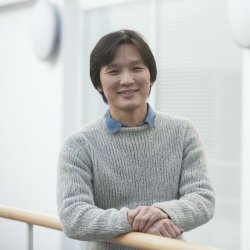
Dr Naratip Santitissadeekorn
Academic and research departments
Mathematics at the Interface Group, School of Mathematics and Physics.About
Biography
I received my PhD from Clarkson University, under the supervision of Professor Erik Bollt, in 2008. My dissertation is titled "Transport Analysis and Motion Estimation of Dynamical Systems of Time-Series data". From 2008-2011, I was a postdoctoral research associate at the University of New South Wales, Sydney, Australia, working with Professor Gary Froyland in a development of numerical techniques for finite-time Lagrangian coherent set identification. The techniques were applied to delimiting the polar vortex and Agulhas rings. From 2011-2014, I was a postdoctoral researcher at the University of North Carolina-Chapel Hill, working with Professor Chris Jones on a data assimilation project.
ResearchResearch interests
- Inverse Problem and Data Assimilation in Geophysical Fluid Dyanmics
- Applications of Lagrangian Coherent Structures (LCS)
- Computational Ergodic Theory
Research interests
- Inverse Problem and Data Assimilation in Geophysical Fluid Dyanmics
- Applications of Lagrangian Coherent Structures (LCS)
- Computational Ergodic Theory
Teaching
- MAT1031: Seminar (Alegebra), Semester 1, 2014/2015
- MAT3003: Bayesian Statistics, Semester 2, 2014/2015
Publications
Many networks have event-driven dynamics (such as communication, social media and criminal networks), where the mean rate of the events occurring at a node in the network changes according to the occurrence of other events in the network. In particular, events associated with a node of the network could increase the rate of events at other nodes, depending on their influence relationship. Thus, it is of interest to use temporal data to uncover the directional, time-dependent, influence structure of a given network while also quantifying uncertainty even when knowledge of a physical network is lacking. Typically, methods for inferring the influence structure in networks require knowledge of a physical network or are only able to infer small network structures. In this paper, we model event-driven dynamics on a network by a multidimensional Hawkes process. We then develop a novel ensemble-based filtering approach for a time-series of count data (i.e., data that provides the number of events per unit time for each node in the network) that not only tracks the influence network structure over time but also approximates the uncertainty via ensemble spread. The method overcomes several deficiencies in existing methods such as existing methods for inferring multidimensional Hawkes processes are too slow to be practical for any network over -50 nodes, can only deal with timestamp data (i.e. data on just when events occur not the number of events at each node), and that we do not need a physical network to start with. Our method is massively parallelizable, allowing for its use to infer the influence structure of large networks (-10, 000 nodes). We demonstrate our method for large networks using both synthetic and real-world email communication data.(c) 2023 The Author(s). Published by Elsevier B.V. This is an open access article under the CC BY license (http://creativecommons.org/licenses/by/4.0/).
Flow fields are determined from image sequences obtained in an experiment in which benthic macrofauna, Arenicola marina, causes water flow and the images depict the distribution of a tracer that is carried with the flow. The experimental setup is such that flow is largely twodimensional, with a localized region where the Arenicola resides, from which flow originates. Here, we propose a novel parametric framework that quantifies such flow that is dominant along the image plane. We adopt a Bayesian framework so that we can impart certain physical constraints on parameters into the estimation process via prior distribution. The primary aim is to approximate the mean of the posterior distribution to present the parameter estimate via Markov Chain Monte Carlo (MCMC). We demonstrate that the results obtained from the proposed method provide more realistic flows (in terms of divergence magnitude) than those computed from classical approaches such as the multi-resolution Horn-Schunk method. This highlights the usefulness of our approach if motion is largely constrained to the image plane with localized fluid sources.
