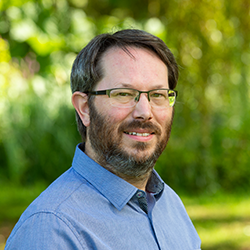
Professor Philip Jackson
Academic and research departments
Centre for Vision, Speech and Signal Processing (CVSSP), School of Computer Science and Electronic Engineering.About
Biography
Sound carries a multiplicity of information about what is going on all around you: from who, what, where and when to the meaning of a story being told. I am interested in what machines can do with acoustical signals, including speech, music and the everyday sounds that surround us.
Through research on projects such as:
- Nephthys
- Columbo
- BALTHASAR
- DANSA
- SAVEE
- DynamicFaces
- QESTRAL
- POSZ
- UDRC2
- S3A
- EyesFree
- Polymersive
- AI4ME.
I have contributed to various sound-related technologies: active noise control for aircraft, speech aero-acoustics, source separation and articulatory models for automatic speech recognition, audio-visual emotion classification and visual speech synthesis, including new techniques for spatial audio and personal sound. Currently sound localisation, audio-visual talker tracking, object-based media production and responsible AI are my foci.
I joined CVSSP in 2002 after a UK postdoctoral fellowship at University of Birmingham, with a PhD in Electronics and Computer Science from University of Southampton (2000) and MA from Cambridge University Engineering Department (1997).
I have over 200 journal, patent, conference and book publications (Google h-index=30) and served as associate editor for Computer Speech and Language (Elsevier), and as reviewer for the Journal of the Acoustical Society of America, IEEE/ACM Transactions on Audio, Speech and Language Processing, IEEE Signal Processing Letters, InterSpeech and ICASSP.
Publications
Weakly supervised audio-visual video parsing (AVVP) methods aim to detect audible-only, visible-only, and audible-visible events using only video-level labels. Existing approaches tackle this by leveraging unimodal and cross-modal contexts. However, we argue that while cross-modal learning is beneficial for detecting audible-visible events, in the weakly supervised scenario, it negatively impacts unaligned audible or visible events by introducing irrelevant modality information. In this paper, we propose CoLeaF, a novel learning framework that optimizes the integration of cross-modal context in the embedding space such that the network explicitly learns to combine cross-modal information for audible-visible events while filtering them out for unaligned events. Additionally, as videos often involve complex class relationships, modelling them improves performance. However, this introduces extra computational costs into the network. Our framework is designed to leverage cross-class relationships during training without incurring additional computations at inference. Furthermore, we propose new metrics to better evaluate a method’s capabilities in performing AVVP. Our extensive experiments demonstrate that CoLeaF significantly improves the state-of-the-art results by an average of 1.9% and 2.4% F-score on the LLP and UnAV-100 datasets, respectively.
3D audio-visual production aims to deliver immersive and interactive experiences to the consumer. Yet, faithfully reproducing real-world 3D scenes remains a challenging task. This is partly due to the lack of available datasets enabling audio-visual research in this direction. In most of the existing multi-view datasets, the accompanying audio is neglected. Similarly, datasets for spatial audio research primarily offer unimodal content, and when visual data is included, the quality is far from meeting the standard production needs. We present "Tragic Talkers", an audio-visual dataset consisting of excerpts from the "Romeo and Juliet" drama captured with microphone arrays and multiple co-located cameras for light-field video. Tragic Talkers provides ideal content for object-based media (OBM) production. It is designed to cover various conventional talking scenarios, such as monologues, two-people conversations, and interactions with considerable movement and occlusion, yielding 30 sequences captured from a total of 22 different points of view and two 16-element microphone arrays. Additionally, we provide voice activity labels, 2D face bounding boxes for each camera view, 2D pose detection keypoints, 3D tracking data of the mouth of the actors, and dialogue transcriptions. We believe the community will benefit from this dataset as it can assist multidisciplinary research. Possible uses of the dataset are discussed.
As audio-visual systems increasingly bring immersive and interactive capabilities into our work and leisure activities, so the need for naturalistic test material grows. New volumetric datasets have captured high-quality 3D video, but accompanying audio is often neglected, making it hard to test an integrated bimodal experience. Designed to cover diverse sound types and features, the presented volumetric dataset was constructed from audio and video studio recordings of scenes to yield forty short action sequences. Potential uses in technical and scientific tests are discussed.
3D audio-visual production aims to deliver immersive and interactive experiences to the consumer. Yet, faithfully reproducing real-world 3D scenes remains a challenging task. This is partly due to the lack of available datasets enabling audio-visual research in this direction. In most of the existing multi-view datasets, the accompanying audio is neglected. Similarly, datasets for spatial audio research primarily offer unimodal content, and when visual data is included, the quality is far from meeting the standard production needs. We present “Tragic Talkers”, an audio-visual dataset consisting of excerpts from the “Romeo and Juliet” drama captured with microphone arrays and multiple co-located cameras for light-field video. Tragic Talkers provides ideal content for object-based media (OBM) production. It is designed to cover various conventional talking scenarios, such as monologues, two-people conversations, and interactions with considerable movement and occlusion, yielding 30 sequences captured from a total of 22 different points of view and two 16-element microphone arrays. Additionally, we provide voice activity labels, 2D face bounding boxes for each camera view, 2D pose detection keypoints, 3D tracking data of the mouth of the actors, and dialogue transcriptions. We believe the community will benefit from this dataset as it can assist multidisciplinary research. Possible uses of the dataset are discussed. The scenes were captured at the Centre for Vision, Speech & Signal Processing (CVSSP) of the University of Surrey (UK) with the aid of two twin Audio-Visual Array (AVA) Rigs. Each AVA Rig is a custom device consisting of a 16-element microphone array and 11 cameras fixed on a flat perspex sheet. For more information, please refer to the paper (see below) or contact the authors.
Room acoustics, and perception thereof, is an important consideration in research, engineering, architecture, creative expression, and many other areas of human activity, particularly indoors. Typical room datasets either contain disparate measurements of diverse spaces, e.g., the OpenAir dataset (Murphy and Shelley, 2010), or rich sets of measurements within a few rooms, e.g., CD4M (Stewart and Sandler, 2010). Development of techniques, such as the RSAO, with 6 degrees of freedom (6DOF) movement in media applications require testing distance-related effects. Hence there is a need for consistency across room measurements in terms of source-room-receiver configuration, such as in (Lokki et al., 2011) but particularly for typical rooms. Those available containing ARIRs, BRIRs and with a consistent measurement procedure tend to be limited in range of rooms measured (Bacila and Lee, 2019). We designed an RIR dataset covering a range of rooms, with typical reverberation times, 1O-ARIRs and BRIRs, and regular measurement procedure including source-receiver distances from 1 m to 3 m in 0.5 m intervals. We measured seven rooms including one room with variable acoustics in two configurations – eight total sets. The rooms within this dataset have mid-range RT60s from 0.24 s to 1.00 s and volumes from 50 m3 to 1600 m3. The accompanying paper includes capture methods, various descriptive metrics and a description of applications across diverse domains. The dataset consists of .WAV files for IRs, .PLY files for LiDAR scans and image files for accompanying 3D pictures. IRs are also available in the SOFA format. The dataset is licensed under CC BY-NC 4.0 and can be accessed from https://cvssp.org/data/SurrRoom1_0/.
In our information-overloaded daily lives, unwanted sounds create confusion, disruption and fatigue in what do and experience. Taking control of your own sound environment, you can design what information to hear and how. Providing personalised sound to different people over loudspeakers enables communication, human connection and social activity in a shared space, meanwhile addressing the individuals’ needs. Recent developments in object-based audio, robust sound zoning algorithms, computer vision, device synchronisation and electronic hardware facilitate personal control of immersive and interactive reproduction techniques. Accordingly, the creative sector is moving towards more demand for personalisation and personalisable content. This tutorial offers participants a novel and timely introduction to the increasingly valuable capability to personalise sound over loudspeakers, alongside resources for the audio signal processing community. Presenting the science behind personalising sound technologies and providing insights for making sound zones in practice, we hope to create better listening experiences. The tutorial attempts a holistic exposition of techniques for producing personal sound over loudspeakers. It incorporates a practical step-by-step guide to digital filter design for real-world multizone sound reproduction and relates various approaches to one another thereby enabling comparison of the listener benefits.
Leveraging machine learning techniques, in the context of object-based media production, could enable provision of personalized media experiences to diverse audiences. To fine-tune and evaluate techniques for personalization applications, as well as more broadly, datasets which bridge the gap between research and production are needed. We introduce and publicly release such a dataset, themed around a UK weather forecast and shot against a blue-screen background, of three professional actors/presenters – one male and one female (English) and one female (British Sign Language). Scenes include both production and research-oriented examples, with a range of dialogue, motions, and actions. Capture techniques consisted of a synchronized 4K resolution 16-camera array, production-typical microphones plus professional audio mix, a 16-channel microphone array with collocated Grasshopper3 camera, and a photogrammetry array. We demonstrate applications relevant to virtual production and creation of personalized media including neural radiance fields, shadow casting, action/event detection, speaker source tracking and video captioning.
Immersive audio-visual perception relies on the spatial integration of both auditory and visual information which are heterogeneous sensing modalities with different fields of reception and spatial resolution. This study investigates the perceived coherence of audiovisual object events presented either centrally or peripherally with horizontally aligned/misaligned sound. Various object events were selected to represent three acoustic feature classes. Subjective test results in a simulated virtual environment from 18 participants indicate a wider capture region in the periphery, with an outward bias favoring more lateral sounds. Centered stimulus results support previous findings for simpler scenes.
This study considers the problem of detecting and locating an active talker's horizontal position from multichannel audio captured by a microphone array. We refer to this as active speaker detection and localization (ASDL). Our goal was to investigate the performance of spatial acoustic features extracted from the multichannel audio as the input of a convolutional recurrent neural network (CRNN), in relation to the number of channels employed and additive noise. To this end, experiments were conducted to compare the generalized cross-correlation with phase transform (GCC-PHAT), the spatial cue-augmented log-spectrogram (SALSA) features, and a recently-proposed beamforming method, evaluating their robustness to various noise intensities. The array aperture and sampling density were tested by taking subsets from the 16-microphone array. Results and tests of statistical significance demonstrate the microphones' contribution to performance on the TragicTalkers dataset, which offers opportunities to investigate audio-visual approaches in the future.
—Active speaker detection (ASD) is a multi-modal task that aims to identify who, if anyone, is speaking from a set of candidates. Current audiovisual approaches for ASD typically rely on visually pre-extracted face tracks (sequences of consecutive face crops) and the respective monaural audio. However, their recall rate is often low as only the visible faces are included in the set of candidates. Monaural audio may successfully detect the presence of speech activity but fails in localizing the speaker due to the lack of spatial cues. Our solution extends the audio front-end using a microphone array. We train an audio convolutional neural network (CNN) in combination with beamforming techniques to regress the speaker's horizontal position directly in the video frames. We propose to generate weak labels using a pre-trained active speaker detector on pre-extracted face tracks. Our pipeline embraces the " student-teacher " paradigm, where a trained " teacher " network is used to produce pseudo-labels visually. The " student " network is an audio network trained to generate the same results. At inference, the student network can independently localize the speaker in the visual frames directly from the audio input. Experimental results on newly collected data prove that our approach significantly outperforms a variety of other baselines as well as the teacher network itself. It results in an excellent speech activity detector too.
Audio-visual speaker tracking has drawn increasing attention over the past few years due to its academic values and wide application. Audio and visual modalities can provide complementary information for localization and tracking. With audio and visual information, the Bayesian-based filter can solve the problem of data association, audio-visual fusion and track management. In this paper, we conduct a comprehensive overview of audio-visual speaker tracking. To our knowledge, this is the first extensive survey over the past five years. We introduce the family of Bayesian filters and summarize the methods for obtaining audio-visual measurements. In addition, the existing trackers and their performance on AV16.3 dataset are summarized. In the past few years, deep learning techniques have thrived, which also boosts the development of audio visual speaker tracking. The influence of deep learning techniques in terms of measurement extraction and state estimation is also discussed. At last, we discuss the connections between audio-visual speaker tracking and other areas such as speech separation and distributed speaker tracking.
Sound event localization and detection (SELD) combines two subtasks: sound event detection (SED) and direction of arrival (DOA) estimation. SELD is usually tackled as an audio-only problem, but visual information has been recently included. Few audio-visual (AV)-SELD works have been published and most employ vision via face/object bounding boxes, or human pose keypoints. In contrast, we explore the integration of audio and visual feature embeddings extracted with pre-trained deep networks. For the visual modality, we tested ResNet50 and Inflated 3D ConvNet (I3D). Our comparison of AV fusion methods includes the AV-Conformer and Cross-Modal Attentive Fusion (CMAF) model. Our best models outperform the DCASE 2023 Task3 audio-only and AV baselines by a wide margin on the development set of the STARSS23 dataset, making them competitive amongst state-of-the-art results of the AV challenge, without model ensembling, heavy data augmentation, or prediction post-processing. Such techniques and further pre-training could be applied as next steps to improve performance.
Conventional audio-visual approaches for active speaker detection (ASD) typically rely on visually pre-extracted face tracks and the corresponding single-channel audio to find the speaker in a video. Therefore, they tend to fail every time the face of the speaker is not visible. We demonstrate that a simple audio convolutional recurrent neural network (CRNN) trained with spatial input features extracted from multichannel audio can perform simultaneous horizontal active speaker detection and localization (ASDL), independently of the visual modality. To address the time and cost of generating ground truth labels to train such a system, we propose a new self-supervised training pipeline that embraces a "student-teacher" learning approach. A conventional pre-trained active speaker detector is adopted as a "teacher" network to provide the position of the speakers as pseudo-labels. The multichannel audio "student" network is trained to generate the same results. At inference, the student network can generalize and locate also the occluded speakers that the teacher network is not able to detect visually, yielding considerable improvements in recall rate. Experiments on the TragicTalkers dataset show that an audio network trained with the proposed self-supervised learning approach can exceed the performance of the typical audio-visual methods and produce results competitive with the costly conventional supervised training. We demonstrate that improvements can be achieved when minimal manual supervision is introduced in the learning pipeline. Further gains may be sought with larger training sets and integrating vision with the multichannel audio system.
This study considers the problem of detecting and locating an active talker's horizontal position from multichannel audio captured by a microphone array. We refer to this as active speaker detection and localization (ASDL). Our goal was to investigate the performance of spatial acoustic features extracted from the multichan-nel audio as the input of a convolutional recurrent neural network (CRNN), in relation to the number of channels employed and additive noise. To this end, experiments were conducted to compare the generalized cross-correlation with phase transform (GCC-PHAT), the spatial cue-augmented log-spectrogram (SALSA) features, and a recently-proposed beamforming method, evaluating their robust-ness to various noise intensities. The array aperture and sampling density were tested by taking subsets from the 16-microphone array. Results and tests of statistical significance demonstrate the micro-phones' contribution to performance on the TragicTalkers dataset, which offers opportunities to investigate audiovisual approaches in the future.
Leveraging machine learning techniques, in the context of object-based media production, could enable provision of personalized media experiences to diverse audiences. To fine-tune and evaluate techniques for personalization applications, as well as more broadly, datasets which bridge the gap between research and production are needed. We introduce and publicly release such a dataset, themed around a UK weather forecast and shot against a blue-screen background, of three professional actors/presenters – one male and one female (English) and one female (British Sign Language). Scenes include both production and research-oriented examples, with a range of dialogue, motions, and actions. Capture techniques consisted of a synchronized 4K resolution 16-camera array, production-typical microphones plus professional audio mix, a 16-channel microphone array with collocated Grasshopper3 camera, and a photogrammetry array. We demonstrate applications relevant to virtual production and creation of personalized media including neural radiance fields, shadow casting, action/event detection, speaker source tracking and video captioning.
IEEE VR 2020 Immersive audio-visual perception relies on the spatial integration of both auditory and visual information which are heterogeneous sensing modalities with different fields of reception and spatial resolution. This study investigates the perceived coherence of audiovisual object events presented either centrally or peripherally with horizontally aligned/misaligned sound. Various object events were selected to represent three acoustic feature classes. Subjective test results in a simulated virtual environment from 18 participants indicate a wider capture region in the periphery, with an outward bias favoring more lateral sounds. Centered stimulus results support previous findings for simpler scenes.
This study considers the problem of detecting and locating an active talker's horizontal position from multichannel audio captured by a microphone array. We refer to this as active speaker detection and localization (ASDL). Our goal was to investigate the performance of spatial acoustic features extracted from the multichannel audio as the input of a convolutional recurrent neural network (CRNN), in relation to the number of channels employed and additive noise. To this end, experiments were conducted to compare the generalized cross-correlation with phase transform (GCC-PHAT), the spatial cue-augmented log-spectrogram (SALSA) features, and a recently-proposed beamforming method, evaluating their robustness to various noise intensities. The array aperture and sampling density were tested by taking subsets from the 16-microphone array. Results and tests of statistical significance demonstrate the microphones' contribution to performance on the TragicTalkers dataset, which offers opportunities to investigate audio-visual approaches in the future.
