to pick up other ideas. In these situations it is the unusual answers which may be of most interest.
For example in the data used as an example for these instructions the question QADVI2 was used to ask respondents what advice they had been given in order to prepare themselves for an impending flood about which they had been warned. It may be interesting to note that out of 324 responses to this question, just three people mentioned warm clothing or blankets. Now it may be the case that for most people the need for warm clothing as you sit out a flood in an upstairs room is too obvious to be worth mentioning, but this may also be a clue that there was a potentially significant gap in the advice actually given to the flood victims in the incidents under consideration. It seems that the value of a detailed qualitative analysis of the responses to such a question is an opportunity to pick up the unexpected ideas which would be so easily overlooked in a statistical analysis.
Summary
There are several step-by-step instructions in the above materials. These have been included to help those who are not familiar with certain aspects of the way MAXqda works. However this is not intended to imply that these are the correct / only / best ways of analysing the responses to open-ended survey questions in MAXqda. These are merely examples of procedures that do work, particularly with data of the sort shown in the examples. However it will always be the case that different data may require different procedures, but we hope that these examples will help some analysts to get over the problem of using unfamiliar software or of using familiar software in an unfamiliar way.
1. Reading the texts – by respondent or by question?
It is possible in NVivo to work with the survey responses in either presentation – all the answers given by each respondent in turn, or all of the responses to one question at a time. However, depending on the way the data was formatted before being imported, one way will be much easier to implement than the other. Please see the document per case section for a discussion of the advantages and disadvantages of each view in various circumstances.
If the data has been formatted and imported in the way suggested by the data preparation instructions on this page then it will be a simple matter to click on source / internals and double click on a document name to see all of the responses to that one question in the detailed view pane. This layout lends itself to the process of reading and coding those responses to themes taken from the data itself. The texts in the detail view pane can be scrolled easily to move forwards and backwards through the data for that question. The respondent who has provided each answer should be clearly visible through the format in which the data was prepared (see Figure 1 for an illustration of this layout).
Figure 1: A basic working screen layout in NVivo 8 showing the responses to one question
Even when the data has been grouped by question it is still possible to view the data grouped by respondent instead.
Select nodes / cases, then open the full list of cases by clicking on the + button beside the header case, and then double click on a single case ID to see all of their responses in the detailed view pane.
The questions for which responses have been collected are visible as document names just above each separate response. This is illustrated in Figure 2. There is more distracting information in the display than is found with the internal source method at Figure 1, and it takes a certain amount of trouble to generate the display for the next case, so this may prove an unsatisfactory basis for detailed analysis and coding work.
TipIf you find that you often want to look at the data in this way you may find it useful to create and save a coding query instead. On the coding criteria / simple tab highlight the "node" button and then use the "select" function to navigate to the required case. |
Figure 2: Responses per case in NVivo 8 – using the query method
2. Developing a coding scheme – manually or by using word frequency tools?
The nature of the analytic strategy will affect whether it is appropriate to develop a coding scheme manually or by using word frequency tools. If you are working deductively a coding scheme may be derived from, or informed by, existing (theoretical) frameworks; in these situations the following comments will not really be relevant. If, on the other hand, you are working inductively and therefore intend to generate coding categories from the ideas mentioned in the response texts themselves then you have a choice as to how to proceed. You may work ‘manually’ by reading the texts and choosing categories that seem to be mentioned in those texts or alternatively let the software help by creating a list of the most frequently used words in the texts and allow code development to be informed by this list.
The manual, or maybe that could be termed "human", method will be required at some stage if really accurate coding is needed, because only human readers can detect all of the subtleties of human expression involving multiple ways of phrasing any particular idea.
However to get started, particularly in a large dataset, it should be worth trying the word count method to get an early idea of the range and salience of words used. The most frequently used words may be expected to provide indications of the most frequently expressed concepts, although multiple possible meanings for some words can complicate this assumption.
Word frequency tools
For NVivo the word count function is found as a word frequency query, either under the new menu (Figure 3) or as a previously saved query.
Figure 3: Start a new word frequency query
The dialogue screen that follows requires some care and attention (see Figure 4 below).
The first field should be set to limit the search to text (ie excluding annotations).
The second field is where the specific document (or question, in the terms of this data) is selected, it has two parts: from the drop-down menu choose selected Items, and then click on the select button to bring up a separate dialogue box where you can navigate to the internal source document with the responses to be analysed and close that with an OK button.
The default setting for the where field of created or modified by any user is probably correct.
It is a matter of judgement how you set the parameters for display words, depending on the nature and volume of your data – in this example we have set these to show the 100 most frequently occurring words of 5 characters or more in length.
TipYou may need to experiment with a variety of settings for these parameters to find what is most effective with your data, and to make your life easier in that process it may be worth ticking the box to add the query to your project before hitting the run button for the first time |
Figure 4: Word frequency set up
Word frequency outputs
NVivo has two possible displays of the output from this query, a basic list of words and the number of times they have been found in the selected document, and a "tag cloud" where the words are listed in alphabetical order but with font size proportional to their frequency, as illustrated in Figure 5 below. The two presentations can be viewed alternately by clicking on the tabs "summary" or "tag cloud" at the right hand side of the panel. In the summary presentation (see figure 6 below) the list can be sorted in ascending or descending order for any of the columns (alphabetical, length or count), controlled by clicking on the column header area.
Not all of the results are meaningful as indications of useful concepts, for example the fifth most frequently used word in this illustration (Figure 6) was "would", used in several different senses of meaning and thus not lending itself to any particular thematic code, so judgement will still be needed to select coding categories, but some useful information can be seen very quickly. This data is about better ways to warn people when a flood is imminent and the prominence of words like "local", "police" and "telephone" is interesting. Note, also, how "loudspeaker" has been split into two separate words, with its plural version being used sometimes, apparently halving its prominence in the tag cloud.
TipIt may be interesting to look at the longest words by sorting on the word length column in the summary display for some complex ideas. In our data it seemed that the tag cloud worked better with a longer minimum word length, but this may have eliminated several important shorter words,so flexibility and imagination should be brought to bear on the choices. |
Figure 5: Word frequency count – tag cloud display output
View selected words in context
If you want to see all of the instances in which a particular word has been used, possibly in order to assess its suitability as a thematic code, this can be done from the word frequency report. In either the summary or the tag cloud view, a double-click on a word in the list will start the generation of a node preview report, and this will appear under a new tab in the detail pane. There will be an item in this report for each occasion that the selected word was found in the document, the keyword will be shown in bold font with several words of context before and after it (the number of context words is set with the ‘narrow’ context option under tools / options). Figure 6 below shows a summary list from a word frequency query in the main detail panel, with a node preview extracted from that report for the word "siren" in an undocked window to the right. In the node preview 5 of the 14 instances in which that word was used are visible (numbered 8 to 12). The narrow context was set to 8 words, sometimes this includes the respondent’s ID and even the beginning of the next response.
Figure 6: Word frequency summary and node preview (undocked)
Developing a coding scheme
By studying the tag cloud, summary outputs and node previews it should be possible to gain some ideas about the most commonly used terms in the text, and these are the obvious starting points for building a coding scheme. If the word frequency outputs are not providing helpful suggestions then you should revert to the manual approach by reading the responses, thinking about their content, and applying codes directly in the traditional manner.
By default, NVivo applies a "stoplist" of 33 short words to be excluded from word frequency calculations. This list cannot be edited by users, although it can be disabled so that no words are excluded. Details of the words in that list and how to disable it can be found in the online help section under "queries and results / types of queries / word frequencies" in the final section under the heading "about the results".
As you develop the ideas for your coding scheme you should also consider an important aspect of its structure. Do you want to create a separate set of codes for each question in your data or have a common coding scheme across all questions? There is no clear solution to this problem as it depends on your own preferences and working practices, the nature of your data and your analytic approach. If the survey questions were largely unrelated to each other, perhaps because they were widely spaced apart at different points in the questionnaire, then a separate group of subcodes for each question may be suitable. However if you expect to be interested in the way some themes arose across multiple questions, then having a more unified coding structure may be more suitable. It is a straightforward operation in NVivo to use the tree nodes area for a hierarchical structure if you decide to create a group of codes for each question in your data.
3. Text finding, searching and autocoding
Finding a word
Another way to see one of your selected key words in its full context is to use the find option. This can be found under the edit menu or by using the binoculars icon on the edit toolbar. You may need to click the cursor into the open source document before the option becomes available, and this function only looks in the open document. The dialogue screen for this is illustrated at Figure 7.
In this example we are searching for "phone", the style setting is not relevant and so the default of "any" is fine. In the options section the "text" setting for "look in" limits the search to the document itself (excluding annotations), and the setting for "search" defines the direction of the search (up, down and all being the choices – up and down searches stop when the program reaches either end of the document, but if you start a search in the middle of a document only the all setting will continue past the end so that the whole document is searched). "Match case" is unlikely to be useful with this sort of data as the interviewers who typed these responses may have been erratic in their use of capital letters. You should probably experiment with the tick for "find whole word" – in this example leaving it unchecked means that "telephone" and "phones" are both found by the search – sometimes this is what you need but sometimes it is not. You may need to drag this dialogue box to one side of the screen so that it does not obscure the texts you are examining, because you will have to click on "find next" each time you want to move on to another hit.