Cellular Automata (CA) models are widely used to study spatial dynamics of urban growth and evolving patterns of land use. One complication across CA approaches is the relatively short period of data available for calibration, providing sparse information on patterns of change and presenting problematic signal-to-noise ratios. To overcome the problem of short-term calibration, this study investigates a novel approach in which the model is calibrated based on the urban morphological patterns that emerge from a simulation starting from urban genesis, i.e., a land cover map completely void of urban land. The application of the model uses the calibrated parameters to simulate urban growth forward in time from a known urban configuration. This approach to calibration is embedded in a new framework for the calibration and validation of a Constrained Cellular Automata (CCA) model of urban growth. The investigated model uses just four parameters to reflect processes of spatial agglomeration and preservation of scarce non-urban land at multiple spatial scales and makes no use of ancillary layers such as zoning, accessibility, and physical suitability. As there are no anchor points that guide urban growth to specific locations, the parameter estimation uses a goodness-of-fit (GOF) measure that compares the built density distribution inspired by the literature on fractal urban form. The model calibration is a novel application of Markov Chain Monte Carlo Approximate Bayesian Computation (MCMC-ABC). This method provides an empirical distribution of parameter values that reflects model uncertainty. The validation uses multiple samples from the estimated parameters to quantify the propagation of model uncertainty to the validation measures. The framework is applied to two UK towns (Oxford and Swindon). The results, including cross-application of parameters, show that the models effectively capture the different urban growth patterns of both towns. For Oxford, the CCA correctly produces the pattern of scattered growth in the periphery, and for Swindon, the pattern of compact, concentric growth. The ability to identify different modes of growth has both a theoretical and practical significance. Existing land use patterns can be an important indicator of future trajectories. Planners can be provided with insight in alternative future trajectories, available decision space, and the cumulative effect of parcel-by-parcel planning decisions.
A number of models – such as the Hawkes process and log Gaussian Cox process – have been used to understand how crime rates evolve in time and/or space. Within the context of these models and actual crime data, parameters are often estimated using maximum likelihood estimation (MLE) on batch data, but this approach has several limitations such as limited tracking in real-time and uncertainty quantification. For practical purposes, it would be desirable to move beyond batch data estimation to sequential data assimilation. A novel and general Bayesian sequential data assimilation algorithm is developed for joint state-parameter estimation for an inhomogeneous Poisson process by deriving an approximating Poisson-Gamma ‘Kalman’ filter that allows for uncertainty quantification. The ensemble-based implementation of the filter is developed in a similar approach to the ensemble Kalman filter, making the filter applicable to large-scale real world applications unlike nonlinear filters such as the particle filter. The filter has the advantage that it is independent of the underlying model for the process intensity, and can therefore be used for many different crime models, as well as other application domains. The performance of the filter is demonstrated on synthetic data and real Los Angeles gang crime data and compared against a very large sample-size particle filter, showing its effectiveness in practice. In addition the forecast skill of the Hawkes model is investigated for a forecast system using the Receiver Operating Characteristic (ROC) to provide a useful indicator for when predictive policing software for a crime type is likely to be useful. The ROC and Brier scores are used to compare and analyse the forecast skill of sequential data assimilation and MLE. It is found that sequential data assimilation produces improved probabilistic forecasts over the MLE.
Characterisation of the urban expansion processes using time series of binary urban/non-urban land cover data is complex due to the need to account for the initial configuration and the rate of urban expansion over the analysed period. Failure to account for these factors makes the interpretation of landscape metrics for compactness, fragmentation, or clumpiness problematic and the comparison between geographical areas and time periods contentious. This paper presents an approach for characterisation using spatio-dynamic modelling which is data-centred using a process based model, Bayesian optimization, cluster identification, and maximum likelihood classification. An application of the approach across 652 functional urban areas in Europe (1975-2014) demonstrates the consistency of the approach and its ability to identify spatial and temporal trends in urban expansion processes.
Scenarios of future urban expansion are expected to be plausible: they must be diverse to reflect future uncertainty, yet realistic in their depiction of urban expansion processes. We investigated the plausibility of scenarios derived from a novel data-driven simulation approach. In a Turing-like test, experts completed a quiz in which they were asked to identify the map showing true urban expansion amidst three model-generated scenarios. Across diverse expansion patterns, ranging from compact to dispersed, the experts had no significant ability to identify the true pattern. The results support the hypothesis that the investigated scenarios are plausible and hence that cluster analysis of estimated dynamic models is a viable method for producing scenarios of future urban expansion.