3D audiovisual production aims to deliver immersive and interactive experiences to the consumer. Yet, faithfully reproducing real-world 3D scenes remains a challenging task. This is partly due to the lack of available datasets enabling audiovisual research in this direction. In most of the existing multi-view datasets, the accompanying audio is neglected. Similarly, datasets for spatial audio research primarily offer unimodal content, and when visual data is included, the quality is far from meeting the standard production needs. We present " Tragic Talkers " , an audiovisual dataset consisting of excerpts from the " Romeo and Juliet " drama captured with microphone arrays and multiple co-located cameras for light-field video. Tragic Talkers provides ideal content for object-based media (OBM) production. It is designed to cover various conventional talking scenarios, such as monologues, two-people conversations, and interactions with considerable movement and occlusion, yielding 30 sequences captured from a total of 22 different points of view and two 16-element microphone arrays. Additionally, we provide voice activity labels, 2D face bounding boxes for each camera view, 2D pose detection keypoints, 3D tracking data of the mouth of the actors, and dialogue transcriptions. We believe the community will benefit from this dataset as it can assist multidisciplinary research. Possible uses of the dataset are discussed. * This is the author's version of the work. It is posted here for your personal use. This paper is published under a Creative Commons Attribution (CC-BY) license. The definitive version was published in CVMP '22, https://doi.org/10.1145/3565516.3565522.
Developments in object-based media and IP-based delivery offer an opportunity to create superior audience experiences through personalisation. Towards the aim of making personalised experiences regularly available across the breadth of audio-visual media, we conducted a study to understand how personalised experiences are being created. This consisted of interviews with producers of six representative case studies, followed by a thematic analysis. We describe the workflows and report on the producers’ experiences and obstacles faced. We found that the metadata models, enabling personalisation, were developed independently for each experience, restricting interoperability of personalisation affordances provided to users. Furthermore, the available tools were not effectively integrated into preferred workflows, substantially increasing role responsibilities and production time. To ameliorate these issues, we propose the development of a unifying metadata framework and novel production tools. These tools should be integrated into existing workflows; improve efficiency using AI; and enable producers to serve more diverse audiences.
Immersive audio-visual perception relies on the spatial integration of both auditory and visual information which are heterogeneous sensing modalities with different fields of reception and spatial resolution. This study investigates the perceived coherence of audio-visual object events presented either centrally or peripherally with horizontally aligned/misaligned sound. Various object events were selected to represent three acoustic feature classes. Subjective test results in a simulated virtual environment from 18 participants indicate a wider capture region in the periphery, with an outward bias favoring more lateral sounds. Centered stimulus results support previous findings for simpler scenes.
The spatial attribute envelopment has long been considered an important property of excellent concert hall acoustics. In the past, research in this area has produced the definition listener envelopment (LEV) and several equations designed to predict it. However with the recent development of multichannel audio systems capable of positioning sound sources all around the listener, it is apparent that the attribute is not so easily defined and that a more appropriate definition may be needed. This poster introduces a definition of envelopment more appropriate for multichannel audio and outlines a recent pilot experiment conducted by the authors.
The QESTRAL model is a perceptual model that aims to predict changes to spatial quality of service (SQoS) between the soundfield reproduced by a reference system and that of an impaired version of the reference system. To calibrate the model subjective data collected from listening tests is required. The QESTRAL model is designed to be format independent and therefore it relies on acoustical measurements of the reproduced soundfield derived using probe signals (or test signals). The measurements are used to create a series of perceptually motivated metrics, which are then fitted to the subjective data using a statistical model. This paper has two parts. The first part describes the implementation and results of a listening experiment designed to investigate changes to spatial quality. The second part, presents results from a calibration and forecasts prediction power (via cross-validation) of the QESTRAL model.
The audio scene from broadcast soccer can be used for identifying highlights from the game. Audio cues derived from these sources provide valuable information about game events, as can the detection of key words used by the commentators. In this paper we interpret the feasibility of incorporating both commentator word recognition and information about the additive background noise in an HMM structure. A limited set of audio cues, which have been extracted from data collected from the 2006 FIFA World Cup, are used to create an extension to the Aurora-2 database. The new database is then tested with various PMC models and compared to the standard baseline, clean and multi-condition training methods. It is found that incorporating SNR and noise type information into the PMC process is beneficial to recognition performance.
Spontaneous speech in videos capturing the speaker's mouth provides bimodal information. Exploiting the relationship between the audio and visual streams, we propose a new visual voice activity detection (VAD) algorithm, to overcome the vulnerability of conventional audio VAD techniques in the presence of background interference. First, a novel lip extraction algorithm combining rotational templates and prior shape constraints with active contours is introduced. The visual features are then obtained from the extracted lip region. Second, with the audio voice activity vector used in training, adaboosting is applied to the visual features, to generate a strong final voice activity classifier by boosting a set of weak classifiers. We have tested our lip extraction algorithm on the XM2VTS database (with higher resolution) and some video clips from YouTube (with lower resolution). The visual VAD was shown to offer low error rates.
Recognition of expressed emotion from speech and facial gestures was investigated in experiments on an audio-visual emotional database. A total of 106 audio and 240 visual features were extracted and then features were selected with Plus l-Take Away r algorithm based on Bhattacharyya distance criterion. In the second step, linear transformation methods, principal component analysis (PCA) and linear discriminant analysis (LDA), were applied to the selected features and Gaussian classifiers were used for classification of emotions. The performance was higher for LDA features compared to PCA features. The visual features performed better than audio features, for both PCA and LDA. Across a range of fusion schemes, the audio-visual feature results were close to that of visual features. A highest recognition rate of 53% was achieved with audio features, 98% with visual features, and 98% with audio-visual features selected by Bhattacharyya distance and transformed by LDA.
Estimating the geometric properties of an indoor environment through acoustic room impulse responses (RIRs) is useful in various applications, e.g., source separation, simultaneous localization and mapping, and spatial audio. Previously, we developed an algorithm to estimate the reflector’s position by exploiting ellipses as projection of 3D spaces. In this article, we present a model for full 3D reconstruction of environments. More specifically, the three components of the previous method, respectively, MUSIC for direction of arrival (DOA) estimation, numerical search adopted for reflector estimation and the Hough transform to refine the results, are extended for 3D spaces. A variation is also proposed using RANSAC instead of the numerical search and the Hough transform wich significantly reduces the run time. Both methods are tested on simulated and measured RIR data. The proposed methods perform better than the baseline, reducing the estimation error.
Recent progresses in Virtual Reality (VR) and Augmented Reality (AR) allow us to experience various VR/AR applications in our daily life. In order to maximise the immersiveness of user in VR/AR environments, a plausible spatial audio reproduction synchronised with visual information is essential. In this paper, we propose a simple and efficient system to estimate room acoustic for plausible reproducton of spatial audio using 360° cameras for VR/AR applications. A pair of 360° images is used for room geometry and acoustic property estimation. A simplified 3D geometric model of the scene is estimated by depth estimation from captured images and semantic labelling using a convolutional neural network (CNN). The real environment acoustics are characterised by frequency-dependent acoustic predictions of the scene. Spatially synchronised audio is reproduced based on the estimated geometric and acoustic properties in the scene. The reconstructed scenes are rendered with synthesised spatial audio as VR/AR content. The results of estimated room geometry and simulated spatial audio are evaluated against the actual measurements and audio calculated from ground-truth Room Impulse Responses (RIRs) recorded in the rooms.
Studies on sound field control methods able to create independent listening zones in a single acoustic space have recently been undertaken due to the potential of such methods for various practical applications, such as individual audio streams in home entertainment. Existing solutions to the problem have shown to be effective in creating high and low sound energy regions under anechoic conditions. Although some case studies in a reflective environment can also be found, the capabilities of sound zoning methods in rooms have not been fully explored. In this paper, the influence of low-order (early) reflections on the performance of key sound zone techniques is examined. Analytic considerations for small-scale systems reveal strong dependence of performance on parameters such as source positioning with respect to zone locations and room surfaces, as well as the parameters of the receiver configuration. These dependencies are further investigated through numerical simulation to determine system configurations which maximize the performance in terms of acoustic contrast and array control effort. The design rules for source and receiver positioning are suggested, for improved performance under a given set of constraints such as a number of available sources, zone locations and the direction of the dominant reflection. © 2013 Acoustical Society of America.
Object-based audio is an emerging representation for audio content, where content is represented in a reproductionformat- agnostic way and thus produced once for consumption on many different kinds of devices. This affords new opportunities for immersive, personalized, and interactive listening experiences. This article introduces an end-to-end object-based spatial audio pipeline, from sound recording to listening. A high-level system architecture is proposed, which includes novel audiovisual interfaces to support object-based capture and listenertracked rendering, and incorporates a proposed component for objectification, i.e., recording content directly into an object-based form. Text-based and extensible metadata enable communication between the system components. An open architecture for object rendering is also proposed. The system’s capabilities are evaluated in two parts. First, listener-tracked reproduction of metadata automatically estimated from two moving talkers is evaluated using an objective binaural localization model. Second, object-based scene capture with audio extracted using blind source separation (to remix between two talkers) and beamforming (to remix a recording of a jazz group), is evaluated with perceptually-motivated objective and subjective experiments. These experiments demonstrate that the novel components of the system add capabilities beyond the state of the art. Finally, we discuss challenges and future perspectives for object-based audio workflows.
The spatial quality of audio content delivery systems is becoming increasingly important as service providers attempt to deliver enhanced experiences of spatial immersion and naturalness in audio-visual applications. Examples are virtual reality, telepresence, home cinema, games and communications products. The QESTRAL project is developing an artificial listener that will compare the perceived quality of a spatial audio reproduction to a reference reproduction. The model is calibrated using data from listening tests, and utilises a range of metrics to predict the resulting spatial sound quality ratings. Potential application areas for the model are outlined, together with exemplary results obtained from some of its component parts.
Object-based audio promises format-agnostic reproduction and extensive personalization of spatial audio content. However, in practical listening scenarios, such as in consumer audio, ideal reproduction is typically not possible. To maximize the quality of listening experience, a different approach is required, for example modifications of metadata to adjust for the reproduction layout or personalization choices. In this paper we propose a novel system architecture for semantically informed rendering (SIR), that combines object audio rendering with high-level processing of object metadata. In many cases, this processing uses novel, advanced metadata describing the objects to optimally adjust the audio scene to the reproduction system or listener preferences. The proposed system is evaluated with several adaptation strategies, including semantically motivated downmix to layouts with few loudspeakers, manipulation of perceptual attributes, perceptual reverberation compensation, and orchestration of mobile devices for immersive reproduction. These examples demonstrate how SIR can significantly improve the media experience and provide advanced personalization controls, for example by maintaining smooth object trajectories on systems with few loudspeakers, or providing personalized envelopment levels. An example implementation of the proposed system architecture is described and provided as an open, extensible software framework that combines object-based audio rendering and high-level processing of advanced object metadata.
Sound field control to create multiple personal audio spaces (sound zones) in a shared listening environment is an active research topic. Typically, sound zones in the literature have aimed to reproduce monophonic audio programme material. The planarity control optimization approach can reproduce sound zones with high levels of acoustic contrast, while constraining the energy flux distribution in the target zone to impinge from a certain range of azimuths. Such a constraint has been shown to reduce problematic self-cancellation artefacts such as uneven sound pressure levels and complex phase patterns within the target zone. Furthermore, multichannel reproduction systems have the potential to reproduce spatial audio content at arbitrary listening positions (although most exclusively target a `sweet spot'). By designing the planarity control to constrain the impinging energy rather tightly, a sound field approximating a plane-wave can be reproduced for a listener in an arbitrarily-placed target zone. In this study, the application of planarity control for stereo reproduction in the context of a personal audio system was investigated. Four solutions, to provide virtual left and right channels for two audio programmes, were calculated and superposed to achieve the stereo effect in two separate sound zones. The performance was measured in an acoustically treated studio using a 60 channel circular array, and compared against a least-squares pressure matching solution whereby each channel was reproduced as a plane wave field. Results demonstrate that planarity control achieved 6 dB greater mean contrast than the least-squares case over the range 250-2000 Hz. Based on the principal directions of arrival across frequency, planarity control produced azimuthal RMSE of 4.2/4.5 degrees for the left/right channels respectively (least-squares 2.8/3.6 degrees). Future work should investigate the perceived spatial quality of the implemented system with respect to a reference stereophonic setup.
Audio systems and recordings are optimized for listening at the ’sweet spot’, but how well do they work elsewhere? An acoustic-perceptual model has been developed that simulates sound reproduction in a variety of formats, including mono, two-channel stereo, five-channel surround and wavefield synthesis. A virtual listener placed anywhere in the listening area is used to extract binaural signals, and hence interaural cues to the spatial attributes of the soundfield. Using subjectively-validated models of spatial sound perception, we can predict the way that human listeners would perceive these attributes, such as the direction (azimuth) and width of a phantom source. Results will be presented across the listening area for different source signals, sound scenes and reproduction systems, illustrating their spatial fidelity in perceptual terms. Future work investigates the effects of typical reproduction degradations.
A binaural sound source localization method is proposed that uses interaural and spectral cues for localization of sound sources with any direction of arrival on the full-sphere. The method is designed to be robust to the presence of reverberation, additive noise and different types of sounds. The method uses the interaural phase difference (IPD) for lateral angle localization, then interaural and spectral cues for polar angle localization. The method applies different weighting to the interaural and spectral cues depending on the estimated lateral angle. In particular, only the spectral cues are used for sound sources near or on the median plane.
Humans are able to identify a large number of environmental sounds and categorise them according to high-level semantic categories, e.g. urban sounds or music. They are also capable of generalising from past experience to new sounds when applying these categories. In this paper we report on the creation of a data set that is structured according to the top-level of a taxonomy derived from human judgements and the design of an associated machine learning challenge, in which strong generalisation abilities are required to be successful. We introduce a baseline classification system, a deep convolutional network, which showed strong performance with an average accuracy on the evaluation data of 80.8%. The result is discussed in the light of two alternative explanations: An unlikely accidental category bias in the sound recordings or a more plausible true acoustic grounding of the high-level categories.
Spatial audio processes (SAPs) commonly encountered in consumer audio reproduction systems are known to generate a range of impairments to spatial quality. Two listening tests (involving two listening positions, six 5-channel audio recordings, and 48 SAPs) indicate that the degree of quality degradation is determined largely by the nature of the SAP but that the effect of a particular SAP can depend on program material and on listening position. Combining off-center listening with another SAP can reduce spatial quality significantly compared to auditioning that SAP centrally. These findings, and the associated listening test data, can guide the development of an artificial-listener-based spatial audio quality evaluation system.
Reproduction of multiple sound zones, in which personal audio programs may be consumed without the need for headphones, is an active topic in acoustical signal processing. Many approaches to sound zone reproduction do not consider control of the bright zone phase, which may lead to self-cancellation problems if the loudspeakers surround the zones. Conversely, control of the phase in a least-squares sense comes at a cost of decreased level difference between the zones and frequency range of cancellation. Single-zone approaches have considered plane wave reproduction by focusing the sound energy in to a point in the wavenumber domain. In this article, a planar bright zone is reproduced via planarity control, which constrains the bright zone energy to impinge from a narrow range of angles via projection in to a spatial domain. Simulation results using a circular array surrounding two zones show the method to produce superior contrast to the least-squares approach, and superior planarity to the contrast maximization approach. Practical performance measurements obtained in an acoustically treated room verify the conclusions drawn under free-field conditions.
The ventriloquism effect describes the phenomenon of audio and visual signals with common features, such as a voice and a talking face merging perceptually into one percept even if they are spatially misaligned. The boundaries of the fusion of spatially misaligned stimuli are of interest for the design of multimedia products to ensure a perceptually satisfactory product. They have mainly been studied using continuous judgment scales and forced-choice measurement methods. These results vary greatly between different studies. The current experiment aims to evaluate audio-visual fusion using reaction time (RT) measurements as an indirect method of measurement to overcome these great variances. A two-alternative forced-choice (2AFC) word recognition test was designed and tested with noise and multi-talker speech background distractors. Visual signals were presented centrally and audio signals were presented between 0° and 31° audio-visual offset in azimuth. RT data were analyzed separately for the underlying Simon effect and attentional effects. In the case of the attentional effects, three models were identified but no single model could explain the observed RTs for all participants so data were grouped and analyzed accordingly. The results show that significant differences in RTs are measured from 5° to 10° onwards for the Simon effect. The attentional effect varied at the same audio-visual offset for two out of the three defined participant groups. In contrast with the prior research, these results suggest that, even for speech signals, small audio-visual offsets influence spatial integration subconsciously.
Human communication is based on verbal and nonverbal information, e.g., facial expressions and intonation cue the speaker’s emotional state. Important speech features for emotion recognition are prosody (pitch, energy and duration) and voice quality (spectral energy, formants, MFCCs, jitter/shimmer). For facial expressions, features related to forehead, eye region, cheek and lip are important. Both audio and visual modalities provide relevant cues. Thus, audio and visual features were extracted and combined to evaluate emotion recognition on a British English corpus. The database of 120 utterances was recorded from an actor with 60 markers painted on his face, reading sentences in seven emotions (N=7): anger, disgust, fear, happiness, neutral, sadness and surprise. Recordings consisted of 15 phonetically-balanced TIMIT sentences per emotion, and video of the face captured by a 3dMD system. A total of 106 utterance-level audio features (prosodic and spectral) and 240 visual features (2D marker coordinates) were extracted. Experiments were performed with audio, visual and audiovisual features. The top 40 features were selected by sequential forward backward search using Bhattacharyya distance criterion. PCA and LDA transformations, calculated on the training data, were applied. Gaussian classifiers were trained with PCA and LDA features. Data was jack-knifed with 5 sets for training and 1 set for testing. Results were averaged over 6 tests. The emotion recognition accuracy was higher for visual features than audio features, for both PCA and LDA. Audiovisual results were close to those with visual features. Higher performance was achieved with LDA compared to PCA. The best recognition rate, 98%, was achieved for 6 LDA features (N-1) with audiovisual and visual features, whereas audio LDA scored 53%. Maximum PCA results for audio, visual and audiovisual features were 41%, 97% and 88% respectively. Future work involves experiments with more subjects and investigating the correlation between vocal and facial expressions of emotion.