Figure 7: Find options
Using these "find" searches can help you to see a frequently used word in its context throughout the set of responses, and this should help you to determine whether it merits being used as a code (or "node" in NVivo terminology).
In many cases you may be happy to use a manual procedure to apply the code to each occurrence in turn, using the repeated find next button to move to the next after each coding operation. However, if you have a very large number of responses it may be worth using a facility to code all of the occurrences of a particular word with a single instruction.
Text searching tools
Although most qualitative analysts will naturally prefer to do all coding work manually it is quite reasonable in some circumstances to use NVivo’s autocoding process. For example quite a lot of responses may be simply "don’t know" because the open-question has not triggered a specific response. Coding such material individually would be tedious work but by running a text search query (probably first with and then without the apostrophe) and using the query options functions these can be allocated to a node quickly and efficiently. With practice more positive common concepts may be identified and also rapidly coded in this way. It should be easier to follow this procedure and then eliminate any incorrect codings, following a consistency check, than to code a large number of very similar statements manually.
This is initially started using the drop-down beside "new" on the main toolbar and selecting "new - text search query".
Figure 8 shows the first stage of creating such a query, the same example word "phone" has been used here as the text to search for. Once again the search is limited to text (ie excluding annotations), but in this option it is necessary to specify which document is to be searched (unlike the Find option which is automatically restricted to the open one).
TipNote in Figure 8 how the "stemmed search" box has been ticked, unfortunately this is not as flexible as the Find option so whilst "phones" will probably be found with this instruction, "telephone" will not be found. It is possible to combine multiple words in the search expression, this will be discussed below but, for the moment, we will keep to a simple term in order to establish the procedure clearly. |
Figure 8: Autocoding from a text search – part 1
Autocoding set-up
Clicking on the "query options" tab opens up the second part of this instruction, as shown in Figure 9. This is where the autocoding element comes in - in the results section of this dialogue box, use the drop-down menu to select a coding option. If there is no existing node for "phone" then you can create a new one (as shown here), however when you re-run the search to add instances of the word "telephone" by altering the "search for" text on the other tab you will need to change this to "merge results into existing node". Then, depending on whether you are creating a new node or merging with an existing one, you either select a location and type in the new name, or select a name (which will have a location already fixed). The description is not essential but is good practice, especially when a code is less closely tied to obvious words.
The "spread coding" section requires more thought. The relevant choices offered in the drop-down menu are: "narrow context", "broad context" and "custom context". The first two of these are defined at a system level, so you may need to close this dialogue and re-set those before trying again. However the custom context option has the same selection choices and so this is a good way to experiment until you find the settings that work best for you and your data.
TipThe decision as to how much text should be included in the autocode should be considered carefully. This controls what you will see when you extract all the passages to which a particular code has been applied. To some extent it depends on how long and rich your response data is, with the quite brief comments in our example data we decided to code the whole response to each applicable code so that we would always be able to see the full context. But if you have quite long and detailed responses then it may be better to limit the codes to a shorter range. This will affect the ease with which you can make subsequent qualitative judgements about the language used by subgroups of respondents, and may affect the counting of code usage if it leads to multiple code applications within some responses. |
Figure 9: Autocoding from a text search - part 2
Autocoding results display
Note also that the "open results" box has been ticked in this example. This means that, when the "run" button is clicked, a new tabbed page will be opened in the detail pane showing the hits that have been coded to this node. The display that will appear is subtly different from that which would be created later by simply opening the telephone node, and this is illustrated in Figure 10. For this example the setting for "broad context" was "surrounding paragraph" and the setting for "narrow context" was "8 words". Note how this report displays the paragraph containing the search word in black text (the "broad context") and also up to 8 words before and 8 words after that paragraph in grey text (the "narrow context"). By turning on the coding stripes for the relevant node it can be seen that only the core paragraph has been coded.
Figure 10: Results following an autocoding query
An important point to note here is that the IDs for the responses have not been included in the coded text. In this display, run as part of the autocoding query, although it is possible to see those IDs because they are in the narrow context extensions, when a code listing for this node is generated from the nodes folder it will only show the text that has been coded. This represents a disadvantage arising from using this method to code responses as it may make it more difficult to locate a specific response at a later stage, for example to relate it to the respondent’s socio-demographic attributes.
TipWe have observed that displaying a node by clicking on its name in the List pane during the same working session as that in which the node was created by autocoding will bring up the extended contexts again, but when the project has been saved and closed then subsequent views of that node will only show the coded text. However, you can then open the source document by clicking on the "text" tab at the right-hand side of the detailed view pane and then double-clicking on the appropriate document icon that will appear in the upper portion of that pane. That document will open in full in a new tab with the relevant coded passages highlighted in it. |
Before moving on you should now check the accuracy of the coding just carried out. Read each coded text in the results display carefully to satisfy yourself that they have been correctly coded. If a text is found to have been coded incorrectly this can be adjusted by highlighting it and clicking on the"uncode" button on the coding toolbar.
Advanced searching
It is unlikely that a single search will exhaust the potential autocoding for one code, and the process can be repeated with variations on the search theme, or multiple terms can be used within a single search and autocode procedure. To continue this example we searched for "telephone" which coded a further four responses, other potentially relevant words to try might be "ring" and "call", although these may generate some incorrect codings as in "ring the church bell" or "call at the door". Ideas for further text searches may be found from the word frequency tables suggested earlier, and those tables should also indicate the variations in spelling which should be included in the searches.
Several words and variations of spelling can be included in one search by using the "special" button on the text search criteria tab shown above in Figure 8. Different words which have equivalent meanings, such as "loudspeaker" and "loudhailer" can be combined with the "OR" operator, and the usual wildcard characters are also available. Care may be necessary to reduce incorrect hits, for example whilst "*phone" will find ‘telephone’ it will also find ‘microphone’ which is probably not an equivalent term in this data.
TipNVivo is a little unhelpful when a series of searches is used with a single node because, after each subsequent search and autocode, the full list of references for that node are displayed, and so it becomes increasingly difficult to identify the new additions from the latest run. If this is a significant problem it may be worth considering creating a new node for each search and then, when they have been checked for accuracy and relevance, merging all these similar nodes into a single node. |
The autocoding process will not complete the task if data reduction to accurate quantities of references is the goal of the analysis (see section 4 below), because of the variety of ways in which many ideas can be expressed. But some time can undoubtedly be saved through the use of well-directed search and autocoding routines.
4. Coding – data indexing versus data reduction
The actual techniques of manually applying codes to segments of text are not discussed here. They are common to all applications of the program and are clearly explained in NVivo’s help manual and in other sources. However, the possible uses to which the analysis of responses to open-ended survey questions may be put is a matter worth discussing further.
As a coding scheme is developed and applied to textual data, the analyst will inevitably encounter uncertainty and doubt. Does the text in front of me represent something different from others I have read before which mentioned a particular keyword? A common solution to this is to be generous and inclusive, applying specific codes to a range of comments that initially appear to be connected to those concepts, with the good intention of returning later and checking the work. This activity may be described as "data indexing" as it facilitates the retrieval of various passages that appear to relate to a particular topic.
When open-ended questions have been asked in survey situations it may be appropriate to generate numerical summaries of the data, probably in the form of statements of the type "X% of responses to this question mentioned Y". The obvious source of the numbers for this output is the coding of concept "Y". However the statement will only be valid if the use of that concept in every one of the responses allocated that code is consistent and equivalent, because the code that is used in this way has effectively replaced the words recorded for each respondent. The original textual data has been reduced to the code label.
When put this way it should be apparent that work needs to be done by the analyst to refine the inclusive indexing codes before they can be safely used as summarising reducing codes. In this example data one respondent said "I liked the automated calls" but another commented "the recorded message isn’t very effective". Initial index coding may have allocated a code "automated messages" to both of these passages but it would be potentially misleading to include both in the percentage of respondents who preferred an automated telephone warning system.
5. Checking summarising codes – consistency and omissions
There are a variety of tools in NVivo to assist with the refinement of codes when they have to be reduced to summarise what was originally said. Two particular aspects should be considered, firstly confirmation that all of the passages connected to any one code are all sufficiently similar to be treated as equivalent, and secondly confirmation that no other passages that are also equivalent have been omitted from that code.
The first step in confirming consistency or equivalence is to extract all of the passages that have been allocated to a code and read them carefully looking for differences of meaning that might justify exclusion from that group. Extra care may be needed if the code has been used with responses to more than one question.
If a code has only been used with a single question then its references can be viewed easily by clicking on that node in the list pane for the nodes section. This command will open the detailed display of references in the detail pane where they can be scrolled backwards and forwards to read and compare. If a hard copy is preferred it can be generated by selecting the print command whilst this output is open in the detail pane.
However, if one node has been used to code the responses to two or more questions then it may be advisable to use a coding query to generate a targeted output for consideration. From the new drop-down menu select new coding query. The simple tab should provide sufficient criteria for this task. Select the relevant node in the top part of this dialogue and the source that holds the responses to the relevant question further down the screen. When the Run command is triggered the detail pane will display the references for that combination of node and source. Again this can be printed by selecting the print command.