The processes of urban growth vary in space and time. There is a lack of model transferability, which means that models estimated for a particular study area and period are not necessarily applicable for other periods and areas. This problem is often addressed through scenario analysis, where scenarios reflect different plausible model realisations based typically on expert consultation. This study proposes a novel framework for data-driven scenario development which, consists of three components - (i) multi-area, multi-period calibration, (ii) growth mode clustering, and (iii) cross-application. The framework finds clusters of parameters, referred to as growth modes: within the clusters, parameters represent similar spatial development trajectories; between the clusters, parameters represent substantially different spatial development trajectories. The framework is tested with a stochastic dynamic urban growth model across European functional urban areas over multiple time periods, estimated using a Bayesian method on an open global urban settlement dataset covering the period 1975–2014. The results confirm a lack of transferability, with reduced confidence in the model over the validation period, compared to the calibration period. Over the calibration period the probability that parameters estimated specifically for an area outperforms those for other areas is 96%. However, over an independent validation period, this probability drops to 72%. Four growth modes are identified along a gradient from compact to dispersed spatial developments. For most training areas, spatial development in the later period is better characterized by one of the four modes than their own historical parameters. The results provide strong support for using identified parameter clusters as a tool for data-driven and quantitative scenario development, to reflect part of the uncertainty of future spatial development trajectories. A promising further application is to use the growth modes to characterize past spatial development patterns. A trend of increasingly dispersed patterns could be identified over the studied functional urban areas which calls for more detailed explorations.
The primary focus is a sequential data assimilation method for count data modelled by an inhomogeneous Poisson process. In particular, a quadratic approximation technique similar to the extended Kalman filter is applied to develop a sub-optimal, discrete-time, filtering algorithm, called the extended Poisson-Kalman filter (ExPKF), where only the mean and covariance are sequentially updated using count data via the Poisson likelihood function. The performance of ExPKF is investigated in several synthetic experiments where the true solution is known. In numerical examples, ExPKF provides a good estimate of the “true” posterior mean, which can be well-approximated by the particle filter (PF) algorithm in the very large sample size limit. In addition, the experiments demonstrates that the ExPKF algorithm can be conveniently used to track parameter changes; on the other hand, a non-filtering framework such as a maximum likelihood estimation (MLE) would require a statistical test for change points or implement time-varying parameters. Finally, to demonstrate the model on real-world data, the ExPKF is used to approximate the uncertainty of urban crime intensity and parameters for self-exciting crime models. The Chicago Police Department’s CLEAR (Citizen Law Enforcement Analysis and Reporting) system data is used as a case study for both univariate and multivariate Hawkes models. An improved goodness of fit measured by the Kolomogrov-Smirnov (KS) statistics is achieved by the filtered intensity. The potential of using filtered intensity to improve police patrolling prioritisation is also tested. By comparing with the prioritisation based on MLE-derived intensity and historical frequency, the result suggests an insignificant difference between them. While the filter is developed and tested in the context of urban crime, it has the potential to make a contribution to data assimilation in other application areas.
We conduct Observing System Simulation Experiments (OSSEs) with Lagrangian data assimilation (LaDA) in two-layer point-vortex systems, where the trajectories of passive tracers (drifters or floats) are observed on one layer that is coupled to another layer with different dynamics. Depending on the initial position of the observed tracers, the model studied here can exhibit nonlinear features that cause the standard Kalman filter and its variants to fail. For this reason, we adopt a Monte Carlo approach known as particle filtering, which takes the nonlinear dynamics into account. The main objective of this paper is to understand the effects of drifter placement and layer coupling on the precision skill of assimilating Lagrangian data into multi-layered models. Therefore, we analyze the quality of the assimilated vortex estimates by assimilating path data from passive tracers launched at different locations, on different layers and in systems with various coupling strengths between layers. We consider two cases: vortices placed on different layers (heton) and on the same layer (non-heton). In both cases we find that launch location, launch layer and coupling strength all play a significant role in assimilation precision skill. However, the specifics of the interplay of these three factors are quite different for the heton case versus the non-heton case. © 2014.
Given a flow on a surface, we consider the problem of connecting two distinct trajectories by a curve of extremal (absolute) instantaneous flux. We develop a complete classification of flux optimal curves, accounting for the possibility of the flux having spatially and temporally varying weight. This weight enables modelling the flux of non-equilibrium distributions of tracer particles, pollution concentrations, or active scalar fields such as vorticity. Our results are applicable to all smooth autonomous flows, area preserving or not. © 2013 Elsevier Ltd.