In this paper, we compare different deep neural networks (DNN) in extracting speech signals from competing speakers in room environments, including the conventional fullyconnected multilayer perception (MLP) network, convolutional neural network (CNN), recurrent neural network (RNN), and the recently proposed capsule network (CapsNet). Each DNN takes input of both spectral features and converted spatial features that are robust to position mismatch, and outputs the separation mask for target source estimation. In addition, a psychacoustically-motivated objective function is integrated in each DNN, which explores perceptual importance of each TF unit in the training process. Objective evaluations are performed on the separated sounds using the converged models, in terms of PESQ, SDR as well as STOI. Overall, all the implemented DNNs have greatly improved the quality and speech intelligibility of the embedded target source as compared to the original recordings. In particular, bidirectional RNN, either along the temporal direction or along the frequency bins, outperforms the other DNN structures with consistent improvement.
Microphone array beamforming can be used to enhance and separate sound sources, with applications in the capture of object-based audio. Many beamforming methods have been proposed and assessed against each other. However, the effects of compact microphone array design on beamforming performance have not been studied for this kind of application. This study investigates how to maximize the quality of audio objects extracted from a horizontal sound field by filter-and-sum beamforming, through appropriate choice of microphone array design. Eight uniform geometries with practical constraints of a limited number of microphones and maximum array size are evaluated over a range of physical metrics. Results show that baffled circular arrays outperform the other geometries in terms of perceptually relevant frequency range, spatial resolution, directivity and robustness. Moreover, a subjective evaluation of microphone arrays and beamformers is conducted with regards to the quality of the target sound, interference suppression and overall quality of simulated music performance recordings. Baffled circular arrays achieve higher target quality and interference suppression than alternative geometries with wideband signals. Furthermore, subjective scores of beamformers regarding target quality and interference suppression agree well with beamformer onaxis and off-axis responses; with wideband signals the superdirective beamformer achieves the highest overall quality.
In object-based spatial audio system, positions of the audio objects (e.g. speakers/talkers or voices) presented in the sound scene are required as important metadata attributes for object acquisition and reproduction. Binaural microphones are often used as a physical device to mimic human hearing and to monitor and analyse the scene, including localisation and tracking of multiple speakers. The binaural audio tracker, however, is usually prone to the errors caused by room reverberation and background noise. To address this limitation, we present a multimodal tracking method by fusing the binaural audio with depth information (from a depth sensor, e.g., Kinect). More specifically, the PHD filtering framework is first applied to the depth stream, and a novel clutter intensity model is proposed to improve the robustness of the PHD filter when an object is occluded either by other objects or due to the limited field of view of the depth sensor. To compensate mis-detections in the depth stream, a novel gap filling technique is presented to map audio azimuths obtained from the binaural audio tracker to 3D positions, using speaker-dependent spatial constraints learned from the depth stream. With our proposed method, both the errors in the binaural tracker and the mis-detections in the depth tracker can be significantly reduced. Real-room recordings are used to show the improved performance of the proposed method in removing outliers and reducing mis-detections.
In this paper we describe a method for the synthesis of visual speech movements using a hybrid unit selection/model-based approach. Speech lip movements are captured using a 3D stereo face capture system, and split up into phonetic units. A dynamic parameterisation of this data is constructed which maintains the relationship between lip shapes and velocities; within this parameterisation a model of how lips move is built and is used in the animation of visual speech movements from speech audio input. The mapping from audio parameters to lip movements is disambiguated by selecting only the most similar stored phonetic units to the target utterance during synthesis. By combining properties of model-based synthesis (e.g. HMMs, neural nets) with unit selection we improve the quality of our speech synthesis.
In this paper we describe a parameterisation of lip movements which maintains the dynamic structure inherent in the task of producing speech sounds. A stereo capture system is used to reconstruct 3D models of a speaker producing sentences from the TIMIT corpus. This data is mapped into a space which maintains the relationships between samples and their temporal derivatives. By incorporating dynamic information within the parameterisation of lip movements we can model the cyclical structure, as well as the causal nature of speech movements as described by an underlying visual speech manifold. It is believed that such a structure will be appropriate to various areas of speech modeling, in particular the synthesis of speech lip movements.
This paper describes a computational model for the prediction of perceived spatial quality for reproduced sound at arbitrary locations in the listening area. The model is specifically designed to evaluate distortions in the spatial domain such as changes in source location, width and envelopment. Maps of perceived spatial quality across the listening area are presented from our initial results.
Audio from broadcast soccer can be used for identifying highlights from the game. We can assume that the basic construction of the auditory scene consists of two additive parallel audio streams, one relating to commentator speech and the other relating to audio captured from the ground level microphones. Audio cues derived from these sources provide valuable information about game events, as can the detection of key words used by the commentators, which are useful for identifying highlights. We investigate word recognition in a connected digit experiment providing additive noise that is present in broadcast soccer audio. A limited set of background soccer noises, extracted from the FIFA World Cup 2006 recordings, were used to create an extension to the Aurora-2 database. The extended data set was tested with various HMM and parallel model combination (PMC) configurations, and compared to the standard baseline, with clean and multi-condition training methods. It was found that incorporating SNR and noise type information into the PMC process was beneficial to recognition performance with a reduction in word error rate from 17.5% to 16.3% over the next best scheme when using the SNR information.Future work will look at non stationary soccer noise types and multiple statenoise models.
The QESTRAL project has developed an artificial listener that compares the perceived quality of a spatial audio reproduction to a reference reproduction. Test signals designed to identify distortions in both the foreground and background audio streams are created for both the reference and the impaired reproduction systems. Metrics are calculated from these test signals and are then combined using a regression model to give a measure of the overall perceived spatial quality of the impaired reproduction compared to the reference reproduction. The results of the model are shown to match closely the results obtained in listening tests. Consequently, the model can be used as an alternative to listening tests when evaluating the perceived spatial quality of a given reproduction system, thus saving time and expense.
It is of interest to create regions of increased and reduced sound pressure ('sound zones') in an enclosure such that different audio programs can be simultaneously delivered over loudspeakers, thus allowing listeners sharing a space to receive independent audio without physical barriers or headphones. Where previous comparisons of sound zoning techniques exist, they have been conducted under favorable acoustic conditions, utilizing simulations based on theoretical transfer functions or anechoic measurements. Outside of these highly specified and controlled environments, real-world factors including reflections, measurement errors, matrix conditioning and practical filter design degrade the realizable performance. This study compares the performance of sound zoning techniques when applied to create two sound zones in simulated and real acoustic environments. In order to compare multiple methods in a common framework without unduly hindering performance, an optimization procedure for each method is first used to select the best loudspeaker positions in terms of robustness, efficiency and the acoustic contrast deliverable to both zones. The characteristics of each control technique are then studied, noting the contrast and the impact of acoustic conditions on performance.
A binaural sound source localization method is proposed that uses interaural and spectral cues for localization of sound sources with any direction of arrival on the full-sphere. The method is designed to be robust to the presence of reverberation, additive noise and different types of sounds. The method uses the interaural phase difference (IPD) for lateral angle localization, then interaural and spectral cues for polar angle localization. The method applies different weighting to the interaural and spectral cues depending on the estimated lateral angle. In particular, only the spectral cues are used for sound sources near or on the median plane.
A physical model, built to investigate the aeroacoustic properties of voiced fricative speech, was used to study the amplitude modulation of the turbulence noise it generated. The amplitude and fundamental frequency of glottal vibration, relative positions of the constriction and obstacle, and the flow rate were varied. Measurements were made from pressure taps in the duct wall and the sound pressure at the open end. The high-pass filtered sound pressure was analyzed in terms of the magnitude and phase of the turbulence noise envelope. The magnitude and phase of the observed modulation was related to the upstream pressure. The effects of moving the obstacle with respect to the constriction are reported (representative of the teeth and the tongue in a sibilant fricative respectively). These results contribute to the development of a parametric model of the aeroacoustic interaction of voicing with turbulence noise generation in speech.
Recent attention to the problem of controlling multiple loudspeakers to create sound zones has been directed towards practical issues arising from system robustness concerns. In this study, the effects of regularization are analyzed for three representative sound zoning methods. Regularization governs the control effort required to drive the loudspeaker array, via a constraint in each optimization cost function. Simulations show that regularization has a significant effect on the sound zone performance, both under ideal anechoic conditions and when systematic errors are introduced between calculation of the source weights and their application to the system. Results are obtained for speed of sound variations and loudspeaker positioning errors with respect to the source weights calculated. Judicious selection of the regularization parameter is shown to be a primary concern for sound zone system designers - the acoustic contrast can be increased by up to 50dB with proper regularization in the presence of errors. A frequency-dependent minimum regularization parameter is determined based on the conditioning of the matrix inverse. The regularization parameter can be further increased to improve performance depending on the control effort constraints, expected magnitude of errors, and desired sound field properties of the system. © 2013 Acoustical Society of America.
The ability to predict the acoustics of a room without acoustical measurements is a useful capability. The motivation here stems from spatial audio reproduction, where knowledge of the acoustics of a space could allow for more accurate reproduction of a captured environment, or for reproduction room compensation techniques to be applied. A cuboid-based room geometry estimation method using a spherical camera is proposed, assuming a room and objects inside can be represented as cuboids aligned to the main axes of the coordinate system. The estimated geometry is used to produce frequency-dependent acoustic predictions based on geometrical room modelling techniques. Results are compared to measurements through calculated reverberant spatial audio object parameters used for reverberation reproduction customized to the given loudspeaker set up.
We present a novel method for speech separation from their audio mixtures using the audio-visual coherence. It consists of two stages: in the off-line training process, we use the Gaussian mixture model to characterise statistically the audio-visual coherence with features obtained from the training set; at the separation stage, likelihood maximization is performed on the independent component analysis (ICA)-separated spectral components. To address the permutation and scaling indeterminacies of the frequency-domain blind source separation (BSS), a new sorting and rescaling scheme using the bimodal coherence is proposed.We tested our algorithm on the XM2VTS database, and the results show that our algorithm can address the permutation problem with high accuracy, and mitigate the scaling problem effectively.
Virtual Reality (VR) systems have been intensely explored, with several research communities investigating the different modalities involved. Regarding the audio modality, one of the main issues is the generation of sound that is perceptually coherent with the visual reproduction. Here, we propose a pipeline for creating plausible interactive reverb using visual information: first, we characterize real environment acoustics given a pair of spherical cameras; then, we reproduce reverberant spatial sound, by using the estimated acoustics, within a VR scene. The evaluation is made by extracting the room impulse responses (RIRs) of four virtually rendered rooms. Results show agreement, in terms of objective metrics, between the synthesized acoustics and the ones calculated from RIRs recorded within the respective real rooms.
When a noise process is modulated by a deterministic signal, it is often useful to determine the signal's parameters. A method of estimating the modulation index m is presented for noise whose amplitude is modulated by a periodic signal, using the magnitude modulation spectrum (MMS). The method is developed for application to real discrete signals with time- varying parameters, and extended to a 3D time-frequency- modulation representation. In contrast to squared-signal approaches, MMS behaves linearly with the modulating function allowing separate estimation of m for each harmonic. Simulations evaluate performance on synthetic signals, compared with theory, favouring a first-order MMS estimator.
In this paper the mixing vector (MV) in the statistical mixing model is compared to the binaural cues represented by interaural level and phase differences (ILD and IPD). It is shown that the MV distributions are quite distinct while binaural models overlap when the sources are close to each other. On the other hand, the binaural cues are more robust to high reverberation than MV models. According to this complementary behavior we introduce a new robust algorithm for stereo speech separation which considers both additive and convolutive noise signals to model the MV and binaural cues in parallel and estimate probabilistic time-frequency masks. The contribution of each cue to the final decision is also adjusted by weighting the log-likelihoods of the cues empirically. Furthermore, the permutation problem of the frequency domain blind source separation (BSS) is addressed by initializing the MVs based on binaural cues. Experiments are performed systematically on determined and underdetermined speech mixtures in five rooms with various acoustic properties including anechoic, highly reverberant, and spatially-diffuse noise conditions. The results in terms of signal-to-distortion-ratio (SDR) confirm the benefits of integrating the MV and binaural cues, as compared with two state-of-the-art baseline algorithms which only use MV or the binaural cues.
Planarity panning (PP) and planarity control (PC) have previously been shown to be efficient methods for focusing directional sound energy into listening zones. In this paper, we consider sound field control for two listeners. First, PP is extended to create spatial audio for two listeners consuming the same spatial audio content. Then, PC is used to create highly directional sound and cancel interfering audio. Simulation results compare PP and PC against pressure matching (PM) solutions. For multiple listeners listening to the same content, PP creates directional sound at lower effort than the PM counterpart. When listeners consume different audio, PC produces greater acoustic contrast than PM, with excellent directional control except for frequencies where grating lobes generate problematic interference patterns.
Studies on perceived audio-visual spatial coherence in the literature have commonly employed continuous judgment scales. This method requires listeners to detect and to quantify their perception of a given feature and is a difficult task, particularly for untrained listeners. An alternative method is the quantification of a percept by conducting a simple forced choice test with subsequent modeling of the psychometric function. An experiment to validate this alternative method for the perception of azimuthal audio-visual spatial coherence was performed. Furthermore, information on participant training and localization ability was gathered. The results are consistent with previous research and show that the proposed methodology is suitable for this kind of test. The main differences between participants result from the presence or absence of musical training.
In this paper we present a system for localization and separation of multiple speech sources using phase cues. The novelty of this method is the use of Random Sample Consensus (RANSAC) approach to find consistency of interaural phase differences (IPDs) across the whole frequency range. This approach is inherently free from phase ambiguity problems and enables all phase data to contribute to localization. Another property of RANSAC is its robustness against outliers which enables multiple source localization with phase data contaminated by reverberation noise. Results of RANSAC based localization are fed into a mixture model to generate time-frequency binary masks for separation. System performance is compared against other well known methods and shows similar or improved performance in reverberant conditions.
Whilst sound zoning methods have typically been studied under anechoic conditions, it is desirable to evaluate the performance of various methods in a real room. Three control methods were implemented (delay and sum, DS; acoustic contrast control, ACC; and pressure matching, PM) on two regular 24-element loudspeaker arrays (line and circle). The acoustic contrast between two zones was evaluated and the reproduced sound fields compared for uniformity of energy distribution. ACC generated the highest contrast, whilst PM produced a uniform bright zone. Listening tests were also performed using monophonic auralisations from measured system responses to collect ratings of perceived distraction due to the alternate audio programme. Distraction ratings were affected by control method and programme material. Copyright © (2013) by the Audio Engineering Society.
The ability to replicate a plane wave represents an essential element of spatial sound field reproduction. In sound field synthesis, the desired field is often formulated as a plane wave and the error minimized; for other sound field control methods, the energy density or energy ratio is maximized. In all cases and further to the reproduction error, it is informative to characterize how planar the resultant sound field is. This paper presents a method for quantifying a region's acoustic planarity by superdirective beamforming with an array of microphones, which analyzes the azimuthal distribution of impinging waves and hence derives the planarity. Estimates are obtained for a variety of simulated sound field types, tested with respect to array orientation, wavenumber, and number of microphones. A range of microphone configurations is examined. Results are compared with delay-and-sum beamforming, which is equivalent to spatial Fourier decomposition. The superdirective beamformer provides better characterization of sound fields, and is effective with a moderate number of omni-directional microphones over a broad frequency range. Practical investigation of planarity estimation in real sound fields is needed to demonstrate its validity as a physical sound field evaluation measure. © 2013 Acoustical Society of America.
We present PAT, a transformer-based network that learns complex temporal co-occurrence action dependencies in a video by exploiting multi-scale temporal features. In existing methods, the self-attention mechanism in transformers loses the temporal positional information, which is essential for robust action detection. To address this issue, we (i) embed relative positional encoding in the self-attention mechanism and (ii) exploit multi-scale temporal relationships by designing a novel non-hierarchical network, in contrast to the recent transformer-based approaches that use a hierarchical structure. We argue that joining the self-attention mechanism with multiple sub-sampling processes in the hierarchical approaches results in increased loss of positional information. We evaluate the performance of our proposed approach on two challenging dense multi-label benchmark datasets, and show that PAT improves the current state-of-the-art result by 1.1% and 0.6% mAP on the Charades and MultiTHUMOS datasets, respectively, thereby achieving the new state-of-the-art mAP at 26.5% and 44.6%, respectively. We also perform extensive ablation studies to examine the impact of the different components of our proposed network.
Virtual Reality (VR) systems have been intensely explored, with several research communities investigating the different modalities involved. Regarding the audio modality, one of the main issues is the generation of sound that is perceptually coherent with the visual reproduction. Here, we propose a pipeline for creating plausible interactive reverb using visual information: first, we characterize real environment acoustics given a pair of spherical cameras; then, we reproduce reverberant spatial sound, by using the estimated acoustics, within a VR scene. The evaluation is made by extracting the room impulse responses (RIRs) of four virtually rendered rooms. Results show agreement, in terms of objective metrics, between the synthesized acoustics and the ones calculated from RIRs recorded within the respective real rooms.
In order to maximise the immersion in VR environments, a plausible spatial audio reproduction synchronised with visual information is essential. In this work, we propose a pipeline to create plausible interactive audio from a pair of 360 degree cameras.