After checking all of the passages linked to a single code it should be possible to write a concise definition of that code. In NVivo this can be typed into the properties box for that node. In the list pane for the nodes section, right-click on the relevant node name and select free node properties (or tree node properties if applicable), then type the definition into the description field. If you find it difficult to write a concise definition of a code then it may be inferred that you should not refer to the number of references to that code in any data reducing statements.
It is more difficult to search for code omissions, these are passages which are closely equivalent to those already allocated to a particular code but which have not yet been allocated themselves. One possible method for doing this is to filter-out all of the segments which have been allocated the code and then carry out a series of text searches using key words connected to that code on the remaining text passages. In NVivo this can be done by using a compound query, see an illustration in Figure 11.
Figure 11: Compound query to find coding omissions
Using the new drop-down menu select a new compound query, as shown in Figure 11 above. The first subquery should be a text search where the criteria button is used to enter possible key words associated with the relevant code. The second subquery should be a coding query, set to the relevant code. And these two should be linked by the "AND NOT" option. Thus this compound query asks for content containing the specified text in passages that have not been coded to the specified code. Finally it will probably be necessary to limit the query to the relevant source document by selecting that at the “In” field. On the Query Options tab it is useful to set the spread to “Broad context” to help consider the meaning of any positive results. It would be useful to tick the box to add this query to the project because it will need to be run multiple times with variations of the text to be searched on, and that would be easier to edit without having to reset all the other criteria for each run.
For this sort of query an empty result represents a success, because it means that the search text has not been found in any relevant passages that have not been coded. Any positive results will need to be investigated carefully as they potentially represent omitted references to that code.
Clearly, if the original coding was done by using text searches and autocoding routines as described in section 3 above, there will be little point in repeating those queries at this stage as a check. However, if the main coding work was done manually, that is to say by reading the responses and selecting appropriate codes by hand, then this automated checking procedure should provide a worthwhile check on the accuracy of those human decision processes. In this latter situation the Word Frequency Count information illustrated in Section 2 above may provide useful clues for the texts to be used as criteria in the compound query.
Each of these processes may seem to involve a lot of work, so judgement will be necessary to decide how much is appropriate. These checks are important if you are going to use the code frequencies in any statistical analysis or reporting, they are not so significant if you are merely indexing the ideas in your data. If you started with a clear coding scheme and precise definitions of the codes before you began interpreting the texts then you may be more confident that you have applied the codes consistently and accurately. However, if you have worked more inductively, gradually refining the meanings and uses of the codes with ideas found within the texts, then you are more likely to have inconsistencies between the coding you did on earlier readings and those you did later. It is also important to check for these types of error if more than one person has been involved in coding any particular set of texts.
6. Looking for similarities or differences?
When analysing the responses to open ended survey questions it may well be easy to slip into the expectation that the most frequently used codes, or rather the concepts to which they refer, are the most important. After all, these are the items that seem to have the most interpretive and statistical ‘weight’. However, it should always be worth looking out for contributions which are different from the common ideas. One-off comments will never feature in the quantitative tables because, by definition, they lack numerical support. But a small number of individuals may well take the opportunity of an open-ended question to add an unexpected thought and these contributions represent a challenge and an opportunity for the analyst.
It is worth considering what the purpose behind the inclusion of an open ended question in the survey was. In many situations previous research will have revealed the most likely answers and these will have been included as response categories in closed questions asked elsewhere in the survey, but then an open question has been included to pick up other ideas. In these situations it is the unusual answers which may be of most interest. It is for this reason that it is worth analysing the open-ended questions systematically.
For instance, in the data used as an example for these instructions the question QBETT2 was used to ask people what better ways they could think of to warn people when a flood is likely to happen. It may be interesting to note that, out of 229 responses to this question, just four mentioned the problem of previous warnings that had not been followed by actual flooding leading to the important warning being ignored, the so-called “crying wolf” scenario. Maybe more people had this experience but not everybody is brave enough to admit that they ignored a valid warning. Many more people said that they would like to have had an earlier warning and more time to prepare for the flood, but if this obvious response is acted on then it seems likely that there will be more false alarms and a danger that the whole warning system falls into disrepute. It seems that the value of a detailed qualitative analysis of the responses to such a question is an opportunity to pick up the unexpected ideas which would be so easily overlooked in a statistical analysis.
Such uncommon ideas may be found by checking the codes which have low frequencies, or by creating an extra code during a manual coding process specifically to identify them – maybe named "unusual" or even "Z unusual" to anchor it at the bottom of the coding list.
Conclusions
There are many lengthy step-by-step instructions in the above materials. These have been included to help those who are not familiar with certain aspects of the way NVivo works. However this is not intended to imply that these are the correct/only/best ways of analysing the responses to open-ended survey questions in NVivo. These are merely examples of procedures that do work, particularly with data of the sort shown in the examples. However it will always be the case that different data may require different procedures, but we hope that these examples will help some analysts to get over the problem of using unfamiliar software or of using familiar software in an unfamiliar way.
1. Reading the texts – by respondent or by question?
QDA Miner offers several different ways of approaching this sort of data and the final decision of how to do this will be determined by personal preference and analytic approach.
Figure 1 shows the main working screen (with the coding panel temporarily closed). In this display the user can select any respondent by clicking on their line in the cases panel (here case #34 has been selected). The variables panel beneath that has been expanded to show all the variables that have been brought into this project, and those with response texts can be identified by the word "[document]" here, where this word is in capital letters there is a text response in the dataset but where it is in lower case then there is no response. To read any of these texts it is necessary to click on the appropriate tab label at the top of the documents panel (here "QMORE" has been clicked and shows up in bold font). So the text on display is the response to question "QMORE" made by case #34.
Now by clicking on the other tab labels (particularly those with "[DOCUMENT]" in capitals in the variables panel) one can read each of the responses provided by this case. Or, leaving "QMORE" in bold, either by clicking on the forward and back scrolling arrows in the lowest toolbar or on the cases labels instead one can read each of the responses to this question. The choice to work by case or by question is completely open (see document per case vs document per question section above).
Figure 1: Main display screen
However, where there are many gaps in the responses, because most respondents only answered a few of the open questions, this approach will be frustrating with many empty screens being seen. In this situation a text retrieval report may be found to be a more satisfactory alternative.
The text retrieval report is found under the analyse main menu. First let us consider using this to generate a list of all the responses to a single question. In Figure 2 just three settings are required in the dialog box for this operation: beside "search in" use the drop-down menu to select the document label for the required question, set the "search unit" to "paragraphs", and click the radio button beside "retrieve all units". Hit the "search" button to see the report within the same window. Note how "search expression" and "search hits" are the two tab labels within this window, it is easy to switch back and forth between these to try different settings and see their effects.
Figure 2: Text retrieval dialog settings to view all responses to QMORE
Figure 3 shows an example report for the document "QMORE" placed over the main working window. The query has found 361 hits, so these represent the full set of responses to this single question. A tick has been placed in the “multilines grid” box just above the results list, and this forces the complete display of the longer texts (such as that by case #26). It will be important to be able to read complete texts to be sure that nothing is missed in the analysis. Note also that the report window is synchronised with the main screen so, when an item in the report window is selected, the main screen behind it will change to display that response and the variables panel will display the attributes for that respondent.
Figure 3: Text retrieval report on document QMORE – responses for one question
Secondly, if you prefer to read all of the responses made by each respondent, then select all of the documents at the "search in" setting on the "search expression" screen (Figure 2). You will get a longer report, but it will be sorted by case number before variable. Figure 4 shows an illustration of this using the same dataset as before. Once again the search window is synchronised with the main window, so selecting one item in the search window brings up all of its related data in the main window, and codes can be applied to individual responses in the main window without closing the search window.
In both Figure 3 and Figure 4 the same particular response has been selected, that of case #32 to question QMORE. In Figure 3 we can easily compare what this respondent said with the responses to this question made by other cases. In Figure 4 we can easily read all of the responses made by this case to all of the questions, so #32 only answered three questions while #34 answered four questions.
Figure 4: Text retrieval report - responses from all respondents to all questions
Further, it is possible in QDA Miner to control the sequence in which cases are listed according to their values in a variable. The default, case number, is simply the order in which they were arranged in the spreadsheet from which the data were imported. By using the menu option cases / grouping / descriptor... the user can select one or two variables as grouping terms and also include those values in the display in the cases panel. These settings then apply to the text retrieval reports as well, so that they will be grouped according to the same variables. For example this facility might enable the analyst to read the responses to a question about flood warnings firstly by those who did receive a warning and then by those who were not warned.
2. Developing a coding scheme – manually or by using word frequencies?
Many analysis projects will be set up with a coding scheme derived from other work or sources; in these situations the following comments will not really be relevant. If, on the other hand, you are expecting to derive your coding categories from the ideas mentioned in the response texts themselves then you have a choice as to whether to do this by reading the texts and choosing categories that seem to be mentioned in those texts (I have termed this "manually" for want of a better term) or alternatively to let the software help by creating a list of the most frequently used words in the texts.