A novel probabilistic methodology is applied to identify optimally coherent structures associated with Agulhas Rings, within a time varying velocity field in the South Atlantic Ocean, as simulated by an eddy-permitting ocean general model. It is shown that this technique provides a way of identifying the three-dimensional shape of a particular Ring in the upper ocean and tracking its evolution over space and time. Based on this three-dimensional representation we can accurately measure the amount of water mass remaining in an Agulhas Ring over time and consequently how much heat or salt is released from the structure as it decays. Identification techniques based on relative vorticity or the Okubo-Weiss parameter have previously been developed for a surface snapshot. Extending these methods in the vertical direction in the upper ocean and comparing the decay of all three-dimensional structures obtained by different methods, we demonstrate that our technique is able to define structures that are more coherent over time than classical methods. While our investigation concentrates on a single Agulhas Ring located in the Cape-Basin from May 2000 over 6. months, the technique may be extended to examine multiple Rings and other coherent structures that are involved in the Agulhas leakage. © 2012 Elsevier Ltd.
It has been known that noise in a stochastically perturbed dynamical system can destroy what was the original zero-noise case barriers in the phase space (pseudobarrier). Noise can cause the basin hopping. We use the Frobenius-Perron operator and its finite rank approximation by the Ulam-Galerkin method to study transport mechanism of a noisy map. In order to identify the regions of high transport activity in the phase space and to determine flux across the pseudobarriers, we adapt a new graph theoretical method which was developed to detect active pseudobarriers in the original phase space of the stochastic dynamic. Previous methods to identify basins and basin barriers require a priori knowledge of a mathematical model of the system, and hence cannot be applied to observed time series data of which a mathematical model is not known. Here we describe a novel graph method based on optimization of the modularity measure of a network and introduce its application for determining pseudobarriers in the phase space of a multi-stable system only known through observed data. © 2007 Elsevier Ltd. All rights reserved.
We describe a mathematical formalism and numerical algorithms for identifying and tracking slowly mixing objects in nonautonomous dynamical systems. In the autonomous setting, such objects are variously known as almost-invariant sets, metastable sets, persistent patterns, or strange eigenmodes, and have proved to be important in a variety of applications. In this current work, we explain how to extend existing autonomous approaches to the nonautonomous setting. We call the new time-dependent slowly mixing objects coherent sets as they represent regions of phase space that disperse very slowly and remain coherent. The new methods are illustrated via detailed examples in both discrete and continuous time.
We introduce a general-purpose method for optimising the mixing rate of advective fluid flows. An existing velocity field is perturbed in a C 1 neighborhood to maximize the mixing rate for flows generated by velocity fields in this neighborhood. Our numerical approach is based on the infinitesimal generator of the flow and is solved by standard linear programming methods. The perturbed flow may be easily constrained to preserve the same steady state distribution as the original flow, and various natural geometric constraints can also be simply applied. The same technique can also be used to optimize the mixing rate of advection-diffusion flow models by manipulating the drift term in a small neighborhood.
In this paper, we present an approach to approximate the Frobenius-Perron transfer operator from a sequence of time-ordered images, that is, a movie dataset. Unlike time-series data, successive images do not provide a direct access to a trajectory of a point in a phase space; more precisely, a pixel in an image plane. Therefore, we reconstruct the velocity field from image sequences based on the infinitesimal generator of the Frobenius-Perron operator. Moreover, we relate this problem to the well-known optical flow problem from the computer vision community and we validate the continuity equation derived from the infinitesimal operator as a constraint equation for the optical flow problem. Once the vector field and then a discrete transfer operator are found, then, in addition, we present a graph modularity method as a tool to discover basin structure in the phase space. Together with a tool to reconstruct a velocity field, this graph-based partition method provides us with a way to study transport behavior and other ergodic properties of measurable dynamical systems captured only through image sequences.
The flow field in a cylindrical container driven by a flat bladed impeller was investigated using particle image velocimetry (PIV). Three Reynolds numbers (0.02, 8, 108) were investigated for different impeller locations within the cylinder. The results showed that vortices were formed at the tips of the blades and rotated with the blades. As the blades were placed closer to the wall the vortices interacted with the induced boundary layer on the wall to enhance both regions of vorticity. Finite time lyapunov exponents (FTLE) were used to determine the lagrangian coherent structure (LCS) fields for the flow. These structures highlighted the regions where mixing occurred as well as barriers to fluid transport. Mixing was estimated using zero mass particles convected by numeric integration of the experimentally derived velocity fields. The mixing data confirmed the location of high mixing regions and barriers shown by the LCS analysis. The results indicated that mixing was enhanced within the region described by the blade motion as the blade was positioned closed to the cylinder wall. The mixing average within the entire tank was found to be largely independent of the blade location and flow Reynolds number. © 2011 American Society of Mechanical Engineers.