This paper explores the recognition of expressed emotion from speech and facial gestures for the speaker-dependent case. Experiments were performed on an English audio-visual emotional database consisting of 480 utterances from 4 English male actors in 7 emotions. A total of 106 audio and 240 visual features were extracted and features were selected with Plus l-Take Away r algorithm based on Bhattacharyya distance criterion. Linear transformation methods, principal component analysis (PCA) and linear discriminant analysis (LDA), were applied to the selected features and Gaussian classifiers were used for classification. The performance was higher for LDA features compared to PCA features. The visual features performed better than the audio features and overall performance improved for the audio-visual features. In case of 7 emotion classes, an average recognition rate of 56% was achieved with the audio features, 95% with the visual features and 98% with the audio-visual features selected by Bhattacharyya distance and transformed by LDA. Grouping emotions into 4 classes, an average recognition rate of 69% was achieved with the audio features, 98% with the visual features and 98% with the audio-visual features fused at decision level. The results were comparable to the measured human recognition rate with this multimodal data set.
In applications such as virtual and augmented reality, a plausible and coherent audio-visual reproduction can be achieved by deeply understanding the reference scene acoustics. This requires knowledge of the scene geometry and related materials. In this paper, we present an audio-visual approach for acoustic scene understanding. We propose a novel material recognition algorithm, that exploits information carried by acoustic signals. The acoustic absorption coefficients are selected as features. The training dataset was constructed by combining information available in the literature, and additional labeled data that we recorded in a small room having short reverberation time (RT60). Classic machine learning methods are used to validate the model, by employing data recorded in five rooms, having different sizes and RT60s. The estimated materials are utilized to label room boundaries, reconstructed by a visionbased method. Results show 89 % and 80 % agreement between the estimated and reference room volumes and materials, respectively.
The QESTRAL project aims to develop an artificial listener for comparing the perceived quality of a spatial audio reproduction against a reference reproduction. This paper presents implementation details for simulating the acoustics of the listening environment and the listener’s auditory processing. Acoustical modeling is used to calculate binaural signals and simulated microphone signals at the listening position, from which a number of metrics corresponding to different perceived spatial aspects of the reproduced sound field are calculated. These metrics are designed to describe attributes associated with location, width and envelopment attributes of a spatial sound scene. Each provides a measure of the perceived spatial quality of the impaired reproduction compared to the reference reproduction. As validation, individual metrics from listening test signals are shown to match closely subjective results obtained, and can be used to predict spatial quality for arbitrary signals.
For subjective experimentation on 3D audio systems, suitable programme material is needed. A large-scale recording session was performed in which four ensembles were recorded with a range of existing microphone techniques (aimed at mono, stereo, 5.0, 9.0, 22.0, ambisonic, and headphone reproduction) and a novel 48-channel circular microphone array. Further material was produced by remixing and augmenting pre-existing multichannel content. To mix and monitor the programme items (which included classical, jazz, pop and experimental music, and excerpts from a sports broadcast and a lm soundtrack), a flexible 3D audio reproduction environment was created. Solutions to the following challenges were found: level calibration for different reproduction formats; bass management; and adaptable signal routing from different software and fille formats.
This paper describes a computational model for the prediction of perceived spatial quality for reproduced sound at arbitrary locations in the listening area. The model is specifically designed to evaluate distortions in the spatial domain such as changes in source location, width and envelopment. Maps of perceived spatial quality across the listening area are presented from our initial results.
Audio (spectral) and modulation (envelope) frequencies both carry information in a speech signal. While low modulation frequencies (2-20Hz) convey syllable information, higher modulation frequencies (80-400Hz) allow for assimilation of perceptual cues, e.g., the roughness of amplitude-modulated noise in voiced fricatives, considered here. Psychoacoustic 3-interval forced-choice experiments measured AM detection thresholds for modulated noise accompanied by a tone with matching fundamental frequency at 125Hz: (1) tone-to-noise ratio (TNR) and phase between tone and noise envelope were varied, with silence between intervals; (2) as (1) with continuous tone throughout each trial; (3) duration and noise spectral shape were varied. Results from (1) showed increased threshold (worse detection) for louder tones (40-50dB TNR). In (2), a similar effect was observed for the in-phase condition, but out-of-phase AM detection appeared immune to the tone. As expected, (3) showed increased thresholds for shorter tokens, although still detectable at 60ms, and no effect for spectral shape. The phase effect of (2) held for the short stimuli, with implications for fricative speech tokens (40ms-100ms). Further work will evaluate the strength of this surprisingly robust cue in speech.
Automatic and fast tagging of natural sounds in audio collections is a very challenging task due to wide acoustic variations, the large number of possible tags, the incomplete and ambiguous tags provided by different labellers. To handle these problems, we use a co-regularization approach to learn a pair of classifiers on sound and text. The first classifier maps low-level audio features to a true tag list. The second classifier maps actively corrupted tags to the true tags, reducing incorrect mappings caused by low-level acoustic variations in the first classifier, and to augment the tags with additional relevant tags. Training the classifiers is implemented using marginal co-regularization, pair of which draws the two classifiers into agreement by a joint optimization. We evaluate this approach on two sound datasets, Freefield1010 and Task4 of DCASE2016. The results obtained show that marginal co-regularization outperforms the baseline GMM in both ef- ficiency and effectiveness.
Recent work into 3D audio reproduction has considered the definition of a set of parameters to encode reverberation into an object-based audio scene. The reverberant spatial audio object (RSAO) describes the reverberation in terms of a set of localised, delayed and filtered (early) reflections, together with a late energy envelope modelling the diffuse late decay. The planarity metric, originally developed to evaluate the directionality of reproduced sound fields, is used to analyse a set of multichannel room impulse responses (RIRs) recorded at a microphone array. Planarity describes the spatial compactness of incident sound energy, which tends to decrease as the reflection density and diffuseness of the room response develop over time. Accordingly, planarity complements intensity-based diffuseness estimators, which quantify the degree to which the sound field at a discrete frequency within a particular time window is due to an impinging coherent plane wave. In this paper, we use planarity as a tool to analyse the sound field in relation to the RSAO parameters. Specifically, we use planarity to estimate two important properties of the sound field. First, as high planarity identifies the most localised reflections along the RIR, we estimate the most planar portions of the RIR, corresponding to the RSAO early reflection model and increasing the likelihood of detecting prominent specular reflections. Second, as diffuse sound fields give a low planarity score, we investigate planarity for data-based mixing time estimation. Results show that planarity estimates on measured multichannel RIR datasets represent a useful tool for room acoustics analysis and RSAO parameterisation.
We present PAT, a transformer-based network that learns complex temporal co-occurrence action dependencies in a video by exploiting multi-scale temporal features. In existing methods, the self-attention mechanism in transformers loses the temporal positional information, which is essential for robust action detection. To address this issue, we (i) embed relative positional encoding in the self-attention mechanism and (ii) exploit multi-scale temporal relationships by designing a novel non hierarchical network, in contrast to the recent transformer-based approaches that use a hierarchical structure. We argue that joining the self-attention mechanism with multiple sub-sampling processes in the hierarchical approaches results in increased loss of positional information. We evaluate the performance of our proposed approach on two challenging dense multi-label benchmark datasets, and show that PAT improves the current state-of-the-art result by 1.1% and 0.6% mAP on the Charades and MultiTHUMOS datasets, respectively, thereby achieving the new state-of-the-art mAP at 26.5% and 44.6%, respectively. We also perform extensive ablation studies to examine the impact of the different components of our proposed network.
Object-based audio can be used to customize, personalize, and optimize audio reproduction depending on the speci?c listening scenario. To investigate and exploit the bene?ts of object-based audio, a framework for intelligent metadata adaptation was developed. The framework uses detailed semantic metadata that describes the audio objects, the loudspeakers, and the room. It features an extensible software tool for real-time metadata adaptation that can incorporate knowledge derived from perceptual tests and/or feedback from perceptual meters to drive adaptation and facilitate optimal rendering. One use case for the system is demonstrated through a rule-set (derived from perceptual tests with experienced mix engineers) for automatic adaptation of object levels and positions when rendering 3D content to two- and ?ve-channel systems.
Acoustic reflector localization is an important issue in audio signal processing, with direct applications in spatial audio, scene reconstruction, and source separation. Several methods have recently been proposed to estimate the 3D positions of acoustic reflectors given room impulse responses (RIRs). In this article, we categorize these methods as “image-source reversion”, which localizes the image source before finding the reflector position, and “direct localization”, which localizes the reflector without intermediate steps. We present five new contributions. First, an onset detector, called the clustered dynamic programming projected phase-slope algorithm, is proposed to automatically extract the time of arrival for early reflections within the RIRs of a compact microphone array. Second, we propose an image-source reversion method that uses the RIRs from a single loudspeaker. It is constructed by combining an image source locator (the image source direction and range (ISDAR) algorithm), and a reflector locator (using the loudspeaker-image bisection (LIB) algorithm). Third, two variants of it, exploiting multiple loudspeakers, are proposed. Fourth, we present a direct localization method, the ellipsoid tangent sample consensus (ETSAC), exploiting ellipsoid properties to localize the reflector. Finally, systematic experiments on simulated and measured RIRs are presented, comparing the proposed methods with the state-of-the-art. ETSAC generates errors lower than the alternative methods compared through our datasets. Nevertheless, the ISDAR-LIB combination performs well and has a run time 200 times faster than ETSAC.
Whilst it is possible to create exciting, immersive listening experiences with current spatial audio technology, the required systems are generally difficult to install in a standard living room. However, in any living room there is likely to already be a range of loudspeakers (such as mobile phones, tablets, laptops, and so on). ____Media device orchestration" (MDO) is the concept of utilising all available devices to augment the reproduction of a media experience. In this demonstration, MDO is used to augment low channel count renderings of various programme material, delivering immersive three-dimensional audio experiences.
Estimating and parameterizing the early and late reflections of an enclosed space is an interesting topic in acoustics. With a suitable set of parameters, the current concept of a spatial audio object (SAO), which is typically limited to either direct (dry) sound or diffuse field components, could be extended to afford an editable spatial description of the room acoustics. In this paper we present an analysis/synthesis method for parameterizing a set of measured room impulse responses (RIRs). RIRs were recorded in a medium-sized auditorium, using a uniform circular array of microphones representing the perspective of a listener in the front row. During the analysis process, these RIRs were decomposed, in time, into three parts: the direct sound, the early reflections, and the late reflections. From the direct sound and early reflections, parameters were extracted for the length, amplitude, and direction of arrival (DOA) of the propagation paths by exploiting the dynamic programming projected phase-slope algorithm (DYPSA) and classical delay-and-sum beamformer (DSB). Their spectral envelope was calculated using linear predictive coding (LPC). Late reflections were modeled by frequency-dependent decays excited by band-limited Gaussian noise. The combination of these parameters for a given source position and the direct source signal represents the reverberant or “wet” spatial audio object. RIRs synthesized for a specified rendering and reproduction arrangement were convolved with dry sources to form reverberant components of the sound scene. The resulting signals demonstrated potential for these techniques, e.g., in SAO reproduction over a 22.2 surround sound system.
The perceived impression of an acoustical environment provides context, perspective and continuity to a reproduced auditory scene, giving cues to the apparent source distance, the scene width and depth, and envelopment. In many creative applications, the role of 'reverb' serves to set the scene in a way that is plausibly congruent, without disrupting the impression. The Binaural EBU ADM Renderer (BEAR; European Broadcast Union; Audio Definition Model) delivers spatial audio over headphones using object-based principles, where each sound element carries spatial metadata. The BEAR codebase was extended to encode and render an impression of room acoustics via the Reverberant Spatial Audio Object (RSAO). B-format room impulse responses (RIRs) across a diverse set of typical rooms were encoded into RSAO parameters for rendering with sources and comparison with corresponding binaural measurements (BRIRs). A web-based listening test was designed for participants to rate the perceptual similarity using a multiple stimulus rating interface. The binaural stimuli compared the RSAO rendering of the target room, the BRIRs as hidden reference, and renderings for the rooms deemed most and least similar to the target room in a pilot test. The results identify significant trends and statistical tests determine whether the RSAO-encoded rooms were perceived as plausible renditions in terms of similarity. Future work would explore the performance of this approach in interactive audiovisual applications.
The aperiodic noise source in fricatives is characteristically amplitude modulated by voicing. Previous psychoacoustic studies have established that observed levels of AM in voiced fricatives are detectable, and its inclusion in synthesis has improved speech quality. Phonological voicing in fricatives can be cued by a number of factors: the voicing fundamental, duration of any devoicing, duration of frication, and formant transitions. However, the possible contribution of AM has not been investigated. In a cue trading experiment, subjects distinguished between the nonsense words ’ahser’ and ’ahzer’. The voicing boundary was measured along a formant-transition duration continuum, as a function of AM depth, voicing amplitude and masking of the voicing component by low-frequency noise. The presence of AM increased voiced responses by approximately 30%. The ability of AM to cue voicing was strongest at greater modulation depths and when voicing was unavailable as a cue, as might occur in telecommunication systems or noisy environments. Further work would examine other fricatives and phonetic contexts, as well as interaction with other cues.
Reverberation is a common problem for many speech technologies, such as automatic speech recognition (ASR) systems. This paper investigates the novel combination of precedence, binaural and statistical independence cues for enhancing reverberant speech, prior to ASR, under these adverse acoustical conditions when two microphone signals are available. Results of the enhancement are evaluated in terms of relevant signal measures and accuracy for both English and Polish ASR tasks. These show inconsistencies between the signal and recognition measures, although in recognition the proposed method consistently outperforms all other combinations and the spectral-subtraction baseline.
Object-based audio production requires the positional metadata to be defined for each point-source object, including the key elements in the foreground of the sound scene. In many media production use cases, both cameras and microphones are employed to make recordings, and the human voice is often a key element. In this research, we detect and locate the active speaker in the video, facilitating the automatic extraction of the positional metadata of the talker relative to the camera’s reference frame. With the integration of the visual modality, this study expands upon our previous investigation focused solely on audio-based active speaker detection and localization. Our experiments compare conventional audio-visual approaches for active speaker detection that leverage monaural audio, our previous audio-only method that leverages multichannel recordings from a microphone array, and a novel audio-visual approach integrating vision and multichannel audio. We found the role of the two modalities to complement each other. Multichannel audio, overcoming the problem of visual occlusions, provides a double-digit reduction in detection error compared to audio-visual methods with single-channel audio. The combination of multichannel audio and vision further enhances spatial accuracy, leading to a four-percentage point increase in F1 score on the Tragic Talkers dataset. Future investigations will assess the robustness of the model in noisy and highly reverberant environments, as well as tackle the problem of off-screen speakers.
Motion capture (mocap) is widely used in a large number of industrial applications. Our work offers a new way of representing the mocap facial dynamics in a high resolution 3D morphable model expression space. A data-driven approach to modelling of facial dynamics is presented. We propose a way to combine high quality static face scans with dynamic 3D mocap data which has lower spatial resolution in order to study the dynamics of facial expressions.
This paper presents an investigation of the visual variation on the bilabial plosive consonant /p/ in three coarticulation contexts. The aim is to provide detailed ensemble analysis to assist coarticulation modelling in visual speech synthesis. The underlying dynamics of labeled visual speech units, represented as lip shape, from symmetric VCV utterances, is investigated. Variation in lip dynamics is quantitively and qualitatively analyzed. This analysis shows that there are statistically significant differences in both the lip shape and trajectory during coarticulation.
In this paper, we propose a divide-and-conquer approach using two generative adversarial networks (GANs) to explore how a machine can draw colorful pictures (bird) using a small amount of training data. In our work, we simulate the procedure of an artist drawing a picture, where one begins with drawing objects’ contours and edges and then paints them different colors. We adopt two GAN models to process basic visual features including shape, texture and color. We use the first GAN model to generate object shape, and then paint the black and white image based on the knowledge learned using the second GAN model. We run our experiments on 600 color images. The experimental results show that the use of our approach can generate good quality synthetic images, comparable to real ones.
The topic of sound zone reproduction, whereby listeners sharing an acoustic space can receive personalized audio content, has been researched for a number of years. Recently, a number of sound zone systems have been realized, moving the concept towards becoming a practical reality. Current implementations of sound zone systems have relied upon conventional loudspeaker geometries such as linear and circular arrays. Line arrays may be compact, but do not necessarily give the system the opportunity to compensate for room reflections in real-world environments. Circular arrays give this opportunity, and also give greater flexibility for spatial audio reproduction, but typically require large numbers of loudspeakers in order to reproduce sound zones over an acceptable bandwidth. Therefore, one key area of research standing between the ideal capability and the performance of a physical system is that of establishing the number and location of the loudspeakers comprising the reproduction array. In this study, the topic of loudspeaker configurations was considered for two-zone reproduction, using a circular array of 60 loudspeakers as the candidate set for selection. A numerical search procedure was used to select a number of loudspeakers from the candidate set. The novel objective function driving the search comprised terms relating to the acoustic contrast between the zones, array effort, matrix condition number, and target zone planarity. The performance of the selected sets using acoustic contrast control was measured in an acoustically treated studio. Results demonstrate that the loudspeaker selection process has potential for maximising the contrast over frequency by increasing the minimum contrast over the frequency range 100--4000 Hz. The array effort and target planarity can also be optimised, depending on the formulation of the objective function. Future work should consider greater diversity of candidate locations.