The manual method will be required at some stage if really accurate coding is needed, because only human readers can detect all of the subtleties of human expression involving multiple ways of phrasing any particular idea. However to get started, particularly in a large dataset, it should be worth trying the word count method to get an early idea of the range and density of words used. The most frequently used words may be expected to provide indications of the most frequently expressed concepts.
QDA Miner has a substantial extra module designed for automating the process of searching for equivalent meanings in multiple texts called "WordStat". This module is a sophisticated suite of content analysis programs with considerably more functionality than will be described here. However, as there is no word frequency function in the main QDA Miner program it will be necessary to use WordStat for that purpose.
Before looking in some detail at the use of WordStat it may be worth summarising three different approaches to this analysis challenge. If your approach is to be mainly deductive, because you have a good idea of the concepts that you are looking for (and maybe also the language in which they may be expressed) then you probably do not need the WordStat module, you can create the basic coding scheme first and then use various text retrieval strategies to identify the responses that those codes should be applied to. Secondly, if your data is not particularly ‘rich’ in detail (especially if it was heavily mediated by interviewers paraphrasing the responses as they typed them) but you wish to work inductively and develop the coding structure from the response data, then the text retrieval and query by example tools in QDA Miner may yet be sufficient for your purposes. The WordStat module really becomes useful when the language recorded in the data is likely to be more expressive and differentiating, or where a similar analysis is likely to be repeated on fresh data around the same topics from time to time (so that the effort of developing categorisation "dictionaries" is repaid with labour-saving efficiencies).
TipThe decision whether to use WordStat may have significant financial implications if you are considering purchasing the software for this analysis as, depending on the status of the purchasing organisation, WordStat may be almost as expensive or even more expensive than the QDA Miner program. This is why these instructions include some suggestions of analysis without the use of WordStat. |
Although most qualitative researchers may prefer to do the coding work manually, that is to say by reading each response and making a personal judgement as to which codes to apply to it, there will be a lot of scope for allocating codes automatically at the large scale end of the survey spectrum where QDA Miner comes into its own. These functions are available in the core QDA Miner program and in the WordStat add-on module and are described below with the other routines in which they are embedded.
3. Developing and applying a coding scheme in QDA Miner only
The initial, basic, approach to analysing responses to an open-ended question asked in a survey generally involves the analyst reading a sample of those responses and noting down the concepts which can be identified within that sub-set of the data. The list of concepts is then studied to see if it can be simplified by grouping some similar ideas together in fairly inclusive ways, and a systematic list of codes can then be developed from that list of grouped ideas.
If you are working manually, with only the main QDA Miner program to assist you, it will be important to note down the particular words that you notice in the data as indicators of the presence of a potential theme. One way of doing this is to have a blank sheet of paper on which you write down the useful words and to try to group those words along separate lines from the outset. So in our data we analysed a question that had asked what more advice people should have been given prior to the flooding event. One set of words that we noticed included the following "wash cuts, disinfectant, portable loo, boiling water, fill bath, contamination, health, safety" and these all seemed to indicate a possible theme connected with health. However these words did not appear neatly in the order just listed but were interspersed with other words that gradually built up other themes. Soon the blank sheet of paper was covered in webs of connections as a variety of themes emerged from the data.
The next step is to identify common labels that effectively describe each sub-group or theme, as these will become the code labels in the next phase of the work. It is important to recognise that these groups and labels may change as your understanding of the data grows, but you have to start somewhere. What you are doing is effectively the first stage of much content analysis, you are building "dictionaries" for use with the program. This use of the term "dictionary" is different from the common meaning because you are not attempting to define the meaning of each code label with precision, you are instead attempting to identify sets of words with similar or related meanings, an activity which is more commonly attached to the term "thesaurus". QDA Miner and WordStat use both of these terms in various places.
Having identified some themes, and a set of words found in the data that can be associated with each, from a subset of the data for one question, you are ready to use the program to explore these with the full response set for that question. There are three possible ways to proceed in QDA Miner – by using a text retrieval and thesaurus approach, by using a code and keyword retrieval approach, or by using a query by example approach – each will be described briefly below. Each has its own advantages and disadvantages and there is no reason why a combination of all three should not be used sometimes.
Text retrieval and thesaurus
We have already described the initial text retrieval process to extract all of the responses to one question. This procedure will now be taken a stage further. Start a new text retrieval from the analyse menu and select the question code required for the "search in:" field. Set the "search unit:" to "paragraphs" as before and leave the "uncoded text segments only" box empty. This time click in the radio button beside "search for text:" and then click on the red book symbol at the extreme right of that option field to open the thesaurus editor. Figure 5, below, shows an illustration of the thesaurus editor when it is first opened with the example data inserted by the program.
Figure 5: Thesaurus editor in QDA Miner
The program suggests four initial categories for illustrative purposes, "broken, earlier, exam, and good", here "earlier" has been selected and the suggested words for that category can be seen in the "content:" panel. Once you have grasped the idea you can delete these illustrative categories and start to create your own, by using the "delete" and "new" buttons on the left. To continue our earlier example, we created a new category for "health" and then added the words already identified with it into the "content:" panel to create Figure 6.
Figure 6: Creating new categories in the thesaurus editor
Note in Figure 6 how the underscore character has been used to create terms with two words and the asterisk has been used as a wild card character so that words like "boiled" and "boiling" will be picked up in addition to "boil". When the "OK" button is clicked the new category is added to the thesaurus, as can be seen in Figure 7.
Figure 7: a new category added to the thesaurus
Further words can be added to the category content at any future stage by using the "edit" button, which will reopen the category editor. At this stage more categories can be created as required by using the "new" button again. To use a category, highlight it in the thesaurus editor and click on the "insert" button to add the highlighted category to the text retrieval expression with the prefix "@". Running the search will then generate a list of hits, each containing at least one of the words or phrases listed in the category content field. Figure 8 shows the output achieved with this category in our example data.
Figure 8: Retrieval for category "health"
In Figure 8 it can be seen in the text column that the words in the selected thesaurus category have been highlighted in bold font and capital letters, and the number of highlighted items is shown for each response in the fifth column ("Nb hits"). The wild card characters worked successfully, but the phrase "fill_bath" was unsuccessful as it was not matched with the phrase "fill the bath" used by case #378, so some refinement of the category may be necessary ("fill_*_bath" would match both "fill a bath" and "fill the bath").
On reading closely we decided that the final hit in Figure 8 used the word "safe" in a different sense and so we did not want to code that with all of the other hits. It could be removed from the list of hits by selecting the row (case #1179) and clicking on the dustbin icon in the toolbar within the search hits window. Note that this does not delete any data from the project, it just excludes it from these search results. To code the remaining 10 hits with a single code, say called "health and safety", use the pull-down menu in this window to select an existing code, or click on the "+" icon to create a new code, and then click on the double highlighters icon (which becomes available when a code has been selected) to apply it.
TipNote that the code will be applied to whatever data you have asked to have listed in the search expression. In this example we asked for "paragraphs" as the search unit and so the full response has been extracted for each hit and the code will be applied to each applicable response in full. We could have asked for "sentence" and then the coding would have been applied just to each sentence that included one or more of the words in the thesaurus category. (Our data was made up of short statements so the paragraph unit is meaningful; in some other circumstances responses may be much longer, so there is an interaction between the data collection, preparation and analysis in this respect). |
TipIf more words are identified subsequently that relate to this code, they can be added to the category and the search can be repeated. Unfortunately, if you repeat the autocode QDA Miner will duplicate the coding in all of the previously coded hits, so you may need to check each hit separately and apply any subsequent coding more carefully. |
Code and keyword retrieval
This approach is essentially similar to the thesaurus approach described above but, instead of creating categories in the text search thesaurus, it uses the "keyword" field in each code definition. Figure 9 illustrates the dialog box that appears each time you create a new code in QDA Miner.
Figure 9: New code dialog box
Here a code has been created to capture comments about various types of vulnerable people within the coding group "more advice". The set of words that have been typed in the "keywords:" panel here can be used in a similar way to the set of words entered into a thesaurus category, and similar use can be made of wild card characters and underscores to create phrases.
To use the code keywords you need to apply the menu option analyse / keyword retrieval which brings up the dialog box shown in Figure 10, below. When the option is first selected the dialog box looks rather different as only the first field can be seen, but when "<internal keywords>" is selected from the pull-down menu in that field then the rest of this dialog comes into view.
Figure 10: Keyword retrieval
The main search parameters work similarly to those in the text retrieval dialog box and have been set here to search in the single question document "QMORE" and to return whole paragraphs. The keyword filtering section has then been used to select the "vulnerable" group from the list, and the default setting of "at least 1 of these keywords" accepted. When the search button is clicked the search hits will be displayed in a similar way to those of the text retrieval searches illustrated above, where they can be reviewed, any that are not relevant to the specific theme can be excluded, and the remainder coded in a single process as before.