Given a sequence of empirical distribution data (e.g. a movie of a spatiotemporal process such as a fluid flow), this work develops an ensemble data assimilation method to estimate the transition probability that represents a finite approximation of the Frobenius-Perron operator. This allows a dynamical systems knowledge to be incorporated into a prior ensemble, which provides sensible estimates in instances of limited observation. We demonstrate improved estimates over a constrained optimization approach (based on a quadratic programming problem) which does not impose a prior on the solution except for Markov properties. The estimated transition probability then enables several probabilistic analysis of dynamical systems. We focus only on the identification of coherent patterns from the estimated Markov transition to demonstrate its application as a proof-of-concept. To the best of our knowledge, there have not been many works on data-driven methods to identify coherent patterns from this type of data. While here the results are presented only in the context of dynamical systems applications, this work we present here has the potential to make a contribution in wider application areas that require the estimation of transition probabilities from a time-ordered spatio-temporal distribution data.
We introduce a data assimilation method to estimate model parameters with observations of passive tracers by directly assimilating Lagrangian Coherent Structures. Our approach differs from the usual Lagrangian Data Assimilation approach, where parameters are estimated based on tracer trajectories. We employ the Approximate Bayesian Computation (ABC) framework to avoid computing the likelihood function of the coherent structure, which is usually unavailable. We solve the ABC by a Sequential Monte Carlo (SMC) method, and use Principal Component Analysis (PCA) to identify the coherent patterns from tracer trajectory data. Our new method shows remarkably improved results compared to the bootstrap particle filter when the physical model exhibits chaotic advection.
We study the transport properties of nonautonomous chaotic dynamical systems over a finite-time duration. We are particularly interested in those regions that remain coherent and relatively nondispersive over finite periods of time, despite the chaotic nature of the system. We develop a novel probabilistic methodology based upon transfer operators that automatically detect maximally coherent sets. The approach is very simple to implement, requiring only singular vector computations of a matrix of transitions induced by the dynamics. We illustrate our new methodology on an idealized stratospheric flow and in two and three-dimensional analyses of European Centre for Medium Range Weather Forecasting (ECMWF) reanalysis data.
The "edge" of the Antarctic polar vortex is known to behave as a barrier to the meridional (poleward) transport of ozone during the austral winter. This chemical isolation of the polar vortex from the middle and low latitudes produces an ozone minimum in the vortex region, intensifying the ozone hole relative to that which would be produced by photochemical processes alone. Observational determination of the vortex edge remains an active field of research. In this paper, we obtain objective estimates of the structure of the polar vortex by introducing a technique based on transfer operators that aims to find regions with minimal external transport. Applying this technique to European Centre for Medium-Range Weather Forecasts (ECMWF) ERA-40 three-dimensional velocity data, we produce an improved three-dimensional estimate of the vortex location in the upper stratosphere where the vortex is most pronounced. This computational approach has wide potential application in detecting and analyzing mixing structures in a variety of atmospheric, oceanographic, and general fluid dynamical settings.
This book connects many concepts in dynamical systems with mathematical tools from areas such as graph theory and ergodic theory.
This paper presents an approach for simultaneous estimation of the state and unknown parameters in a sequential data assimilation framework. The state augmentation technique, in which the state vector is augmented by the model parameters, has been investigated in many previous studies and some success with this technique has been reported in the case where model parameters are additive. However, many geophysical or climate models contains non-additive parameters such as those arising from physical parametrization of sub-grid scale processes, in which case the state augmentation technique may become ineffective since its inference about parameters from partially observed states based on the cross covariance between states and parameters is inadequate if states and parameters are not linearly correlated. In this paper, we propose a two-stages filtering technique that runs particle filtering (PF) to estimate parameters while updating the state estimate using Ensemble Kalman filter (ENKF; these two "sub-filters" interact. The applicability of the proposed method is demonstrated using the Lorenz-96 system, where the forcing is parameterized and the amplitude and phase of the forcing are to be estimated jointly with the states. The proposed method is shown to be capable of estimating these model parameters with a high accuracy as well as reducing uncertainty while the state augmentation technique fails.