Multi-point approaches for sound field control generally sample the listening zone(s) with pressure microphones, and use these measurements as an input for an optimisation cost function. A number of techniques are based on this concept, for single-zone (e.g. least-squares pressure matching (PM), brightness control, planarity panning) and multi-zone (e.g. PM, acoustic contrast control, planarity control) reproduction. Accurate performance predictions are obtained when distinct microphone positions are employed for setup versus evaluation. While, in simulation, one can afford a dense sampling of virtual microphones, it is desirable in practice to have a microphone array which can be positioned once in each zone to measure the setup transfer functions between each loudspeaker and that zone. In this contribution, we present simulation results over a fixed dense set of evaluation points comparing the performance of several multi-point optimisation approaches for 2D reproduction with a 60 channel circular loudspeaker arrangement. Various regular setup microphone arrays are used to calculate the sound zone filters: circular grid, circular, dual-circular, and spherical arrays, each with different numbers of microphones. Furthermore, the effect of a rigid spherical baffle is studied for the circular and spherical arrangements. The results of this comparative study show how the directivity and effective frequency range of multi-point optimisation techniques depend on the microphone array used to sample the zones. In general, microphone arrays with dense spacing around the boundary give better angular discrimination, leading to more accurate directional sound reproduction, while those distributed around the whole zone enable more accurate prediction of the reproduced target sound pressure level.
Frequency-invariant beamformers are useful for spatial audio capture since their attenuation of sources outside the look direction is consistent across frequency. In particular, the least-squares beamformer (LSB) approximates arbitrary frequency-invariant beampatterns with generic microphone configurations. This paper investigates the effects of array geometry, directivity order and regularization for robust hypercardioid synthesis up to 15th order with the LSB, using three 2D 32-microphone array designs (rectangular grid, open circular, and circular with cylindrical baffle). While the directivity increases with order, the frequency range is inversely proportional to the order and is widest for the cylindrical array. Regularization results in broadening of the mainlobe and reduced on-axis response at low frequencies. The PEASS toolkit was used to evaluate perceptually beamformed speech signals.
Recent progresses in Virtual Reality (VR) and Augmented Reality (AR) allow us to experience various VR/AR applications in our daily life. In order to maximise the immersiveness of user in VR/AR environments, a plausible spatial audio reproduction synchronised with visual information is essential. In this paper, we propose a simple and efficient system to estimate room acoustic for plausible reproducton of spatial audio using 360 degrees cameras for VR/AR applications. A pair of 360 degrees images is used for room geometry and acoustic property estimation. A simplified 3D geometric model of the scene is estimated by depth estimation from captured images and semantic labelling using a convolutional neural network (CNN). The real environment acoustics are characterised by frequency -dependent acoustic predictions of the scene. Spatially synchronised audio is reproduced based on the estimated geometric and acoustic properties in the scene. The reconstructed scenes are rendered with synthesised spatial audio as VR/AR content. The results of estimated room geometry and simulated spatial audio are evaluated against the actual measurements and audio calculated from ground -truth Room Impulse Responses (RIRs) recorded in the rooms. Details about the data underlying this work, along with the terms for data access, are available from: http://dx.doi.org/10.15126/surreydata.00812228
Most current perceptual models for audio quality have so far tended to concentrate on the audibility of distortions and noises that mainly affect the timbre of reproduced sound. The QESTRAL model, however, is specifically designed to take account of distortions in the spatial domain such as changes in source location, width and envelopment. It is not aimed only at codec quality evaluation but at a wider range of spatial distortions that can arise in audio processing and reproduction systems. The model has been calibrated against a large database of listening tests designed to evaluate typical audio processes, comparing spatially degraded multichannel audio material against a reference. Using a range of relevant metrics and a sophisticated multivariate regression model, results are obtained that closely match those obtained in listening tests.
In this paper, a novel probabilistic Bayesian tracking scheme is proposed and applied to bimodal measurements consisting of tracking results from the depth sensor and audio recordings collected using binaural microphones. We use random finite sets to cope with varying number of tracking targets. A measurement-driven birth process is integrated to quickly localize any emerging person. A new bimodal fusion method that prioritizes the most confident modality is employed. The approach was tested on real room recordings and experimental results show that the proposed combination of audio and depth outperforms individual modalities, particularly when there are multiple people talking simultaneously and when occlusions are frequent.
Humans are able to identify a large number of environmental sounds and categorise them according to high-level semantic categories, e.g. urban sounds or music. They are also capable of generalising from past experience to new sounds when applying these categories. In this paper we report on the creation of a data set that is structured according to the top-level of a taxonomy derived from human judgements and the design of an associated machine learning challenge, in which strong generalisation abilities are required to be successful. We introduce a baseline classification system, a deep convolutional network, which showed strong performance with an average accuracy on the evaluation data of 80.8%. The result is discussed in the light of two alternative explanations: An unlikely accidental category bias in the sound recordings or a more plausible true acoustic grounding of the high-level categories.
Typical methods for binaural source separation consider only the direct sound as the target signal in a mixture. However, in most scenarios, this assumption limits the source separation performance. It is well known that the early reflections interact with the direct sound, producing acoustic effects at the listening position, e.g. the so-called comb filter effect. In this article, we propose a novel source separation model, that utilizes both the direct sound and the first early reflection information to model the comb filter effect. This is done by observing the interaural phase difference obtained from the timefrequency representation of binaural mixtures. Furthermore, a method is proposed to model the interaural coherence of the signals. Including information related to the sound multipath propagation, the performance of the proposed separation method is improved with respect to the baselines that did not use such information, as illustrated by using binaural recordings made in four rooms, having different sizes and reverberation times.
We present a framework for speech-driven synthesis of real faces from a corpus of 3D video of a person speaking. Video-rate capture of dynamic 3D face shape and colour appearance provides the basis for a visual speech synthesis model. A displacement map representation combines face shape and colour into a 3D video. This representation is used to efficiently register and integrate shape and colour information captured from multiple views. To allow visual speech synthesis viseme primitives are identified from the corpus using automatic speech recognition. A novel nonrigid alignment algorithm is introduced to estimate dense correspondence between 3D face shape and appearance for different visemes. The registered displacement map representation together with a novel optical flow optimisation using both shape and colour, enables accurate and efficient nonrigid alignment. Face synthesis from speech is performed by concatenation of the corresponding viseme sequence using the nonrigid correspondence to reproduce both 3D face shape and colour appearance. Concatenative synthesis reproduces both viseme timing and co-articulation. Face capture and synthesis has been performed for a database of 51 people. Results demonstrate synthesis of 3D visual speech animation with a quality comparable to the captured video of a person.
Recent studies show that visual information contained in visual speech can be helpful for the performance enhancement of audio-only blind source separation (BSS) algorithms. Such information is exploited through the statistical characterisation of the coherence between the audio and visual speech using, e.g. a Gaussian mixture model (GMM). In this paper, we present two new contributions. An adapted expectation maximization (AEM) algorithm is proposed in the training process to model the audio-visual coherence upon the extracted features. The coherence is exploited to solve the permutation problem in the frequency domain using a new sorting scheme. We test our algorithm on the XM2VTS multimodal database. The experimental results show that our proposed algorithm outperforms traditional audio-only BSS.
In this paper we propose a cuboid-based air-tight indoor room geometry estimation method using combination of audio-visual sensors. Existing vision-based 3D reconstruction methods are not applicable for scenes with transparent or reflective objects such as windows and mirrors. In this work we fuse multi-modal sensory information to overcome the limitations of purely visual reconstruction for reconstruction of complex scenes including transparent and mirror surfaces. A full scene is captured by 360 cameras and acoustic room impulse responses (RIRs) recorded by a loudspeaker and compact microphone array. Depth information of the scene is recovered by stereo matching from the captured images and estimation of major acoustic reflector locations from the sound. The coordinate systems for audiovisual sensors are aligned into a unified reference frame and plane elements are reconstructed from audio-visual data. Finally cuboid proxies are fitted to the planes to generate a complete room model. Experimental results show that the proposed system generates complete representations of the room structures regardless of transparent windows, featureless walls and shiny surfaces.
Reproduction of personal sound zones can be attempted by sound field synthesis, energy control, or a combination of both. Energy control methods can create an unpredictable pressure distribution in the listening zone. Sound field synthesis methods may be used to overcome this problem, but tend to produce a lower acoustic contrast between the zones. Here, we present a cost function to optimize the cancellation and the plane wave energy over a range of incoming azimuths, producing a planar sound field without explicitly specifying the propagation direction. Simulation results demonstrate the performance of the methods in comparison with the current state of the art. The method produces consistent high contrast and a consistently planar target sound zone across the frequency range 80-7000Hz. Copyright © (2013) by the Audio Engineering Society.
A simple multiple-level HMM is presented in which speech dynamics are modelled as linear trajectories in an intermediate, formant-based representation and the mapping between the intermediate and acoustic data is achieved using one or more linear transformations. An upper-bound on the performance of such a system is established. Experimental results on the TIMIT corpus demonstrate that, if the dimension of the intermediate space is suficiently high or the number of articulatory-to-acoustic mappings is sufjciently large, then this upper-bound can be achieved.
Sound eld control methods can be used to create multiple zones of audio in the same room. Separation achieved by such systems has classically been evaluated using physical metrics including acoustic contrast and target-to-interferer ratio (TIR). However, to optimise the experience for a listener it is desirable to consider perceptual factors. A search procedure was used to select 5 loudspeakers for production of 2 sound zones using acoustic contrast control. Comparisons were made between searches driven by physical (programme-independent TIR) and perceptual (distraction predictions from a statistical model) cost func- Tions. Performance was evaluated on TIR and predicted distraction in addition to subjective ratings. The perceptual cost function showed some benefits over physical optimisation, although the model used needs further work. Copyright © (2013) by the Audio Engineering Society.
Underdetermined reverberant speech separation is a challenging problem in source separation that has received considerable attention in both computational auditory scene analysis (CASA) and blind source separation (BSS). Recent studies suggest that, in general, the performance of frequency domain BSS methods suffer from the permutation problem across frequencies which degrades in high reverberation, meanwhile, CASA methods perform less effectively for closely spaced sources. This paper presents a method to address these limitations, based on the combination of binaural and BSS cues for the automatic classification of time-frequency (T-F) units of the speech mixture spectrogram. By modeling the interaural phase difference, the interaural level difference and frequency-bin mixing vectors, we integrate the coherent information for each source within a probabilistic framework. The Expectation Maximization (EM) algorithm is then used iteratively to refine the soft assignment of T-F regions to sources and re-estimate their model parameters. The coherence between the left and right recordings is also calculated to model the precedence effect which is then incorporated to the algorithm to reduce the effect of reverberation. Binaural room impulse responses for 5 different rooms with various acoustic properties have been used to generate the source images and the mixtures. The proposed method compares favorably with state-of-the-art baseline algorithms by Mandel et al. and Sawada et al., in terms of signal-to-distortion ratio (SDR) of the separated source signals.
Reverberant speech source separation has been of great interest for over a decade, leading to two major approaches. One of them is based on statistical properties of the signals and mixing process known as blind source separation (BSS). The other approach named as computational auditory scene analysis (CASA) is inspired by human auditory system and exploits monaural and binaural cues. In this paper these two approaches are studied and compared in more depth.
Since the mid 1990s, acoustics research has been undertaken relating to the sound zone problem—using loudspeakers to deliver a region of high sound pressure while simultaneously creating an area where the sound is suppressed—in order to facilitate independent listening within the same acoustic enclosure. The published solutions to the sound zone problem are derived from areas such as wave field synthesis and beamforming. However, the properties of such methods differ and performance tends to be compared against similar approaches. In this study, the suitability of energy focusing, energy cancelation, and synthesis approaches for sound zone reproduction is investigated. Anechoic simulations based on two zones surrounded by a circular array show each of the methods to have a characteristic performance, quantified in terms of acoustic contrast, array control effort and target sound field planarity. Regularization is shown to have a significant effect on the array effort and achieved acoustic contrast, particularly when mismatched conditions are considered between calculation of the source weights and their application to the system.
In our information-overloaded daily lives, unwanted sounds create confusion, disruption and fatigue in what do and experience. Taking control of your own sound environment, you can design what information to hear and how. Providing personalised sound to different people over loudspeakers enables communication, human connection and social activity in a shared space, meanwhile addressing the individuals’ needs. Recent developments in object-based audio, robust sound zoning algorithms, computer vision, device synchronisation and electronic hardware facilitate personal control of immersive and interactive reproduction techniques. Accordingly, the creative sector is moving towards more demand for personalisation and personalisable content. This tutorial offers participants a novel and timely introduction to the increasingly valuable capability to personalise sound over loudspeakers, alongside resources for the audio signal processing community. Presenting the science behind personalising sound technologies and providing insights for making sound zones in practice, we hope to create better listening experiences. The tutorial attempts a holistic exposition of techniques for producing personal sound over loudspeakers. It incorporates a practical step-by-step guide to digital filter design for real-world multizone sound reproduction and relates various approaches to one another thereby enabling comparison of the listener benefits.
Frequency-invariant beamformers are useful for spatial audio capture since their attenuation of sources outside the look direction is consistent across frequency. In particular, the least-squares beamformer (LSB) approximates arbitrary frequency-invariant beampatterns with generic microphone configurations. This paper investigates the effects of array geometry, directivity order and regularization for robust hypercardioid synthesis up to 15th order with the LSB, using three 2D 32-microphone array designs (rectangular grid, open circular, and circular with cylindrical baffle). While the directivity increases with order, the frequency range is inversely proportional to the order and is widest for the cylindrical array. Regularization results in broadening of the mainlobe and reduced on-axis response at low frequencies. The PEASS toolkit was used to evaluate perceptually beamformed speech signals.
In order to maximise the immersion in VR environments, a plausible spatial audio reproduction synchronised with visual information is essential. In this work, we propose a pipeline to create plausible interactive audio from a pair of 360 degree cameras. Details about the data underlying this work, along with the terms for data access, are available from: http://dx.doi.org/10.15126/surreydata.00812228.
Recent progresses in Virtual Reality (VR) and Augmented Reality (AR) allow us to experience various VR/AR applications in our daily life. In order to maximise the immersiveness of user in VR/AR environments, a plausible spatial audio reproduction synchronised with visual information is essential. In this paper, we propose a simple and efficient system to estimate room acoustic for plausible reproducton of spatial audio using 360° cameras for VR/AR applications. A pair of 360° images is used for room geometry and acoustic property estimation. A simplified 3D geometric model of the scene is estimated by depth estimation from captured images and semantic labelling using a convolutional neural network (CNN). The real environment acoustics are characterised by frequency-dependent acoustic predictions of the scene. Spatially synchronised audio is reproduced based on the estimated geometric and acoustic properties in the scene. The reconstructed scenes are rendered with synthesised spatial audio as VR/AR content. The results of estimated room geometry and simulated spatial audio are evaluated against the actual measurements and audio calculated from ground-truth Room Impulse Responses (RIRs) recorded in the rooms.
We present a novel method for extracting target speech from auditory mixtures using bimodal coherence, which is statistically characterised by a Gaussian mixture modal (GMM) in the offline training process, using the robust features obtained from the audio-visual speech. We then adjust the ICA-separated spectral components using the bimodal coherence in the time-frequency domain, to mitigate the scale ambiguities in different frequency bins. We tested our algorithm on the XM2VTS database, and the results show the performance improvement with our proposed algorithm in terms of SIR measurements.
‘New’ media and algorithmic rules underlying emerging technologies present particular challenges in fieldwork. The opacity of their design, and, sometimes, their real or perceived status as ‘not quite here yet’ – makes speaking about these challenging in the field. In this paper, we suggest that there is promise and potential in using vignettes and scenarios from fictionalised accounts of the uses of emerging and new technologies, drawing upon data from a three-wave citizens’ council on data-driven media personalisation. We situate our paper within the methodological approaches seen in scholarship in user centric algorithm studies (Siles, 2023; Swart, 2021; Hargottai et al, 2021) and design futuring within HCI (Dunne and Raby 2013; Lindley and Coulton 2015). We outline the empirical case study of embedding vignettes within our citizens councils. We argue, first, that vignettes and scenarios help make ‘new’ technologies and often abstract algorithms more concrete, thereby drawing out lived experiences of the social dynamics of new media. Second, we suggest that vignettes and scenarios, by centring unknown others in the narrative, help draw out users’ normative reflections on what good looks like in contemporary datafied societies.
Object-based audio is gaining momentum as a means for future audio productions to be format-agnostic and interactive. Recent standardization developments make recommendations for object formats, however the capture, production and reproduction of reverberation is an open issue. In this paper, we review approaches for recording, transmitting and rendering reverberation over a 3D spatial audio system. Techniques include channel-based approaches where room signals intended for a specific reproduction layout are transmitted, and synthetic reverberators where the room effect is constructed at the renderer. We consider how each approach translates into an object-based context considering the end-to-end production chain of capture, representation, editing, and rendering. We discuss some application examples to highlight the implications of the various approaches.
Frequency-invariant beamformers are useful for spatial audio capture since their attenuation of sources outside the look direction is consistent across frequency. In particular, the least-squares beamformer (LSB) approximates arbitrary frequency-invariant beampatterns with generic microphone configurations. This paper investigates the effects of array geometry, directivity order and regularization for robust hypercardioid synthesis up to 15th order with the LSB, using three 2D 32-microphone array designs (rectangular grid, open circular, and circular with cylindrical baffle). While the directivity increases with order, the frequency range is inversely proportional to the order and is widest for the cylindrical array. Regularization results in broadening of the mainlobe and reduced on-axis response at low frequencies. The PEASS toolkit was used to evaluate perceptually beamformed speech signals.