TipIt may be asked what the difference is between the thesaurus approach and the keyword approach. The most significant difference concerns re-use of the categories. The keywords for a code are specific to that code in the current project alone and cannot be used anywhere else directly. The thesaurus categories are specific to the program set-up on the current computer and so could be used directly in another project as long as it is being run on the same computer. We understand that in the future there may be additional facilities to save and copy a thesaurus between computers, but that is not currently available in QDA Miner v3.2.4. |
Query by example
The third approach using functions available in QDA Miner alone uses the query by example routine which is found in the analyse menu. Figure 11, below, shows an illustration of the dialog box with an initial search text of "any advice got none at all" entered as the starting example. This text was chosen as typical of several responses in the reviewed sub-sample without precisely copying any of them.
Figure 11: Query by example – initial search text
When the "search" button was clicked the following screen appeared under the "search results" tab within the same dialog box.
Figure 12: Query by example – first iteration of results
The next stage of the process involves working down the dialog shown in Figure 12 and changing the question marks in the left margin to train the program so that it can identify other equivalent responses accurately. A single click on a question mark changes it to a green tick to mark a ‘relevant’ response, a double-click changes it to a red cross to indicate an ‘irrelevant’ response, and a third click cancels the cross and returns it to the indeterminate question mark. When a sufficient number of these hits has been so marked, you should click on the "search again" button (which becomes active once some hits have been marked) to re-run the search and obtain a more accurate set of results. This is an incremental process, in which you "teach" the program what you want and what you don’t want, so that it can find further similar responses to those that you have told it you are interested in.
TipIt is important to mark approximately as many crosses as ticks in this process, so that the program has some guidance as to what to exclude, otherwise it will add many more suggested hits because they include words similar to those in less relevant parts of the good hits. |
Figure 13: Query by example – first suggestions marked-up
Figure 13, above, shows the same initial page of suggestions during the marking-up phase. Of the responses on view, some 12 items appear to have much in common with each other around the theme of not having been given any advice. But three items have been excluded as irrelevant because they introduce different concepts ("help" and "sandbags") and do not refer to the common theme directly. The item highlighted in blue is still under consideration, one interpretation of it could be similar to the current theme on the grounds that this respondent did not receive advice before the flood as they seem to have got it afterwards, but, if we ‘tick’ this one as also relevant, the program will probably include other responses that include words like "afterwards" and "before" in the next iteration and that may not be helpful. On balance we decided to exclude this response and to develop another theme around the timing of advice separately from this theme of no advice being received.
The single page of suggestions shown in Figure 13 above is probably not sufficient for this process, so it is necessary to use the scroll bar in order to view and mark-up more suggestions before clicking on the "search again" button to get a second iteration of the query. Those responses that have already been marked retain their ticks or crosses after the second search but new suggestions will be inserted in the list and these should be read and marked as before. If this second iteration brings up a suggestion with a new word or phrase strongly associated with the current theme but not previously marked, then it will probably be worthwhile running a third iteration of the search, after ticking that response, to see if the program can find other responses with that word or phrase and a similar meaning.
It is necessary to continue marking-up the search hits until you reach the point where you are no longer finding any new relevant responses. It is not necessary to keep on marking crosses, unless you are planning to repeat the search in a further iteration of the process. But you do have to positively mark all of the hits that you wish to be coded and the purpose of this routine is to provide you with a list of responses that become progressively less similar to the examples you have chosen, so that you can judge when to stop marking. At that point you should click on the third tab label in the dialog box, "selected hits", to get a view similar to Figure 14, below.
Figure 14: Query by example – coding selected hits
Figure 14 illustrates the final stage of the procedure. It shows part of the list of 43 hits which had been ticked during the three iterations of the searching procedure, all of these hits can be checked with the help of the scroll bar on the right. Provided you are confident that your list is satisfactory then the whole list can be coded in one process. Either select an existing code with the pull-down menu for the "CODE:" field (the code "any" has been selected here) or create a new code by using the "+" icon in the toolbar, and then click on the double highlighter pen icon in the toolbar to apply that code to all of the selected hits (here to 43 responses).
TipIn this illustration we did not use two of the initial settings on the search criterion tab at Figure 11, so some brief comments about those may be useful here. The option to use "fuzzy string matching" may be useful in some situations. This allows the program to include more words that are similar to the initial text, for example misspellings and grammatical variations. This should broaden the coverage of the query and lead to more suggestions being offered, but it may also increase the burden on the analyst with more irrelevant hits to be marked off, so some experimentation with this option is recommended. Also there is a tick box option by "Do not retrieve already tagged segments" and this may be useful when you are repeating a query to extend a code’s application. The problem may be that the exclusion may be applied to a response which does not have the code being worked on (although it should be so coded) but which already has another unrelated code, so this may not be as helpful as it looks. |
It is, of course, also possible in QDA Miner to use the text search function to search directly for specific words or phrases entered in the "search for text:" field and then to apply a code to the set of results generated in that way. The disadvantage of this procedure may be the risk of duplicating the code application when subsequent searches are run for similar terms when two or more of these may occur in the same response. It is possible to use multiple terms as search parameters from the outset but the advantage of the thesaurus and keyword approaches is that a record is created of the words and phrases that have been used, and there are some possibilities of re-using these sets of terms with other data.
4. Developing and applying a coding scheme with WordStat
When the WordStat module has been installed its functions are loaded with the menu option analyse / content analysis within QDA Miner. A preliminary dialog screen requires the selection of the document variables to be analysed, probably one question should be analysed at a time so pick just one for now, at the next choice click by "all text" for a comprehensive review, and initially select the "... descriptive analysis only" option below that.
WordStat opens with a screen similar to that shown in Figure 15. This initial screen can be distinctly daunting, especially if you have a fairly simple dataset, because this is a sophisticated analysis program, but it can be used to run a simple word frequency routine.
Looking at Figure 15, the window can be divided into four horizontal sections; a set of six tabs at the top, a set of four tick boxes for the "dictionaries" element, the "dictionary viewer" part with two sub-tabs, and the bottom bar with some results statistics. As shown in Figure 15, remove any ticks from the boxes in the upper section, this makes the lower section with its "exclusion list" and "categorisation dictionary" tabs inoperative. Then, click on the "frequencies" tab in the top section and you will see a word frequency table for the document (or documents) that you selected at the start of this process.
TipLater, when you have become more familiar with this program, it may be useful to try ticking the "substitution:" box, with the "lemmatization" option selected, in order to bring together words with a common stem in order to see what effect this has on your subsequent work. |
TipAs a separate experiment, you could explore the effect of applying an Exclusion list. This is a list of words to be ignored by the program on the grounds that they are unlikely to be helpful in the analysis and may make it more difficult to see the important patterns. Words like "and", "the" and "at" may have little to contribute because they are so common that they are found in almost every response. |
Figure 15: Initial WordStat screen
For an example of the frequencies screen see Figure 17 below.
Because the option to use an exclusion dictionary was unchecked, the resulting word frequency table probably includes many words that do not help your analysis. However the default exclusion list is quite extensive and may remove words whose frequency you do wish to see. You can view the default exclusion list by clicking on the dictionaries tab at the top of the screen, and then on the exclusion list tab in the lower part of that screen ("dictionary viewer"). If you are going to use WordStat for more analyses of this sort, you may find it useful to build your own exclusion list (the icons at the right hand end of the exclusion line in the dictionaries section of Figure 15 control this).
One problem that probably arises with much open-ended survey question data is the mis-spelling of words, because accurate spelling may not be regarded as a high priority in the survey situation. WordStat has some useful tools for correcting spellings and it may be helpful to apply these at an early stage. Firstly, you should check that the correct spelling dictionary is being used, and this can be done via the options tab and its speller / thesaurus sub-tab. Then, if you click on the frequencies tab and its sub-tab unknown words, you can select an appropriate minimum frequency (we have used "1" to see all unknown words) and hit the search button (flashlight icon) to display all sets of words found that do not match the dictionary.
Figure 16, below, shows a screenshot of the spelling correction stage. Select a misspelled word in the middle window, right-click for a context menu and select "keyword in context" to view all of the occurrences of that word if you want to check how it has been used in the responses. In Figure 16 the word "personaly" is being checked and its two occurrences are shown in the lower overlapping window. When you have identified a word to be corrected, close the keyword-in-context window if it has been opened, then select "replace in text with" if it has indeed been mis-spelled and either select one of the offered corrections in the dialog box that then opens or type in your own correction and press OK, so that a record appears in the right hand panel under "replacements to be performed:". In this example we chose to correct "didnt" as "didn’t" and subsequently to correct "personaly" as "personally". When you have corrected as many words as you wish, click on the "perform replacements" button on the right and the source texts will be altered accordingly throughout the QDA Miner suite of programs. This simple routine should save you trouble later if you want to autocode for meaning using any of the correct versions of these words.
Figure 16: Spelling corrections in WordStat
At any stage, after correcting spellings or altering an exclusion dictionary for example, you can return to the frequencies page, select the "included" sub-tab, and view the amended results. Figure 17 shows a display of this page. The default exclusion list has been applied in this example, removing several words which will not help the analysis much, and some potential themes are already apparent in this document ("floodline", "furniture", "garden" etc). This display can be sorted by frequency using the pull-down menu above the table, where other sort options are available, or by clicking on the appropriate column header. It is advisable to check the alphabetical sort sometimes (as shown here) because validly spelled variations of words (such as singular and plural versions) may still be shown separately (depending on the substitution/lemmatization setting on the dictionaries page).
Figure 17: Frequencies page in WordStat
TipIt is possible to display the exclusion list (as in Figure 17) by clicking on that phrase in the left hand panel, the full list then appears in the lower part of the left hand panel. To help show this, the category list has also been collapsed to its root. As can be seen here the default exclusion list is extensive, it was developed for particular analysis purposes and may not be appropriate for some types of dataset, for example it would exclude "after" and "afterwards" but these words may be significant in our example dataset when considering the timing of advice and warnings about floods. We would suggest not applying any exclusion list at first but to consider developing and applying your own list if you find your frequency table is getting clogged-up with too many trivial words. |
TipA useful feature of this page is that a keyword retrieval function is available through the magnifying glass icon above the table. This opens in a separate window so that it can be used without obscuring the frequency table, and it has considerable functionality to include multiple words and apply filters. Alternatively the keyword-in-context tab can be selected to see an alternative way of examining how a single word has been used in the data. Both of these functions have selection boxes that work interactively with the frequency table list to aid the examination of important words. Moving between these various displays should help the formulation of principle coding themes. More will be written about the differences between keyword retrieval and keyword-in-context further down this page. |
As a further aid to developing ideas about themes within the data it is possible to look at frequently used phrases in the text. These can be seen on the phrase finder tab. Various parameters can be set by the user but the defaults are a good place to start. Figure 18 shows an illustration of this function. Note the parameter settings near the top of the screen, "min words: 2 - max words: 5 - min frequency: 3 - sort by: frequency" and then the search button in the form of a flashlight icon which has to be clicked to create the phrase list in the central panel. An icon of two overlapping rectangles switches on the overlapping phrases panel which is also shown in this illustration, this lists other phrases which overlap in whole or in part with the phrase selected in the central panel.
Figure 18: WordStat phrase finder screen
TipThe extraction of phrases is particularly sensitive to the application of an exclusion dictionary and / or substitutions (such as lemmatization) as set on the dictionaries tab. With this data the act of turning on the default exclusion dictionary (part of which can be seen in the bottom left corner of Figure 18) reduced the number of phrases from 407 matching the search parameters (indicated in the bottom bar of the screen) to just 16 phrases. And lemmatization made many phrases much harder to interpret as grammatical stems replaced many words. So care should be taken when those functions are combined with the phrase finder tool. |
It is now possible to do the coding work within WordStat, building on the inductive derivation of coding themes described above. Although there are several different ways of analysing the texts in WordStat there is only one with a link to the coding function. This is to be found on the frequencies page, by using the keyword retrieval button (the magnifying glass icon, sixth from the left), and generating a report similar to that of the text retrieval in the main program. An alternative way to open this is to right click on a word in the frequencies list and select keyword retrieval from the context menu, this jumps straight to the results screen for the selected word but the criterion can still be adjusted as described below.
Figure 19, below, shows this keyword retrieval with a simple filtering criterion. Note that the keywords should be selected using the pull-down menu, which accesses the word list shown in the frequencies window (although only in alphabetical order). Complex and sophisticated combinations of keywords can be used to generate reports with this function. However the results screen, which is activated when the search button is clicked, has facilities to add or select codes, remove single items from the report, and apply the selected code to individual items or the whole report list in ways similar to those described for the text retrieval report above. The only difference is that it is not possible to work interactively between this report and the QDA Miner main screen in order to apply codes manually to carefully selected individual passages of text, these codes have to be applied to the whole sentence or paragraph (depending on the retrieve setting on the criterion screen).
Figure 19: WordStat keyword retrieval function
It is unlikely that a single search will exhaust the potential autocoding for one code, and the process can be repeated with variations on the search theme. Where several different words can be identified as relating to a particular coding theme they can be used in separate retrievals or combined in complex ones.
The autocoding process will not complete the task if data reduction to accurate quantities of references is the goal of the analysis (see section 4 below). There will often be responses which relate to a particular theme without using any of the main keywords associated with that theme. So a combination of autocoding and human interpretation is needed to achieve a high level of accuracy. However, when dealing with extremely large numbers of responses in a large scale survey that degree of accuracy may not be necessary and the automated procedures may deliver all that is required, provided they are used with imagination and thoroughness.
It is suggested that the word frequency and phrase finder tools may be used in an exploratory way to generate ideas for themes and codes from within the data. This is unlikely to be a linear process, instead requiring a lot of movement between different views of the data. It will often be useful to view a selected word or phrase in all of its contexts, which can be done from the menu available with a right click, and the context displays can be sorted by case number, keyword and before, or keyword and after (these latter two sorts referring to the preceding or succeeding words beside the keywords). This can be very helpful in identifying whether a word or phrase has been used with a consistent meaning or not. When such themes have been identified they may be noted, with their distinguishing words or phrases, for subsequent coding back in QDA Miner, or alternatively they may be autocoded directly from within WordStat as explained above.
5. Coding – data indexing versus data reduction
The actual techniques of manually applying codes to segments of text are not discussed here. They are common to all applications of the program and are clearly explained in QDA Miner’s help manual and in other sources. However, the possible uses to which the analysis of responses to open-ended survey questions may be put is a matter worth discussing further.
As a coding scheme is developed and applied to textual data, the analyst will inevitably encounter uncertainty and doubt. Does the text in front of me represent something different from others I have read before which mentioned a particular keyword? A common solution to this is to be generous and inclusive, applying specific codes to a range of comments that initially appear to be connected to those concepts, with the good intention of returning later and checking the work. This activity may be described as "data indexing" as it facilitates the retrieval of various passages that appear to relate to a particular topic.
When open-ended questions have been asked in survey situations it may be anticipated that the analyst will often be asked to generate numerical summaries of the data, probably in the form of statements of the type "X% of responses to this question mentioned Y". The obvious source of the numbers for this output is the coding of concept "Y". However the statement will only be valid if the use of that concept in every one of the responses allocated that code is consistent and equivalent, because the code that is used in this way has effectively replaced the words recorded for each respondent. The original textual data has been reduced to the code label.
When put this way it should be apparent that work needs to be done by the analyst to refine the inclusive indexing codes before they can be safely used as summarising reducing codes. In this example data one respondent answered the question about the advice they had received by saying "... move self and belongings upstairs. Contact floodline. I think there were a few tags to put on things in the kitchen. The pack itself was very well done and well thought out ..." while another dismissed this as "... a flood pack stating obvious, silly stickers ...". Initial index coding may have allocated a code "given flood pack" to both of these passages but it could be potentially misleading to include both in a percentage of respondents who referred to the flood packs as though these comments are equivalent to each other.
It may be anticipated that more use will be made of automatic coding procedures in QDA Miner than other CAQDAS programs because it has such sophisticated tools for analysing text, and it will process large volumes of textual data quickly with those tools. In such circumstances the analyst needs to be aware of the risks of incorrectly applied codes but, where the numbers are large, a few errors will have little impact on percentage statistics so the concern needs to be kept in proportion.
6. Checking summarising codes – consistency and omissions
There are a variety of tools in QDA Miner to assist with the refinement of codes when they have to be reduced to summarise what was originally said. Two particular aspects should be considered, firstly confirmation that all of the passages connected to any one code are all sufficiently similar to be treated as equivalent, and secondly confirmation that no other passages that are also equivalent have been omitted from that code.
The first step in confirming consistency or equivalence is to extract all of the passages that have been allocated to a code and check them carefully. This can be done visually in QDA Miner or with further software assistance in WordStat. Run the command analyse / coding retrieval and select the relevant document and code to be checked from the drop-down menus, then click on the search button. It is likely that you will start to read the coded texts looking for any that seem different from the rest but, if the resulting number of hits is very large, it may be more practical to use WordStat to analyse this set of responses. It is possible to jump into WordStat directly from many of the report screens in QDA Miner and the subsequent content analysis will be carried out on only the texts that make up that report, the icon to do this is a magnifying glass within the report window. It is not necessarily easy to identify the rare items in a set which do not belong there by using content analysis tools which were designed to identify the most common themes. However, using the keyword retrieval (via a right click and context menu) from the frequencies page on the most commonly found words should pull up subsets of the data with high degrees of consistency. Similar searches can be run on some of the least frequently used words to check that these are not out of place.
After checking all of the passages linked to a single code it should be possible to write a concise definition of that code, possibly incorporating the most frequently used keywords from the above WordStat frequencies page. This definition may be typed into the description field for that code by selecting edit code from the context menu when you right click on the code label in the codes panel of the main QDA Miner screen. If you find it difficult to write a concise definition of a code then it may be inferred that you should not refer to the number of references to that code in any data reducing statements.
It is more difficult to search for code omissions, these are passages which are closely equivalent to those already allocated to a particular code but which have not yet been allocated themselves. One possible method for doing this is to filter-out all of the passages which have been allocated to the code and then carry out a content analysis on the remainder using key words connected with that code.