Acoustic event detection for content analysis in most cases relies on lots of labeled data. However, manually annotating data is a time-consuming task, which thus makes few annotated resources available so far. Unlike audio event detection, automatic audio tagging, a multi-label acoustic event classification task, only relies on weakly labeled data. This is highly desirable to some practical applications using audio analysis. In this paper we propose to use a fully deep neural network (DNN) framework to handle the multi-label classification task in a regression way. Considering that only chunk-level rather than frame-level labels are available, the whole or almost whole frames of the chunk were fed into the DNN to perform a multi-label regression for the expected tags. The fully DNN, which is regarded as an encoding function, can well map the audio features sequence to a multi-tag vector. A deep pyramid structure was also designed to extract more robust high-level features related to the target tags. Further improved methods were adopted, such as the Dropout and background noise aware training, to enhance its generalization capability for new audio recordings in mismatched environments. Compared with the conventional Gaussian Mixture Model (GMM) and support vector machine (SVM) methods, the proposed fully DNN-based method could well utilize the long-term temporal information with the whole chunk as the input. The results show that our approach obtained a 15% relative improvement compared with the official GMM-based method of DCASE 2016 challenge.
The process of understanding acoustic properties of environments is important for several applications, such as spatial audio, augmented reality and source separation. In this paper, multichannel room impulse responses are recorded and transformed into their direction of arrival (DOA)-time domain, by employing a superdirective beamformer. This domain can be represented as a 2D image. Hence, a novel image processing method is proposed to analyze the DOA-time domain, and estimate the reflection times of arrival and DOAs. The main acoustically reflective objects are then localized. Recent studies in acoustic reflector localization usually assume the room to be free from furniture. Here, by analyzing the scattered reflections, an algorithm is also proposed to binary classify reflectors into room boundaries and interior furniture. Experiments were conducted in four rooms. The classification algorithm showed high quality performance, also improving the localization accuracy, for non-static listener scenarios.
Deep neural networks (DNN) have recently been shown to give state-of-the-art performance in monaural speech enhancement. However in the DNN training process, the perceptual difference between different components of the DNN output is not fully exploited, where equal importance is often assumed. To address this limitation, we have proposed a new perceptually-weighted objective function within a feedforward DNN framework, aiming to minimize the perceptual difference between the enhanced speech and the target speech. A perceptual weight is integrated into the proposed objective function, and has been tested on two types of output features: spectra and ideal ratio masks. Objective evaluations for both speech quality and speech intelligibility have been performed. Integration of our perceptual weight shows consistent improvement on several noise levels and a variety of different noise types.
Recent work on a reverberant spatial audio object (RSAO) encoded spatial room impulse responses (RIRs) as object-based metadata which can be synthesized in an object-based renderer. Encoding reverberation into metadata presents new opportunities for end users to interact with and personalize reverberant content. The RSAO models an RIR as a set of early re ections together with a late reverberation filter. Previous work to encode the RSAO parameters was based on recordings made with a dense array of omnidirectional microphones. This paper describes RSAO parameterization from first-order Ambisonic (B-Format) RIRs, making the RSAO compatible with existing spatial reverb libraries. The object-based implementation achieves reverberation time, early decay time, clarity and interaural cross-correlation similar to direct Ambisonic rendering of 13 test RIRs.
Most of the binaural source separation algorithms only consider the dissimilarities between the recorded mixtures such as interaural phase and level differences (IPD, ILD) to classify and assign the time-frequency (T-F) regions of the mixture spectrograms to each source. However, in this paper we show that the coherence between the left and right recordings can provide extra information to label the T-F units from the sources. This also reduces the effect of reverberation which contains random reflections from different directions showing low correlation between the sensors. Our algorithm assigns the T-F regions into original sources based on weighted combination of IPD, ILD, the observation vectors models and the estimated interaural coherence (IC) between the left and right recordings. The binaural room impulse responses measured in four rooms with various acoustic conditions have been used to evaluate the performance of the proposed method which shows an improvement of more than 1:4 dB in signal-to-distortion ratio (SDR) in room D with T60 = 0:89 s over the state-of-the-art algorithms.
Recent studies show that facial information contained in visual speech can be helpful for the performance enhancement of audio-only blind source separation (BSS) algorithms. Such information is exploited through the statistical characterization of the coherence between the audio and visual speech using, e.g., a Gaussian mixture model (GMM). In this paper, we present three contributions. With the synchronized features, we propose an adapted expectation maximization (AEM) algorithm to model the audiovisual coherence in the off-line training process. To improve the accuracy of this coherence model, we use a frame selection scheme to discard nonstationary features. Then with the coherence maximization technique, we develop a new sorting method to solve the permutation problem in the frequency domain. We test our algorithm on a multimodal speech database composed of different combinations of vowels and consonants. The experimental results show that our proposed algorithm outperforms traditional audio-only BSS, which confirms the benefit of using visual speech to assist in separation of the audio. © 2011 Elsevier B.V. All rights reserved.
We present an analysis of linear feature extraction techniques to derive a compact and meaningful representation of the articulatory data. We used 14-channel EMA (ElectroMagnetic Articulograph) data from two speakers from the MOCHA database [A.A. Wrench. A new resource for production modelling in speech technology. In Proc. Inst. of Acoust., Stratford-upon-Avon, UK, 2001.]. As representations, we considered the registered articulator fleshpoint coordinates, transformed PCA (Principal Component Analysis) and LDA (Linear Discriminant Analysis) features. Various PCA schemes were considered, grouping coordinates according to correlations amongst the articulators. For each phone, critical dimensions were identified using the algorithm in [Veena D Singampalli and Philip JB Jackson. Statistical identification of critical, dependent and redundant articulators. In Proc. Interspeech, Antwerp, Belgium, pages 70-73, 2007.]: critical articulators with registered coordinates, and critical modes with PCA and LDA. The phone distributions in each representation were modelled as univariate Gaussians and the average number of critical dimensions was controlled using a threshold on the 1-D Kullback Leibler divergence (identification divergence). The 14-D KL divergence (evaluation divergence) was employed to measure goodness of fit of the models to estimated phone distributions. Phone recognition experiments were performed using coordinate, PCA and LDA features, for comparison. We found that, of all representations, the LDA space yielded the best fit between the model and phone pdfs. The full PCA representation (including all articulatory coordinates) gave the next best fit, closely followed by two other PCA representations that allowed for correlations across the tongue. At the threshold where average number of critical dimensions matched those obtained from IPA, the goodness of fit improved by 34% (22%/46% for male/female data) when LDA was used over the best PCA representation, and by 72% (77%/66%) over articulatory coordinates. For PCA and LDA, the compactness of the representation was investigated by discarding the least significant modes. No significant change in the recognition performance was found as the dimensionality was reduced from 14 to 8 (95% confidence t-test), although accuracy deteriorated as further modes were discarded. Evaluation divergence also reflected this pattern. Experiments on LDA features increased recognition accuracy by 2% on average over the best PC representation. An articulatory interpretation of the PCA and LDA modes is discussed. Future work focuses on articulatory trajectory generation in feature spaces guided by the findings of this study.
We present a novel pipeline to estimate reverberant spatial audio object (RSAO) parameters given room impulse responses (RIRs) recorded by ad-hoc microphone arrangements. The proposed pipeline performs three tasks: direct-to-reverberant-ratio (DRR) estimation; microphone localization; RSAO parametrization. RIRs recorded at Bridgewater Hall by microphones arranged for a BBC Philharmonic Orchestra performance were parametrized. Objective measures of the rendered RSAO reverberation characteristics were evaluated and compared with reverberation recorded by a Soundfield microphone. Alongside informal listening tests, the results confirmed that the rendered RSAO gave a plausible reproduction of the hall, comparable to the measured response. The objectification of the reverb from in-situ RIR measurements unlocks customization and personalization of the experience for different audio systems, user preferences and playback environments.
Techniques such as multi-point optimization, wave field synthesis and ambisonics attempt to create spatial effects by synthesizing a sound field over a listening region. In this paper, we propose planarity panning, which uses superdirective microphone array beamforming to focus the sound from the specified direction, as an alternative approach. Simulations compare performance against existing strategies, considering the cases where the listener is central and non-central in relation to a 60 channel circular loudspeaker array. Planarity panning requires low control effort and provides high sound field planarity over a large frequency range, when the zone positions match the target regions specified for the filter calculations. Future work should implement and validate the perceptual properties of the method.
In this paper we examine how the term ‘Audio Augmented Reality’ (AAR) is used in the literature, and how the concept is used in practice. In particular, AAR seems to refer to a variety of closely related concepts. In order to gain a deeper understanding of disparate work surrounding AAR, we present a taxonomy of these concepts and highlight both canonical examples in each category, as well as edge cases that help define the category boundaries.
This work aims to improve the quality of visual speech synthesis by modelling its emotional characteristics. The emotion specific speech content is analysed based on the 3D video dataset of expressive speech. Preliminary results indicate a promising relation between the chosen features of visual speech and emotional content.
Recent advances in human-computer interaction technology go beyond the successful transfer of data between human and machine by seeking to improve the naturalness and friendliness of user interactions. An important augmentation, and potential source of feedback, comes from recognizing the user's expressed emotion or affect. This chapter presents an overview of research efforts to classify emotion using different modalities: audio, visual and audio-visual combined. Theories of emotion provide a framework for defining emotional categories or classes. The first step, then, in the study of human affect recognition involves the construction of suitable databases. The authors describe fifteen audio, visual and audio-visual data sets, and the types of feature that researchers have used to represent the emotional content. They discuss data-driven methods of feature selection and reduction, which discard noise and irrelevant information to maximize the concentration of useful information. They focus on the popular types of classifier that are used to decide to which emotion class a given example belongs, and methods of fusing information from multiple modalities. Finally, the authors point to some interesting areas for future investigation in this field, and conclude. © 2011, IGI Global.
A compact, data-driven statistical model for identifying roles played by articulators in production of English phones using 1D and 2D articulatory data is presented. Articulators critical in production of each phone were identified and were used to predict the pdfs of dependent articulators based on the strength of articulatory correlations. The performance of the model is evaluated on MOCHA database using proposed and exhaustive search techniques and the results of synthesised trajectories presented.
Single-channel signal separation and deconvolution aims to separate and deconvolve individual sources from a single-channel mixture and is a challenging problem in which no prior knowledge of the mixing filters is available. Both individual sources and mixing filters need to be estimated. In addition, a mixture may contain non-stationary noise which is unseen in the training set. We propose a synthesizing-decomposition (S-D) approach to solve the single-channel separation and deconvolution problem. In synthesizing, a generative model for sources is built using a generative adversarial network (GAN). In decomposition, both mixing filters and sources are optimized to minimize the reconstruction error of the mixture. The proposed S-D approach achieves a peak-to-noise-ratio (PSNR) of 18.9 dB and 15.4 dB in image inpainting and completion, outperforming a baseline convolutional neural network PSNR of 15.3 dB and 12.2 dB, respectively and achieves a PSNR of 13.2 dB in source separation together with deconvolution, outperforming a convolutive non-negative matrix factorization (NMF) baseline of 10.1 dB.
Binaural recording technology offers an inexpensive, portable solution for spatial audio capture. In this paper, a full-sphere 2D localization method is proposed which utilizes the Model-Based Expectation-Maximization Source Separation and Localization system (MESSL). The localization model is trained using a full-sphere head related transfer function dataset and produces localization estimates by maximum-likelihood of frequency-dependent interaural parameters. The model’s robustness is assessed using matched and mismatched HRTF datasets between test and training data, with environmental sounds and speech. Results show that the majority of sounds are estimated correctly with the matched condition in low noise levels; for the mismatched condition, a ‘cone of confusion’ arises with albeit effective estimation of lateral angles. Additionally, the results show a relationship between the spectral content of the test data and the performance of the proposed method.
Sound Event Localization and Detection (SELD) is a task that involves detecting different types of sound events along with their temporal and spatial information, specifically, detecting the classes of events and estimating their corresponding direction of arrivals at each frame. In practice, real-world sound scenes might be complex as they may contain multiple overlapping events. For instance, in DCASE challenges task 3, each clip may involve simultaneous occurrences of up to five events. To handle multiple overlapping sound events, current methods prefer multiple output branches to estimate each event, which increases the size of the models. Therefore, current methods are often difficult to be deployed on the edge of sensor networks. In this paper, we propose a method called Probabilistic Localization and Detection of Independent Sound Events with Transformers (PLDISET), which estimates numerous events by using one output branch. The method has three stages. First, we introduce the track generation module to obtain various tracks from extracted features. Then, these tracks are fed into two transformers for sound event detection (SED) and localization, respectively. Finally, one output system, including a linear Gaussian system and regression network, is used to estimate each track. We give the evaluation resn results of our model on DCASE 2023 Task 3 development dataset.
The two distinct sound sources comprising voiced frication, voicing and frication, interact. One effect is that the periodic source at the glottis modulates the amplitude of the frication source originating in the vocal tract above the constriction. Voicing strength and modulation depth for frication noise were measured for sustained English voiced fricatives using high-pass filtering, spectral analysis in the modulation (envelope) domain, and a variable pitch compensation procedure. Results show a positive relationship between strength of the glottal source and modulation depth at voicing strengths below 66 dB SPL, at which point the modulation index was approximately 0.5 and saturation occurred. The alveolar [z] was found to be more modulated than other fricatives.
In existing audio-visual blind source separation (AV-BSS) algorithms, the AV coherence is usually established through statistical modelling, using e.g. Gaussian mixture models (GMMs). These methods often operate in a lowdimensional feature space, rendering an effective global representation of the data. The local information, which is important in capturing the temporal structure of the data, however, has not been explicitly exploited. In this paper, we propose a new method for capturing such local information, based on audio-visual dictionary learning (AVDL). We address several challenges associated with AVDL, including cross-modality differences in size, dimension and sampling rate, as well as the issues of scalability and computational complexity. Following a commonly employed bootstrap coding-learning process, we have developed a new AVDL algorithm which features, a bimodality balanced and scalable matching criterion, a size and dimension adaptive dictionary, a fast search index for efficient coding, and cross-modality diverse sparsity. We also show how the proposed AVDL can be incorporated into a BSS algorithm. As an example, we consider binaural mixtures, mimicking aspects of human binaural hearing, and derive a new noise-robust AV-BSS algorithm by combining the proposed AVDL algorithm with Mandel’s BSS method, which is a state-of-the-art audio-domain method using time-frequency masking. We have systematically evaluated the proposed AVDL and AV-BSS algorithms, and show their advantages over the corresponding baseline methods, using both synthetic data and visual speech data from the multimodal LILiR Twotalk corpus.
We propose a novel method for full-sphere binaural sound source localization that is designed to be robust to real world recording conditions. A mask is proposed that is designed to remove diffuse noise and early room reflections. The method makes use of the interaural phase difference (IPD) for lateral angle localization and spectral cues for polar angle localization. The method is tested using different HRTF datasets to generate the test data and training data. The method is also tested with the presence of additive noise and reverberation. The method outperforms the state of the art binaural localization methods for most testing conditions.
Natural-sounding reproduction of sound over headphones requires accurate estimation of an individual's Head-Related Impulse Responses (HRIRs), capturing details relating to the size and shape of the body, head and ears. A stereo-vision face capture system was used to obtain 3D geometry, which provided surface data for boundary element method (BEM) acoustical simulation. Audio recordings were filtered by the output HRIRs to generate samples for a comparative listening test alongside samples generated with dummy-head HRIRs. Preliminary assessment showed better localization judgements with the personalized HRIRs by the corresponding participant, whereas other listeners performed better with dummy-head HRIRs, which is consistent with expectations for personalized HRIRs. The use of visual measurements for enhancing users' auditory experience merits investigation with additional participants.
State-of-the-art binaural objective intelligibility measures (OIMs) require individual source signals for making intelligibility predictions, limiting their usability in real-time online operations. This limitation may be addressed by a blind source separation (BSS) process, which is able to extract the underlying sources from a mixture. In this study, a speech source is presented with either a stationary noise masker or a fluctuating noise masker whose azimuth varies in a horizontal plane, at two speech-to-noise ratios (SNRs). Three binaural OIMs are used to predict speech intelligibility from the signals separated by a BSS algorithm. The model predictions are compared with listeners' word identification rate in a perceptual listening experiment. The results suggest that with SNR compensation to the BSS-separated speech signal, the OIMs can maintain their predictive power for individual maskers compared to their performance measured from the direct signals. It also reveals that the errors in SNR between the estimated signals are not the only factors that decrease the predictive accuracy of the OIMs with the separated signals. Artefacts or distortions on the estimated signals caused by the BSS algorithm may also be concerns.
Information from video has been used recently to address the issue of scaling ambiguity in convolutive blind source separation (BSS) in the frequency domain, based on statistical modeling of the audio-visual coherence with Gaussian mixture models (GMMs) in the feature space. However, outliers in the feature space may greatly degrade the system performance in both training and separation stages. In this paper, a new feature selection scheme is proposed to discard non-stationary features, which improves the robustness of the coherence model and reduces its computational complexity. The scaling parameters obtained by coherence maximization and non-linear interpolation from the selected features are applied to the separated frequency components to mitigate the scaling ambiguity. A multimodal database composed of different combinations of vowels and consonants was used to test our algorithm. Experimental results show the performance improvement with our proposed algorithm.