There is no simple query that allows one to search for all responses to one question that have not been allocated to one code, but it is possible to create a neutral code and apply it to all of the paragraphs in a document variable and then one can search for all the neutrally coded paragraphs that do not enclose the code of interest. So, in this example, a text retrieval was run to select all paragraphs in document QLOC2 (without any other selection criteria). This found 91 hits (or response paragraphs). A simple code "Qloc2" was created and applied to all of these hits using the "code all hits" icon in the report window. Then a code retrieval report was run as shown in Figure 20, using the code "never listen to it" as the code of interest. There were 17 positive allocations of this code, so the number of responses without it was expected to be 91 – 17 = 74 and, as Figure 20 shows, that many were identified by this report.
The results of this retrieval can be scanned visually for passages which may match the definition for the code of interest. These would represent errors or omissions from that code.
Figure 20: Code retrieval to search for code omissions
Once again, by clicking on the magnifying glass icon within the code retrieval search hits window, it is possible to move this subset of the data into WordStat and use that module to identify possible errors or omissions for this code. Now, if the coding has been done with reasonable care so far, the items of interest may be expected to be found in the lowest frequencies so an alphabetical listing of keywords may be useful to prove the satisfactory absence of the main terms.
7. Looking for similarities or differences?
When analysing the responses to open ended survey questions it may well be easy to slip into the expectation that the most frequently used codes, or rather the concepts to which they refer, are the most important. After all, these are the items that seem to have the most statistical ‘weight’. However, it should always be worth looking out for contributions which are different from the common ideas. One-off comments will never feature in the quantitative tables because, by definition, they lack numerical support. But a small number of individuals may well take the opportunity of an open-ended question to add an unexpected thought and these contributions represent a challenge and an opportunity for the analyst.
Perhaps it is worth asking yourself what was the purpose behind the inclusion of an open ended question in the survey. In many situations previous research will have revealed the most likely answers and these will have been included as response categories in closed questions asked elsewhere in the survey, but then an open question has been included to pick up other ideas. In these situations it is the unusual answers which may be of most interest.
For example in the data used as an example for these instructions the question QADVI2 was used to ask respondents what advice they had been given in order to prepare themselves for an impending flood about which they had been warned. It may be interesting to note that out of 324 responses to this question, just three people mentioned warm clothing or blankets. It may be the case that for most people the need for warm clothing as you sit out a flood in an upstairs room is too obvious to be worth mentioning, but this may also be a clue that there was a potentially significant gap in the advice actually given to the flood victims in the incidents under consideration. It seems that the value of a detailed qualitative analysis of the responses to such a question is an opportunity to pick up the unexpected ideas which would be so easily overlooked in a statistical analysis.
Those using QDA Miner to analyse this sort of data may find locating these unusual responses particularly challenging as the design of this program is so geared to identifying commonalities in the texts. It is probably a good idea to set up a special code to be used to index any such rarer ideas that are noticed during the course of the analysis. In all probability this code would be allocated manually whenever a response is found which seems to be outside the common themes. Then, at a late stage in the analysis, a report could be generated to output all the texts allocated as "unusual" for manual review and consideration. This should provide a counterweight to the degree of automation suggested in the guidance above.
Exporting data from CAQDAS software
Data can be exported into SPSS or MS Excel for subsequent statistical manipulation. The export strategies outlined below can only be effected after the data have been imported and coded systematically in a CAQDAS software project.
1. Use of code matrix browser
Creating a Microsoft Excel file with the count of code frequencies for each respondent is quite straightforward in MAXqda. It is a special application of the code matrix browser. Before starting the routine you should activate all of the cases (this can be done simply with a Ctrl + click on the group header in the document system window, or a right click on the group header followed by selecting "activate all documents" from the context menu that appears). Then you need to activate all of the codes that you want included in the export table – this may not be absolutely every item in your code system as, for example, there would be no need to include the question identifier codes that the system set-up when you first imported the data into the program – Ctrl + click on each hierarchical group of codes that you want to include will achieve this.
With the activations in place, select visual tools / code matrix browser from the menu bar or the appropriate icon from the visual tools toolbar, and confirm "yes" to the dialog "only for activated documents and codes?". The routine will then process the data, which may take a few moments if you have large numbers of cases and codes.
Figure 1, below, shows a portion of the output for our example data. This takes the form of a very wide matrix with 1,257 columns of data (because we had 1,257 respondents) and a row for each code. It is possible to view different parts of the table by using a slider to the right of the toolbar in the report window or by using a windows slider at the bottom of the report. We applied relevant codes only once to each response so the frequencies in this table are either 1 or blank.
Figure 1: Code matrix browser for all cases and selected codes
The procedure for exporting this report is simply to click on the "Excel" icon at the left of the toolbar in the Browser window (as can be seen in Figure 1, above). This opens MS Excel, imports the data, and transposes the data so that the codes appear in columns and the cases in rows. It also inserts a zero in all of the blank cells of the table. So the result in our example is a spreadsheet with 1,257 rows and 37 data columns, as illustrated in Figure 2, below.
Figure 2: Excel table following export from MAXqda
2. Editing in MS Excel
If you are intending to move this data into SPSS or another statistical program you may need to do some further editing. The code names in the top row of the table may appear unhelpful with the hierarchical group name and backslash preceding the important part of the name, but these can be manually edited within Excel quite easily to match whatever restrictions the statistical software may impose on variable names. The case identifiers may be rather more difficult to turn into a form that enables matching with those already in the statistical software because the distinguishing number is preceded by unwanted text.
The first step in cleaning up the case identifiers is to insert two new columns between the current column A and the first column of code data, ie between columns A and B in Figure 2. The corrected IDs will be created in these new columns. In our example the required data are the last five digits of the text in column A, and these can be extracted by typing the logic =RIGHT(A2,5) into the cell at B2 (in the new empty column just previously inserted) and then copying that logic all the way down column B. It will then be necessary to convert these logical statements that display the correct digits into absolute values in the new column C. This final step involves copying all of column B and then pasting it into column C with a "paste special" command to store "values" only. When this has been completed and checked for accuracy, columns A and B can be deleted and the workbook can be saved.
The column headers in row 1 of the spreadsheet are likely to be used by the statistics package as the names of the new variables whose data is in those columns. Here the problem lies with the prefix path labels that MAXqda has inserted in front of the code name. On the assumption that the number of variables exported is not very large it is probably simplest to edit these manually as with any text cell in MS Excel.
When you are satisfied that the row and column identifiers are in the correct format for importing into the statistics program, save and close the spreadsheet file.
3. Checking data transfer
Whenever large amounts of data are moved between programs there will be potential sources of error so, before continuing with the analysis in your statistical package, it would be advisable to check a small sample of cases in that package to confirm that the code frequencies have been matched with the correct cases. This is not simply a matter of confirming that the total frequency for each imported code variable is the same in MAXqda and your statistical program but also that individual cases have correct codes.
1. Use of matrix coding query
In summary the procedure for extracting code frequencies at the level of respondent cases is to create a matrix coding query with a row for each case and a column for each code variable, and to store the results of running that query as a new matrix. That new matrix can then be exported to MS Excel in a readily accessible format.
Open a new query by navigating to the queries folder, clicking on the "new" button in the main toolbar and selecting new matrix coding query in this folder from the pull-down menu there. It is probably a good idea to save this query for possible re-use so, before getting into the detailed parameters, click on the "add to project" box and give the query a name on the general tab that immediately opens up. Then click on the "matrix coding criteria" tab which has three tabbed sub-pages "rows", "columns" and "matrix". Working initially on the "rows" page, click on the "select" button in the "define more rows" section to open the "select project items" dialog window.
Figure 1: Select project items within matrix coding query set-up
As shown in Figure 1, above, a click on the "cases" folder in the left-hand panel opens up the list of case identifiers in the right-hand panel, and a click in the check box beside the "cases" folder in the left-hand panel inserts ticks for all of the cases on the right. Now, a click on the "OK" button will return you to the query dialog where a further click on the "add to list” button is necessary in order to insert all of these cases into the rows there, as shown in Figure 2.
Figure 2: Matrix coding query – case identifiers in rows
Next, click on the "columns" tab to define the other dimension of the table. The centre of the dialog will be blank until you have selected the appropriate codes and added them to the query. As with the rows tab, click on the "select" button in the section "define more columns" to open the "select project items" dialog once more. This time, as shown in Figure 3 below, click on the "tree nodes" (or "free nodes" if that is where the relevant codes are listed) in the left-hand panel to view lists of codes in the right-hand panel. If you have grouped codes in hierarchical structures you may need to expand these by clicking on the "+" boxes beside the group headers to see the full lists.
Figure 3: Selecting codes for frequencies output
Here, we have expanded the group "better sources" and then selected all of its members by clicking on the check box above the lists "automatically select hierarchy" and then the check box beside "better sources". If you wish to exclude some codes from the export from within the group, a further click on the check box beside those items will untick them. Alternatively, you may prefer to select specific codes for the export by ticking them directly one at a time. As with the cases before, when you are satisfied with your selections click on the "OK" button in this dialog box and then the "add to list" button in the query dialog box. This should bring you to a view similar to Figure 4.
Figure 4: Matrix coding query – code / variables in columns
Whether you need to specify the source documents in the lower part of the query dialog will probably depend on how you have organised the coding scheme for your data. If you created a separate set of codes for each question/source document then the selection of a set of codes will effectively limit the data extraction to the source with which those codes were used and no further selection will be necessary. On the other hand, if you have used some codes with two or more question documents then you may need to consider whether to limit the output to the code frequencies for a single question. To restrict the query to codes applied to a particular question you will need to use the pull-down menu beside "in" at the bottom of the dialog (see Figure 4, above) to change "all sources" to "selected items", the "select" button to the right of this then becomes active and will take you once more to the select project items dialog where you can select the required document from the "internals" folder in ways similar to the selection of cases and nodes above.
TipEven though it may not be strictly necessary to restrict the query to a selected source document, there may be a speed advantage in applying such a limit to save the program searching in lots of unnecessary locations. |
Having specified the rows, columns and, if necessary, sources for the query, you should now click on the third tab of this section and open the "matrix" page, as illustrated in Figure 5 below. Here the default setting of "AND" in the first field is the correct one for this application of the query function. It is likely too that the default of "project item name" for the name display is better than the alternative "hierarchical name" in order to get more usable variable names as the column headers in the output table.
Figure 5: Settings for the matrix page
Finally, having completed the specification for the contents of the output, you need to click on the "query options" tab in order to direct the output to a results table which can be exported to MS Excel. This page is shown in Figure 6, below. It is probably advisable to set the first field on this page to "create results as new matrix" (as shown here) rather than the default "preview" in order to get the table stored in the results section of your project where it can be accessed again much more quickly than by re-running the query. Setting the option in this way activates the "select" button for the "location" field and the "name" field for finding the results table again in the future.
Figure 6: Query options page settings
As can be seen in Figure 6, there are two check boxes in this dialog but these only become active when the "result" option is changed to "create results as new matrix". The first of these, "open results", simply makes the program open the display of the output table in the detailed view panel when the search and calculations are complete. The second, "create results if empty" appears to refer to the entire query and not to individual data lines thus, as long as there is at least one code reference in the set of codes and sources that you have defined on the matrix coding criteria pages, you will get a full table listing every case and code that you have defined whether you tick this box or not. In our example data we have tried running the same query with this box ticked and unticked and on both occasions obtained a matrix with 1,257 rows, even though some of them had zero for every variable.
Finally, you can press the "run" button to set the query running. This will save the query in the "queries" folder with the name you gave it on the "general" tab, store the output table in the "results" folder with the name you gave it on the "query options" tab, and display the table in the detailed view panel (if you ticked the "open results" box). The "apply" and "OK" buttons will simply save the query without running it.
A portion of the output table obtained from this query is shown in Figure 7, below.
Figure 7: Output table displayed in detailed view panel
In Figure 7 the "grid" toolbar has been used to set the display to show "coding references" in the cells in order to obtain the frequencies that are the purpose of this export. In our data these are either 1 or 0 as relevant codes were only applied once to a response, no matter how many times key words were used within that response. The "matrix cell shading" menu has been used to highlight the cells containing "1". Note how the query setting to display project item names has generated simple code names in the column headings.
To export this table to MS Excel, use the menu option project / export result. This opens a common MS Windows dialog box for you to select a folder and file name for the spreadsheet file, with the default file type of "MS Excel Files (*.xls)".
TipIf you have used the cell shading option as illustrated in Figure 7 it will be included in the exported file and shown in Excel, so if you want to have plain white cells only it is recommended that you cancel the cell shading before creating the export file |
2. Editing in MS Excel
When you open the file you have just created in MS Excel it will look exactly like the one displayed within NVivo. This means that the row and column titles will be identical to those in NVivo and that may not be what you want when you import this data into your statistical package. In order to match up this data with the other quantitative data it will be important to make the case identifiers fit the format of their equivalents in the statistics package, and you will want to have variable names that are usable in the statistics package. Thus some editing of the first row and column of the data is likely to be necessary.
It is most likely that the case identifiers in the statistics package are simply the numbers at the end of the alpha-numeric strings that have been used in NVivo. These can be extracted with a few procedures outlined below.
The first step in cleaning up the case identifiers is to insert two new columns between the current column A and the first column of code data, i.e. between columns A and B in the workbook. The corrected IDs will be created in these new columns. In our example the required data are the last five digits of the text in column A, and these can be extracted by typing the logic =RIGHT(A2,5) into the cell at B2 (in the new empty column just previously inserted) and then copying that logic all the way down column B. It will then be necessary to convert these logical statements that display the correct digits into absolute values in the new column C. This final step involves copying all of column B and then pasting it into column C with a "paste special" command to store "values" only. When this has been completed and checked for accuracy, columns A and B can be deleted and the workbook can be saved.
The column headers in row 1 of the spreadsheet are likely to be used by the statistics package as the names of the new variables whose data is in those columns. Here the problem lies with the prefix letters that NVivo has inserted in front of the code name. On the assumption that the number of variables exported is not very large it is probably simplest to edit these manually as with any text cell in MS Excel.
When you are satisfied that the row and column identifiers are in the correct format for importing into the statistics program, save and close the spreadsheet file.
3. Checking data transfer
Whenever large amounts of data are moved between programs there will be potential sources of error so, before continuing with the analysis in your statistical package, it would be advisable to check a small sample of cases in that package to confirm that the code frequencies have been matched with the correct cases. This is not simply a matter of confirming that the total frequency for each imported code variable is the same in NVivo and your statistical program but also that individual cases have correct codes.
1. Project export code statistics
Creating a Microsoft Excel file with the count of code frequencies for each respondent is quite straightforward in QDA Miner as there is a specific routine designed for this task. It is found in the menu option project / export / code statistics and this brings up the dialog box shown in Figure 1.
Figure 1: Export code statistics dialog
In Figure 1, above, the necessary fields have been completed for the task of exporting all the thematic codes applied to the question document "QMORE". The alternative options for the "export:" field are "occurrence, word count, and rate per 1000 words" – with the way we have applied relevant codes only once to each response it is likely that occurrence and frequency would generate identical results of 0 or 1 for each cell in the table. In the second field a pull-down menu offers a full list of codes but the alternative way, using the colourful icon to the right, offers a hierarchical table making it easier to select a group of codes by ticking the box of its header item. One or more question documents can be selected with the pull-down menu for the field beside "in:", on this occasion we have restricted the export to a single document variable.
An important field is to tick the "case descriptor" check box as this is the setting that will generate the case identifier label for each row in the output table. However, before proceeding to run the procedure it is worth checking that the case descriptor in use is as helpful as possible, because this item can be set to many different values by the user. What will appear in the spreadsheet table as the label for each row is the description for each case currently appearing in the CASES panel of your QDA Miner working screen. To adjust the descriptor use the menu option cases / grouping / descriptor... which brings up the dialog illustrated in Figure 2 below.
Figure 2: Setting the case descriptor field to ID
For this purpose the case description that is needed is one that includes the number by which respondents are identified in the statistics package, so that the new frequency variables can be matched with the other quantitative data associated with each case. In our example the "ID" variable is the one needed, so that has been entered between braces in the "description string:" field. Note also that the setting of "<none>" is the one required in the "grouping" field for this output.
Returning to the export code statistics dialog, when you are satisfied that the correct settings are in the necessary fields, click on the "OK" button to run the routine. You may notice a blue progress bar in the bottom left corner of the QDA Miner main screen but this procedure runs very quickly. When the calculations are complete a standard MS Windows dialog appears for you to enter a file name and location to store the data in MS Excel (*.xls) format.
2. Editing in MS Excel
When you open the file that you have just created in Excel, the only editing that you may need to do is to clean-up the case identifiers in order to make them match those already in the statistics database. In our example we had the word "case" as a prefix to each unique ID number and needed to remove this. A quick way of doing this for a large number of cases in Excel is described below.
The first step in cleaning up the case identifiers is to insert two new columns between the current column A and the first column of code data, ie between columns A and B in the workbook. The corrected IDs will be created in these new columns. In our example the required data are the last five digits of the text in column A, and these can be extracted by typing the logic =RIGHT(A2,5) into the cell at B2 (in the new empty column just previously inserted) and then copying that logic all the way down column B. It will then be necessary to convert these logical statements that display the correct digits into absolute values in the new column C. This final step involves copying all of column B and then pasting it into column C with a "paste special" command to store "values" only. When this has been completed and checked for accuracy, columns A and B can be deleted and the workbook can be saved.
The column headers should not need any editing as QDA Miner exports the plain code name without any additional text or prefix. Provided these names are unique in your database they should be an adequate basis for the variable names in the statistics program.
3. Checking data transfer
Whenever large amounts of data are moved between programs there will be potential sources of error so, before continuing with the analysis in your statistical package, it would be advisable to check a small sample of cases in that package to confirm that the code frequencies have been matched with the correct cases. This is not simply a matter of confirming that the total frequency for each imported code variable is the same in QDA Miner and your statistical program but also that individual cases have correct codes.