This article presents findings from a rigorous, three-wave series of qualitative research into public expectations of data-driven media technologies, conducted in England, United Kingdom. Through a range of carefully chosen scenarios and deliberations around the risks and benefits afforded by data-driven media personalisation technologies and algorithms, we paid close attention to citizens' voices as our multidisciplinary team sought to engage the public on what 'good' might look like in the context of media personalisation. We paid particular attention to risks and opportunities, examining practical use-cases and scenarios, and our three-wave councils culminated in citizens producing recommendations for practice and policy. In this article, we focus particularly on citizens' ethical assessment, critique and improvements proposed on media personalisation methods in relation to benefits, fairness, safety, transparency and accountability. Our findings demonstrate that public expectations and trust in data-driven technologies are, fundamentally, conditional, with significant emphasis placed on transparency, inclusiveness and accessibility. Our findings also point to the context dependency of public expectations, which appears more pertinent to citizens, in hard political as opposed to entertainment spaces. Our conclusions are significant for global data-driven media personalisation environments - in terms of embedding citizens' focus on transparency and accountability, but equally, also, we argue that strengthening research methodology, innovatively and rigorously to build in citizen voices at the very inception and core of design - must become a priority in technology development.
Previously-obtained data, quantifying the degree of quality degradation resulting from a range of spatial audio processes (SAPs), can be used to build a regression model of perceived spatial audio quality in terms of previously developed spatially and timbrally relevant metrics. A generalizable model thus built, employing just five metrics and two principal components, performs well in its prediction of the quality of a range of program types degraded by a multitude of SAPs commonly encountered in consumer audio reproduction, auditioned at both central and off-center listening positions. Such a model can provide a correlation to listening test data of r = 0.89, with a root mean square error (RMSE) of 11%, making its performance comparable to that of previous audio quality models and making it a suitable core for an artificial-listener-based spatial audio quality evaluation system.
The pitch-scaled harmonic filter (PSHF) is a technique for decomposing speech signals into their voiced and unvoiced constituents. In this paper, we evaluate its ability to reconstruct the time series of the two components accurately using a variety of synthetic, speech-like signals, and discuss its performance. These results determine the degree of confidence that can be expected for real speech signals: typically, 5 dB improvement in the signal-to-noise ratio (HNR) in the anharmonic component. A selection of the analysis oportunities that the decomposition offers is demonstrated on speech recording, including dynamic HNR estimation and separate linear prediction analyses of the two components. These new capabilities provided by the PSHF can facilitate discovering previously hidden features and investigating interactions of unvoiced sources, such as friction, with voicing.
The two distinct sound sources comprising voiced frication, voicing and frication, interact. One effect is that the periodic source at the glottis modulates the amplitude of the frication source originating in the vocal tract above the constriction. Voicing strength and modulation depth for frication noise were measured for sustained English voiced fricatives using high-pass filtering, spectral analysis in the modulation (envelope) domain, and a variable pitch compensation procedure. Results show a positive relationship between strength of the glottal source and modulation depth at voicing strengths below 66 dB SPL, at which point the modulation index was approximately 0.5 and saturation occurred. The alveolar [z] was found to be more modulated than other fricatives.
The aim of the study is to learn the relationship between facial movements and the acoustics of speech sounds. We recorded A database of 3D video of the face, including markers, and corresponding synchronized audio of a single speaker. The database consists of 110 English sentences. These sentences were selected for strong expressive content in the fundamental emotions: Anger, Surprise, Sadness, Happiness, Fear and Disgust. Comparisons are made with the same sentences with neutral expression. Principal component analysis of the marker movements was performed to identify significant modes of variation. The results of this analysis show that there are various characteristic difference between visual features of emotional versus neutral speech. The findings of the current research provide a basis for generating realistic animations of emotional speech for applications such as computer games and films.
We describe a method for the synthesis of visual speech movements using a hybrid unit selection/model-based approach. Speech lip movements are captured using a 3D stereo face capture system and split up into phonetic units. A dynamic parameterisation of this data is constructed which maintains the relationship between lip shapes and velocities; within this parameterisation a model of how lips move is built and is used in the animation of visual speech movements from speech audio input. The mapping from audio parameters to lip movements is disambiguated by selecting only the most similar stored phonetic units to the target utterance during synthesis. By combining properties of model-based synthesis (e.g., HMMs, neural nets) with unit selection we improve the quality of our speech synthesis.
Underdetermined reverberant speech separation is a challenging problem in source sep- aration that has received considerable attention in both computational auditory scene analysis (CASA) and blind source separation (BSS). Recent studies suggest that, in general, the performance of frequency domain BSS methods suffer from the permuta- tion problem across frequencies which degrades in high reverberation, meanwhile, CASA methods perform less effectively for closely spaced sources. This paper presents a method to address these limitations, based on the combination of monaural, binaural and BSS cues for the automatic classification of time-frequency (T-F) units of the speech mixture spectrogram. By modeling the interaural phase difference, the interaural level difference and frequency-bin mixing vectors, we integrate the coherence information for each source within a probabilistic framework. The Expectation-Maximization (EM) algorithm is then used iteratively to refine the soft assignment of TF regions to sources and re-estimate their model parameters. It is observed that the reliability of the cues affects the accu- racy of the estimates and varies with respect to cue type and frequency. As such, the contribution of each cue to the assignment decision is adjusted by weighting the log- likelihoods of the cues empirically, which significantly improves the performance. Results are reported for binaural speech mixtures in five rooms covering a range of reverberation times and direct-to-reverberant ratios. The proposed method compares favorably with state-of-the-art baseline algorithms by Mandel et al. and Sawada et al., in terms of signal- to-distortion ratio (SDR) of the separated source signals. The paper also investigates the effect of introducing spectral cues for integration within the same framework. Analysis of the experimental outcomes will include a comparison of the contribution of individual cues under varying conditions and discussion of the implications for system optimization.
Phonetic detail of voiced and unvoiced fricatives was examined using speech analysis tools. Outputs of eight f0 trackers were combined to give reliable voicing and f0 values. Log - energy and Mel frequency cepstral features were used to train a Gaussian classifier that objectively labeled speech frames for frication. Duration statistics were derived from the voicing and frication labels for distinguishing between unvoiced and voiced fricatives in British English and European Portuguese.
Room Impulse Responses (RIRs) measured with microphone arrays capture spatial and nonspatial information, e.g. the early reflections’ directions and times of arrival, the size of the room and its absorption properties. The Reverberant Spatial Audio Object (RSAO) was proposed as a method to encode room acoustic parameters from measured array RIRs. As the RSAO is object-based audio compatible, its parameters can be rendered to arbitrary reproduction systems and edited to modify the reverberation characteristics, to improve the user experience. Various microphone array designs have been proposed for sound field and room acoustic analysis, but a comparative performance evaluation is not available. This study assesses the performance of five regular microphone array geometries (linear, rectangular, circular, dual-circular and spherical) to capture RSAO parameters for the direct sound and early reflections of RIRs. The image source method is used to synthesise RIRs at the microphone positions as well as at the centre of the array. From the array RIRs, the RSAO parameters are estimated and compared to the reference parameters at the centre of the array. A performance comparison among the five arrays is established as well as the effect of a rigid spherical baffle for the circular and spherical arrays. The effects of measurement uncertainties, such as microphone misplacement and sensor noise errors, are also studied. The results show that planar arrays achieve the most accurate horizontal localisation whereas the spherical arrays perform best in elevation. Arrays with smaller apertures achieve a higher number of detected reflections, which becomes more significant for the smaller room with higher reflection density.
The QESTRAL model is a perceptual model that aims to predict changes to spatial quality of service between a reference system and an impaired version of the reference system. To achieve this, the model required calibration using perceptual data from human listeners. This paper describes the development, implementation and outcomes of a series of listening experiments designed to investigate the spatial quality impairment of 40 processes. Assessments were made using a multi-stimulus test paradigm with a label-free scale, where only the scale polarity is indicated. The tests were performed at two listening positions, using experienced listeners. Results from these calibration experiments are presented. A preliminary study on the process of selecting of stimuli is also discussed.
In this paper, we propose an iterative deep neural network (DNN)-based binaural source separation scheme, for recovering two concurrent speech signals in a room environment. Besides the commonly-used spectral features, the DNN also takes non-linearly wrapped binaural spatial features as input, which are refined iteratively using parameters estimated from the DNN output via a feedback loop. Different DNN structures have been tested, including a classic multilayer perception regression architecture as well as a new hybrid network with both convolutional and densely-connected layers. Objective evaluations in terms of PESQ and STOI showed consistent improvement over baseline methods using traditional binaural features, especially when the hybrid DNN architecture was employed. In addition, our proposed scheme is robust to mismatches between the training and testing data.
Audio production is moving toward an object-based approach, where content is represented as audio together with metadata that describe the sound scene. From current object definitions, it would usually be expected that the audio portion of the object is free from interfering sources. This poses a potential problem for object-based capture, if microphones cannot be placed close to a source. This paper investigates the application of microphone array beamforming to separate a mixture into distinct audio objects. Real mixtures recorded by a 48-channel microphone array in reflective rooms were separated, and the results were evaluated using perceptual models in addition to physical measures based on the beam pattern. The effect of interfering objects was reduced by applying the beamforming techniques.
For a sound source on the median-plane of a binaural system, interaural localization cues are absent. So, for robust binaural localization of sound sources on the median-plane, localization methods need to be designed with this in consideration. We compare four median-plane binaural sound source localization methods. Where appropriate, adjustments to the methods have been made to improve their robustness to real world recording conditions. The methods are tested using different HRTF datasets to generate the test data and training data. Each method uses a different combination of spectral and interaural localization cues, allowing for a comparison of the effect of spectral and interaural cues on median-plane localization. The methods are tested for their robustness to different levels of additive noise and different categories of sound.
An objective prediction model for the sensation of sound envelopment in five-channel reproduction is important for evaluating spatial quality. Regression analysis was used to map the listening test scores on a variety of audio sources and the objective measures extracted from the recordings themselves. By following an iterative process, a prediction model with five features was constructed. The validity of the model was tested in a second set of subjective scores and showed a correlation coefficient of 0.9. Among the five features: sound distribution and interaural cross-correlation contributed substantially to the sensation of envelopment. The model did not require access to the original audio. Scales used for listening tests were defined by audible anchors.
This paper describes the development of an unintrusive objective model, developed independently as a part of the QESTRAL project, for predicting the sensation of envelopment arising from commercially available 5-channel surround sound recordings. The model was calibrated using subjective scores obtained from listening tests that used a grading scale defined by audible anchors. For predicting subjective scores, a number of features based on Interaural Cross Correlation (IACC), Karhunen-Loeve Transform (KLT) and signal energy levels were extracted from recordings. The ridge regression technique was used to build the objective model and a calibrated model was validated using a listening test scores database obtained from a different group of listeners, stimuli and location. The initial results showed a high correlation between predicted and actual scores obtained from the listening tests.
A decomposition algorithm that uses a pitch-scaled harmonic filter was evaluated using synthetic signals and applied to mixed-source speech, spoken by three subjects, to separate the voiced and unvoiced parts. Pulsing of the noise component was observed in voiced frication, which was analyzed by complex demodulation of the signal envelope. The timing of the pulsation, represented by the phase of the anharmonic modulation coefficient, showed a step change during a vowel-fricative transition corresponding to the change in location of the sound source within the vocal tract. Analysis of fricatives //[phonetic beta], v, [edh], z, [yog], [vee with swirl], [backward glottal stop]// demonstrated a relationship between steady-state phase and place, and f0 glides confirmed that the main cause was a place-dependent delay.
Boundary estimation from an acoustic room impulse response (RIR), exploiting known sound propagation behavior, yields useful information for various applications: e.g., source separation, simultaneous localization and mapping, and spatial audio. The baseline method, an algorithm proposed by Antonacci et al., uses reflection times of arrival (TOAs) to hypothesize reflector ellipses. Here, we modify the algorithm for 3-D environments and for enhanced noise robustness: DYPSA and MUSIC for epoch detection and direction of arrival (DOA) respectively are combined for source localization, and numerical search is adopted for reflector estimation. Both methods, and other variants, are tested on measured RIR data; the proposed method performs best, reducing the estimation error by 30%.
Environmental audio tagging aims to predict only the presence or absence of certain acoustic events in the interested acoustic scene. In this paper we make contributions to audio tagging in two parts, respectively, acoustic modeling and feature learning. We propose to use a shrinking deep neural network (DNN) framework incorporating unsupervised feature learning to handle the multi-label classification task. For the acoustic modeling, a large set of contextual frames of the chunk are fed into the DNN to perform a multi-label classification for the expected tags, considering that only chunk (or utterance) level rather than frame-level labels are available. Dropout and background noise aware training are also adopted to improve the generalization capability of the DNNs. For the unsupervised feature learning, we propose to use a symmetric or asymmetric deep de-noising auto-encoder (syDAE or asyDAE) to generate new data-driven features from the logarithmic Mel-Filter Banks (MFBs) features. The new features, which are smoothed against background noise and more compact with contextual information, can further improve the performance of the DNN baseline. Compared with the standard Gaussian Mixture Model (GMM) baseline of the DCASE 2016 audio tagging challenge, our proposed method obtains a significant equal error rate (EER) reduction from 0.21 to 0.13 on the development set. The proposed asyDAE system can get a relative 6.7% EER reduction compared with the strong DNN baseline on the development set. Finally, the results also show that our approach obtains the state-of-the-art performance with 0.15 EER on the evaluation set of the DCASE 2016 audio tagging task while EER of the first prize of this challenge is 0.17.
Object-based audio has the potential to enable multime- dia content to be tailored to individual listeners and their reproduc- tion equipment. In general, object-based production assumes that the objects|the assets comprising the scene|are free of noise and inter- ference. However, there are many applications in which signal separa- tion could be useful to an object-based audio work ow, e.g., extracting individual objects from channel-based recordings or legacy content, or recording a sound scene with a single microphone array. This paper de- scribes the application and evaluation of blind source separation (BSS) for sound recording in a hybrid channel-based and object-based workflow, in which BSS-estimated objects are mixed with the original stereo recording. A subjective experiment was conducted using simultaneously spoken speech recorded with omnidirectional microphones in a rever- berant room. Listeners mixed a BSS-extracted speech object into the scene to make the quieter talker clearer, while retaining acceptable au- dio quality, compared to the raw stereo recording. Objective evaluations show that the relative short-term objective intelligibility and speech qual- ity scores increase using BSS. Further objective evaluations are used to discuss the in uence of the BSS method on the remixing scenario; the scenario shown by human listeners to be useful in object-based audio is shown to be a worse-case scenario.
A multiplanar Dynamic Magnetic Resonance Imaging (MRI) technique that extends our earlier work on single-plane Dynamic MRI is described. Scanned images acquired while an utterance is repeated are recombined to form pseudo-time-varying images of the vocal tract using a simultaneously recorded audio signal. There is no technical limit on the utterance length or number of slices that can be so imaged, though the number of repetitions required may be limited by the subject's stamina. An example of [pasi] imaged in three sagittal planes is shown; with a Signa GE 0.5 T MR scanner, 360 tokens were reconstructed to form a sequence of 39 3-slice 16 ms frames. From these, a 3-D volume was generated for each frame, and tract surfaces outlined manually. Parameters derived from these include: palate-tongue distances for [a,s,i]; estimates of tongue volume and of the area function using only the midsagittal, and then all three slices. These demonstrate the accuracy and usefulness of the technique.
In this project we have involved four high-achieving pre-university summer placement students in the development of undergraduate teaching materials, namely tutorial videos for first year undergraduate Electrical and Electronic Engineering lab, and computer simulations of didactic semiconductor structures for an Electrical Science first year compulsory taught module. Here we describe our approach and preliminary results.
A sound source's apparent distance provides information about spatial relations that can have primary salience relative to other dimensions, including azimuth and elevation in the scene of a story told in 3D. Having methods to create a sense of presence and to control this attribute in immersive content are therefore valuable capabilities in sound design, particularly in object-based audio. This paper examines the ability of the reverberant spatial audio object (RSAO) to cue apparent source distance, using acoustic parameters extracted from publicly-available spatial room impulse responses (RIRs) of real environments measured at a range of source-receiver distances. The RSAO's spatio-temporal reverb representation derived from the directional B-format RIRs encoded the timing, direction and timbre of early reflections, as well as the onset, colouration and decay times of the late reverberation, which were rendered over a 42.1 setup in an acoustically- treated listening room to provide a quasi-transparent pipeline for the reproduced room impression, from recording to listening. An objective analysis of re-synthesised RIRs and re-estimated parameters demonstrated the pipeline's transparency for most parameters. However spectral leakage of bandpass filters in the late tail encouraged reverb time convergence across bands. Formal listening tests evaluated the apparent source distance that participants perceived via a multi-stimulus rating method. Statistical analysis indicates participants perceived reproduced distance changes, with logarithmic distance resolution inside the rooms' critical distance. Beyond this, ratings tended to saturate. These effects were clearer in the large hall than in the classroom, and for the voice source than the percussion. The results suggest that the RSAO can provide appropriate cues for source distance perception with resolution comparable to natural sound fields. Further work will investigate how distance perception performs across reproduction setups and develop methods to extrapolate source distance by adapting the RSAO parameters.
In immersive and interactive audio-visual content, there is very significant scope for spatial misalignment between the two main modalities. So, in productions that have both 3D video and spatial audio, the positioning of sound sources relative to the visual display requires careful attention. This may be achieved in the form of object-based audio, moreover allowing the producer to maintain control over individual elements within the mix. Yet each object?s metadata is needed to define its position over time. In the present study, audio-visual studio recordings were made of short scenes representing three genres: drama, sport and music. Foreground video was captured by a light-field camera array, which incorporated a microphone array, alongside more conventional sound recording by spot microphones and a first-order ambisonic room microphone. In the music scenes, a direct feed from the guitar pickup was also recorded. Video data was analysed to form a 3D reconstruction of the scenes, and human figure detection was applied to the 2D frames of the central camera. Visual estimates of the sound source positions were used to provide ground truth. Position metadata were encoded within audio definition model (ADM) format audio files, suitable for standard object-based rendering. The steered response power of the acoustical signals at the microphone array were used, with the phase transform (SRP-PHAT), to determine the dominant source position(s) at any time, and given as input to a Sequential Monte Carlo Probability Hypothesis Density (SMC-PHD) tracker. The output of this was evaluated in relation to the ground truth. Results indicate a hierarchy of accuracy in azimuth, elevation and range, in accordance with human spatial auditory perception. Azimuth errors were within the tolerance bounds reported by studies of the Ventriloquism Effect, giving an initial promising indication that such an approach may open the door to object-based production for live events.
Durations of real speech segments do not generally exhibit exponential distributions, as modelled implicitly by the state transitions of Markov processes. Several duration models were considered for integration within a segmental-HMM recognizer: uniform, exponential, Poisson, normal, gamma and discrete. The gamma distribution fitted that measured for silence best, by an order of magnitude. Evaluations determined an appropriate weighting for duration against the acoustic models. Tests showed a reduction of 2 % absolute (6+ % relative) in the phone-classification error rate with gamma and discrete models; exponential ones gave approximately 1 % absolute reduction, and uniform no significant improvement. These gains in performance recommend the wider application of explicit duration models.
Sound zone systems aim to control sound fields in such a way that multiple listeners can enjoy different audio programs within the same room with minimal acoustic interference. Often, there is a trade-off between the acoustic contrast achieved between the zones and the fidelity of the reproduced audio program in the target zone. A listening test was conducted to obtain subjective measures of distraction, target quality, and overall quality of listening experience for ecologically valid programs within a sound zoning system. Sound zones were reproduced using acoustic contrast control, planarity control, and pressure matching applied to a circular loudspeaker array. The highest mean overall quality was a compromise between distraction and target quality. The results showed that the term “distraction” produced good agreement among listeners, and that listener ratings made using this term were a good measure of the perceived effect of the interferer.
For many audio applications, availability of recorded multi-channel room impulse responses (MC-RIRs) is fundamental. They enable development and testing of acoustic systems for reflective rooms. We present multiple MC-RIR datasets recorded in diverse rooms, using up to 60 loudspeaker positions and various uniform compact microphone arrays. These datasets complement existing RIR libraries and have dense spatial sampling of a listening position. To reveal the encapsulated spatial information, several state of the art room visualization methods are presented. Results confirm the measurement fidelity and graphically depict the geometry of the recorded rooms. Further investigation of these recordings and visualization methods will facilitate object-based RIR encoding, integration of audio with other forms of spatial information, and meaningful extrapolation and manipulation of recorded compact microphone array RIRs.
The acoustic environment affects the properties of the audio signals recorded. Generally, given room impulse responses (RIRs), three sets of parameters have to be extracted in order to create an acoustic model of the environment: sources, sensors and reflector positions. In this paper, the cross-correlation based iterative sensor position estimation (CISPE) algorithm is presented, a new method to estimate a microphone configuration, together with source and reflector position estimators. A rough measurement of the microphone positions initializes the process; then a recursive algorithm is applied to improve the estimates, exploiting a delay-and-sum beamformer. Knowing where the microphones lie in the space, the dynamic programming projected phase slope algorithm (DYPSA) extracts the times of arrival (TOAs) of the direct sounds from the RIRs, and multiple signal classification (MUSIC) extracts the directions of arrival (DOAs). A triangulation technique is then applied to estimate the source positions. Finally, exploiting properties of 3D quadratic surfaces (namely, ellipsoids), reflecting planes are localized via a technique ported from image processing, by random sample consensus (RANSAC). Simulation tests were performed on measured RIR datasets acquired from three different rooms located at the University of Surrey, using either a uniform circular array (UCA) or uniform rectangular array (URA) of microphones. Results showed small improvements with CISPE pre-processing in almost every case.
Object-based audio is gaining momentum as a means for future audio content to be more immersive, interactive, and accessible. Recent standardization developments make recommendations for object formats, however, the capture, production and reproduction of reverberation is an open issue. In this paper, parametric approaches for capturing, representing, editing, and rendering reverberation over a 3D spatial audio system are reviewed. A framework is proposed for a Reverberant Spatial Audio Object (RSAO), which synthesizes reverberation inside an audio object renderer. An implementation example of an object scheme utilising the RSAO framework is provided, and supported with listening test results, showing that: the approach correctly retains the sense of room size compared to a convolved reference; editing RSAO parameters can alter the perceived room size and source distance; and, format-agnostic rendering can be exploited to alter listener envelopment.
Natural-sounding reproduction of sound over headphones requires accurate estimation of an individual’s Head-Related Impulse Responses (HRIRs), capturing details relating to the size and shape of the body, head and ears. A stereo-vision face capture system was used to obtain 3D geometry, which provided surface data for boundary element method (BEM) acoustical simulation. Audio recordings were filtered by the output HRIRs to generate samples for a comparative listening test alongside samples generated with dummy-head HRIRs. Preliminary assessment showed better localization judgements with the personalized HRIRs by the corresponding participant, whereas other listeners performed better with dummy-head HRIRs, which is consistent with expectations for personalized HRIRs. The use of visual measurements for enhancing users’ auditory experience merits investigation with additional participants.
A statistical technique for identifying critical, dependent and redundant articulators in English phones was applied to 1D and 2D distributions of articulatograph coordinates. Results compared well with phonetic descriptions from the IPA chart with some interesting findings for fricatives and alveolar stops. An extension of the method is discussed.
Representing a complex acoustic scene with audio objects is desirable but challenging in object-based spatial audio production and reproduction, especially when concurrent sound signals are present in the scene. Source separation (SS) provides a potentially useful and enabling tool for audio object extraction. These extracted objects are often remixed to reconstruct a sound field in the reproduction stage. A suitable SS method is expected to produce audio objects that ultimately deliver high quality audio after remix. The performance of these SS algorithms therefore needs to be evaluated in this context. Existing metrics for SS performance evaluation, however, do not take into account the essential sound field reconstruction process. To address this problem, here we propose a new SS evaluation method which employs a remixing strategy similar to the panning law, and provides a framework to incorporate the conventional SS metrics. We have tested our proposed method on real-room recordings processed with four SS methods, including two state-of-the art blind source separation (BSS) methods and two classic beamforming algorithms. The evaluation results based on three conventional SS metrics are analysed.
At the University of Surrey (Guildford, UK), we have brought together research groups in different disciplines, with a shared interest in audio, to work on a range of collaborative research projects. In the Centre for Vision, Speech and Signal Processing (CVSSP) we focus on technologies for machine perception of audio scenes; in the Institute of Sound Recording (IoSR) we focus on research into human perception of audio quality; the Digital World Research Centre (DWRC) focusses on the design of digital technologies; while the Centre for Digital Economy (CoDE) focusses on new business models enabled by digital technology. This interdisciplinary view, across different traditional academic departments and faculties, allows us to undertake projects which would be impossible for a single research group. In this poster we will present an overview of some of these interdisciplinary projects, including projects in spatial audio, sound scene and event analysis, and creative commons audio.
Decomposition of speech signals into simultaneous streams of periodic and aperiodic information has been successfully applied to speech analysis, enhancement, modification and recently recognition. This paper examines the effect of different weightings of the two streams in a conventional HMM system in digit recognition tests on the Aurora 2.0 database. Comparison of the results from using matched weights during training showed a small improvement of approximately 10% relative to unmatched ones, under clean test conditions. Principal component analysis of the covariation amongst the periodic and aperiodic features indicated that only 45 (51) of the 78 coefficients were required to account for 99% of the variance, for clean (multi-condition) training, which yielded an 18.4% (10.3%) absolute increase in accuracy with respect to the baseline. These findings provide further evidence of the potential for harmonically-decomposed streams to improve performance and substantially to enhance recognition accuracy in noise.
We propose a new method for source separation by synthesizing the source from a speech mixture corrupted by various environmental noise. Unlike traditional source separation methods which estimate the source from the mixture as a replica of the original source (e.g. by solving an inverse problem), our proposed method is a synthesis-based approach which aims to generate a new signal (i.e. “fake” source) that sounds similar to the original source. The proposed system has an encoder-decoder topology, where the encoder predicts intermediate-level features from the mixture, i.e. Mel-spectrum of the target source, using a hybrid recurrent and hourglass network, while the decoder is a state-of-the-art WaveNet speech synthesis network conditioned on the Mel-spectrum, which directly generates time-domain samples of the sources. Both objective and subjective evaluations were performed on the synthesized sources, and show great advantages of our proposed method for high-quality speech source separation and generation.
In this paper we propose a pipeline for estimating acoustic 3D room structure with geometry and attribute prediction using spherical 360° cameras. Instead of setting microphone arrays with loudspeakers to measure acoustic parameters for specific rooms, a simple and practical single-shot capture of the scene using a stereo pair of 360° cameras can be used to simulate those acoustic parameters. We assume that the room and objects can be represented as cuboids aligned to the main axes of the room coordinate (Manhattan world). The scene is captured as a stereo pair using off-the-shelf consumer spherical 360 cameras. A cuboid-based 3D room geometry model is estimated by correspondence matching between captured images and semantic labelling using a convolutional neural network (SegNet). The estimated geometry is used to produce frequency-dependent acoustic predictions of the scene. This is, to our knowledge, the first attempt in the literature to use visual geometry estimation and object classification algorithms to predict acoustic properties. Results are compared to measurements through calculated reverberant spatial audio object parameters used for reverberation reproduction customized to the given loudspeaker set up.
The challenge of installing and setting up dedicated spatial audio systems can make it difficult to deliver immersive listening experiences to the general public. However, the proliferation of smart mobile devices and the rise of the Internet of Things mean that there are increasing numbers of connected devices capable of producing audio in the home. ____Media device orchestration" (MDO) is the concept of utilizing an ad hoc set of devices to deliver or augment a media experience. In this paper, the concept is evaluated by implementing MDO for augmented spatial audio reproduction using objectbased audio with semantic metadata. A thematic analysis of positive and negative listener comments about the system revealed three main categories of response: perceptual, technical, and content-dependent aspects. MDO performed particularly well in terms of immersion/envelopment, but the quality of listening experience was partly dependent on loudspeaker quality and listener position. Suggestions for further development based on these categories are given.
Probabilistic models of binaural cues, such as the interaural phase difference (IPD) and the interaural level difference (ILD), can be used to obtain the audio mask in the time-frequency (TF) domain, for source separation of binaural mixtures. Those models are, however, often degraded by acoustic noise. In contrast, the video stream contains relevant information about the synchronous audio stream that is not affected by acoustic noise. In this paper, we present a novel method for modeling the audio-visual (AV) coherence based on dictionary learning. A visual mask is constructed from the video signal based on the learnt AV dictionary, and incorporated with the audio mask to obtain a noise-robust audio-visual mask, which is then applied to the binaural signal for source separation. We tested our algorithm on the XM2VTS database, and observed considerable performance improvement for noise corrupted signals.
Proceedings of the ICA 2019 and EAA Euroregio : 23rd International Congress on Acoustics, integrating 4th EAA Euroregio 2019 : 9-13 September 2019, Aachen, Germany / proceedings editor: Martin Ochmann, Michael Vorländer, Janina Fels 23rd International Congress on Acoustics, integrating 4th EAA Euroregio 2019, ICA 2019, Aachen, Germany, 9 Sep 2019 - 13 Sep 2019; Aachen (2019). Published by Aachen
For many audio applications, availability of recorded multi-channel room impulse responses (MC-RIRs) is fundamental. They enable development and testing of acoustic systems for reflective rooms. We present multiple MC-RIR datasets recorded in diverse rooms, using up to 60 loudspeaker positions and various uniform compact microphone arrays. These datasets complement existing RIR libraries and have dense spatial sampling of a listening position. To reveal the encapsulated spatial information, several state of the art room visualization methods are presented. Results confirm the measurement fidelity and graphically depict the geometry of the recorded rooms. Further investigation of these recordings and visualization methods will facilitate object-based RIR encoding, integration of audio with other forms of spatial information, and meaningful extrapolation and manipulation of recorded compact microphone array RIRs.
A multiplanar Dynamic Magnetic Resonance Imaging (MRI) technique that extends our earlier work on single-plane Dynamic MRI is described. Scanned images acquired while an utterasne is repeated are recombined to form pseudo-time-varying images of the vocal tract using a simultaneously recorded audio signal. There is no technical limit on the utterance length or number of slices that can be so imaged, though the number of repetitions required may be limited by the subject's stamina. An example of [pasi] imaged in three sagittal planes is shown; with a Signa GE 0.5T MR scanner, 360 tokens were reconstructed to form a sequence of 39 3-slice 16ms frames. From these, a 3-D volume was generated for each time frame, and tract surfaces outlined manually. Parameters derived from these include: palate-tongue distances for [a,s,i]; estimates of tongue volume and of the area function using only the midsagittal, and then all three slices. These demonstrate the accuracy and usefulness of the technique.
Recent advances in human-computer interaction technology go beyond the successful transfer of data between human and machine by seeking to improve the naturalness and friendliness of user interactions. An important augmentation, and potential source of feedback, comes from recognizing the user‘s expressed emotion or affect. This chapter presents an overview of research efforts to classify emotion using different modalities: audio, visual and audio-visual combined. Theories of emotion provide a framework for defining emotional categories or classes. The first step, then, in the study of human affect recognition involves the construction of suitable databases. The authorsdescribe fifteen audio, visual and audio-visual data sets, and the types of feature that researchers have used to represent the emotional content. They discuss data-driven methods of feature selection and reduction, which discard noise and irrelevant information to maximize the concentration of useful information. They focus on the popular types of classifier that are used to decide to which emotion class a given example belongs, and methods of fusing information from multiple modalities. Finally, the authors point to some interesting areas for future investigation in this field, and conclude.
Apparatus and methods are disclosed for performing object-based audio rendering on a plurality of audio objects which define a sound scene, each audio object comprising at least one audio signal and associated metadata. The apparatus comprises: a plurality of renderers each capable of rendering one or more of the audio objects to output rendered audio data; and object adapting means for adapting one or more of the plurality of audio objects for a current reproduction scenario, the object adapting means being configured to send the adapted one or more audio objects to one or more of the plurality of renderers.
AbstractAs personalised immersive display systems have been intensely explored in virtual reality (VR), plausible 3D audio corresponding to the visual content is required to provide more realistic experiences to users. It is well known that spatial audio synchronised with visual information improves a sense of immersion but limited research progress has been achieved in immersive audio-visual content production and reproduction. In this paper, we propose an end-to-end pipeline to simultaneously reconstruct 3D geometry and acoustic properties of the environment from a pair of omnidirectional panoramic images. A semantic scene reconstruction and completion method using a deep convolutional neural network is proposed to estimate the complete semantic scene geometry in order to adapt spatial audio reproduction to the scene. Experiments provide objective and subjective evaluations of the proposed pipeline for plausible audio-visual VR reproduction of real scenes.
The visual and auditory modalities are the most important stimuli for humans. In order to maximise the sense of immersion in VR environments, a plausible spatial audio reproduction synchronised with visual information is essential. However, measuring acoustic properties of an environment using audio equipment is a complicated process. In this chapter, we introduce a simple and efficient system to estimate room acoustic for plausible spatial audio rendering using 360 ∘ cameras for real scene reproduction in VR. A simplified 3D semantic model of the scene is estimated from captured images using computer vision algorithms and convolutional neural network (CNN). Spatially synchronised audio is reproduced based on the estimated geometric and acoustic properties in the scene. The reconstructed scenes are rendered with synthesised spatial audio.
This paper presents a new method for reverberant speech separation, based on the combination of binaural cues and blind source separation (BSS) for the automatic classification of the time-frequency (T-F) units of the speech mixture spectrogram. The main idea is to model interaural phase difference, interaural level difference and frequency bin-wise mixing vectors by Gaussian mixture models for each source and then evaluate that model at each T-F point and assign the units with high probability to that source. The model parameters and the assigned regions are refined iteratively using the Expectation-Maximization (EM) algorithm. The proposed method also addresses the permutation problem of the frequency domain BSS by initializing the mixing vectors for each frequency channel. The EM algorithm starts with binaural cues and after a few iterations the estimated probabilistic mask is used to initialize and re-estimate the mix- ing vector model parameters. We performed experiments on speech mixtures, and showed an average of about 0.8 dB improvement in signal-to-distortion (SDR) over the binaural-only baseline
Studies on sound field control methods able to create independent listening zones in a single acoustic space have recently been undertaken due to the potential of such methods for various practical applications, such as individual audio streams in home entertainment. Existing solutions to the problem have shown to be effective in creating high and low sound energy regions under anechoic conditions. Although some case studies in a reflective environment can also be found, the capabilities of sound zoning methods in rooms have not been fully explored. In this paper, the influence of low-order (early) reflections on the performance of key sound zone techniques is examined. Analytic considerations for small-scale systems reveal strong dependence of performance on parameters such as source positioning with respect to zone locations and room surfaces, as well as the parameters of the receiver configuration. These dependencies are further investigated through numerical simulation to determine system configurations which maximize the performance in terms of acoustic contrast and array control effort. The design rules for source and receiver positioning are suggested, for improved performance under a given set of constraints such as a number of available sources, zone locations, and the direction of the dominant reflection.